Book contents
- Frontmatter
- Contents
- Preface
- 1 Introduction
- 2 Theory of Tests, p-Values, and Confidence Intervals
- 3 From Scientific Theory to Statistical Hypothesis Test
- 4 One-Sample Studies with Binary Responses
- 5 One-Sample Studies with Ordinal or Numeric Responses
- 6 Paired Data
- 7 Two-Sample Studies with Binary Responses
- 8 Assumptions and Hypothesis Tests
- 9 Two-Sample Studies with Ordinal or Numeric Responses
- 10 General Methods for Frequentist Inferences
- 11 k-Sample Studies and Trend Tests
- 12 Clustering and Stratification
- 13 Multiplicity in Testing
- 14 Testing from Models
- 15 Causality
- 16 Censoring
- 17 Missing Data
- 18 Group Sequential and Related Adaptive Methods
- 19 Testing Fit, Equivalence, and Noninferiority
- 20 Power and Sample Size
- 21 Bayesian Hypothesis Testing
- References
- Notation Index
- Concept Index
- References
References
Published online by Cambridge University Press: 17 April 2022
- Frontmatter
- Contents
- Preface
- 1 Introduction
- 2 Theory of Tests, p-Values, and Confidence Intervals
- 3 From Scientific Theory to Statistical Hypothesis Test
- 4 One-Sample Studies with Binary Responses
- 5 One-Sample Studies with Ordinal or Numeric Responses
- 6 Paired Data
- 7 Two-Sample Studies with Binary Responses
- 8 Assumptions and Hypothesis Tests
- 9 Two-Sample Studies with Ordinal or Numeric Responses
- 10 General Methods for Frequentist Inferences
- 11 k-Sample Studies and Trend Tests
- 12 Clustering and Stratification
- 13 Multiplicity in Testing
- 14 Testing from Models
- 15 Causality
- 16 Censoring
- 17 Missing Data
- 18 Group Sequential and Related Adaptive Methods
- 19 Testing Fit, Equivalence, and Noninferiority
- 20 Power and Sample Size
- 21 Bayesian Hypothesis Testing
- References
- Notation Index
- Concept Index
- References
Summary
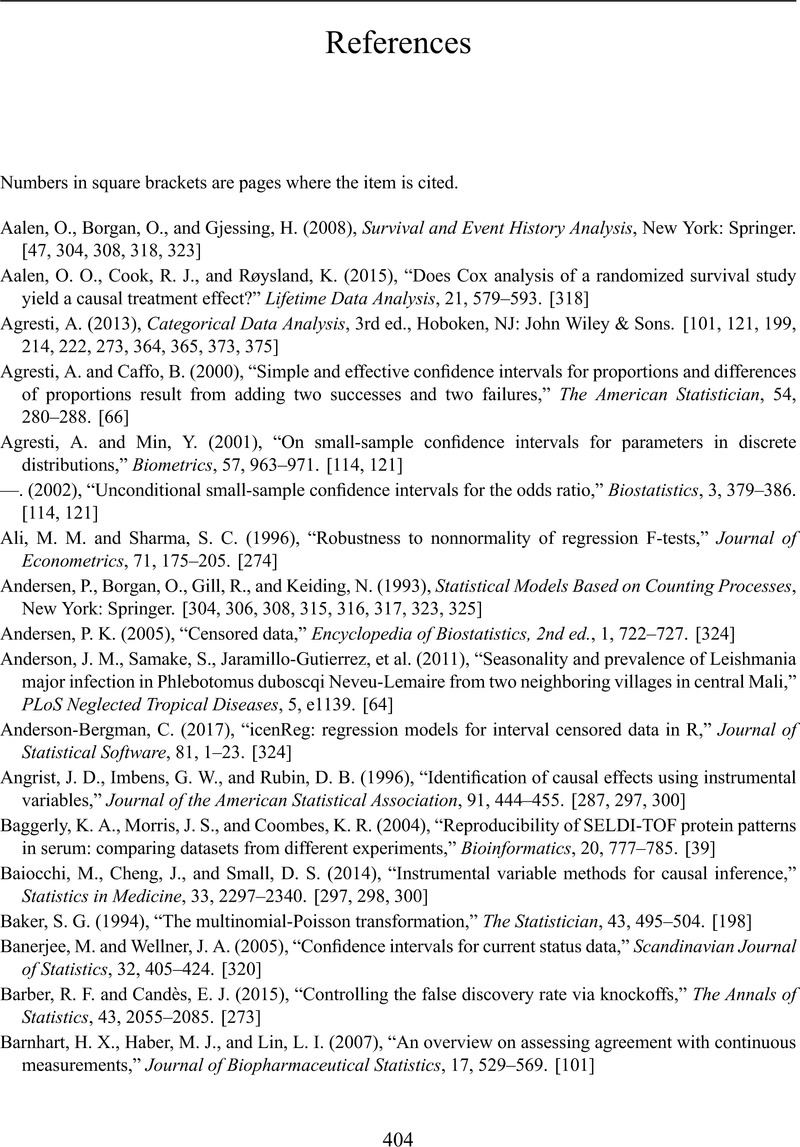
- Type
- Chapter
- Information
- Statistical Hypothesis Testing in ContextReproducibility, Inference, and Science, pp. 404 - 419Publisher: Cambridge University PressPrint publication year: 2022