Policy Significance Statement
This paper focuses on standard-making for robotic technologies, particularly in the domains of personal care, rehabilitation, and agriculture. Its significance is threefold. First, it defends the importance of informing policies with the knowledge generated when robots are developed. Second, it introduces LIAISON, which aims to explore to what extent compliance tools could serve as data generators to unravel an optimal regulatory framing for robot technologies. Third, it presents some of the main findings and lessons learned from evidence-based policymaking for emerging robotics that the project has found and discusses.
1. Introduction
Society is being automated. From milking and farming robots that support resource efficiency and increase productivity in agriculture (Driessen and Heutinck, Reference Driessen and Heutinck2015; Aravind et al., Reference Aravind, Raja and Pérez-Ruiz2017) to warehouse robots that allow faster, better, and more efficient logistics (Bogue, Reference Bogue2016), technology is transforming society (European Parliament, 2020). As Healy (Reference Healy and Khan2012) highlights, technological transformation inevitably has consequences, some of which are anticipated, intended, and desirable, and others that are unanticipated and undesirable, or whichever form it takes (i.e., unanticipated and desirable). While the benefits abound, the increasingly direct interaction between humans and machines and the direct control that machines exert over the world inevitably have potentially far-reaching consequences. For instance, they can cause harm in a way that humans cannot necessarily correct or oversee (Amodei et al., Reference Amodei, Olah, Steinhardt, Christiano, Schulman and Mané2016; Hendrycks et al., Reference Hendrycks, Carlini, Schulman and Steinhardt2021), their continuous use could increase isolation, deception, and loss of dignity (Sharkey and Sharkey, Reference Sharkey and Sharkey2012), and they may call into existence discriminatory responses that are not always sufficiently transparent and may seriously affect society, among which minority populations (Zarsky, Reference Zarsky2016; Felzmann et al., Reference Felzmann, Fosch-Villaronga, Lutz and Tamò-Larrieux2019).
Although a growing body of literature highlights these legal and ethical consequences robots raise (Wynsberghe, Reference Van Wynsberghe2016; Leenes et al., Reference Leenes, Palmerini, Koops, Bertolini, Salvini and Lucivero2017; Fosch-Villaronga, Reference Fosch-Villaronga2019), robot developers struggle to always and adequately integrate these dimensions into their creations. Often, there are information asymmetries among developers regarding the legal and ethical boundaries to be respected for such new creations that, in theory, aim to solve problems (Cihon et al., Reference Cihon, Kleinaltenkamp, Schuett and Baum2021). This may be because, as Carr (Reference Carr2011) highlights, technology developers are so focused on solving a problem that they are oblivious to the broader consequences of their technology. Also, technology developers often times initially focus on creating a product and successfully bringing it to market, before they take into consideration the wider ethical and legal concerns—including, for instance, privacy and data protection, and diversity and inclusion consideration—that their creations may raise (Fosch-Villaronga and Drukarch, Reference Fosch-Villaronga and Drukarch2020; Søraa and Fosch-Villaronga, Reference Søraa and Fosch-Villaronga2020). Even where clear policies are in place to prevent or mitigate any such risks, technology developers experience difficulties in translating these oftentimes abstract codes of conduct into development decisions or they fail to incorporate other aspects than mere physical safety into their robot design to ensure their devices are comprehensively safe. All of this while assistive robots interact with users socially and with vulnerable populations that may require other safeguards to ensure the human–robot interaction is safe, including trust, cybersecurity, and cognitive aspects (Fosch-Villaronga and Mahler, Reference Fosch-Villaronga and Mahler2021; Martinetti et al., Reference Martinetti, Chemweno, Nizamis and Fosch-Villaronga2021). Moreover, technology users generally tend to be preoccupied with the benefits and gains obtained from new technologies than with whether and, if so, how they affect them negatively (Carr, Reference Carr2011).
At the same time, however, policymakers (i.e., public, private, and third sector organizations, characterized by complex interactions and varied motivations and interests, all involved in “multi-level governance,”, that make and implement policies [Marks and Hooghe, Reference Marks and Hooghe2003]) are not always sufficiently well-equipped to address the legal and ethical concerns raised by the introduction of new technologies or, at least, in a way and through tools that are suitable and understandable for technology developers. As such, an increasing gap has come to exist between the policy cycle’s speed and that of technological and social change (Marchant et al., Reference Marchant, Allenby and Herkert2011; Sucha and Sienkiewicz, Reference Sucha and Sienkiewicz2020), which is especially becoming wider and more prominent in the field of robotics and artificial intelligence (AI) (Calo et al., Reference Calo, Froomkin and Kerr2016; Palmerini et al., Reference Palmerini, Bertolini, Battaglia, Koops, Carnevale and Salvini2016; Leenes et al., Reference Leenes, Palmerini, Koops, Bertolini, Salvini and Lucivero2017; Wischmeyer and Rademacher, Reference Wischmeyer and Rademacher2020). Regulations often fail to frame technological developments accurately, and the regulatory landscape is currently populated by a myriad of fragmented regulations, abstract codes of conduct, and ethical guidelines. This all demands the development of “new legal measures and governance mechanisms (…) to be put in place to ensure adequate protection from adverse impact as well as enabling proper enforcement and oversight, without stifling beneficial innovation” (High-Level Expert Group on AI (AI HLEG), 2019).
