Introduction
Leishmaniasis is included in the list of WHO as one of the major neglected tropical diseases worldwide (Torres-Guerrero et al., Reference Torres-Guerrero, Quintanilla-Cedillo, Ruiz-Esmenjaud and Arenas2017). The parasitic disease, Leishmaniasis is transmitted to humans by the bite of a parasite-infected, female sandfly (Phlebotomus). Mild Cutaneous Leishmaniasis, serious Mucocutaneous Leishmaniasis and fatal Visceral Leishmaniasis are the three clinical forms of the disease. The parasite, Leishmania donovani is the causative agent for Leishmaniasis in Sri Lanka (Karunaweera et al., Reference Karunaweera, Ginige, Senanayake, Silva, Manamperi, Samaranayake, Siriwardana, Gamage, Senerath and Zhou2020). However, there is currently no ideal vaccine or a drug of choice available to cure any form of Leishmaniasis. Therefore it is an urgent requirement to investigate new oral drugs that are safe and inexpensive to treat Leishmaniasis.
Targeting sterol metabolism of Leishmania donovani is considered as a therapeutic strategy to inhibit its growth. Among enzymes participating in the sterol biosynthesis pathway, squalene synthase has been identified as a potent drug target to develop anti-parasitic drugs. According to the previous studies, two quinuclidine based inhibitors namely E5700 and ER-119884 have shown inhibitory effects against squalene synthase of Trypanosoma cruzi and Leishmania amazonensis (Fernandes Rodrigues et al., Reference Fernandes Rodrigues, Concepcion, Rodrigues, Caldera, Urbina and Souza2008; Urbina et al., Reference Urbina, Concepcion, Caldera, Payares, Sanoja, Otomo and Hiyoshi2004). Therefore the inhibition of Leishmania donovani squalene synthase in sterol metabolism could be a promising therapy to prevent the proliferation of parasites due to the collapse of ergosterol production.
Computational study revealed both natural alkaloids interact with active site residues of LdSQS. These compounds could be further evaluated as potent antileishmanial compounds against LdSQS enzyme.
Objective
In the present study, we attempted to elucidate the three-dimensional structure of Leishmania donovani squalene synthase by homology modeling which is considered to be the most accurate method of structure modeling. The reported inhibitor, E5700 (control) and natural alkaloids were used to discover potential antileishmanial activity against modeled protein by structure-based drug discovery using AutoDock software (Morris et al., Reference Morris, Huey, Lindstrom, Sanner, Belew, Goodsell and Olson2009). This kind of computer-aided drug design method is useful to reduce the burden of laborious work and money wastage in drug development.
Methods
The 3D structure of LdSQS was not publicly available in the RCSB Protein Data Bank (https://www.rcsb.org/). Therefore the amino acid sequence of LdSQS (ID: Q257D4) was retrieved from UniProtKB database (https://www.uniprot.org/). The best homologous template was identified by BLASTp. Both C and N termini, segments of LdSQS which are lack of matching template residues were removed. The final optimal alignment was carried out in Clustal Omega server (Sievers & Higgins, Reference Sievers and Higgins2017). Totally 50 models of LdSQS were generated using Modeller v9.22 (Eswar et al., Reference Eswar, Webb, Marti-Renom, Madhusudhan, Eramian, Shen, Pieper and Sali2006). Out of 50 models, the best model was selected based on the least DOPE score. Further refinement of selected 3D model was performed in the UCSF Chimera v.1.12 (Pettersen et al., Reference Pettersen, Goddard, Huang, Couch, Greenblatt, Meng and Ferrin2004) using quality information from MolProbity server (http://molprobity.biochem.duke.edu/) (Chen et al., Reference Chen, Arendall, Headd, Keedy, Immormino, Kapral, Murray, Richardson and Richardson2009). Final energy minimization (1000 steps Steepest Descent in AMBER force field) was continued to remove clashes. Eventually the refined model was subjected to quality validation using SAVES v5.0 server (https://servicesn.mbi.ucla.edu/SAVES/) and ProSA server (Weiderstein & Sippl, Reference Wiederstein and Sippl2007). The best predicted model was executed for virtual screening. All ligands (Figure 1(C)) except E5700 (reported inhibitor taken from PDB ID: 3WCC) were fetched by PubChem CID into UCSF Chimera. Then hydrogens and gastieger charges were added to all ligands. The binding site residues were determined by redocking of template inhibitor (PDB ID: 3WCC) with the modeled protein. The grid box parameters and binding site residues selected for LdSQS are shown in Table 1(D). Molecular simulation was carried out by AutoDock 4.2 using default parameters. The interactions of docked complexes were analyzed using PLIP server (https://projects.biotec.tu-dresden.de/plip-web/plip) (Salentin et al., Reference Salentin, Schreiber, Haupt, Adasme and Schroeder2015) and visualized in UCSF Chimera. The pkCSM server (http://biosig.unimelb.edu.au/pkcsm/) (Pires et al., Reference Pires, Blundell and Ascher2015) was used to ADMET analysis of all ligands.

