1. Introduction
Mental representations remain the central posits of psychology after many decades of scrutiny. But what are mental representations and what forms do they take in nature? In other words, what is the format of thought? This paper revisits an old answer to this question: The language-of-thought hypothesis (LoTH).
LoTH is liable to evoke memories of the previous century: Foundational discussions about the structure of thought in the 1970s, the rise of connectionism in the 1980s, and debates about systematicity and productivity in the 1990s. Now, well into the twenty-first century, it might seem that LoTH is a relic, like Freud's tripartite cognitive architecture or Skinnerian behaviorism – a topic of historical interest, but no longer at the center of scientific or philosophical inquiry into the mind.
We will argue for the opposite view: In the half century since Fodor's (Reference Fodor1975) foundational discussion, the case for the LoTH has only grown stronger over time. The chief aim of this paper is to showcase LoTH's explanatory breadth and power in light of recent developments in cognitive science. Computational cognitive science, comparative and developmental psychology, social psychology, and perceptual psychology have all advanced independently, yet evidence from these disparate fields points to the same overall picture: Contemporary cognitive science presupposes the language-of-thought (LoT).
The theoretical literature on LoTH is massive and extremely important for understanding the hypothesis and its historical roots. Given space constraints, we will have to ignore huge portions of this literature. We aim simply to provide the strongest article-sized empirical case for LoTH. As a result, we're forced to ignore a great deal of empirical evidence in favor of LoTH. Work in syntax, semantics, psycholinguistics, and philosophy-of-mind has often been taken to bolster LoTH (Fodor, Reference Fodor1975, Reference Fodor1987). Although the relevance of linguistics (broadly construed) to LoTH remains strong, we situate largely independent forms of evidence at the center of our case. We focus primarily on areas (e.g., perception, system-1 reasoning, animal cognition) that seem less language-like. If even these apparent problem areas offer evidence for LoTH, then we should be optimistic about finding evidence for LoTH throughout much of the mind.
In section 2, we specify which systems of representation count as LoTs. Some of the conclusions of this section will be a bit surprising, as the natural inferences one should draw from the standard characterization of LoTH have largely been ignored since the view's inception. Then, in sections 3–6, we marshal evidence for LoTH from across the cognitive sciences. Section 3 reviews recent LoT-based developments in computational cognitive science, section 4 surveys a mass of data from the study of human perception, section 5 considers evidence from developmental and comparative psychology, and section 6 examines evidence from social psychology.
We think that LoTH is indispensable to a computational account of the mind. But the empirical case for the view does not stem from the idea that LoTH is the “only game in town,” which it is not (and never really was). Instead, we contend, LoTH is the best game in town. For a wide variety of phenomena, it does the best job of explaining why biological minds work in the peculiar ways they do.
Our defense of LoTH doesn't presuppose a single, large-scale opponent. Broadly speaking, our opponents are reductionists of various stripes, for example, traditional neural reductionists (Bickle, Reference Bickle2003; Churchland, Reference Churchland1981), theorists who reduce LoT-like cognition to natural language (Berwick & Chomsky, Reference Berwick and Chomsky2016; Hinzen & Sheehan, Reference Hinzen and Sheehan2013), critics of representationalism (Hutto & Myin, Reference Hutto and Myin2013; Schwitzgebel, Reference Schwitzgebel and Nottelman2013), associationists (Dickinson, Reference Dickinson2012; Papineau, Reference Papineau2003; Rydell & McConnell, Reference Rydell and McConnell2006), and most prominently in recent years, reductionist deep-learning approaches (LeCun, Bengio, & Hinton, Reference LeCun, Bengio and Hinton2015).Footnote 1 However, with the exception of deep neural networks (DNNs), we will mostly avoid direct engagement with these views – not because they are not of interest, but because the best counter to reductionism is simply to demonstrate the explanatory successes of LoT-like representational structures. In the context of system-1 cognition, for example, our primary opponents will be associationists; in the context of perception science, where associationism is less prominent, our foil will be rival iconic/imagistic formats. This focus on multiple corners of cognitive science will demonstrate two rare virtues of LoTH: Its unificatory power across disciplines and its generalizability across content domains.
2. What is a language-of-thought?
Classic defenses of LoTH often equated it with the view that mental representations are structured (Fodor, Reference Fodor1987; Fodor & Pylyshyn, Reference Fodor and Pylyshyn1988). The route from this identification to the “Only Game in Town” argument is simple – mental representations must have some sort of structure for computational explanations to succeed, and if LoTH follows from that simple fact, it's hard to envision viable alternatives. Arguably, this emphasis on structure per se was influenced by the idea that the primary alternatives to LoTH were connectionist models that lacked structured representations altogether (Rumelhart & McClelland, Reference Rumelhart and McClelland1986; cf. Smolensky, Reference Smolensky1990).
However, we don't assume this dialectic here. The main reason is that we think there are structured (i.e., nonatomic) representations couched in non-LoT-like formats. Iconic representations are perhaps the clearest example. Operations like mental rotation (Shepard & Metzler, Reference Shepard and Metzler1971) and scanning (Kosslyn, Ball, & Reiser, Reference Kosslyn, Ball and Reiser1978) are inexplicable without appeal to structured representations, but at least some of those representations seem to have an iconic, rather than LoT-like, representational format (Carey, Reference Carey2009; Fodor, Reference Fodor, McLaughlin and Cohen2007; Kosslyn, Reference Kosslyn1980; Quilty-Dunn, Reference Quilty-Dunn2020b; Toribio, Reference Toribio2011; cf. Pylyshyn, Reference Pylyshyn2002). Other potential formats include analog magnitudes (Carey, Reference Carey2009; Clarke, Reference Clarke2022; Clarke & Beck, Reference Clarke and Beck2021; Mandelbaum, Reference Mandelbaum2013; Meck & Church, Reference Meck and Church1983), vectors in multidimensional similarity spaces (Gauker, Reference Gauker2011), mental maps (Camp, Reference Camp2007; Rescorla, Reference Rescorla2009; Shea, Reference Shea2018; Tolman, Reference Tolman1948), mental models (Johnson-Laird, Reference Johnson-Laird2006), graphical models (Danks, Reference Danks2014), semantic pointers (Eliasmith, Reference Eliasmith2013), pattern-separated representations (Yassa & Stark, Reference Yassa and Stark2011; cf. Quiroga, Reference Quiroga2020), neural representations at various scales (Barack & Krakauer, Reference Barack and Krakauer2021), and much more. We're happy to let a thousand representational formats bloom.
We take LoTH to describe a representational format with six distinctive properties beyond merely having structure. Many, perhaps all, of these properties are not necessary for a representational scheme to count as an LoT, and some may be shared with other formats. We regard these properties as (somewhat) independent axes on which a format can be assessed for how LoT-like it is. If LoT is a natural kind, then these properties should cluster together homeostatically – that is, if some properties are instantiated, it raises the probability that others are as well (Boyd, Reference Boyd and Wilson1999). These six features each expand the expressive power of abstract, domain-general cognition, making it advantageous for them to evolve as a cluster. We also suspect there might be distinct LoTs with only partially overlapping properties, perhaps arising in different species or different systems within the same mind. The properties adumbrated here don't necessarily exhaust the characterization of LoTH. The crux of the paper includes several sections devoted to empirical evidence, and a fuller picture of LoTH will emerge throughout.
Before moving to the list of core LoT properties, some caveats about how our approach differs from classic defenses of LoTH. First, although LoTH is sometimes understood as the hypothesis that mental representations have the same structure as natural language, this is not our strategy. Although some theorists have posited LoT to explain natural-language processing and even play a constitutive role in the compositional semantics of natural language (Fodor, Reference Fodor1987; Pinker, Reference Pinker1994), our plan is to search for LoTs outside natural-language-guided contexts. We will examine LoT-like structures that are less connected to natural language and thus represent stringent test cases for LoTH: Mid-level vision, nonverbal minds, and system-1 cognition. LoTH as we'll defend it is committed to representational formats that are language-like in some broad respects, but independent characterizations are provided by both the logical character of LoT (i.e., the way it resembles formal languages that may be radically unlike natural language) and the previous theoretical literature on LoTH, which commits to certain distinctive features. As long as one agrees that an important class of mental representations has many or all of these features, there is no need to quibble about the analogy to natural language.
Second, we will avoid direct discussion of two features of thought that have dominated earlier discussions, namely, systematicity and productivity (Fodor & Pylyshyn, Reference Fodor and Pylyshyn1988). We agree with the widespread view that any format worth calling an LoT must not only have structure, but it must be compositional: It must include complex representations that are a function of simple elements plus their mode of combination (cf. Szabo, Reference Szabo, Hinzen, Machery and Werning2011). But as Camp (Reference Camp2007) and others argue, this feature is arguably present in various representational forms, including maps, and thus is not sufficient for ensuring an LoT. Compositionality that is fully systematic and productive is very good evidence for LoT-like architectures, but we want to leave open whether some of the LoT-like structures we'll explore are fully systematic and productive. As a historical note, this caveat is in keeping with earlier discussions, in which systematicity and productivity were each considered “a contingent feature of thought” (Fodor, Reference Fodor1987, p. 152) that evidences LoTH rather than a constitutive requirement. This caveat also dovetails with the previous one about relaxing the analogy with natural language – while, for example, recursive productivity might be a key feature of natural language (Chomsky, Reference Chomsky2017), we allow that some LoT-based systems may fail to be recursive. Finally, although we believe systematicity and productivity were good arguments for LoTH, the nature of these cognitive features and their presence in biological minds, including nonverbal ones, is well-trodden ground (Camp, Reference Camp2009; Carruthers, Reference Carruthers and Lurz2009). Because our goal is to point in new directions for LoTH, we will invoke systematicity and productivity sparingly, mostly keeping instead to the six core properties listed below. These properties are intended to capture the spirit of earlier presentations of LoTH – a combinatorial, symbolic representational format that facilitates logical, structure-sensitive operations (Fodor & Pylyshyn, Reference Fodor and Pylyshyn1988) – while framing an updated discussion more closely tied to contemporary experimental research.
Property 1: Discrete constituents. Typical iconic representations holistically encode features and individuals (Fodor, Reference Fodor, McLaughlin and Cohen2007; Hummel, Reference Hummel and Reisburg2013; Kosslyn, Thompson, & Ganis, Reference Kosslyn, Thompson and Ganis2006), while LoT representations comprise distinct constituents corresponding to individuals and their separable features. In a sentence like “That is a pink square object,” the predicate “square” can be deleted without any other constituents being deleted. In an iconic representation of a pink square, the relationship between the individual, its color, and its shape is more intertwined. “Pink square” can be the output of a merge operation (Chomsky, Reference Chomsky1995) while the part of the icon that represents pink and the part that represents square are one and the same.
Property 2: Role-filler independence. LoT architectures have a distinctive syntax: They combine constituents in a way that maintains independence between syntactic roles and the constituents that fill them (Frankland & Greene, Reference Frankland and Greene2020; Hummel, Reference Hummel2011; Martin & Doumas, Reference Martin and Doumas2020). The role agent is present in “John loves Mary” and “Mary loves John.” The identity of the role is independent of what fills it (“Mary,” “John”). Likewise, each constituent maintains its identity independent of its current role (“John” can be agent or patient). Role-filler independence captures the rule-based syntactic characteristics of LoT-like compositionality: The syntactic structure is typed independently of its particular constituents, and the constituents are typed independently of how they happen to compose on a particular occasion. In map-like representations, for example, changing the spatial position of a marker changes not only the putative “predicate” (e.g., tree) but also the spatial content of the marker (e.g., its position relative to other map elements); thus maps fail to exhibit full role-filler independence (Kulvicki, Reference Kulvicki2015). Similarly, connectionist models that bind contents through tensor products (Eliasmith, Reference Eliasmith2013; Palangi, Smolensky, He, & Deng, Reference Palangi, Smolensky, He and Deng2018; Smolensky, Reference Smolensky1990) can simulate compositionality, but fail to preserve identity of the original representational elements; thus they sacrifice role-filler independence, and with it classical compositionality (Eliasmith, Reference Eliasmith2013, p. 125ff; Hummel, Reference Hummel2011).