To address this issue and in line with this increasingly more prominent call to action, the H2020 COVR Project, which stands for “Being safe around collaborative and versatile robots in shared spaces,” aims to present detailed safety assessment instructions to robot developers and make the safety assessment process clearer and simplerFootnote 1, which may allow, in turn, robots to be used in a more trustworthy and responsible way. In this sense, this EU-funded project sought to develop a tool to better equip robot developers with knowledge about laws and standards that is relevant for them throughout the development of their creations. To this end, the H2020 COVR consortium created the COVR Toolkit (“toolkit”),Footnote 2 an online software application that, among other things, aims at aiding developers in the identification of legislation and standards that are relevant to them in the framing of their robot development process and eventual product outcome. More specifically, the toolkit compiles safety regulations for collaborative robots or cobots, that is, robots developed to work in close proximity with humans (Surdilovic et al., Reference Surdilovic2011) in a variety of domains, among which manufacturing, agriculture, and healthcare.
Compliance tools, such as the toolkit, represent a practical step toward bridging legal knowledge gaps among developers. Still, while these tools may help robot developers in their efforts toward robot legal compliance, new robot applications may nevertheless fail to fit into existing (robot) categories. A “feeding robot,” for instance, may be composed of a robotic wheelchair, an industrial arm, and a feeding function (Herlant, Reference Herlant2018) and may be difficult to classify in existing laws and regulations that cover wheelchairs, industrial arms, but not such a complex cyber-physical system. Moreover, current standards (e.g., ISO 13482:2014 Personal Care Robots), laws (e.g., Medical Device Regulation, 2017b), and proposed regulations (e.g., AI Act, 2021) which are often technology-neutral are oftentimes enacted at a time when practices (if any) are at early stages of implementation and impacts are still unknown, often resulting in dissonances about their protected scope (Fosch-Villaronga, Reference Fosch-Villaronga2019; Winfield, Reference Winfield2019; Salvini et al., Reference Salvini, Paez-Granados and Billard2021).Footnote 3 Providing developers with legal information that may be outdated or unclear may do little to help them integrate these considerations into their R&D processes and may have ulteriorly adverse effects once their technologies are put to use in practice.
It is in this state of affairs that the LIAISON project has been set in motion.Footnote 4 LIAISON stands for “Liaising robot development and policymaking to reduce the complexity in robot legal compliance” and received the COVR Award, which is a Financial Support to Third Parties (FSTP) from the H2020 COVR Project.Footnote 5 LIAISON departs from the idea that developers may identify legal inconsistencies among regulations or may call into existence new categories of devices that struggle to fit any of the categories as established in the law during the legal compliance process. At the same time, patient organizations and other actors may identify other safety requirements (physical and psychological alike) which remain uncovered in existing legislation but that are nevertheless essential to cover in order to protect user safety. It is against this background that LIAISON has put forward the idea that this currently uncaptured knowledge could be formalized and serve as data to improve regulation. In other words, following the ideal that lawmaking “needs to become more proactive, dynamic, and responsive” (Fenwick et al., Reference Fenwick, Kaal and Vermeulen2016), LIAISON attempts to formalize a communication process between robot developers and regulators from which policies could learn, thereby channeling robot policy development from a bottom-up perspective (Fosch-Villaronga and Heldeweg, Reference Fosch-Villaronga and Heldeweg2018, Reference Fosch-Villaronga, Heldeweg and Pons-Rovira2019). To test its model, LIAISON focuses on personal care robots (ISO 13482:2014), rehabilitation robots (IEC 80601–2–78–2019), and agricultural robots (ISO 18497:2018).
This contribution explains the inner workings and findings of the LIAISON project. After a short introduction, Section 2 frames the inefficiency and inadequacy of emerging robot governance. Section 3 introduces the LIAISON model and methodology, thereby highlighting the theoretical model envisioned and its practical application. Section 4 highlights the essential findings and lessons learned from building a dynamic framework for evidence-based robot governance as derived throughout the LIAISON project. The paper concludes with a summary of the achieved results and their potential impact on the governance of emerging technologies.