Figure 1. (A). The superimposition of template protein 3WCA_A (Blue) and the 3D modeled structure of LdSQS (Green) (RMSD=0.306A°). (B). Sequence alignment of LdSQS (Target) with the template protein PDB ID: 3WCA_A. The conserved residues are represented as asterisk, highly similar residues as colon and similar residues as full stop. (C-E). 3D structures of ligands used for the study. [C] Holamine (PubChem CID: 12310548), [D] E5700 (Reported Inhibitor) (PubChem CID: 56947067), [E] Ancistrotanzanine B (PubChem CID: 10002166)
Table 1. (A). Identification of the best homologous template using BLAST against Protein Data Bank (PDB). (B). Structure validation results of LdSQS (Modeled Protein). (C). Protein-ligand interaction analysis of docked complexes. (D). Grid box parameters selected for LdSQS (Modeled Protein), based on binding site residues (Spacing was set up at 0.375A°). (E). Drug likeness and in silico ADMET (Absorption, Distribution, Metabolism, Excretion and Toxicity) prediction of ligands.

RMSD* – Root Mean Square Deviation
LBE* – Lowest Binding Energy (kcal/mol) , EIC** – Estimated Inhibition constant (Ki)(nM)
VDss* - Volume of Distribution Human, BBB** – Blood Brain Barrier
CYP*** – Cytochrome P450 Enzymes
Results
The BLASTp search identified PDB ID: 3WCA (A chain) as the best homologous template for homology modeling (Table 1(A)). The pairwise alignment (Figure 1(B)) and superimposition of template with final LdSQS model (Figure 1(A)) shows 61.42 % sequence similarity and 0.306A° RMSD respectively. The quality validation of the LdSQS model evaluated via PROCHECK, ERRAT, VERIFY 3D and ProSA, is shown in Table 1(B) & Figure 2(A-E). The validation results prove LdSQS as a good quality model. Following docking simulations, the highest affinity towards LdSQS was observed for E5700 (-9.75 kcal/mol) closely followed by Ancistrotanzanine B (-9.55 kcal/mol) and Holamine (-8.79 kcal/mol) respectively. The reported inhibitor formed three hydrogen bonds with the key residues Val139, Cys255 and Gln259 of LdSQS while Holamine bound to Val139 and Gln259 through hydrogen bond interactions. The best docking alkaloid, Ancistrotanzanine B was stabilized only by hydrophobic interactions. The best binding conformation of each ligand is depicted in Figure 3. All ligands (Figure 1(C)) satisfied Lipinski’s rule of five which is essential for Drug likeness. According to ADMET predictions (Table 1(E)) except Ancistrotanzanine B, all show hepatotoxicity.