Role-filler independence might seem similar to the property of having discrete constituents, but they're not equivalent. One could posit discrete constituents in an unordered set, for example, without positing a role that maintains its identity across multiple fillers. There's also nothing in the positing of discrete constituents per se that precludes the type-identity of those constituents from shifting in various contexts (e.g., GREEN APPLE and GREEN PEN might be complexes of discrete constituents, but the copresence of APPLE vs. PEN might change the identity of GREEN; Travis, Reference Travis2001).
Property 3: Predicate–argument structure. One distinctively LoT-like mode of combination is predication, in which a predicate is applied to an argument to yield a truth-evaluable structure. Simple sentences like “John smokes” and “Mary is tall” are paradigmatic examples. Other representational formats, such as images and maps, are assessable for accuracy, but often (perhaps always) fail to exhibit truth-evaluable predicate–argument structure (Camp, Reference Camp, Grzankowski and Montague2018; Kulvicki, Reference Kulvicki2015; Rescorla, Reference Rescorla2009). We'll usually interpret predicate–argument structure as requiring both discrete constituents and role-filler independence, that is, as requiring constituents that function as predicates and arguments but maintain type-identity, and as having predicative syntactic structures that can be operated on independently of the content of nonlogical constituents. Thus this condition is not merely that the system must be capable of expressing propositions like <John smokes> (a condition that can be met by even the simplest neural nets, where <John smokes> can be represented by an unstructured node), but rather that this predicate–argument structure is instantiated in the representational vehicle itself (see, e.g., Fodor, Reference Fodor1987).
Property 4: Logical operators. One hallmark of LoT architectures is the use of logical symbols like NOT, AND, OR, and IF. These operators are discrete constituents that compose into larger structures, a hallmark of LoT-like symbols more generally. Logical operators don't obviously presuppose subsentential LoT-like structure, because one could imagine appending such operators to otherwise unstructured formats, or to maps (Rescorla, Reference Rescorla2009). But they are one piece of an overall LoT-friendly picture, positing discrete constituents that allow for formal-syntactic operations. For example, consider an operation that runs from A-OR-B and NOT-A to B; even if A and B are atomic symbols or maps, their un-LoT-like properties are irrelevant because the operation is sensitive to the logical structure alone. Finding evidence for explicit, discrete logical operators should therefore increase our credence in LoTH, all else equal. We'll construe logical operators as requiring role-filler independence, in that, for example, negation operators are the same no matter what proposition they negate.
Property 5: Inferential promiscuity. LoT architectures have been useful in characterizing inferential transitions, especially logical inferences (Braine & O'Brien, Reference Braine and O'Brien1998; Fodor & Pylyshyn, Reference Fodor and Pylyshyn1988; Quilty-Dunn & Mandelbaum, Reference Quilty-Dunn and Mandelbaum2018a; Rips, Reference Rips1994; cf. Johnson-Laird, Reference Johnson-Laird2006). LoT-like representations should not only encode information, but they should be usable for inference in a way that is automatic and independent of natural language.Footnote 2 The automaticity point is important: The theories of logical inference just cited share an appeal to computational processes that transform representations with one logical form into representations with another logical form in accordance with rules that are built into the architecture (i.e., merely procedural, not explicitly represented, and thus not amenable to intervention from representational states; Quilty-Dunn & Mandelbaum, Reference Quilty-Dunn and Mandelbaum2018b). If these theories are even roughly on the right track, then we should find evidence for logical-form-sensitive computation outside conscious, controlled, natural-language-guided contexts.
Property 6: Abstract conceptual content. LoTH has historically been opposed to concept empiricism, the view that concepts are sensory-based (Barsalou, Reference Barsalou1999; Prinz, Reference Prinz2002). It is logically compatible with other core LoT properties that some LoTs might be modality-specific (e.g., different LoT symbol types and/or syntactic rules for each modality). But there is no a priori reason to expect that primitive LoT symbols – unlike, for example, iconic or analog formats – will be limited to a certain range of properties (e.g., sensory properties, the referents of simple concepts for classical empiricists). Thus we should expect (ceteris paribus) LoT symbols to represent abstract categories without representing specific details (e.g., a symbol that encodes bottle and no particular shape or color). There is therefore a nondemonstrative but bidirectional relationship between LoTs and abstract contents: Many LoTs should be expected to encode abstract content, and abstract content is naturally represented by means of discrete LoT-like symbols.
The hypothesis that these features cluster together generates nontrivial predictions. Once we've isolated a particular representation type, evidence for any two features (e.g., discrete constituents and abstract conceptual content) may look completely different. Nonetheless, LoTH predicts that these sorts of evidence should tend to cooccur. This cooccurrence would be surprising from a theory-neutral point of view, but not from the perspective of LoTH. We will use just this sort of clustering-based approach to mount an abductive, empirical argument for LoTH. We focus on independently identified systems to observe whether these six properties cluster in them: Perception, physical reasoning in infants and animals, and system-1 cognition.
3. LoTs in computational cognitive science
Before we turn to the bulk of our evidence, we first consider the status of LoTH in computational modeling – a topic of pressing concern as the advance of artificial intelligence (AI) has made LoT appear antiquated to some researchers. LoT-style models naturally grew out of symbolic computation (Fodor, Reference Fodor1975; Schneider, Reference Schneider2011; cf. Field, Reference Field1978; Harman, Reference Harman1973), including “GOFAI” (“Good Old-Fashioned Artificial Intelligence”; Haugeland, Reference Haugeland1985). As new computational methods arose that did not presuppose symbolic computation, such as connectionism with its subsymbolic elements, LoT-style architectures grew detractors. With recent successes of subsymbolic deep neural networks (DNNs) (e.g., Google AI's Google Translate, Deep Mind's success with AlphaFold at modeling protein structure and with AlphaZero and MuZero at dominating complex games; Schrittwieser et al., Reference Schrittwieser, Antonoglou, Hubert, Simonyan, Sifre, Schmitt and Silver2020), LoT-like architectures may appear obsolete.
However, LoT has seen a resurgence in a computational framework that has led to breakthroughs within cognitive science: Bayesianism. Because Bayesian models of cognition are based on probabilistic updating, they appear to present alternatives to LoTH, which posits logical inference. However, Bayesian computational psychology naturally complements LoT architectures (Erdogan, Yildirim, & Jacobs, Reference Erdogan, Yildirim and Jacobs2015; Goodman & Lassiter, Reference Goodman, Lassiter, Lappin and Fox2015; Goodman, Tenenbaum, Feldman, & Griffiths, Reference Goodman, Tenenbaum, Feldman and Griffiths2008b; Goodman, Tenenbaum, & Gerstenberg, Reference Goodman, Tenenbaum, Gerstenberg, Margolis and Laurence2015; Kemp, Reference Kemp2012; Overlan, Jacobs, & Piantadosi, Reference Overlan, Jacobs and Piantadosi2017; Piantadosi & Jacobs, Reference Piantadosi and Jacobs2016; Piantadosi, Tenenbaum, & Goodman, Reference Piantadosi, Tenenbaum and Goodman2012, Reference Piantadosi, Tenenbaum and Goodman2016; Ullman, Goodman, & Tenenbaum, Reference Ullman, Goodman and Tenenbaum2012; Yildirim & Jacobs, Reference Yildirim and Jacobs2015). Wedding probabilistic reasoning to symbolic system processing has led to the “probabilistic language-of-thought” (PLoT) (Goodman et al., Reference Goodman, Tenenbaum, Gerstenberg, Margolis and Laurence2015).
PLoTs share a core set of properties: A set of primitives with basic operations for their combination (such as the lambda calculus, e.g., Church from Goodman, Mansinghka, Roy, Bonawitz, & Tenenbaum, Reference Goodman, Mansinghka, Roy, Bonawitz, Tenenbaum, McAllester and Myllymaki2008a). Primitives correspond to atomic concepts, which are recursively combined to form concepts of arbitrary complexity (Fodor, Reference Fodor1998; Quilty-Dunn, Reference Quilty-Dunn2021). All one must do is define a set of primitives, and a set of rules for combination and the system is capable of constructing a potentially infinite string of well-formed formulae (Chomsky, Reference Chomsky1965).
Bayesianism adds probabilistic inference to the traditional LoT machinery. One way of accomplishing this is by having a likelihood function that is noisy (combining this with a preference for simplicity, either because it's explicitly specified as a prior for the system, or because it falls out as a function of other constraints). PLoTs are classical symbolic systems that display all the hallmarks of LoT architectures, such as discrete constituents, role-filler independence, predicate–argument structure, productive and systematic compositionality, and inferential promiscuity. They are also, however, flexible probabilistic computational programs, because all other aspects of symbol processing (e.g., how they are combined, which processes use them, which information gets updated for them, even their basic semantics) can be determined probabilistically.
Versions of the PLoT have made serious progress in a number of specific areas, for example, learning taxonomical hierarchical structures such as kinship (Katz, Goodman, Kersting, Kemp, & Tenenbaum, Reference Katz, Goodman, Kersting, Kemp and Tenenbaum2008; Kemp, Reference Kemp2012; Mollica & Piantadosi, Reference Mollica and Piantadosi2015), causality (Goodman, Ullman, & Tenenbaum, Reference Goodman, Ullman and Tenenbaum2011), number (Piantadosi et al., Reference Piantadosi, Tenenbaum and Goodman2012), analogical reasoning (Cheyette & Piantadosi, Reference Cheyette and Piantadosi2017), theory acquisition (Ullman et al., Reference Ullman, Goodman and Tenenbaum2012), programs (Liang, Jordan, & Klein, Reference Liang, Jordan and Klein2010), mapping sentences to logical form (Zettlemoyer & Collins, Reference Zettlemoyer and Collins2005), general Boolean concept learning (Goodman et al., Reference Goodman, Mansinghka, Roy, Bonawitz, Tenenbaum, McAllester and Myllymaki2008a, Reference Goodman, Tenenbaum, Feldman and Griffiths2008b), and moral rule learning (Nichols, Reference Nichols2021). The sheer breadth and depth of the Bayesian computational revolution itself provides strong evidence in favor of the viability of the LoT. Instead of computational psychology showing that the LoT is a stale theory of the past, it shows how robust, flexible, powerful, and necessary the LoT is in order to ground our computational cognitive science in a way that maps onto human data.
The models that best approximate one type of human concept learning (e.g., learning that a wudsy is the tallest object that is either blue or green) are ones where a fuller set of classical logical connectives are hard-coded as primitives. For instance, Piantadosi et al. (Reference Piantadosi, Tenenbaum and Goodman2016) taught participants Boolean and quantificational concepts, then built different LoT models in a lambda calculus and compared them to the human data (Fig. 1a). They found that the models that least resembled human performance tended to have the least LoT-like structure. Models that lacked built-in connectives and represented only primitive features or similarity to exemplars performed poorly, as did models that merely learned response biases and only represented TRUE and FALSE categorization judgments. LoTs built with a single connective from which all others are constructed (such as NAND or conjunctions of Horn clauses, disjunctions with at most one nonnegated disjunct) fared better, but not as well as LoTs with the full suite of Boolean operators (conjunction, disjunction, negation, conditional, and biconditional), which in turn were outperformed by models supplanted further with built-in (first-order) quantifiers.Footnote 3 Although wudsy is not an ordinary lexical concept, it is a learnable concept for humans and its acquisition is best modeled by an LoT-like architecture. Thus Piantadosi et al.'s findings provide an existence proof for the utility of LoT-like architectures in the acquisition of logically complex, nonlexical concepts.

Figure 1. (a) Participants draw inferences about the referent of novel terms like wudsy based on examples; reprinted from Piantadosi et al. (Reference Piantadosi, Tenenbaum and Goodman2016), Figure 1, with permission from American Psychological Association. (b) Participants encode shapes and reidentify them using minimal description length in a PLoT; reprinted from Sablé-Meyer et al. (Reference Sablé-Meyer, Ellis, Tenenbaum and Dehaene2021a), with permission from Mathias Sablé-Meyer. (c) Primitive operations in a geometrical PLoT; reprinted from Sablé-Meyer et al. (Reference Sablé-Meyer, Ellis, Tenenbaum and Dehaene2021a), with permission from Mathias Sablé-Meyer.