2. Aligning Robot Development and Regulation: A New Model
2.1. Difficulties in regulating emerging robotics
New technologies are a representation of the progress of science, offering possibilities until recently unimaginable and solving problems faster, better, and more innovative than humans have ever been able to do. Our legal system is characterized by our drive to regulate almost everything we perceive as human beings and even far beyond, creating a so-called horror vacui system, thereby preventing legal lacunae from presenting themselves and ensuring legal certainty at all times. Consequently, our legal system has produced many laws covering a wide range of phenomena and developments, including newly developed technologies such as robot technologies. As products, robots widely differ in embodiment, capabilities, context of use, intended target users, and many regulations that may already apply to them (Leenes et al., Reference Leenes, Palmerini, Koops, Bertolini, Salvini and Lucivero2017). While the benefits abound, however, new technologies inevitably disrupt how we conceive reality, leading us to question and challenge existing norms and push us toward an increasingly louder call for legal change. While technology’s pace dramatically accelerates, however, (adequate) legal responsiveness does not always follow as a consequent step (Marchant and Wallach, Reference Marchant and Wallach2015). The rapid automation of processes once performed by humans creates a regulatory disconnect in which “the covering descriptions employed by the regulation no longer correspond to the technology” or “the technology and its applications raise doubts as to the value compact that underlies the regulatory scheme” (Brownsword, Reference Brownsword2008; Brownsword and Goodwin, Reference Brownsword and Goodwin2012). New robots may not fit into existing (robot) categories, legislation might be outdated and include confusing categories, and technology-neutral regulations may be hard to follow for developers concerned about their particular case (Fosch-Villaronga, Reference Fosch-Villaronga, Wenger, Chevallereau, Pisla, Bleuler and Rodić2016; European Parliament, 2017a). The regulatory challenges arising from the novelty of the technology is a problem often disregarded among scientists (Yang et al., Reference Yang, Bellingham, Dupont, Fischer, Floridi, Full, Jacobstein, Kumar, McNutt, Merrifield and Nelson2018), although it may have ulterior consequences for safety, security, and dignity (Fosch-Villaronga, Reference Fosch-Villaronga, Heldeweg and Pons-Rovira2019).
An illustrative example of this can be found in the domain of healthcare robotics. From exoskeletons to surgery robots and therapeutic robots, healthcare robots challenge the timeliness of laws and regulatory standards that were unprepared for robots that, for instance, would help wheelchair users walk again (Tucker et al., 2015), perform surgeries autonomously (Shademan et al., Reference Shademan, Decker, Opfermann, Leonard, Krieger and Kim2016), or support children under autism spectrum disease in learning emotions (Scassellati et al., Reference Scassellati, Admoni and Matarić2012; Fosch-Villaronga and Heldeweg, Reference Fosch-Villaronga, Heldeweg and Pons-Rovira2019). Furthermore, current legal frameworks tend to overfocus on physical safety but fail to account for other essential aspects like security, privacy, discrimination, psychological aspects, and diversity, which play a crucial role in robot safety. Consequently, healthcare robots generally fail to provide an adequate level of safety “in the wild” (Gruber, Reference Gruber2019). Moreover, healthcare robots increased levels of autonomy and complex interaction with humans blur practitioners and developers’ roles and responsibilities and affect society (Carr, Reference Carr2011; Yang et al., Reference Yang, Cambias, Cleary, Daimler, Drake, Dupont and Taylor2017; Boucher et al., Reference Boucher, Bentzen, Lațici, Madiega, Schmertzing and Szczepański2020; Fosch-Villaronga et al., Reference Fosch-Villaronga, Khanna, Drukarch and Custers2021). In another example, a recent consultation stressed that European harmonized standards do not cover automated vehicles, additive manufacturing, collaborative robots/systems, or robots outside the industrial environment (Spiliopoulou-Kaparia, Reference Spiliopoulou-Kaparia2017).
That the speed of technological change challenges regulation is not new. This all makes it highly unsurprising that robot developers struggle to find responses to the arisen challenges of their creations in existing regulations. Robots are complex devices, often combining hardware products, like actuators, with software applications and digital services. Consequently, technology-neutral regulation might fail to squarely frame their development and clarify the interplay between different instruments. It may also lack safeguards for emerging risks. For instance, the impact assessment on the Machinery Directive 2006/42/EC revision highlighted how developers must take several pieces of legislation for the same product to ensure its compatibility with all the applicable norms. Given this room for overlap, “there is a risk of applying the wrong piece of legislation and the related voluntary standards, thus negatively influencing safety and compliance of the product” (European Commission, 2020). This remark explains why legal requirements often play a marginal role in robotics. Instead, the self-perception of safety risks and economic concerns often guides developers, who feel pressured to rely on insufficient testing (Van Rompaey et al., Reference Van Rompaey, Jønsson and Jørgesen2021). Developers also rely on harmonized standards, such as ISO 13482:2014, which show compliance with existing pieces of legislation (in this case, with the Machinery Directive).Footnote 6 Oftentimes, however, these standards are not enacted inclusively and lack representation of affected users. Therefore, developers may comply with legislation that does not frame these developments correctly (Fosch-Villaronga, Reference Fosch-Villaronga, Wenger, Chevallereau, Pisla, Bleuler and Rodić2016; Fosch-Villaronga and Virk, Reference Fosch-Villaronga, Virk, Rodić and Borangiu2016; Salvini et al., Reference Salvini, Paez-Granados and Billard2021).
