1325 results in Communications, Information Theory and Security
Contents
-
- Book:
- Regulating Cross-Border Data Flows
- Published by:
- Anthem Press
- Published online:
- 22 November 2022
- Print publication:
- 16 August 2022, pp v-vi
-
- Chapter
- Export citation
Preface
-
- Book:
- Regulating Cross-Border Data Flows
- Published by:
- Anthem Press
- Published online:
- 22 November 2022
- Print publication:
- 16 August 2022, pp xi-xvi
-
- Chapter
- Export citation
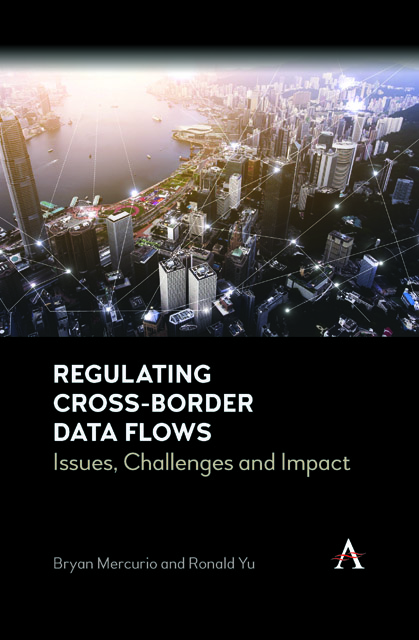
Regulating Cross-Border Data Flows
- Issues, Challenges and Impact
-
- Published by:
- Anthem Press
- Published online:
- 22 November 2022
- Print publication:
- 16 August 2022
Index
-
- Book:
- Regulating Cross-Border Data Flows
- Published by:
- Anthem Press
- Published online:
- 22 November 2022
- Print publication:
- 16 August 2022, pp 95-97
-
- Chapter
- Export citation
5 - Considerations in Moving Forward
-
- Book:
- Regulating Cross-Border Data Flows
- Published by:
- Anthem Press
- Published online:
- 22 November 2022
- Print publication:
- 16 August 2022, pp 59-70
-
- Chapter
- Export citation
2 - Key Considerations
-
- Book:
- Regulating Cross-Border Data Flows
- Published by:
- Anthem Press
- Published online:
- 22 November 2022
- Print publication:
- 16 August 2022, pp 9-18
-
- Chapter
- Export citation
References
-
- Book:
- Regulating Cross-Border Data Flows
- Published by:
- Anthem Press
- Published online:
- 22 November 2022
- Print publication:
- 16 August 2022, pp 71-94
-
- Chapter
- Export citation
3 - Competing Models of Data Governance
-
- Book:
- Regulating Cross-Border Data Flows
- Published by:
- Anthem Press
- Published online:
- 22 November 2022
- Print publication:
- 16 August 2022, pp 19-38
-
- Chapter
- Export citation
Acknowledgements
-
- Book:
- Regulating Cross-Border Data Flows
- Published by:
- Anthem Press
- Published online:
- 22 November 2022
- Print publication:
- 16 August 2022, pp vii-viii
-
- Chapter
- Export citation
Frontmatter
-
- Book:
- Regulating Cross-Border Data Flows
- Published by:
- Anthem Press
- Published online:
- 22 November 2022
- Print publication:
- 16 August 2022, pp i-iv
-
- Chapter
- Export citation
1 - Introduction
-
- Book:
- Regulating Cross-Border Data Flows
- Published by:
- Anthem Press
- Published online:
- 22 November 2022
- Print publication:
- 16 August 2022, pp 1-8
-
- Chapter
- Export citation
List of Abbreviations
-
- Book:
- Regulating Cross-Border Data Flows
- Published by:
- Anthem Press
- Published online:
- 22 November 2022
- Print publication:
- 16 August 2022, pp ix-x
-
- Chapter
- Export citation
3 - Introduction to Data Mining
-
- Book:
- Data Analytics for Cybersecurity
- Published online:
- 10 August 2022
- Print publication:
- 21 July 2022, pp 29-59
-
- Chapter
- Export citation
11 - Future Directions in Data Analytics for Cybersecurity
-
- Book:
- Data Analytics for Cybersecurity
- Published online:
- 10 August 2022
- Print publication:
- 21 July 2022, pp 147-164
-
- Chapter
- Export citation
6 - Anomaly Detection for Cybersecurity
-
- Book:
- Data Analytics for Cybersecurity
- Published online:
- 10 August 2022
- Print publication:
- 21 July 2022, pp 91-100
-
- Chapter
- Export citation
Index
-
- Book:
- Data Analytics for Cybersecurity
- Published online:
- 10 August 2022
- Print publication:
- 21 July 2022, pp 189-192
-
- Chapter
- Export citation
5 - Types of Cyberattacks
-
- Book:
- Data Analytics for Cybersecurity
- Published online:
- 10 August 2022
- Print publication:
- 21 July 2022, pp 78-90
-
- Chapter
- Export citation
References
-
- Book:
- Data Analytics for Cybersecurity
- Published online:
- 10 August 2022
- Print publication:
- 21 July 2022, pp 165-188
-
- Chapter
- Export citation
10 - Human-Centered Data Analytics for Cybersecurity
-
- Book:
- Data Analytics for Cybersecurity
- Published online:
- 10 August 2022
- Print publication:
- 21 July 2022, pp 137-146
-
- Chapter
- Export citation
2 - Understanding Sources of Cybersecurity Data
-
- Book:
- Data Analytics for Cybersecurity
- Published online:
- 10 August 2022
- Print publication:
- 21 July 2022, pp 14-28
-
- Chapter
- Export citation