Bayesian computational psychology provides evidence that we can learn complex concepts by running probabilistic inductions over a distinctive sort of representational system. This system exploits a rich array of discrete constituents (including predicates and logical operators) that compose into predicate–argument structures of the form A wudsy is an F; these structures function as inferentially promiscuous hypotheses and incorporate built-in logical operators that obey role-filler independence: In other words, this system is an LoT.Footnote 4
Similar architectures have recently been used to capture representations of geometrical structure (Amalric et al., Reference Amalric, Wang, Pica, Figueira, Sigman and Dehaene2017; Romano et al., Reference Romano, Salles, Amalric, Dehaene, Sigman and Figueira2018; Roumi, Marti, Wang, Amalric, & Dehaene, Reference Roumi, Marti, Wang, Amalric and Dehaene2021; Sablé-Meyer, Ellis, Tenenbaum, & Dehaene, Reference Sablé-Meyer, Ellis, Tenenbaum and Dehaene2021a, Reference Sablé-Meyer, Fagot, Caparos, van Kerkoerle, Amalric and Dehaene2021b). For example, Amalric et al. (Reference Amalric, Wang, Pica, Figueira, Sigman and Dehaene2017) gave participants a task: Observe a sequence of dots and guess where the next dot will appear. They developed a “language-of-geometry” (see also Romano et al., Reference Romano, Salles, Amalric, Dehaene, Sigman and Figueira2018) and found that the complexity of descriptions in this language predicted human error patterns. Sablé-Meyer et al. (Reference Sablé-Meyer, Ellis, Tenenbaum and Dehaene2021a) modified this language (including, e.g., accommodating curve-tracing). Participants took as long as needed to encode shapes, and then reidentified them after a brief delay (Fig. 1b). Description complexity in Sablé-Meyer et al.'s PLoT (Fig. 1c) predicted the duration of both encoding and reidentification.
Our primary aim in this section is to point out that not all cutting-edge computational cognitive science is opposed to LoTH.Footnote 5 Indeed, some of the most impressive work in this area relies on LoTs to model human cognition. Current DNNs may be less well-equipped to capture these capacities. For example, Sablé-Meyer et al. (Reference Sablé-Meyer, Fagot, Caparos, van Kerkoerle, Amalric and Dehaene2021b) examined performance of French adults, Himba adults (who lacked formal education or lexical items for geometric shapes and didn't grow up in a “carpentered world”), and French kindergartners on an “intruder” task where they had to detect an unusual shape in a crowd of shapes. They found that performance in humans was most similar to a model where shapes are “mentally encoded as a symbolic list of discrete geometric properties” (Sablé-Meyer et al., Reference Sablé-Meyer, Fagot, Caparos, van Kerkoerle, Amalric and Dehaene2021b, p. 5). This LoT-like model was contrasted with state-of-the-art deep convolutional neural networks (DCNNs) as well as nonconvolutional DNNs (specifically, variational autoencoders), and the LoT model outperformed the alternatives. Furthermore, PLoTs are capable of encoding domain-general models that underwrite commonsensical reasoning, a well-known limitation of extant DNNs (Peters & Kriegeskorte, Reference Peters and Kriegeskorte2021; Zhu et al., Reference Zhu, Gao, Fan, Huang, Edmonds, Liu and Zhu2020). Given the expressive flexibility of PLoTs and their ability to model concept acquisition from just a single data point, they exhibit some advantages over DNN architectures (Piantadosi et al., Reference Piantadosi, Tenenbaum and Goodman2016, p. 414; cf. Brown et al., Reference Brown, Mann, Ryder, Subbiah, Kaplan, Dhariwal and Amodei2020; but see Ye & Durrett, Reference Ye and Durrett2022).
To be clear on the dialectic, many theorists are inclined to point to advances in AI as sufficient evidence against the LoTH. PLoTs serve as an existence proof that LoT architectures are useful in computational modeling. Our claim is not that DNNs will never be able to model these data; indeed, because DNNs are universal function approximators, perhaps such a claim is ipso facto false. Other learning policies (e.g., meta-learning; Finn, Yu, Zhang, Abbeel, & Levine, Reference Finn, Yu, Zhang, Abbeel and Levine2017) or architectures (e.g., transformers; Vaswani et al., Reference Vaswani, Shazeer, Parmar, Uszkoreit, Jones, Gomez and Polosukhin2017) may turn out to match symbolic models at mimicking acquisition of logically complex concepts and geometrical encoding in humans. We also grant that DNNs are useful for various engineering purposes outside the context of modeling biological competences. Our claim is simply that computational modeling has not left LoT-like symbolic models behind; LoTH remains fruitful in twenty-first-century computational cognitive science.
It is well-understood by contributors to this literature that “the form that [LoT] takes has been modeled in many different ways depending on the problem domain” (Romano et al., Reference Romano, Salles, Amalric, Dehaene, Sigman and Figueira2018, p. 2). The PLoTs used to model geometrical cognition possess discrete constituents that combine recursively to form more complex shapes, exhibiting role-filler independence, and encode abstract geometric “primitives” (Amalric et al., Reference Amalric, Wang, Pica, Figueira, Sigman and Dehaene2017) like symmetry and rotation independently of low-level properties. Other PLoTs used to model (complex) concept acquisition possess all these features plus logical operators and predication. Of course, whether any or all of these PLoTs turn out to be isomorphic to human cognition is still – like most questions in cognitive science – open. The two morals we stress are (a) that many of these models are meant to test concrete representational formats at the algorithmic level, (b) that these models implement LoTs, and (c) that they sometimes match human performance better than competitor models.
4. Perception
LoTH is often framed as a thesis about thought – that is, post-perceptual central cognition. The idea that perception itself might be couched in an LoT is often ignored (cf. Fodor, Reference Fodor1975, Ch. 1; Pylyshyn, Reference Pylyshyn2003). Indeed, characterizations of many anti-LoTH views, for example, concept empiricism, appeal to the hypothesis that conceptual representations have the same format as perceptual representations, implicitly ruling out the possibility of LoT in perception (Machery, Reference Machery2016; Prinz, Reference Prinz2002).
We propose instead to take it as an empirical question whether LoT-like representations are deployed in perception, and we'll argue that the answer is likely “Yes.” If cognition is largely LoT-like, and perception feeds information to cognition, then we should expect at least some elements of perception to be LoT-like, because the two systems need to interface (Cavanagh, Reference Cavanagh2021; Mandelbaum, Reference Mandelbaum2018; Quilty-Dunn, Reference Quilty-Dunn2020a). Our case studies include perceptual representations of objects (e.g., object files), relations within objects (e.g., part-whole relations), and relations between objects.
4.1 Object files
Object files are perceptual representations that select individuals, track them across time and space, and store information about them in visual working memory (VWM). This construct is probed via independent, but converging methods, including: Multiple-object tracking (Fig. 2a; Pylyshyn & Storm, Reference Pylyshyn and Storm1988), object-based VWM storage (Fig. 2b; Hollingworth & Rasmussen, Reference Hollingworth and Rasmussen2010), physical reasoning, especially in infants (Fig. 2c; Xu & Carey, Reference Xu and Carey1996), and object-specific preview benefits (Fig. 2d; Kahneman, Treisman, & Gibbs, Reference Kahneman, Treisman and Gibbs1992). These methods cluster around a common underlying representation, standardly taken to be a unified representational kind (Carey, Reference Carey2009; Green & Quilty-Dunn, Reference Green and Quilty-Dunn2021; Scholl & Leslie, Reference Scholl, Leslie, Lepore and Pylyshyn1999; Smortchkova & Murez, Reference Smortchkova, Murez, Smortchkova, Dolega and Schlicht2020). Object files are extremely well-studied, are generated by encapsulated perceptual processes (Mitroff, Scholl, & Wynn, Reference Mitroff, Scholl and Wynn2005; Scholl, Reference Scholl2007) that operate prior to and independently of natural-language-guided cognition (Carey, Reference Carey2009), and are widely believed to have some sort of compositional structure (minimally, object–property bindings), making them an excellent test case for LoTH.

Figure 2. (a) Multiple-object tracking: A subset of visible items (“targets”) is tracked while others (“distractors”) are ignored; reprinted from Pylyshyn (Reference Pylyshyn2004), Figure 1, with permission from Taylor & Francis. (b) Object-based VWM storage: A change detection task demonstrates that color is recalled for each object despite location changes, providing just one example piece of evidence that object-based storage in VWM uses object-file representations; reprinted from Hollingworth and Rasmussen (Reference Hollingworth and Rasmussen2010), Figure 2, with permission from American Psychological Association. (c) Object-based physical reasoning: Objects pop out from behind an occluder, and preverbal infants rely on spatiotemporal information (and featural and categorical information – see section 5) to keep track of the number of objects, as evidenced by their increased looking time when an unexpected number of items is displayed; reprinted from Xu and Carey (Reference Xu and Carey1996), Figure 1, with permission from Elsevier. (d) Object-specific preview benefit: A feature is previewed in each of two visible objects before disappearing, after which the objects move to new locations, and a target feature appears. Subjects show a benefit in reaction time when discriminating the feature if reappears in the same object, illustrating that object-file representations store object properties across spatiotemporal changes; reprinted from Mitroff et al. (Reference Mitroff, Scholl and Wynn2005), Figure 4, with permission from Elsevier.
According to Carey's (Reference Carey2009) seminal theory of core cognition, object files are amodal but iconic in format (cf. Xu, Reference Xu2019). Nonetheless, we believe an LoT-based model is better suited to the data than an iconic model (Green & Quilty-Dunn, Reference Green and Quilty-Dunn2021; Quilty-Dunn, Reference Quilty-Dunn2020a, Reference Quilty-Dunn2020c). As far as we know, the possibility of logical operators in object files hasn't been studied. However, converging evidence suggests that object files have discrete constituents, role-filler independence, predicate–argument structure, and abstract conceptual content. In section 5, we'll explore the inferential promiscuity of object files in physical reasoning.
4.1.1
First, object files exhibit a decomposition into discrete constituents. Unlike rival models (e.g., iconic models), an LoT-based model of object perception predicts that featural representations should easily break apart from (i) representations of individuals and (ii) other featural representations.
Representations of color and shape frequently come apart from representations of objects without disrupting multiple-object tracking (Fig. 2a) (Bahrami, Reference Bahrami2003; Zhou, Luo, Zhou, Zhuo, & Chen, Reference Zhou, Luo, Zhou, Zhuo and Chen2010; cf. Pylyshyn, Reference Pylyshyn2007). In VWM, object files dynamically lose featural information like color and orientation independently of one another (Bays, Wu, & Husain, Reference Bays, Wu and Husain2011; Fougnie & Alvarez, Reference Fougnie and Alvarez2011) and VWM resources are depleted independently for color and orientation (Markov, Tiurina, & Utochkin, Reference Markov, Tiurina and Utochkin2019; Wang, Cao, Theeuwes, Olivers, & Wang, Reference Wang, Cao, Theeuwes, Olivers and Wang2017). Similar results hold for real-world stimuli. The state of a book (open or closed) is remembered or forgotten independently of its color or token identity (Brady, Konkle, Alvarez, & Oliva, Reference Brady, Konkle, Alvarez and Oliva2013), and the identity and state of multiple real-world objects are independently swapped in VWM (Markov, Utochkin, & Brady, Reference Markov, Utochkin and Brady2021). These effects are independent of natural-language encoding: They persist when subjects engage in articulatory suppression (Fougnie & Alvarez, Reference Fougnie and Alvarez2011; Tikhonenko, Brady, & Utochkin, Reference Tikhonenko, Brady and Utochkin2021), and preverbal infants can lose featural information in VWM but maintain a “featureless” pointer-like component of an object file (Kibbe & Leslie, Reference Kibbe and Leslie2011).
In summary, object files in online tracking and VWM appear to break apart freely into discrete constituents, including representations of individuals and separable feature dimensions. This LoT-like format is independent of natural-language capacities.
4.1.2
Second, object files satisfy demanding constraints on predicate–argument structure. One can grant that object files decompose into discrete constituents but deny that these constituents are ordered into a genuinely sentence-like representation. Here we highlight two constraints on genuinely sentence-like predicate–argument representations: Role-filler independence (one of our six LoT properties) and a grammatical attribution/predication distinction.
Recall that role-filler independence requires that discrete constituents compose into larger structures, but the syntactic structure is typed independently of its particular constituents, and the constituents are typed independently of how they happen to compose on a particular occasion. In a predicate–argument structure in particular, both predicate and argument must maintain type-identity independently of their current bindings – for example, it must be the same JOHN and TALL in TALL(JOHN), TALL(MARY), and SHORT(JOHN).