However, this encourages us to rethink how we can best identify the need to regulate new technologies. Regulation is a very complex concept that calls for a delicate interplay between various constraints, including the plurality and de-centeredness of our legal systems, the unclear fit of the regulated reality to the new development, and unforeseeable impacts of such emerging technology. Being one of the four pillars that make up the regulatory model, legislation frames the rules of power and society’s conduct by establishing rights and obligations for the subjects within a legal system, and it evolves as society changes, including technological developments. Yet, while both technology and regulation evolve, they do not always do so simultaneously or in the same direction (Holder et al., Reference Holder, Khurana, Harrison and Jacobs2016; Newlands et al., Reference Newlands, Lutz, Tamò-Larrieux, Fosch-Villaronga, Scheitlin and Harasgama2020).
A recurrent question is how the law keeps up with such technological advances. Particularly, those advances that have unintended consequences that have ulterior adverse impacts on society and the extent to which there are remedies in place to address those consequences and, potentially, reverse those impacts (Sabel et al., Reference Sabel, Herrigel and Kristensen2018). Premature and obtrusive regulation might cripple scientific advancement and prevent potential advantages from materializing (Brundage and Bryson, Reference Brundage and Bryson2016). This problem might result from ill-informed interventions, where policymakers rush to develop regulatory pieces without sufficient data on the targeted development (Leenes et al., Reference Leenes, Palmerini, Koops, Bertolini, Salvini and Lucivero2017). The lack of a predictable environment and uncertainty regarding the impacts may disincentivize the development and introduction of emergent technologies. However, the ever-present conviction that technological fixes contribute to societal progress usually prevails in the techno-political discourse (Johnston, Reference Johnston2018). All of this depicts an image of the current regulatory environment being characterized by a lacking process for communication between technology developers and regulators or policymakers, multiple regulatory bodies with mismatching interests, and the absence of an understanding of the exact gaps and inconsistencies in existing robot regulatory frameworks. This all leads to a situation in which neither the regulator nor the robot developers and addressees seem to know exactly what needs to be done while user rights might be at stake in any case.
Traditionally, juridical analysis follows top-down approaches to address legal and ethical aspects for robot technologies (Leroux and Roy, Reference Leroux and Roy2012; Leenes et al., Reference Leenes, Palmerini, Koops, Bertolini, Salvini and Lucivero2017). Moreover, there are initiatives that promote reflection upon the consequences of the outcomes of technological research and development (R&D), fostering the incorporation of such reflections into the research or the design process (Stahl and Coeckelbergh, Reference Stahl and Coeckelbergh2016). However, these approaches often presume that the existing laws and norms suffice to understand the consequences of technology. However, research continuously highlights how current norms are unfit for technological progress. As Wischmeyer and Rademacher (Reference Wischmeyer and Rademacher2020) put it, “while the belief that something needs to be done is widely shared, there is far less clarity about what exactly can or should be done, or what effective regulation might look like,” an uncertainty that unfortunately is at the expense of user rights (Fosch-Villaronga and Heldeweg, Reference Fosch-Villaronga and Heldeweg2018). To the eyes of the European Institutions, the High-Level Expert Group on AI (AI HLEG) (2019) highlighted that these technologies demand “new legal measures and governance mechanisms (…) to be put in place to ensure adequate protection from adverse impact as well as enabling proper enforcement and oversight, without stifling beneficial innovation” (HLEG AIa, 2019). In this vein, field knowledge and lessons learned from developers could fuel the improvement of frameworks governing relevant technologies (Weng et al., Reference Weng, Sugahara, Hashimoto and Takanishi2015; Fosch-Villaronga and Heldeweg, Reference Fosch-Villaronga and Heldeweg2018). Generating information about legal inconsistencies, dissonances, and new categories and linking it with the policy cycle is instrumental in providing policymakers with knowledge of unregulated and underestimated challenges (Hert, Reference Hert2005), and in general, of what requires regulatory attention and, thus, speed the creation, revision, or discontinuation of norms governing robot technology, increase their effectiveness in ensuring overall safety, and ensuring legal certainty in a fast-paced changing environment like robotics (Weng et al., Reference Weng, Sugahara, Hashimoto and Takanishi2015; Fosch-Villaronga and Heldeweg, Reference Fosch-Villaronga and Heldeweg2018).
