2076 results in Statistical Physics
Preface
-
- Book:
- Statistical Physics of Liquids at Freezing and Beyond
- Published online:
- 07 September 2011
- Print publication:
- 16 June 2011, pp xiii-xv
-
- Chapter
- Export citation
3 - The satisfiability problem
-
- Book:
- Phase Transitions in Machine Learning
- Published online:
- 05 August 2012
- Print publication:
- 16 June 2011, pp 43-69
-
- Chapter
- Export citation
6 - Searching the hypothesis space
-
- Book:
- Phase Transitions in Machine Learning
- Published online:
- 05 August 2012
- Print publication:
- 16 June 2011, pp 124-139
-
- Chapter
- Export citation
Contents
-
- Book:
- Statistical Physics of Liquids at Freezing and Beyond
- Published online:
- 07 September 2011
- Print publication:
- 16 June 2011, pp vii-xii
-
- Chapter
- Export citation
1 - Statistical physics of liquids
-
- Book:
- Statistical Physics of Liquids at Freezing and Beyond
- Published online:
- 07 September 2011
- Print publication:
- 16 June 2011, pp 1-57
-
- Chapter
- Export citation
Acknowledgments
-
- Book:
- Phase Transitions in Machine Learning
- Published online:
- 05 August 2012
- Print publication:
- 16 June 2011, pp xiii-xiii
-
- Chapter
- Export citation
References
-
- Book:
- Statistical Physics of Liquids at Freezing and Beyond
- Published online:
- 07 September 2011
- Print publication:
- 16 June 2011, pp 540-557
-
- Chapter
- Export citation
9 - The nonequilibrium dynamics
-
- Book:
- Statistical Physics of Liquids at Freezing and Beyond
- Published online:
- 07 September 2011
- Print publication:
- 16 June 2011, pp 443-485
-
- Chapter
- Export citation
8 - The ergodic–nonergodic transition
-
- Book:
- Statistical Physics of Liquids at Freezing and Beyond
- Published online:
- 07 September 2011
- Print publication:
- 16 June 2011, pp 363-442
-
- Chapter
- Export citation
5 - Dynamics of collective modes
-
- Book:
- Statistical Physics of Liquids at Freezing and Beyond
- Published online:
- 07 September 2011
- Print publication:
- 16 June 2011, pp 204-270
-
- Chapter
- Export citation
Index
-
- Book:
- Phase Transitions in Machine Learning
- Published online:
- 05 August 2012
- Print publication:
- 16 June 2011, pp 375-383
-
- Chapter
- Export citation
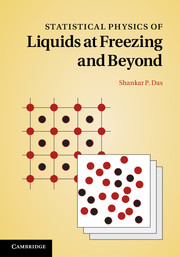
Statistical Physics of Liquids at Freezing and Beyond
-
- Published online:
- 07 September 2011
- Print publication:
- 16 June 2011
13 - Phase transitions in natural systems
-
- Book:
- Phase Transitions in Machine Learning
- Published online:
- 05 August 2012
- Print publication:
- 16 June 2011, pp 313-318
-
- Chapter
- Export citation
Frontmatter
-
- Book:
- Phase Transitions in Machine Learning
- Published online:
- 05 August 2012
- Print publication:
- 16 June 2011, pp i-iv
-
- Chapter
- Export citation
12 - Phase transitions in complex systems
-
- Book:
- Phase Transitions in Machine Learning
- Published online:
- 05 August 2012
- Print publication:
- 16 June 2011, pp 300-312
-
- Chapter
- Export citation
9 - Phase transition in FOL covering test
-
- Book:
- Phase Transitions in Machine Learning
- Published online:
- 05 August 2012
- Print publication:
- 16 June 2011, pp 184-219
-
- Chapter
- Export citation
5 - Machine learning
-
- Book:
- Phase Transitions in Machine Learning
- Published online:
- 05 August 2012
- Print publication:
- 16 June 2011, pp 92-123
-
- Chapter
- Export citation
Notation
-
- Book:
- Phase Transitions in Machine Learning
- Published online:
- 05 August 2012
- Print publication:
- 16 June 2011, pp xiv-xvi
-
- Chapter
- Export citation
Contents
-
- Book:
- The Physics of Foraging
- Published online:
- 05 August 2012
- Print publication:
- 02 June 2011, pp vii-x
-
- Chapter
- Export citation
9 - Optimizing encounter rates
- from Part III - Theory of foraging
-
- Book:
- The Physics of Foraging
- Published online:
- 05 August 2012
- Print publication:
- 02 June 2011, pp 77-84
-
- Chapter
- Export citation