The clear candidates for predicate-like and argument-like representations in object files are representations of properties and representations of individuals, respectively (cf. Cavanagh, Reference Cavanagh2021). Representations of individuals must maintain their identity independently of the properties they bind, because tracking performance is successful while properties change (Flombaum, Kundey, Santons, & Scholl, Reference Flombaum, Kundey, Santons and Scholl2004; Flombaum & Scholl, Reference Flombaum and Scholl2006; Zhou et al., Reference Zhou, Luo, Zhou, Zhuo and Chen2010) and even while properties are forgotten entirely (Bahrami, Reference Bahrami2003; Scholl, Pylyshyn, & Franconeri, Reference Scholl, Pylyshyn and Franconeriunpublished). The computational processes involved in tracking are known as object correspondence processes. Some properties are used to compute object correspondence (e.g., spatiotemporal features and some surface features – see below). However, the fact that the argument-like representation of the tracked individual can persist while many attributed features are changed/lost entails that the representation maintains independence from the properties to which it is bound.
Likewise, representations of properties maintain their identity independently of the object representations to which they're bound. Some evidence for this is the already-cited fact that they regularly come apart from their respective object representations. However, more striking evidence comes from the way in which featural information is “swapped” between objects. Participants often misremember a feature of one object as bound to another object (Bays, Catalao, & Husain, Reference Bays, Catalao and Husain2009), including for real-world stimuli (Markov et al., Reference Markov, Utochkin and Brady2021; Utochkin & Brady, Reference Utochkin and Brady2020). Even during multiple-object tracking, a stored feature of one object (e.g., a previewed numeral) may be swapped with another object if they come too close to each other during tracking (Pylyshyn, Reference Pylyshyn2004). Thus property representations, like individual representations, maintain type-identity across distinct bindings, demonstrating role-filler independence.
The second constraint on predicate–argument structure is a grammatical attribution/predication distinction. In a genuinely sentence-like representation, we can distinguish grammatical positions of predicates. For example:
(1) That spherical object is red.
(2) That red object is spherical.
Both attribute spherical shape to the referent of “That,” but in (1) the predicate falls within the scope of the noun phrase, whereas in (2) it is in main-predicate position.
One way of capturing this distinction is by appeal to the role of the predicate in grounding the reference of the noun phrase. For example, Perner and Leahy characterize thought in terms of file-like representations (cf. Recanati, Reference Recanati2012), which “capture the predicative structure of language, i.e., the distinction between what one is talking about (the subject, topic, i.e., what the file tracks) and what one says about it (the information about the topic, i.e., the information the file has on it)” (Reference Perner and Leahy2016, p. 494). Files have “labels” that are captured by (inter alia) determiner phrases like THE RABBIT as well as file contents that include predicates like +FURRY. The attribution of RABBIT in THE RABBIT plays some reference-grounding role, whereas +FURRY is parasitic on the referent of THE RABBIT and merely predicates a property of that referent (see Burge, Reference Burge2010). In particular, the label-like attributive helps to sustain, and constrain, reference of the file over time.
We can exploit the attribution/predication distinction to see whether the discrete constituents of object files are organized in a genuinely predication-like way, or whether they are merely label-like representations, as in THE RABBIT. The latter format is compatible with an LoT-based model, but part of the virtue of LoTH is that it predicts nontrivial clustering of LoT-like properties. We ought to predict full-blown propositional structures are present in perception as well.
Object files attribute a wide range of properties to their referents, and some of these are used to guide reference to objects. For example, an object file will continue to refer to an object that disappears behind an occluder, but only if it reemerges at a spatiotemporally appropriate location (Scholl & Pylyshyn, Reference Scholl and Pylyshyn1999). However, although object files attribute other features like color, reference to the object is maintained even if it reemerges a totally different color. Generalizations like this have led some researchers to describe spatiotemporal features as aspects of the object-file “label” while surface features are “stored inside the folder” (Flombaum, Scholl, & Santos, Reference Flombaum, Scholl, Santos, Hood and Santos2009, p. 153). Recent evidence casts doubt on strict limitations on which properties are part of the “label.” although earlier theories took spatiotemporal indices to be uniquely privileged (e.g., Leslie, Xu, Tremoulet, & Scholl, Reference Leslie, Xu, Tremoulet and Scholl1998), surface features like color can play an indexing, reference-guiding role in object files, even in ordinary contexts (Hein, Stepper, Hollingworth, & Moore, Reference Hein, Stepper, Hollingworth and Moore2021; Hollingworth & Franconeri, Reference Hollingworth and Franconeri2009; Moore, Stephens, & Hein, Reference Moore, Stephens and Hein2010). However, object files routinely store some featural information (e.g., color or orientation) while completely failing to use it to guide reference to objects (e.g., Gordon & Vollmer, Reference Gordon and Vollmer2010; Gordon, Vollmer, & Frankl, Reference Gordon, Vollmer and Frankl2008; Jiang, Reference Jiang2020; Richard, Luck, & Hollingworth, Reference Richard, Luck and Hollingworth2008; see Quilty-Dunn & Green, Reference Quilty-Dunn and Green2023, for a review).
Object files not only contain discrete constituents, but also the way those constituents are organized satisfies demanding criteria for predicate–argument structure.
4.1.3
Third, object files encode abstract conceptual content. Part of the utility of LoT-like formats is abstracting away from modality-specific information. An LoT allows color and categorical information to be captured in the same representation, as in THAT OBJECT IS A BROWN RABBIT. If object files are LoT-like representations, they not only ought to encode conceptual categories, they ought to do so in a way that abstracts away from sensory details.
The evidence suggests that object files do encode abstract conceptual content. For example, the object-specific preview benefit – a reaction-time benefit in discriminating previously viewed properties of tracked objects (Fig. 2d) – is observed even when the previewed feature is an image of a basic-level category (e.g., APPLE) and the test feature is the corresponding word (e.g., “apple”) (Gordon & Irwin, Reference Gordon and Irwin2000). Similar effects are found for semantic identity of words across fonts (Gordon & Irwin, Reference Gordon and Irwin1996) or basic-level categories across different exemplars (Pollatsek, Rayner, & Collins, Reference Pollatsek, Rayner and Collins1984) and across visual and auditory information (Jordan, Clark, & Mitroff, Reference Jordan, Clark and Mitroff2010; cf. O'Callaghan, Reference O'Callaghan, Mroczko-Wąsowicz and Grushforthcoming). Importantly, these effects do not transfer across associatively related stimuli (e.g., bread–butter), ruling out a reductive associative explanation (Gordon & Irwin, Reference Gordon and Irwin1996).
Similar effects were recently found in preverbal infants. Kibbe and Leslie (Reference Kibbe and Leslie2019) discovered that while infants will not notice whether the first of two serially hidden objects changes its surface features when it reemerges from behind an occluder, they do notice when it changes its category between FACE and BALL. Pomiechowska and Gliga (Reference Pomiechowska and Gliga2021) tested preverbal infants in an EEG change-detection task for familiar categories (e.g., BOTTLE) or unfamiliar categories (e.g., STAPLER). Infants showed an equal response in the negative-central event-related potential (an EEG signature of sustained attention) for across-category and within-category changes for unfamiliar categories, suggesting, unsurprisingly, failure to categorize. But for familiar categories, they showed an increased amplitude only for across-category changes, suggesting that their object files in VWM maintained the conceptual category of the object while visual features decayed.
In adults, VWM seems often to discard specific sensory information in favor of conceptual-category-guided representations (Xu, Reference Xu2017; Reference Xu2020; cf. Gayet, Paffen, & Van der Stigchel, Reference Gayet, Paffen and Van der Stigchel2018; Harrison & Tong, Reference Harrison and Tong2009). Participants recall blurry images as less blurry than they really were, suggesting categorical encoding that “goes beyond simply ‘re-experiencing’ images from the past” (Rivera-Aparicio, Yu, & Firestone, Reference Rivera-Aparicio, Yu and Firestone2021, p. 935). Bae, Olkkonen, Allred, and Flombaum (Reference Bae, Olkkonen, Allred and Flombaum2015) found that object files in online perception and VWM are biased toward the center of color categories, suggesting that object files store a basic-level color category like RED plus a noisy point estimate within the range of possible red shades. This evidence implicates a category-driven format for object-based VWM representations that abstracts away from low-level visual detail.
Object files encode abstract conceptual content in a way that is not reducible to low-level modality-specific information, just as an LoT-based model predicts.
4.2. Structured relations
We've just argued that perceptual representations of individual objects contain discrete constituents that are organized in a predicate–argument structure and predicate abstract conceptual contents – in other words, they're sentences in the LoT. We'll now describe some LoT-like properties of representations used in the perception of structured relations, both within and between objects.
4.2.1
First, our perceptual systems represent hierarchical part-whole structure. Our perceptual systems don't simply select objects and attribute properties to them. They also break objects down into component parts and represent their part-whole structure. When we perceive a pine tree, we see a branch as part of the tree and a needle as part of the branch, with a sense of the borders between these various parts. Thus the visual system makes use of hierarchical structural descriptions (Fig. 3a; Green, Reference Green2019; Hummel, Reference Hummel and Reisburg2013).
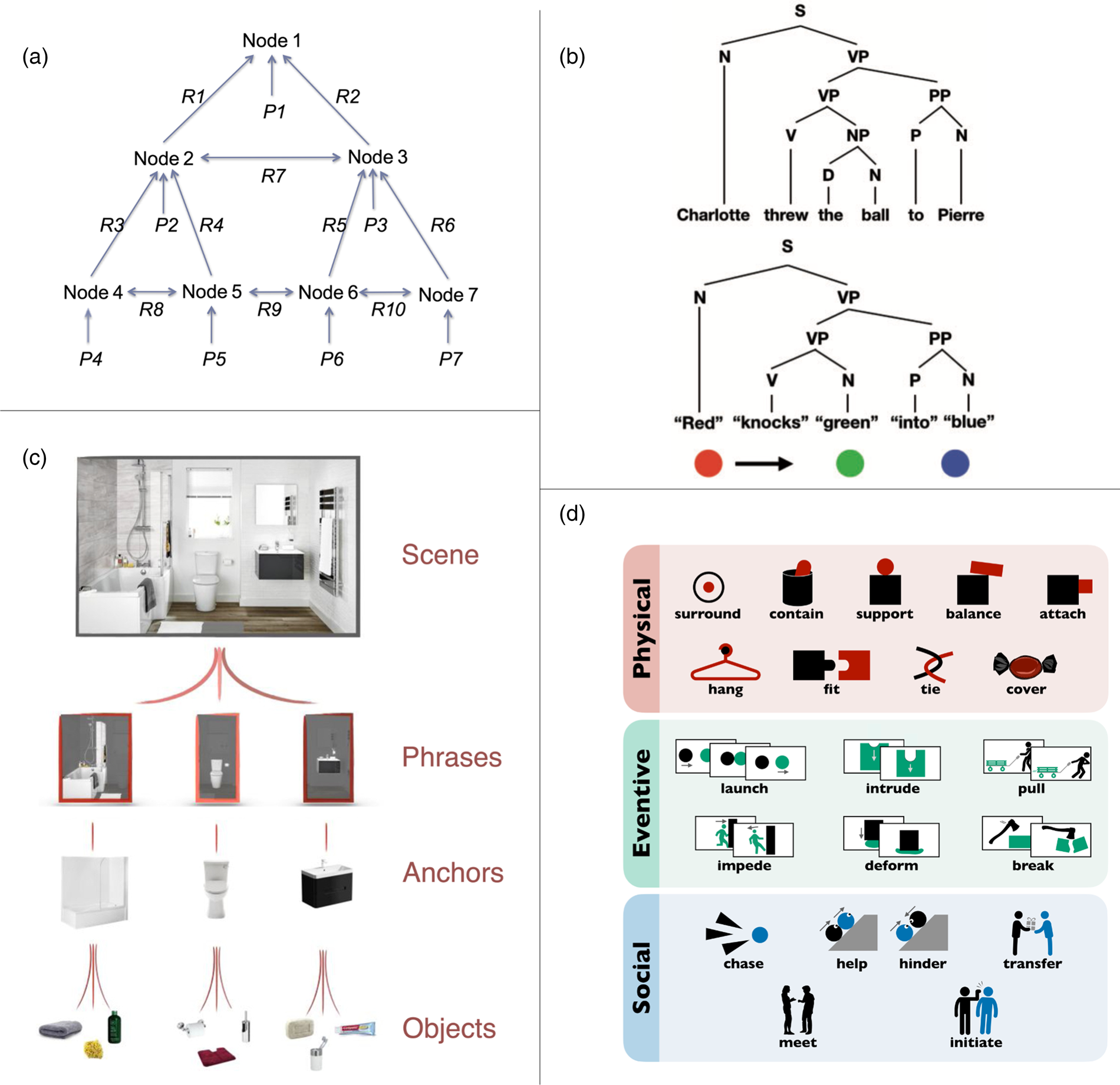
Figure 3. (a) Hierarchical part-whole structural description: Ps = monadic featural properties, horizontal Rs = spatial relations, vertical Rs = mereological relations; reprinted from Green (Reference Green2019), Figure 9, with permission from Wiley. (b) Structural analogy between tree-like structures in natural-language syntax and tree-like perceptual representations of interobject relations; reprinted from Cavanagh (Reference Cavanagh2021), Figure 3, with permission from Sage under CC BY 4.0, cropped and rearranged. (c) Hierarchical structure in scene grammar: Objects are organized relative to “anchors” (relatively large, immobile elements of environments like showers and trees) in phrase-like structural descriptions of normal relative positions; reprinted from Võ, Bettcher, and Draschkow (Reference Võ, Bettcher and Draschkow2019), Figure 2, with permission from Elsevier. (d) Examples of perceived interobject relations; reprinted from Hafri and Firestone (Reference Hafri and Firestone2021), Figure 2, with permission from Elsevier.
