2076 results in Statistical Physics
Chapter 1 - Introduction
-
-
- Book:
- A Complex Systems Approach to Epilepsy
- Published online:
- 06 January 2023
- Print publication:
- 26 January 2023, pp 1-4
-
- Chapter
- Export citation
Index
-
- Book:
- A Complex Systems Approach to Epilepsy
- Published online:
- 06 January 2023
- Print publication:
- 26 January 2023, pp 153-160
-
- Chapter
- Export citation
Chapter 2 - Systems Biology Approaches to the Genetic Complexity of Epilepsy
-
-
- Book:
- A Complex Systems Approach to Epilepsy
- Published online:
- 06 January 2023
- Print publication:
- 26 January 2023, pp 5-18
-
- Chapter
- Export citation
Chapter 6 - The Baseline and Epileptiform EEG
-
-
- Book:
- A Complex Systems Approach to Epilepsy
- Published online:
- 06 January 2023
- Print publication:
- 26 January 2023, pp 72-85
-
- Chapter
- Export citation
Chapter 4 - Phenomenological Mesoscopic Models for Seizure Activity
-
-
- Book:
- A Complex Systems Approach to Epilepsy
- Published online:
- 06 January 2023
- Print publication:
- 26 January 2023, pp 41-60
-
- Chapter
- Export citation
Contents
-
- Book:
- A Complex Systems Approach to Epilepsy
- Published online:
- 06 January 2023
- Print publication:
- 26 January 2023, pp v-v
-
- Chapter
- Export citation
Copyright page
-
- Book:
- A Complex Systems Approach to Epilepsy
- Published online:
- 06 January 2023
- Print publication:
- 26 January 2023, pp iv-iv
-
- Chapter
- Export citation
Contributors
-
- Book:
- A Complex Systems Approach to Epilepsy
- Published online:
- 06 January 2023
- Print publication:
- 26 January 2023, pp vi-viii
-
- Chapter
- Export citation
Chapter 7 - Neuronal Approaches to Epilepsy
-
-
- Book:
- A Complex Systems Approach to Epilepsy
- Published online:
- 06 January 2023
- Print publication:
- 26 January 2023, pp 86-98
-
- Chapter
- Export citation
Chapter 5 - Personalized Network Modeling in Epilepsy
-
-
- Book:
- A Complex Systems Approach to Epilepsy
- Published online:
- 06 January 2023
- Print publication:
- 26 January 2023, pp 61-71
-
- Chapter
- Export citation
Chapter 8 - Mapping Epileptic Networks with Scalp and Invasive EEG
-
-
- Book:
- A Complex Systems Approach to Epilepsy
- Published online:
- 06 January 2023
- Print publication:
- 26 January 2023, pp 99-126
-
- Chapter
- Export citation
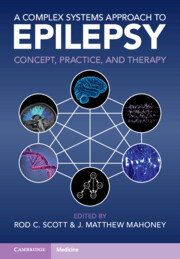
A Complex Systems Approach to Epilepsy
- Concept, Practice, and Therapy
-
- Published online:
- 06 January 2023
- Print publication:
- 26 January 2023
Chapter Five - Percolation on ℤ𝑑
-
- Book:
- Network Models for Data Science
- Published online:
- 22 March 2023
- Print publication:
- 05 January 2023, pp 105-135
-
- Chapter
- Export citation
Subject Index
-
- Book:
- Network Models for Data Science
- Published online:
- 22 March 2023
- Print publication:
- 05 January 2023, pp 477-484
-
- Chapter
- Export citation
Chapter Six - Percolation Beyond ℤ𝑑
-
- Book:
- Network Models for Data Science
- Published online:
- 22 March 2023
- Print publication:
- 05 January 2023, pp 136-160
-
- Chapter
- Export citation
Chapter Sixteen - Graphons as Limits of Networks
-
- Book:
- Network Models for Data Science
- Published online:
- 22 March 2023
- Print publication:
- 05 January 2023, pp 393-410
-
- Chapter
- Export citation
Chapter Fifteen - Examining Network Properties
-
- Book:
- Network Models for Data Science
- Published online:
- 22 March 2023
- Print publication:
- 05 January 2023, pp 374-392
-
- Chapter
- Export citation
Index of Examples
-
- Book:
- Network Models for Data Science
- Published online:
- 22 March 2023
- Print publication:
- 05 January 2023, pp 475-476
-
- Chapter
- Export citation
Chapter Ten - Parametric Network Models
-
- Book:
- Network Models for Data Science
- Published online:
- 22 March 2023
- Print publication:
- 05 January 2023, pp 239-271
-
- Chapter
- Export citation
Dedication
-
- Book:
- Network Models for Data Science
- Published online:
- 22 March 2023
- Print publication:
- 05 January 2023, pp v-vi
-
- Chapter
- Export citation