2.2. LIAISON: Liaising robot development and policymaking
LIAISON contributes to this approach by engaging with representatives from the industry, standardization organizations, and policymakers to unravel the inconsistencies, dissonances, and inaccuracies of existing regulatory efforts and initiatives toward framing robot technologies. Following the belief that lawmaking “needs to become more proactive, dynamic, and responsive” (Fenwick et al., Reference Fenwick, Kaal and Vermeulen2016), LIAISON proposes the formalization of a communication process between robot developers and regulators from which policies could learn, thereby channeling robot policy development from a bottom-up perspective toward a hybrid top-down/bottom-up approach. As such, LIAISON conceives an effective way to extract compliance and technical knowledge from compliance tools (i.e., tools that assist in the process of complying with the applicable legislation), and direct it to policymakers to assist them in unraveling an optimal regulatory framing (including change, revise, or reinterpret) for existing and emerging robot technologies. In particular, LIAISON focused on personal care robots (ISO 13482:2014), rehabilitation robots (IEC 80601–2–78–2019), and agricultural robots (ISO 18497:2018), thereby aiming to uncover any gaps and inconsistencies in different domains and, additionally, gain insight into the usefulness and perceived value of the novel robot governance mechanism it introduces.
Similar to LIAISON, other approaches have also defended the importance of harnessing experimentation as a source of information to devise better regulations (Weng et al., Reference Weng, Sugahara, Hashimoto and Takanishi2015; Shimpo, Reference Shimpo2018; Calleja et al., Reference Calleja, Drukarch and Fosch-Villaronga2022). Moreover, the prospect of regulatory sandboxes for particular applications has raised vivid discussions, particularly in Europe (European Parliament, 2022; Ranchordás, Reference Ranchordás2021; Truby et al., Reference Truby, Brown, Ibrahim and Parellada2022). LIAISON’s model is compatible with those processes, as it also aims at developing models to align robot development and regulation. However, LIAISON stands out for two different reasons. First, it expands the exchange to incorporate compliance tools. That broadening allows targeting different stages of robot development, not only testing phases but also earlier processes like conceptualization and design. Furthermore, it facilitates going beyond identifying new legal issues. LIAISON’s idea is to use the feedback obtained to optimize the regulatory framework, which is a more comprehensive goal, for it also includes, among others, addressing inconsistencies and confusing categories in technical standards or identifying ethical challenges not necessarily needing a legal response. Second, as envisioned in LIAISON, compliance toolkits are not merely information sources. The idea is to further exploit them as platforms for interactive engagement between policymakers and robot developers. Central to it is the notion of facilitating coordination and exchange between different stakeholders, including also academia, industry organizations, and the community. LIAISON’s overarching goal is thus to devise a richer ecosystem of interaction to align policymaking and technological development.
3. The Inner Workings of LIAISON: Methods and Knowledge Extraction Mechanisms
As depicted in Figure 1, LIAISON conceives an effective way to extract compliance and technical knowledge from the COVR Toolkit and direct these data to policy and standard makers to unravel an optimal regulatory framing, including decisions to change, revise, or reinterpret existing regulatory frameworks for existing and emerging robot technologies. More practically, LIAISON’s objective is to clarify what regulatory actions policy and standard makers should take to provide compliance guidance, explain unclear concepts or uncertain applicability domains to improve legal certainty, and inform future regulatory developments for robot technology use and development at the European, National, Regional, or Municipal level (Fosch-Villaronga and Drukarch, Reference Fosch-Villaronga and Drukarch2020). This is achieved through the LIAISON model, which is depicted in Figure 1 below. In general terms, the LIAISON model puts forward a threefold model through which by (a) interacting with compliance tools (in this case in interaction with the COVR Toolkit, but it could also be in interaction with the Assessment List of Trustworthy AI (ALTAI) model developed by the EC)Footnote 7; (b) extracting knowledge from them in partnership with developers and other actors; and (c) sharing this knowledge with engaged regulators to support regulatory action, we can govern robot technology in a more effective way (Fosch-Villaronga and Heldeweg, Reference Fosch-Villaronga and Heldeweg2018, Reference Fosch-Villaronga, Heldeweg and Pons-Rovira2019).