The motivation for classic structural-description accounts of object perception was computational: Positing representations of object parts that compose to generate descriptions of part–whole structure allows for successful computational modeling of object perception (Biederman, Reference Biederman1987; Marr & Nishihara, Reference Marr and Nishihara1978). These models operate just as a classical LoT picture demands, exhibiting systematic and productive compositionality of viewpoint-invariant descriptions of parts (Fig. 3b; Cavanagh, Reference Cavanagh2021). Structural descriptions “are compositional – forming complex structures by combining simple elements – and thus meaningfully symbolic” (Saiki & Hummel, Reference Saiki and Hummel1998b, p. 1146).Footnote 6
One of the key assumptions of such models is that object-part boundaries are psychologically real, that is, two points will be treated differently by the visual system when they lie on the same part as opposed to two different parts of the same object. This assumption turns out to be true (Green, Reference Green2019). For example, a well-known example of object-based attention is that two stimuli are better discriminated when they lie on the same object than different objects, controlling for distance (Duncan, Reference Duncan1984; Egly, Driver, & Rafal, Reference Egly, Driver and Rafal1994). The same is true within parts of objects: Participants are quicker to discriminate targets if they lie on the same part than if they cross a part-boundary (Barenholtz & Feldman, Reference Barenholtz and Feldman2003). Furthermore, unfamiliar object pairs that share structural descriptions are seen as more similar than object pairs that have a higher degree of overall geometrical similarity but different structural descriptions (Barenholtz & Tarr, Reference Barenholtz and Tarr2008).
Role-filler independence emerges directly from structural description models, often explicitly so (Hummel, Reference Hummel, Dietrich and Markman2000). Some independent evidence comes from Saiki and Hummel (Reference Saiki and Hummel1998a), who found that shapes of parts and their spatial relations are not represented holistically – in other words, the type-identity of each part is represented independently of its particular role in the structural description and vice versa. Similarity judgments are also guided independently by part shapes and their interrelations, suggesting role-filler independence (Goldstone, Medin, & Gentner, Reference Goldstone, Medin and Gentner1991).
We don't deny that the visual system also employs holistic view-based template-like representations (Edelman, Reference Edelman1999; Ullman, Reference Ullman1996) and other formats. Our claims are merely (i) structural descriptions are among the many representations used in visual processing, and (ii) they have an LoT-like format comprising discrete constituents ordered in hierarchical ways that preserve role-filler independence (Fig. 3b).
4.2.2
Second, we perceive structured relations between objects. We don't perceive objects as isolated atoms, as if through a telescope. Instead, we see the glass on the table, the pencils in the cup, and so on.
In a recent review, Hafri and Firestone (Reference Hafri and Firestone2021) survey striking evidence that such relations are recovered rapidly and in abstract form in visual processing (Fig. 3d). For example, the visual system distinguishes containment events (one object disappears inside another) from occlusion events (one disappears behind another) (Strickland & Scholl, Reference Strickland and Scholl2015). A hallmark of categorical perception is greater discrimination across- than within-category boundaries; participants are better at identifying changes in the position of two circles if the change places the circles in a distinct relation (e.g., CONTAIN(X,Y), TOUCH(X,Y), etc.), suggesting categorically perceived interobject relations (Lovett & Franconeri, Reference Lovett and Franconeri2017). When participants are searching for a particular relation like cup-contains-phone, they are more likely to have a “false-alarm” for target images that instantiate the same relation, like pan-contains-egg, but not book-on-table (Hafri, Bonner, Landau, & Firestone, Reference Hafri, Bonner, Landau and Firestone2021).
Like structural descriptions, perceptual representations of abstract relations exhibit role-filler independence. Abstract relations apply independently of the relata, and representations of relata persist once the relation is broken – for example, it's the same ON in ON(CAT,COUNTER) and ON(KETTLE,STOVE), and it's the same CAT once the cat leaps off the counter. Hafri et al.'s (Reference Hafri, Bonner, Landau and Firestone2021) finding is especially relevant: The relation CONTAIN(X,Y) governs similarity judgments independently of the relata, about as clear a demonstration of role-filler independence as one could expect to find.
It would be efficient for the visual system to store frequently represented relations. A fascinating recent literature on “scene grammar” (Fig. 3c; Kaiser, Quek, Cichy, & Peelen, Reference Kaiser, Quek, Cichy and Peelen2019; Võ, Reference Võ2021) details effects of representations of structured relations in visual long-term memory on visual search (Draschkow & Võ, Reference Draschkow and Võ2017), categorization (Bar, Reference Bar2004), consciousness (Stein, Kaiser, & Peelen, Reference Stein, Kaiser and Peelen2015), and gaze duration (Võ & Henderson, Reference Võ and Henderson2009). Relational representations in visual long-term memory (e.g., ON(POT,STOVE) = yes, IN(SPATULA,MICROWAVE) = no) aren't based on associations or statistical summaries over low-level properties. They persist despite changes in position and context (Castelhano & Heaven, Reference Castelhano and Heaven2011), thus abstracting away from overlearned associations. Characteristic scene-grammar effects disappear, however, for upside-down stimuli (Stein et al., Reference Stein, Kaiser and Peelen2015), implicating a categorical rather than low-level format. The effects also appear not to rely on summary-statistical information represented outside focal attention (Võ & Henderson, Reference Võ and Henderson2009). Despite developing independently of natural language (Öhlschläger & Võ, Reference Öhlschläger and Võ2020), structured relations in scene grammar display curious hallmarks of language-like formats. For instance, the P600 ERP increases for syntactic violations in language, and also increases for stimuli that violate visual scene “syntax” (e.g., mouse-on-computer instead of mouse-beside-computer; Võ & Wolfe, Reference Võ and Wolfe2013). It's standard to talk of scene grammar as associative, but its relational components satisfy a handful of our LoT hallmarks (e.g., discrete constituents with role-filler independence that encode abstract contents, including categories and relations, and function as arguments in multiplace predicates as in ABOVE(MIRROR,SINK)). Scene grammar is used directly in controlled behavior (e.g., how to arrange a virtual reality scene; Draschkow & Võ, Reference Draschkow and Võ2017); how broadly it can function in logical inference remains to be explored experimentally.
4.3 Vision and DNNs
In sum, our perceptual capacities to identify and track objects, grasp their characteristic structures, and perceive and store their relations with one another, appear to rely on LoT-like representations.
A major source of contemporary skepticism about LoTH is the rise of DNNs. Apart from large language models like GPT-3, nowhere are DNNs more visible as models of human cognitive capacities than in visual perception. Given their successes at image classification and apparent similarities to biological vision, one might wonder whether the subsymbolic network structure of DNNs obviates the need to posit LoT-like structures.
The DNNs that have been most touted as models of biological vision are deep convolutional neural networks (DCNNs) trained to classify images (Kriegeskorte, Reference Kriegeskorte2015; Yamins & DiCarlo, Reference Yamins and DiCarlo2016). After training on large data sets like ImageNet, DCNNs exhibit remarkable levels of performance on image classification. It is important to evaluate comparisons to human vision not simply in terms of performance, but primarily in terms of underlying competence (Chomsky, Reference Chomsky1965). Just as differences in performance need not entail differences in competence (Firestone, Reference Firestone2020), human-like performance on a limited range of tasks need not entail human-like underlying competence. In other words, DCNNs may accomplish image classification while lacking key structural features of human vision, including those relevant to LoTH.
DCNNs have been argued to resemble primate vision in competence as well as performance by appeal to metrics of similarity such as “Representational Similarity Analysis” (Khaligh-Razavi & Kriegeskorte, Reference Khaligh-Razavi and Kriegeskorte2014) and “Brain-Score” (Schrimpf et al., Reference Schrimpf, Kubilius, Hong, Majaj, Rajalingham, Issa and DiCarlo2018). However, there are shortcomings both to earlier findings of high similarity using these metrics and to the metrics themselves. For example, Xu and Vaziri-Pashkam (Reference Xu and Vaziri-Pashkam2021b) used higher quality fMRI data for their representational similarity analysis and found that, contra Khaligh-Razavi and Kriegeskorte's earlier findings, high-performing DCNNs (both feedforward and recurrent) show large-scale dissimilarities to human vision. Brain-Score has been criticized for insufficient sensitivity to architectural distinctions (e.g., feedforward vs. recurrent models): “either the Brain-Score metric or the methodology with which a model is evaluated on it fails to distinguish among what we would think of as fundamentally different types of model architectures” (Lonnqvist, Bornet, Doerig, & Herzog, Reference Lonnqvist, Bornet, Doerig and Herzog2021, p. 3). Furthermore, although Schrimpf et al. (Reference Schrimpf, Kubilius, Hong, Majaj, Rajalingham, Issa and DiCarlo2018) found that Brain-Score positively correlates with image classification performance, it fails to capture the crucially hierarchical structure of human vision. Nonaka, Majima, Aoki, and Kamitani (Reference Nonaka, Majima, Aoki and Kamitani2021) thus developed a “Brain Hierarchy Score” that measures similarities between hierarchical structures, applied it to 29 DNNs, and found a negative correlation between image classification performance and similarity to human vision. This finding provides a striking illustration of how DNNs can excel in performance while veering apart from human competence (see also Fel, Felipe, Linsley, & Serre, Reference Fel, Felipe, Linsley and Serre2022).
Our case for LoT in vision is limited to certain domains: Objects, relations between parts and wholes, and relations between objects. It is not a coincidence, in our view, that DNNs that succeed at image classification exhibit little to no competence in these domains. As Peters and Kriegeskorte write about feedforward DCNNs, “the representations in these models remain tethered to the input and lack any concept of an object. They represent things as stuff” (2021, p. 1128).Footnote 7 It is also not clear that DCNNs are capable of representing global shape, let alone the relation between global shape and object parts (Baker & Elder, Reference Baker and Elder2022). Baker, Lu, Erlikhman, and Kellman (Reference Baker, Lu, Erlikhman and Kellman2020) trained AlexNet, VGG-19, and ResNet-50 to classify circles and squares, but found that these DCNNs relied only on local contour information; circles made of jagged local edges were classified as squares, and squares made of round local curves were classified as circles. The same models (and several others) also could not distinguish possible from impossible shapes, which requires relating local contour information to global shape (Heinke, Wachman, van Zoest, & Leek, Reference Heinke, Wachman, van Zoest and Leek2021). Failures at processing relations hold not only for DNNs that map images to labels, but also those that map labels to images: Conwell and Ullman (Reference Conwell and Ullman2022) fed the text-guided image-generation model DALL-E 2 a set of interobject relations (including those used by Hafri et al., Reference Hafri, Bonner, Landau and Firestone2021) and found that it failed reliably to distinguish, for example, “a spoon in a cup” from “a cup on a spoon.”
