2076 results in Statistical Physics
Preface
-
- Book:
- Complexity Science
- Published online:
- 13 December 2022
- Print publication:
- 17 November 2022, pp xvii-xx
-
- Chapter
- Export citation
References
-
- Book:
- Complexity Science
- Published online:
- 13 December 2022
- Print publication:
- 17 November 2022, pp 411-435
-
- Chapter
- Export citation
5 - Branching Processes
- from II - Mathematical Tools of Complexity Science
-
- Book:
- Complexity Science
- Published online:
- 13 December 2022
- Print publication:
- 17 November 2022, pp 93-109
-
- Chapter
- Export citation
Acknowledgements
-
- Book:
- Complexity Science
- Published online:
- 13 December 2022
- Print publication:
- 17 November 2022, pp xi-xii
-
- Chapter
- Export citation
8 - Network Theory
- from II - Mathematical Tools of Complexity Science
-
- Book:
- Complexity Science
- Published online:
- 13 December 2022
- Print publication:
- 17 November 2022, pp 177-229
-
- Chapter
- Export citation
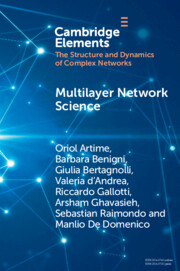
Multilayer Network Science
- From Cells to Societies
-
- Published online:
- 23 August 2022
- Print publication:
- 15 September 2022
-
- Element
- Export citation
Contents
-
- Book:
- The Statistical Mechanics of Irreversible Phenomena
- Published online:
- 14 July 2022
- Print publication:
- 28 July 2022, pp vii-xx
-
- Chapter
- Export citation
2 - Statistical Mechanics
-
- Book:
- The Statistical Mechanics of Irreversible Phenomena
- Published online:
- 14 July 2022
- Print publication:
- 28 July 2022, pp 41-98
-
- Chapter
- Export citation
11 - Reactions
-
- Book:
- The Statistical Mechanics of Irreversible Phenomena
- Published online:
- 14 July 2022
- Print publication:
- 28 July 2022, pp 398-437
-
- Chapter
- Export citation
Dedication
-
- Book:
- The Statistical Mechanics of Irreversible Phenomena
- Published online:
- 14 July 2022
- Print publication:
- 28 July 2022, pp v-vi
-
- Chapter
- Export citation
Frontmatter
-
- Book:
- The Statistical Mechanics of Irreversible Phenomena
- Published online:
- 14 July 2022
- Print publication:
- 28 July 2022, pp i-iv
-
- Chapter
- Export citation
Appendix F - Complements on Fluctuation Relations
-
- Book:
- The Statistical Mechanics of Irreversible Phenomena
- Published online:
- 14 July 2022
- Print publication:
- 28 July 2022, pp 620-629
-
- Chapter
- Export citation
14 - Quantum Statistical Mechanics
-
- Book:
- The Statistical Mechanics of Irreversible Phenomena
- Published online:
- 14 July 2022
- Print publication:
- 28 July 2022, pp 494-519
-
- Chapter
- Export citation
15 - Transport in Open Quantum Systems
-
- Book:
- The Statistical Mechanics of Irreversible Phenomena
- Published online:
- 14 July 2022
- Print publication:
- 28 July 2022, pp 520-564
-
- Chapter
- Export citation
4 - Stochastic Processes
-
- Book:
- The Statistical Mechanics of Irreversible Phenomena
- Published online:
- 14 July 2022
- Print publication:
- 28 July 2022, pp 147-201
-
- Chapter
- Export citation
Preface
-
- Book:
- The Statistical Mechanics of Irreversible Phenomena
- Published online:
- 14 July 2022
- Print publication:
- 28 July 2022, pp xxi-xxiv
-
- Chapter
- Export citation
5 - Fluctuation Relations for Energy and Particle Fluxes
-
- Book:
- The Statistical Mechanics of Irreversible Phenomena
- Published online:
- 14 July 2022
- Print publication:
- 28 July 2022, pp 202-256
-
- Chapter
- Export citation
12 - Active Processes
-
- Book:
- The Statistical Mechanics of Irreversible Phenomena
- Published online:
- 14 July 2022
- Print publication:
- 28 July 2022, pp 438-468
-
- Chapter
- Export citation
7 - Driven Brownian Particles and Related Systems
-
- Book:
- The Statistical Mechanics of Irreversible Phenomena
- Published online:
- 14 July 2022
- Print publication:
- 28 July 2022, pp 296-318
-
- Chapter
- Export citation
Appendix B - Complements on Dynamical Systems Theory
-
- Book:
- The Statistical Mechanics of Irreversible Phenomena
- Published online:
- 14 July 2022
- Print publication:
- 28 July 2022, pp 577-590
-
- Chapter
- Export citation