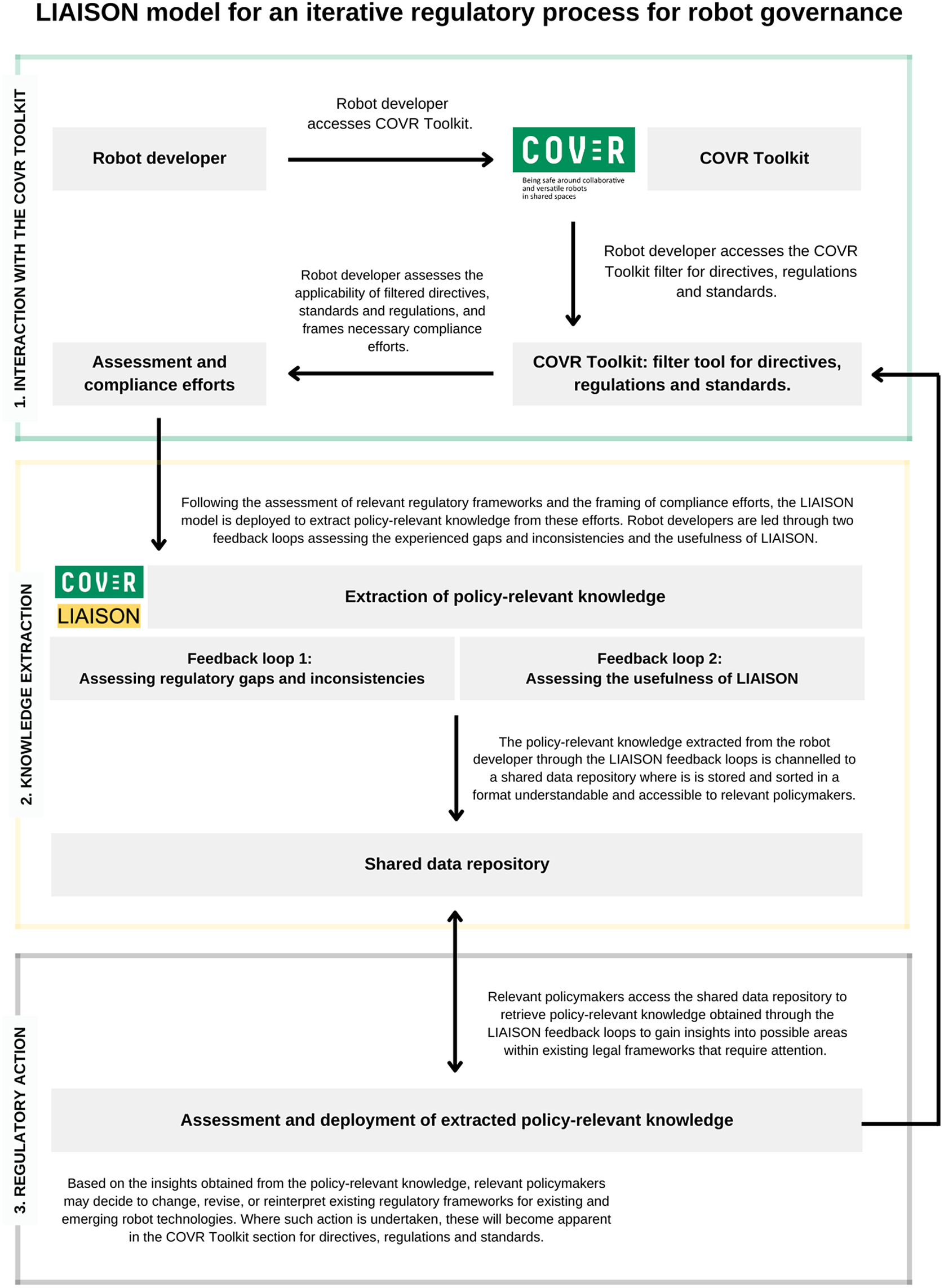
Figure 1. LIAISON model for an iterative regulatory process for robot governance.
Although the LIAISON model depicted in Figure 1 illustrates the model envisioned for practical use in the future, our research focuses on the early exploration of its effectiveness and usefulness. Although aligned with the LIAISON model, the methodologies used for this purpose deviate from the steps in the above figure to some extent. During the LIAISON project, several methods were utilized to identify and map inconsistencies, confusing categories, and uncovered challenges in the chosen regulatory frameworks, namely desktop research, surveys, workshops, community engagement, and exploratory meetings. The following subsections explain in more depth the methods and knowledge extraction mechanisms used throughout the LIAISON project.
3.1. Desktop research
LIAISON aims to uncover the gaps and inconsistencies in the relevant policy documents. To this end, we conducted a desktop research and literature review about robots’ safety compliance landscape (Fosch Villaronga and Drukarch, Reference Fosch and Drukarch2021b). We reviewed existing research on and evaluations of the chosen safety standards. These sources include research articles, web pages, and product catalogs, retrieved from online academic databases and web search results on Google and Google Scholar. The findings resulting from this research indicated that standards governing robots in different domains could benefit from improvement in the broadest sense of the word and highlighted specific areas which may require further investigation (Fosch-Villaronga et al., Reference Fosch-Villaronga, Khanna, Drukarch and Custers2021; Salvini et al., Reference Salvini, Paez-Granados and Billard2021).