To be clear, we make no claims about in-principle limitations of DNNs. The machine-learning literature is extremely fast-moving, and we do not pretend to know what it will look like in even 1 year's time. Moreover, different DNN architectures might better capture the visual processes discussed here. Although convolutional architectures might privilege local image features, perhaps nonconvolutional architectures like vision transformers (Vaswani et al., Reference Vaswani, Shazeer, Parmar, Uszkoreit, Jones, Gomez and Polosukhin2017) are better suited to avoid these limitations and will supersede DCNNs as models of human vision (Tuli, Dasgupta, Grant, & Griffiths, Reference Tuli, Dasgupta, Grant and Griffiths2021). Because DCNNs have accumulated enormous publicity despite apparently lacking basic elements of biological vision like global shape and objecthood, future DNN–human comparisons should be approached with caution. Finally, as was noted long ago, neural-network architectures might be able to implement an LoT architecture (Fodor & Pylyshyn, Reference Fodor and Pylyshyn1988). Indeed, some recent work on DNNs explores implementations of variable binding (Webb, Sinha, & Cohen, Reference Webb, Sinha and Cohen2021; though see Gröndahl & Asokan, Reference Gröndahl and Asokan2022; Miller, Naderi, Mullinax, & Phillips, Reference Miller, Naderi, Mullinax and Phillips2022), a classic example of LoT-like symbolic computation (Gallistel & King, Reference Gallistel and King2011; Green & Quilty-Dunn, Reference Green and Quilty-Dunn2021; Marcus, Reference Marcus2001; Quilty-Dunn, Reference Quilty-Dunn2021). Our six core LoT properties help specify a cluster of features that such an implementation should aim for.
DNNs are marvels of contemporary engineering. It does not follow that they recapitulate architectural aspects of human vision. We agree with Bowers et al.'s (Reference Bowers, Malhotra, Dujmović, Montero, Tsvetkov, Biscione and Blything2022) recent complaint that research on DNNs as models of biological vision is overly focused on performance benchmarks and insufficiently guided by experimental perceptual psychology. Given that DNNs are universal function approximators, and given the vast resources being poured into their development, they will only get closer to human performance over time. But this performance will not reflect core competences of the human visual system unless the relevant models incorporate LoT-like representations of objects and relations.
5. LoTs in nonhuman animals and children
Traditionally, theorists in animal and infant cognition have been reluctant to posit complex cognitive processes, let alone computations over LoT-style representations (e.g., Morgan, Reference Morgan1894; Penn, Holyoak, & Povinelli, Reference Penn, Holyoak and Povinelli2008; Premack, Reference Premack2007; cf. Fitch, Reference Fitch2019). However, the state-of-the-art in comparative and developmental psychology is surprisingly congenial to LoTH.
5.1 Abstract content and physical reasoning
Considerable evidence suggests infants use object files to reason about the identity, location, and numerosity of hidden objects (Carey, Reference Carey2009; Spelke, Reference Spelke1990). However, in a foundational study, Xu and Carey (Reference Xu and Carey1996) found that, although 12-month olds who see a duck and then a ball pop out from behind an occluder expect two objects to be present, 10-month olds don't. This failure might seem to suggest that abstract conceptual content is not usable for physical reasoning in young infants, potentially undermining LoT-based models of infant reasoning (Xu, Reference Xu2019).
However, 10-month olds do succeed for socially significant categories (Bonatti, Frot, Zangl, & Mehler, Reference Bonatti, Frot, Zangl and Mehler2002; Surian & Caldi, Reference Surian and Caldi2010) and objects that are made communicatively salient (Futo, Teglas, Csibra, & Gergely, Reference Futo, Teglas, Csibra and Gergely2010; Xu, Reference Xu2019, p. 843). There is also evidence that priming can allow infants to use information in physical reasoning many months earlier than they would otherwise appear to. Lin et al. (Reference Lin, Li, Gertner, Ng, Fisher and Baillargeon2021) made features (e.g., color) salient by first showing an array of objects that differed along the relevant dimension (e.g., all different colors). This nonverbal priming allowed infants to use information in object files to reason about the individuation of hidden objects 6 months earlier than other methods had detected (e.g., while infants had not shown surprise at a lop-sided object balancing on a ledge until 13 months, Lin et al.'s nonverbal priming of lop-sidedness caused 7-month olds to show the effect).
Infants should therefore be able to use conceptual categories for Xu and Carey's individuation task long before 12 months if the right information is primed first: For example, the relevance of the category's function, a key aspect of artifact concepts (Kelemen & Carey, Reference Kelemen, Carey, Margolis and Laurence2007; cf. Bloom, Reference Bloom1996). Stavans and Baillargeon (Reference Stavans and Baillargeon2018) demonstrated objects' characteristic functions before hiding (Fig. 4a) and found 4-month olds succeeded at Xu and Carey's individuation task, looking longer when only one object was revealed. These results show two key LoT-like features – abstract content and inferential promiscuity – in extremely young preverbal infants. Thus the earlier failures seem to be explained by performance constraints (Stavans, Lin, Wu, & Baillargeon, Reference Stavans, Lin, Wu and Baillargeon2019).

Figure 4. (a) Function demonstrations aid object individuation: In a modification of Xu and Carey's (Reference Xu and Carey1996) paradigm, infants first see the characteristic function of an object demonstrated (e.g., a marker drawing, a knife cutting), and this demonstration primes them to use categorical and featural information about the objects to expect two objects in the test trials (i.e., increased looking time when only one object appears); reprinted from Stavans and Baillargeon (Reference Stavans and Baillargeon2018), Figures 4 and 5, with permission from Wiley. (b) View-invariant information extracted by newborn chicks: Chicks are shown a highly limited set of viewpoints on an object and form an abstract, view-invariant representation; reprinted from Wood and Wood (Reference Wood and Wood2020), Figure 1, with permission from Elsevier.
The use of abstract content in physical reasoning is arguably present throughout the animal kingdom, and is well-studied in primates (e.g., Flombaum et al., Reference Flombaum, Kundey, Santons and Scholl2004) and even some arthropods. Loukola, Perry, Coscos, and Chittka (Reference Loukola, Perry, Coscos and Chittka2017) trained bumblebees through social learning (using a dummy bee) to roll a ball – an unusual behavior for bumblebees in the wild – into the center of a platform for a sucrose reward. When the platform was later rearranged with several balls at various locations that the bees could push into that central area, the bees opted to push balls closest to the center of the platform, even if they differed in color or location from the one they had seen pushed initially. This suggests bumblebees are sensitive to shape in a way that is dissociable from color and location, in contrast to many model-free learning accounts but just as one would expect if shape type is encoded in an LoT. In a similar vein, Solvi, Al-Khudhairy, and Chittka (Reference Solvi, Al-Khudhairy and Chittka2020) found that bumblebees could recognize objects under full light that they had previously encountered only in darkness, suggesting they can transfer shape representations stored through touch to a visual task. Bumblebees therefore appear to represent shape in a way that is dissociable from modality-specific low-level features. These representations figure in practical inferences (thereby displaying inferential promiscuity), and that guides recognition across modalities (thereby displaying abstract content). Furthermore, honeybees trained on a fewer-than relation (e.g., 2 < 5) were able to generalize to cases involving zero items (e.g., 0 < 6) without any zero-item training, implicating an abstract symbolic representation of zero that guides inferential generalization and logico-mathematical reasoning (Howard, Avargues-Weber, Garcia, Greentree, & Dyer, Reference Howard, Avargues-Weber, Garcia, Greentree and Dyer2018; cf. Vasas & Chittka, Reference Vasas and Chittka2019; see Weise, Ortiz, & Tibbetts, Reference Weise, Ortiz and Tibbetts2022, for abstract contents of same and different). Similarly, bees' navigational inferences have been used as an argument for a bee LoT because of their computational complexity (Gallistel, Reference Gallistel2011).
Much of our discussion in sections 4 and 5.1 has concerned abstract (e.g., amodal or view-invariant) object representations, and one might wonder whether these effects are really because of associations between low-level features acquired gradually during development. One might therefore wonder whether DNNs could therefore provide a better explanation for these effects. However, Wood and Wood (Reference Wood and Wood2020) found that newborn chicks showed one-shot learning of abstract object representations (Fig. 4b). Shortly after birth, having been reared in an environment with no movable-object-like stimuli, chicks were shown a virtual three-dimensional (3D)-object rotating either fully 360 degrees, or just 11.25 degrees; later, the chicks successfully recognized the objects from arbitrary viewpoints (equally well under both conditions) and moved toward them. Given the paucity of relevant input, this experiment points away from DNN-based explanations of abstract object representations.
Similarly, Ayzenberg and Lourenco (Reference Ayzenberg and Lourenco2021) showed preverbal infants a single view of 60 degrees of an unfamiliar object; using a looking-time measure, they found that the infants formed an abstract, categorical representation, recognizing the object even when viewpoint and salient surface features had drastically changed. The infants' one-shot category learning outperformed DCNNs trained on millions of labeled images. This divergence between DCNN and human performance echoes independent evidence that DCNNs fail to encode human-like transformation-invariant object representations (Xu & Vaziri-Pashkam, Reference Xu and Vaziri-Pashkam2021a).
5.2 Logical inference
Proponents of LoTH have long held up its ability to explain logical inference in preverbal children and nonhuman animals as a virtue (Cheney & Seyfarth, Reference Cheney and Seyfarth2008; Fodor, Reference Fodor1983; Fodor & Pylyshyn, Reference Fodor and Pylyshyn1988; Gallistel, Reference Gallistel2011; cf. Bermudez, Reference Bermudez2003; Camp, Reference Camp2007, Reference Camp2009; Gauker, Reference Gauker2011). Recent evidence suggests infants and animals may use logical operators in logical inferences.
Consider the growing body of work on disjunctive syllogistic (DS) reasoning. A standard means of testing for this capacity is Call's (Reference Call2004) two-cup task. The task involves placing a reward in one of the two cups behind an occluder. Once the cups are brought back into plain view, the participant is shown that one is empty, and can then choose which of the two cups to select from. Typically, researchers are interested in whether the participant selects the unrevealed cup more often than the revealed one, and whether they choose it without inspecting it first. Such behavior is often taken as evidence that the participant can reason through DS, because there's definitely a reward, and one of the two cups is empty, guaranteeing the location of the reward by DS. A surprising number of animals succeed at this task, as well as children as young as two (Call, Reference Call, Hurley and Nudds2006).
Mody and Carey (Reference Mody and Carey2016) argue that there is a confound in such tasks. Participants could rely on a nonlogical strategy involving modal operators: They could form two unrelated beliefs, MAYBE THERE IS A REWARD IN CUP A and MAYBE THERE IS A REWARD IN CUP B. On this strategy, once shown that cup A is empty, participants simply ignore the possibility that there may be a reward there; left only with the belief that there may be a reward in cup B, they then select cup B. So the authors modified this task, using two rewards and four cups (Fig. 5a). Although children as young as 2.5 succeed at the two-cup task, only 3- and 5-year olds succeed at this four-cup task, with 5-year olds performing best.

Figure 5. (a) Four-cup task: A reward is placed behind an occluder and into one of the two cups, and again for another reward and pair of cups. Then one cup is shown to be empty, and participants who perform disjunctive syllogism can infer that a reward is certain to be in the other cup in that pair; reprinted from Mody and Carey (Reference Mody and Carey2016), Figure 1, with permission from Elsevier. (b) Alternatives in chimps: A reward is placed in one of the two boxes, and chimps pull a string to open the box and reveal the reward. The chimps pull both boxes when they are opaque, suggesting simultaneous representation of two possibilities; reprinted from Engelmann et al. (Reference Engelmann, Völter, O'Madagain, Proft, Haun, Rakoczy and Herrmann2021), Figure 1, with permission from Elsevier. (c) Success on four-cup task by baboons, reprinted from Ferrigno et al. (Reference Ferrigno, Huang and Cantlon2021), Figure 1, Sage.
Pepperberg, Gray, Cornero, Mody, and Carey (Reference Pepperberg, Gray, Cornero, Mody and Carey2019) found that an African gray parrot, Griffin, succeeded at a modified version of the four-cup task. Remarkably, Griffin selected the cup that contained reward (a cashew) on nearly every trial (chance, in this case, was 33%), besting human 5-year olds (whose success is surprisingly variable; Gautam, Suddendorf, & Redshaw, Reference Gautam, Suddendorf and Redshaw2021). More moderate success at the four-cup task has also been achieved with olive baboons (Fig. 5c; Ferrigno, Huang, & Cantlon, Reference Ferrigno, Huang and Cantlon2021).