Figure 2. (A). ProSA z-score plot of the LdSQS protein. The z-score indicates overall model quality. The z-score value (-10.03) of LdSQS falls in the range of PDB proteins submitted by X ray crystallography (Light Blue), indicating a good quality model. (B). ProSA energy profile plot of the LdSQS protein. The plot shows local model quality by plotting energies as a function of amino acid sequence. The ProSA analysis of the model shows maximum residues in the negative energy region, indicating a reliable and good quality structure. (C). Ramachandran plot of the LdSQS protein built by PROCHECK. The red regions represent the most favorable regions of residues, the yellow areas represent additionally allowed regions, the light yellow regions are generously allowed regions and the white fields represent the disallowed regions. (D). ERRAT plot for the LdSQS protein. Black bars depict the error region above 99%, gray bars indicate the error region between 95% & 99% and white bars demonstrate the error region below 95%. The maximum residues of LdSQS are located below 95% error region. The overall quality factor (94.833) of LdSQS indicates a high resolution structure. (E). VERIFY 3D plot of LdSQS protein. The plot predicts 91.69% of the residues in LdSQS have an averaged 3D-1D score >=0.2, verifying the model.
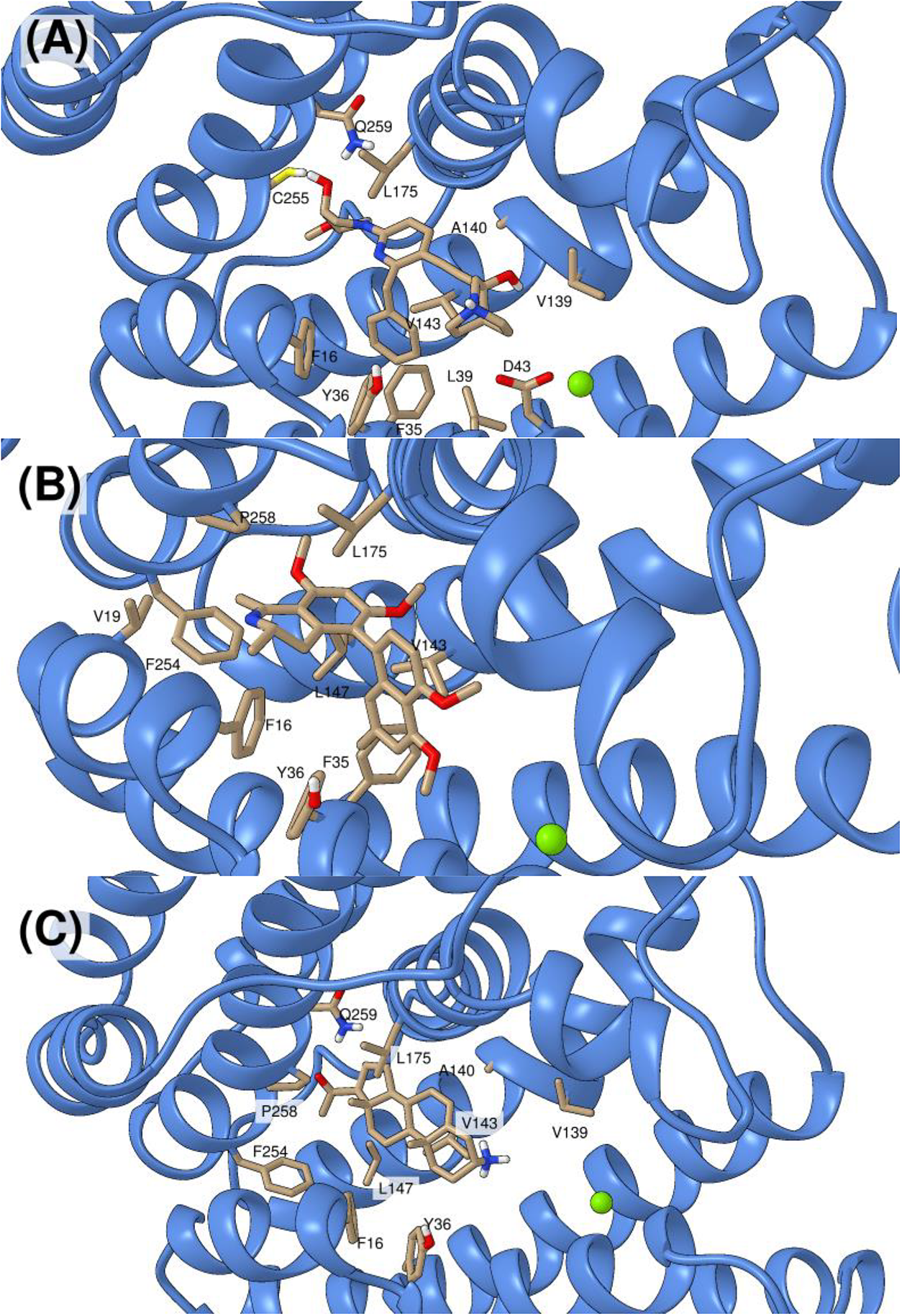
Figure 3 (A-C). Best binding conformation of ligand with LdSQS protein in the binding cavity. These images were generated in UCSF Chimera. (A). Best binding conformation of E5700 (Reported Inhibitor) to LdSQS. (B). Best binding conformation of Ancistrotanzanine B to LdSQS. (C). Best binding conformation of Holamine to LdSQS
Discussions
Due to high sequence identity, query coverage and structure completeness, a single template was selected for modeling of LdSQS. All validation results including ERRAT, PROCHECK, VERIFY3D, ProSA and RMSD were in the acceptable ranges verifying a high quality model. The negative and low binding energy of docked complex demonstrates the strong affinity between LdSQS and the ligand occupying the most favorable conformation. Binding energy of the reported inhibitor (-9.75) was superior to natural alkaloids (-9.55 & -8.79), showing the strongest affinity. Previous studies have reported E5700 (Fernandes