We compiled these findings and further validated the preliminary results with exploratory meetings and formal engagements with representatives from standardization organizations (International Standard Organization) and public policymakers (European Commission). As part of LIAISON, three formal meetings were held with relevant policy and standard makers at an early stage of the project to explore how the LIAISON model is perceived by them and how they can contribute to LIAISON in helping relevant policy and standard makers frame robot development adequately. The policy and standard makers involved for this purpose represent both private standardization organizations and the European Commission.Footnote 8 The purpose of these formal meetings was to present relevant policymakers with the idea of putting in place compliance tools as a potential source of data for policy purposes and action, thereby ultimately aiming to understand what information would be helpful and relevant to them in their activities toward framing robot technologies from a policy perspective (Fosch Villaronga and Drukarch, Reference Fosch Villaronga and Drukarch2021d).
3.2. Feedback loops: Survey distribution
The findings derived from this validated desktop research formed the basis of the first and second feedback loops as depicted in Figure 1 above. The surveys that were prepared to this end were intended to engage with the broader community of relevant stakeholders (i.e., robot developers, private and public policymakers, representatives from academia, ethicists, and representatives from interest groups). The purpose of the surveys in the first feedback loop was not only to ask these stakeholders questions to validate our findings but also to help us identify and map existing inconsistencies, confusing categories, and uncovered challenges they experienced in relation to ISO 13482:2014 (on personal care robots), IEC 80601-2-78:2019 (on rehabilitation robotics), and ISO 18497:2018 (on agricultural machinery and tractors). These feedback surveys covered a general assessment of the standards, including usability and satisfaction, language, and layout. The survey also asked respondents to evaluate their experienced inconsistencies and incompleteness in the relevant standards. The third part of the survey gave the possibility to respondents to express their concerns about the standard, and how would they improve them so we could transmit this feedback further. Moreover, the second feedback loop covered a survey that aimed to assess the usefulness of the LIAISON model as a mechanism to better align robot development and policymaking activities. Iterations to the surveys were made following a feedback round among the relevant policymakers which whom we interacted at an early stage in the project to understand what knowledge would be relevant to them and how they perceived the LIAISON model. Links to the surveys in both feedback loops following iteration can be found in Table 1 below.
Table 1. Liaison surveys

The online surveys were distributed across the networks built by the two major European Digital Innovation Hubs (DIH) on Healthcare Robots and on agricultural robots, from now on DIH HEROFootnote 9/DIH AGROBOFOOD).Footnote 10 Within these DIH, we have engaged with involved researchers and work package leaders on standardization and ethics. Moreover, we have established collaboration between LIAISON and both DIH to engage their respective communities in the LIAISON activities. This included their active support in the distribution and refinement of the above feedback surveys, a collaborative workshop at the European Robotics Forum (ERF)Footnote 11, and possibilities for further joint domain-specific webinars at a later date, domain-specific discussion on identified issues in current robot regulatory frameworks (e.g., CEMA industry expert discussion on ISO 18497:2018). Finally, we have further expanded on the partnership with the COVR Project for the same purposes.
We have also engaged with the wider community through a variety of social media platforms, including Twitter and LinkedIn. These interactions included the sharing of the surveys and sending out invitations for the dedicated workshops, and community engagement activities which are further described in Section 3.3. Additionally, the call for participation was featured in the “Exoskeleton Report”.Footnote 12 To further stress our outreach, we actively engaged with relevant stakeholders in our networks (including robot developers, policymakers, and academia) to expand the reach of LIAISON. Examples include collaborations with PAL Robotics,Footnote 13 the Robotics4EU Project,Footnote 14 which aims to increase awareness about ethics, legal, socio-economic, cybersecurity, data protection and further non-technological aspects of robotics, Agreenculture,Footnote 15 a French company that designs, develops, and produces autonomous solutions for the agricultural world, the European Agricultural Machinery Association (CEMA),Footnote 16 EC representatives, academia, and the wider community present at the above ERF workshopsFootnote 17.
3.3. Intensifying knowledge collection: Dedicated workshops in key venues
To avoid low response rates and increase the focus of the obtained responses, the LIAISON project organized a set of interactive workshops, community engagement activities, and formal meetings which included the organization of three dedicated workshops and presentations at the ERF (13–15 April 2021), and the European Commission workshops on “Trends and Developments in Artificial Intelligence: Standards Landscaping and Gap Analysis on the Safety of Autonomous Robots” (2–3 March 2021). Furthermore, we convened several formal engagements with the respective DIH communities, the industry, academia, policymakers, and industry associations to this end.Footnote 18 These dedicated efforts offered an opportunity to explore the potential of extracting policy-relevant knowledge through a more interactive presentation of the surveys that formed part of the feedback loops.