A straightforward way of understanding these results is to accept that at least some nonhuman animals are competent with DS. To execute that inference, one needs two sentential connectives, NOT and OR. These must be combined, syntactically, with representations of states of affairs.
The failure of younger kids at Mody and Carey's four-cup task at first looks like bad news for LoTH. However, it might only reflect a failure with using negation, rather than with logical inference more broadly (Feiman, Mody, & Carey, Reference Feiman, Mody and Carey2022). Moreover, as with Xu's (Reference Xu2019) arguments against LoT-like format in object files, the possibility of performance demands masking an underlying LoT-based competence is plausible. The four-cup task requires kids to track four cups divided into two pairs and two occluded stickers, which is demanding on VWM; indeed, animals that outperform children tend to have superior VWM capacity (Pepperberg et al., Reference Pepperberg, Gray, Cornero, Mody and Carey2019, p. 417; cf. Cheng & Kibbe, Reference Cheng and Kibbe2021). As Pepperberg et al. point out, younger children also act more impulsively than older ones, sometimes ignoring relevant knowledge in demanding tasks. Thus we should look for less demanding tasks before ruling out LoT-like logical inference in children. For example, we could look for independent psychophysical signatures of DS as performed by adults and see whether those signatures are present in children in simpler tasks.
Cesana-Arlotti et al. (Reference Cesana-Arlotti, Martín, Téglás, Vorobyova, Cetnarski and Bonatti2018) showed 12-month olds and adults two objects hidden behind occluders (e.g., a snake and ball); they saw one placed in a cup without knowing which, and finally the unmoved object (e.g., snake) popped out, allowing subjects to infer the identity of the cup-hidden object (ball). When the cup-hidden object was revealed, infants' looking time showed they expected it to be the yet-unseen object (ball). This finding is compatible with nonlogic-based explanations. However, Cesana-Arlotti et al. found that adults performing DS showed an oculomotor signature: During inference, their pupils dilated and eyes darted to the still-hidden object. This same signature was found in the infants, implicating the same underlying computations.
Genuine DS should be domain-general. Cesana-Arlotti, Kovács, and Téglás (Reference Cesana-Arlotti, Kovács and Téglás2020) used a similar paradigm to test DS in 12-month olds, this time relying on their knowledge of others' preferences. Participants learned an agent's preference among objects (ball vs. car); the nonpreferred object then briefly popped out from behind its occluder, after which the agent reached behind one of the occluders. Twelve-month olds looked longer when the nonpreferred object was reached for. Cesana-Arlotti and Halberda (Reference Cesana-Arlotti and Halberda2022) also found that 2.5-year olds, who fail the four-cup task, nonetheless reason by exclusion across word-learning, social-learning, and explicit negation with a common saccade pattern: They saccade to the to-be-excluded item, return to the target item, and fail to show “redundant” saccades – evidence of low confidence – after target selection. This pattern suggests a domain-general inferential mechanism that delivers high-confidence conclusions, a functional profile one should expect if children perform DS.
Leahy and Carey (Reference Leahy and Carey2020) provide an alternative, non-DS-based explanation of successful reasoning by exclusion via sequentially simulating alternative possibilities. However, chimpanzees, at least, are able to represent distinct possible states of affairs simultaneously. Engelmann et al. (Reference Engelmann, Völter, O'Madagain, Proft, Haun, Rakoczy and Herrmann2021) used a modified two-cup task in which the empty cup was not revealed. Chimps could pull ropes for both cups, or pull just one rope for one cup, causing the second cup to fall out of reach. Overwhelmingly they expended extra energy to pull both ropes when the cups were opaque, but pulled just one when the cups were transparent (Fig. 5b).Footnote 8 Pulling two ropes is hedging under uncertainty, suggesting chimps simultaneously represent two locations as possibly reward-laden.
Furthermore, 12-month olds seem to use the same computations adults do to reason by exclusion, as measured by oculomotor signatures (Cesana-Arlotti et al., Reference Cesana-Arlotti, Martín, Téglás, Vorobyova, Cetnarski and Bonatti2018). It's possible that adults do both DS and simulation-based or icon-based reasoning in these tasks. But given independent reasons to think these tasks run on LoT-like object representations in VWM and adults' capacity for DS, and the relative lack of evidence for multiple redundant reasoning processes underlying task performance, our working hypothesis is that infant's oculomotor behavior is evidence for LoT-based DS.
Logical inference without language is a rapidly developing research area, and central contributors to this research such as Carey are skeptical of the “thicker” interpretations of the data we defend. Although we anticipate further plot twists will emerge in study of infant and nonhuman inference, we take the current state of the literature to favor an LoT-based account of DS in infants and animals and to bear promise for many LoTH-based lines of research in the development of logical operators.
6. LoTs in social psychology: The logic of system 1
One source of opposition to LoTH stems from treatments of attitudes and system-1 processing in social psychology. In traditional dual-process theory, system 1 (“S1”) is governed by shallow heuristic, associative, nonrule-based processing (Evans & Stanovich, Reference Evans and Stanovich2013; Sloman, Reference Sloman1996). Dual-process theories originate partly from the heuristics-and-biases tradition, where fast responding purportedly demonstrates irrationality (cf. Gigerenzer & Gaissmaier, Reference Gigerenzer and Gaissmaier2011; Mandelbaum, Reference Mandelbaum2020).
One may doubt the irrationality of S1 processing. As case studies we'll discuss two paradigms used to investigate characteristically S1 thought: Unconscious reasoning in implicit attitudes in the implicit association test and belief bias cases (though the same morals hold for other paradigms such as base rate inferences and cognitive reflection test; Bago & De Neys, Reference Bago and De Neys2017, Reference Bago and De Neys2019, Reference Bago and De Neys2020; De Neys, Cromheeke, & Osman, Reference De Neys, Cromheeke and Osman2011; De Neys & Franssens, Reference De Neys and Franssens2009; De Neys & Glumicic, Reference De Neys and Glumicic2008; De Neys, Rossi, & Houdé, Reference De Neys, Rossi and Houdé2013; Gangemi, Bourgeois-Gironde, & Mancini, Reference Gangemi, Bourgeois-Gironde and Mancini2015; Johnson, Tubau, & De Neys, Reference Johnson, Tubau and De Neys2016; Pennycook, Trippas, Handley, & Thompson, Reference Pennycook, Trippas, Handley and Thompson2014; Stupple, Ball, Evans, & Kamal-Smith, Reference Stupple, Ball, Evans and Kamal-Smith2011; Thompson & Johnson, Reference Thompson and Johnson2014; Thompson, Turner, & Pennycook, Reference Thompson, Turner and Pennycook2011).Footnote 9
6.1 Logic, load, and LoT
Failures of syllogistic reasoning are commonplace and well-publicized. In particular, belief biases – cases where people mistakenly use the truth of a conclusion in judging an argument's validity, ignoring logical form – are legion (Markovits & Nantel, Reference Markovits and Nantel1989). Even outside of the belief bias people are forever affirming-the-consequent, denying the antecedent, and confusing validity and truth.
Difficulties in reasoning are prima facie problematic for LoTH. The more errors we make in reasoning, the less it seems like we need an inferential apparatus to explain people's thinking. LoT is tailor-made to explain formal reasoning – that is, reasoning based on the structure, rather than the content, of one's premises (Fodor & Pylyshyn, Reference Fodor and Pylyshyn1988; Quilty-Dunn & Mandelbaum, Reference Quilty-Dunn and Mandelbaum2018a, Reference Quilty-Dunn and Mandelbaum2018b). So, failures in reasoning – traditionally seen as because of heuristic S1 processing – are seen as reasons for believing that S1 is associative rather than LoT-like (see, e.g., Gawronski & Bodenhausen, Reference Gawronski and Bodenhausen2006; Rydell & McConnell, Reference Rydell and McConnell2006; Sloman, Reference Sloman1996). However, a closer look at the data shows evidence for nonassociative, LoT-like, logic-sensitive reasoning in S1.
“Conflict problems” are cases where validity and believability conflict, that is, valid syllogisms with unbelievable conclusions or invalid syllogisms with believable conclusions. All other problems (valid/believable; invalid/unbelievable) are “nonconflict.” Some examples:
-
(Conflict: Valid/Unbelievable)
-
P1: All birds fly
-
P2: Penguins are birds
-
C: Penguins fly
-
(Conflict: Invalid/Believable)
-
P1: All birds fly
-
P2: Penguins are birds
-
C: Penguins swim
-
(No Conflict: Valid/Believable)
-
P1: All birds have feathers
-
P2: Penguins are birds
-
C: Penguins have feathers
-
(No Conflict: Invalid/Unbelievable)
-
P1: All birds have feathers
-
P2: Penguins are birds
-
C: Penguins fly
If S1 is not logic-sensitive, then conflict problems should not hamper believability judgments, because belief bias is driven by nonlogical factors. Yet logic-sensitive judgments occur even when subjects are explicitly instructed to focus on believability, and even under extreme cognitive load. Logical responses thus seem to be generated automatically. People are less confident and slower on conflict problems than nonconflict problems regardless of whether they are judging belief or logic (Handley & Trippas, Reference Handley and Trippas2015; Howarth, Handley, & Polito, Reference Howarth, Handley and Polito2021; Trippas, Thompson, & Handley, Reference Trippas, Thompson and Handley2017). That is, they'll be slower to judge that “Penguins fly” is false if it is a conclusion of a valid argument than a conclusion of an invalid one. Moreover, those who correctly solve syllogism validity questions in conflict problems do so even under intense time pressure and additional memory load, ensuring the shutdown of system-2 processes (Bago & De Neys, Reference Bago and De Neys2017). That is, correct responding happens right away; giving participants additional time to think adds little accuracy.
Just as the believability of a conclusion can interfere with validity judgments, so too can the logical form of an argument affect believability judgments. In fact, there is evidence that logical responding is more automatic than belief-based responding; derailing logical responding impedes belief-based responding more than vice versa (Handley, Newstead, & Trippas, Reference Handley, Newstead and Trippas2011; Howarth, Handley, & Walsh, Reference Howarth, Handley and Walsh2016; Trippas et al., Reference Trippas, Thompson and Handley2017). For example, in Trippas et al. (Reference Trippas, Thompson and Handley2017), conflict impeded believability judgments more than validity judgments for modus ponens. Sensitivity to logical form persists whether subjects are under load or not (and whether asked to evaluate validity or not), showing that the relevant differences are because of S1 processing (Trippas, Handley, Verde, & Morsanyi, Reference Trippas, Handley, Verde and Morsanyi2016). Even when asked to respond randomly, participants still show implicit sensitivity to logical form (Howarth et al., Reference Howarth, Handley and Polito2021). Automatic logical sensitivity also has very little individual difference between subjects, suggesting it reflects fundamental architectural features of cognition (Ghasemi, Handley, & Howarth, Reference Ghasemi, Handley and Howarth2021). Logical inferences are also made automatically during reading (Dabkowski & Feiman, Reference Dabkowski and Feiman2021; Lea, Reference Lea1995; Lea, Mulligan, & Walton, Reference Lea, Mulligan and Walton2005). As one would expect if logic was intuitive, subliminally presented premises trigger modus ponens inferences (Reverberi, Pischedda, Burigo, & Cherubini, Reference Reverberi, Pischedda, Burigo and Cherubini2012).
Far from undermining LoTH, dual-process architectures vindicate LoTH. They demonstrate abstract logic-based inferential promiscuity outside controlled, conscious cognition using discrete symbols that maintain role-filler independence (e.g., P must be the same symbol in P → Q).
6.2 The logic of implicit attitudes
Implicit attitudes are typically assumed to be associative. However, Mandelbaum (Reference Mandelbaum2016) and De Houwer (Reference De Houwer2019) documented the effects of “logical interventions” on implicit attitudes, that is, cases where one can change implicit attitudes not by counterconditioning or extinction, as would be expected if they had associative structure, but instead by merely changing the logically pertinent evidence. Logical (or “propositional”) interventions on attitudes are only possible given that we have predicate–argument structure, logical operators, and inferential promiscuity.