Rodrigues et al., Reference Fernandes Rodrigues, Concepcion, Rodrigues, Caldera, Urbina and Souza2008) as an anti-proliferative agent against squalene synthase of Leishmania amazonensis. For the reported inhibitor, experimental data revealed MIC values 30 nM for NR strain and 50 nM for Josefa strain of Leishmania amazonensis promastigotes. The inhibitory effect of E5700 was also confirmed by current docking studies. Interestingly, Ancistrotanzanine B showed binding energy close to E5700 and stabilized only by hydrophobic interactions to LdSQS showing no potential risk of hepatotoxicity.
Conclusions
The 3D structure of LdSQS was evaluated by homology modeling. The generated model was validated stereochemically and structurally by different tools to obtain a good quality model. Then molecular docking was carried out to find binding affinity and molecular interactions between LdSQS and ligands. Virtual screening revealed that the most interacting binding site residues of LdSQS are Phe16, Val19, Phe35, Tyr36, Leu39, Asp43, Val139, Ala140, Val143, Leu147, Leu175, Phe254, Cys255, Pro258 and Gln259.Both natural alkaloids showed close binding affinity compared to reported inhibitor and admissible ADME profile except hepatotoxicity for Holamine. Therefore homology model of LdSQS and natural alkaloids can be further structurally optimized to design potent inhibitors with antileishmanial activity.
Acknowledgements
The author would like to thank Prof. Nadira Karunaweera, Department of Parasitology, Faculty of Medicine, University of Colombo, Sri Lanka, for the help and support.
Author Contributions
I confirm that I carried out all the work and no other co-worker was involved. The author read and approved the final manuscript.
Funding Information
This research received no specific grant from any funding agency, commercial or not – for- profit sectors.
Conflict of Interest
The author declares no conflict of interest.
Data Availability
The author confirms that the data supporting the findings of this study are available within the article.
Comments
Comments to the Author: A more in depth description of limitations and future work is required. Larger space should be given to the survey of the state of the art.
Proofreading is required (sometimes, there is no space between words)