Take Kurdi and Dunham (Reference Kurdi and Dunham2021). Their basic paradigm consisted of a learning and testing phase. In a learning phase participants saw sentences of the form: “If you see a green circle, you can conclude that Ibbonif is trustworthy; if you see a purple pentagon, you can conclude that Ibbonif is malicious.” This design cleverly pits associative versus propositional (i.e., LoT) processes against each other: If the implicit attitude processor is associative then Ibbonif should come out as neutral as Ibbonif is being associated with both positive (trustworthy) and negative (malicious) adjectives. If the processor is sensitive to propositional values however, then the implicit attitude acquired should be dependent on which conditional's antecedent was satisfied (i.e., which shape appears). Participants then moved onto the testing phase which consisted of explicit and implicit attitude testing (via the IAT). Results showed that participant attitudes tracked the logical form of the stimuli during the testing phase. So, using the sample text above, if participants saw a purple pentagon they would conclude that Ibbonif (and the group that he was from, the Niffites, denoted from the suffix on the name) was negatively valenced.
Kurdi and Dunham had ample variations in the paradigm all showing similar LoT-based effects on implicit attitudes. Importantly, LoT-based inferences can be seen even when the response is normatively inappropriate, as in an affirming-the-consequent syllogism (study 3). In the learning phase, participants saw sentences such as “If you see a green circle, you can conclude that Ibbonif is malicious”; however, instead of seeing a green circle, they would then see an, for example, orange square. Thus the correct inference to make is that nothing can be inferred from the setup. If implicit attitudes are updated only by an associative processor, then the valence of the predicate in the consequent should dictate the participants' responses. If instead attitudes are sensitive to the logical form of the inventions, then one of the two things should happen: For those subjects who correctly realize that this is an affirming-the-consequent argument they should form no opinion about the person or group in question. However, the subset of people who incorrectly affirm the consequent should make the wrong inference and infer that the consequent accurately describes the person or group in question. Participants were given a control question to see if they were apt to explicitly affirm the consequent. Those who did also changed their implicit attitudes in line with the affirming-the-consequent stimuli they would later see in the experiment; the implicit attitudes of those who rejected the affirming-the-consequent control question, on the contrary, correctly tracked the logical implications of the stimuli by failing to update at all (similar results hold for denying the antecedent). Given a sufficiently creative setup, one can infer logical processes at play even in the absence of inference, or during misinference (Quilty-Dunn & Mandelbaum, Reference Quilty-Dunn and Mandelbaum2018a).
Similar variations abound. If the associative account were correct then merely giving a major premise that is clearly valenced should set the associative value of the target: Giving participants sentences such as “If you see a purple pentagon, you can conclude that Ibbonif is malicious” should make one associate IBBONIF and negative valence via “malicious.” Except that isn't what happens – if subjects are given the conditional premise with no follow-up they withhold forming any valenced implicit attitudes, unlike what associative theory would predict.Footnote 10 The concept IBBONIF needs to be linked with the attribute MALICIOUS in a way that is impervious to associative factors, but sensitive to counterevidence. A predicate–argument structure with MALICIOUS as predicate and IBBONIF as argument predicts just this functional profile.
The Kurdi and Dunham is just one of a near-deluge of recent studies showing the efficacy of logical interventions compared to the impotence of associative interventions (Cone & Ferguson, Reference Cone and Ferguson2015; De Houwer, Reference De Houwer2006; Gast & De Houwer, Reference Gast and De Houwer2013; Mann & Ferguson, Reference Mann and Ferguson2015, Reference Mann and Ferguson2017; Mann, Cone, Heggeseth, & Ferguson, Reference Mann, Cone, Heggeseth and Ferguson2019; Van Dessel, De Houwer, Gast, Smith, & De Schryver, Reference Van Dessel, De Houwer, Gast, Smith and De Schryver2016; Van Dessel, Gawronski, Smith, & De Houwer, Reference Van Dessel, Gawronski, Smith and De Houwer2017a; Van Dessel, Mertens, Smith, & De Houwer, Reference Van Dessel, Mertens, Smith and De Houwer2017b; Van Dessel, Ye, & De Houwer, Reference Van Dessel, Ye and De Houwer2019). Telling participants that they will see a pairing of a group with pictures of pleasant (or unpleasant) things is much more effective at fixing implicit attitudes than repeatedly pairing the group and the pleasant/unpleasant things. One-shot learning trumps 37 associative pairings. Even when associative and one-shot propositional learning are combined, the associative trials add no detectable valence to the implicit attitude formed from the one-shot propositional trial (Kurdi & Banaji, Reference Kurdi and Banaji2017). That is, direct exposure to associative pairings isn't necessary or sufficient for forming or changing implicit attitudes, and its effect on attitudes doesn't compare to a single exposure to a sentence. Even when repeated exposure causes some mental representation of the categories to be formed, just telling participants whether the stimuli are diagnostic modulates learning (e.g., if told the data aren't diagnostic, learning is inhibited, and if told the data are diagnostic, learning is increased). This suggests that the representations acquired are being used as beliefs (Quilty-Dunn & Mandelbaum, Reference Quilty-Dunn and Mandelbaum2018b), and updated in a logical, inferentially promiscuous way (Kurdi & Banaji, Reference Kurdi and Banaji2019). The primacy of diagnostic information over repeated exposure is a consistent finding, showing the inadequacies of associative models (e.g., Mann et al., Reference Mann, Cone, Heggeseth and Ferguson2019; Mann & Ferguson, Reference Mann and Ferguson2015, Reference Mann and Ferguson2017).
In short, implicit attitudes – far from being a problem area for LoT – instead demand evidence-sensitive, inferentially promiscuous predicate–argument structures that incorporate abstract logical operators.
7. Conclusion
More than half a century after the cognitive revolution of the 1950s, mental representations remain the central theoretical posits of psychology. Although our picture of the mind has gotten more and more complex over time, computational operations over structured symbols remain foundational to our explanations of behavior. At least some of these symbols – those involved in certain aspects of probabilistic inference, concept acquisition, S1 cognition, object-based and relational perceptual processing, infant and animal reasoning, and likely elsewhere – are couched in an LoT. That doesn't mean that all perceptual and cognitive processing is LoT-symbol manipulation. We believe in other vehicles of thought, including associations (Quilty-Dunn & Mandelbaum, Reference Quilty-Dunn, Mandelbaum, Chan and Nes2020), icons (Quilty-Dunn, Reference Quilty-Dunn2020b), and much more. Our claim is somewhat modest: Many representational formats across many cognitive systems are LoTs.
We don't deny the successes of DCNNs; perhaps they accurately model some aspects of biological cognition (Buckner, Reference Buckner2019; Shea, Reference Shea2023). It remains open that DNNs might mimic the performance of biological perception and cognition across a wide variety of domains and tasks by implementing core features of LoTs (cf. Zhu et al., Reference Zhu, Gao, Fan, Huang, Edmonds, Liu and Zhu2020). We agree with a recent review of DCNNs that a “key question for current research is how structured representations and computations may be acquired through experience and implemented in biologically plausible neural networks” (Peters & Kriegeskorte, Reference Peters and Kriegeskorte2021, p. 1137). Given the evidence above, matching the competences of biological minds will require implementing a class of structured representations that uses discrete constituents to encode abstract contents and organizes them into inferentially promiscuous predicate–argument structures that can incorporate logical operators and exhibit role-filler independence.
There is much more to say about evidence for LoT, including abstract, compositional reasoning in aphasics (Varley, Reference Varley2014), and potential neural underpinnings for LoT (Frankland & Greene, Reference Frankland and Greene2020; Gershman, Reference Gershman2022; Roumi et al., Reference Roumi, Marti, Wang, Amalric and Dehaene2021; Wang et al., Reference Wang, Amalric, Fang, Jiang, Pallier, Figueira and Dehaene2019). LoTs ought to provide “common codes” that interface across diverse systems (Dennett, Reference Dennett and Dennett1978; Pylyshyn, Reference Pylyshyn1973). Central topics here include LoTs at the interfaces of language (Dunbar & Wellwood, Reference Dunbar and Wellwood2016; Harris, Reference Harris2022; Pietroski, Reference Pietroski2018) and action (Mylopoulos, Reference Mylopoulos2021; Shepherd, Reference Shepherd2021).
The big picture is that LoTH remains a thriving research program. LoTH allows us to distinguish psychological kinds in a remarkably fine-grained way, offering promising avenues for future research. LoTs might differ across systems within a single mind, or between species (Porot, Reference Porot2019). Although it's likely, for example, that object tracking and S1 reasoning differ in the representational primitives they employ, we don't know whether or how their compositional principles differ. Similarly, we don't know how representations that guide logical inference in baboons differ from those that bees use in social learning, or that infants use in physical reasoning. Differences in conceptual repertoire or syntactic rules provide dimensions along which to type cognitive systems. Future work can focus on decrypting the specific symbols and transformation rules at work in each case, and how these symbols interface with non-LoT mental media.
One might also find subclusters of LoT-like properties. It may be that, for example, properties encoding logical operators and making abstract logical contents available for inference form a “logic” subcluster, and predicate–argument structure, role-filler independence, and abstract contents form a “predication” subcluster. In that case, LoT qua natural kind may be a genus of which these subclusters are species (as an analogy, consider how mental icons may be a genus-level kind with high species-level variation between, e.g., visual images and abstract mental models).
Finally, little is known about the evolutionary emergence of LoT in our ancestors or phylogenetically distant LoT-based minds. Our ignorance leaves open the possibility that, given LoTs' computational utility, very different biological minds converged on them independently. An outstanding research goal is to construct a typology of LoTs within and across species, allowing us to better understand the varieties of expressive power in naturally occurring representational systems (Mandelbaum et al., Reference Mandelbaum, Dunham, Feiman, Firestone, Green, Harris and Quilty-Dunnunder review).
Acknowledgments
For helpful discussion and comments, we thank Zed Adams, Jake Beck, Ned Block, Luca Bonatti, Tyler Brooke-Wilson, Chiara Brozzo, Susan Carey, Wayne Christensen, Sam Clarke, Jonathan Cohen, Carl Craver, Roman Feiman, Chaz Firestone, Carolina Flores, E. J. Green, Dan Harris, Zoe Jenkin, Benedek Kurdi, Kevin Lande, Manolo Martinez, Michael Murez, David Neely, Shaun Nichols, Jonathan Phillips, Henry Schiller, Nick Shea, Joshua Shepherd, Brent Strickland, Victor Verdejo, audiences at the Institute of Philosophy in London, Washington University in St Louis, University of Barcelona, Nantes University, the San Diego Winter Workshop in Philosophy of Perception, Cornell University, and the joint meeting of the SPP/ESPP. We are grateful to BBS's reviewers for comments which greatly improved the paper.
Competing interest
None.
Target article
The best game in town: The reemergence of the language-of-thought hypothesis across the cognitive sciences
Related commentaries (30)
A language of episodic thought?
Advanced testing of the LoT hypothesis by social reasoning
Animal thought exceeds language-of-thought
Compositionality in visual perception
Concept learning in a probabilistic language-of-thought. How is it possible and what does it presuppose?
Developmental and multiple languages-of-thought
Do nonlinguistic creatures deploy mental symbols for logical connectives in reasoning?
Evidence for LoTH: Slim pickings
Incomplete language-of-thought in infancy
Is core knowledge in the format of LOT?
Is evidence of language-like properties evidence of a language-of-thought architecture?
Is language-of-thought the best game in the town we live?
Language-of-thought hypothesis: Wrong, but sometimes useful?
Linguistic meanings in mind
Linguistic structure and the languages-of-thought
Natural logic and baby LoTH
Neither neural networks nor the language-of-thought alone make a complete game
Never not the best: LoT and the explanation of person-level psychology
On the hazards of relating representations and inductive biases
Perception is iconic, perceptual working memory is discursive
Properties of LoTs: The footprints or the bear itself?
Putting relating at the core of language-of-thought
Representational structures only make their mark over time: A case from memory
Stop me if you've heard this one before: The Chomskyan hammer and the Skinnerian nail
The computational and the representational language-of-thought hypotheses
The language of tactile thought
The language-of-thought as a working hypothesis for developmental cognitive science
The reemergence of the language-of-thought hypothesis: Consequences for the development of the logic of thought
Toward biologically plausible artificial vision
Using the sender–receiver framework to understand the evolution of languages-of-thought
Author response
The language-of-thought hypothesis as a working hypothesis in cognitive science