3.4. Data collection and redirection
The data retrieved from the relevant surveys—the responses provided to the relevant survey questions—have been channeled to a so-called “shared data repository,” currently comprising a comprehensive Google sheets file. This shared data repository has formed the basis for the creation of domain-specific recommendations to the relevant policymakers, as engaged with at the start of the project. Moreover, the retrieved data also formed the basis for well-informed recommendations on the value and usefulness of the LIAISON model as a means to improve the adequacy of robot policies more generally. These recommendations were based on an in-depth analysis of the responses to the surveys and those obtained through the interactions during the interactive workshops, community engagement activities, and formal meetings highlighted in Section 3.3. Any policy changes implemented on the basis of data-driven recommendation put forward as part of the LIAISON project will, then, be presented in the COVR Toolkit as the presented standards, directives, and regulations, allowing the envisioned iterative regulatory process for robot governance to restart (i.e., the robot policy selection and legal compliance stage). For the purpose of this paper, the results obtained through the feedback loops have been analyzed to provide a set of general recommendations as to the perceived value of the LIAISON model among relevant stakeholders, and specific recommendations as to the perceived gaps and inconsistencies in the standards that we have investigated in the context of the LIAISON project.
4. Key Findings, Lessons Learned, and Discussion from the LIAISON ProjectFootnote 19
This section discusses the key findings, lessons learned, and discussion following from putting the LIAISON model into practice. Table 2 below provides an overview of these findings and will be elaborated in the following subsections.
Table 2. Key findings, lessons learned, and discussion from the LIAISON project

4.1. A mere website showing regulations is not enough help to robot developers comply
Robot manufacturers deal with many different legal frameworks, including standards and regulations. While compliance tools like the H2020 COVR Toolkit can help in this respect, the platform leaves room for desire: especially for new robot manufacturers, clarifying the applicable legal framework would help reduce the complexity in robot legal compliance. For instance, the COVR Toolkit does not distinguish between directives, which target EU member states and potentially lead to national legislation, and regulations, which are directly applicable. Furthermore, it does not explain the relationship between standards (usually non-binding) and the law (directives/regulation). Consequently, it is not clear what binding legal frameworks robot developers ought to comply with and which recommended frameworks concretize abstract legal principles.
Since current laws do not necessarily target specific types of robots, it is crucial to distill abstract standards into particular requirements capable of guiding developers in the development of a specific robot type. At present, the platform is limited to a small number of robot types and lacks a regulatory model personalized to each robot type, missing the opportunity to present developers with a clear picture of which laws apply to their robot technology. Logically, the platform is not intended to provide legal advice and may want to avoid any liability resulting from it.
In this vein, the platform is unidirectional: it merely presents legal information. This has the problem that, first, it needs to be updated and maintained with relevant developments. Most importantly, however, there is no engagement or feedback envisioned with the community. Following our engagement with the DIH, DIH-HERO, and DIH-AgROBOfood, it became clear that the LIAISON model can serve as a means to strengthen the ecosystem created by these European initiatives by engaging them directly for governance purposes. Such an engagement could help identify dissonances and share lessons learned and tips from developers, which could be more valuable than a static information provision and allow developers to make their voice heard in robot governance activities (Fosch Villaronga and Drukarch, Reference Fosch Villaronga and Drukarch2021c). Over time, the generated knowledge, stored and made available through a shared data repository (see Section 3.4 above), could be helpful to policymakers to enact policies more attuned to stakeholder needs and rights. Eventually, the COVR compliance tool could further include technical solutions to facilitate the interaction between developers and policymakers. The importance and added value of combining the policy appraisal mechanism introduced through LIAISON with the COVR compliance tool has also been confirmed in the literature, which states that “better tools and appraisal design can lead directly to better policy appraisal and hence better policies” (Adelle et al., Reference Adelle, Jordan and Turnpenny2012).
4.2. An ecosystem encompassing all stakeholder interests is lacking
Within the context of robot regulation, there is a large ecosystem involving public policymakers, standard organizations, robot developers/manufacturers, and end-users. Yet it is noticeable that a common platform for channeling the interaction between public policymakers, standard organizations, robot developers/manufacturers, and end-users is currently lacking (Fosch Villaronga and Drukarch, Reference Fosch Villaronga and Drukarch2021c). The lack of such a platform encompassing all stakeholder interests may entail many consequences. First, it prevents an active engagement with the affected stakeholders. For instance, ISO and standardization organizations alike welcome many stakeholders to participate in their standardization activities, but they do not proactively seek any stakeholder involvement, for example, they do not ask user groups to be involved. Over time, this creates power imbalances concerning the creation and production of norms geared toward framing robot development and user rights protection.
LIAISON highlighted how reshaping the mode of interactions yields helpful feedback from the community and thus facilitates the iterative regulatory process (Fosch Villaronga and Drukarch, Reference Fosch Villaronga and Drukarch2021c). It is thus essential to devise a mechanism for bringing together all stakeholders to align their efforts and interests into making current and future robots safe to use. To create an optimal regulatory framework for new technologies, the European Commission needs to better communicate with society, standard makers, and developers. A platform based on the model that LIAISON proposes could be beneficial in improving the communication between all stakeholders involved in the development and regulation (be it through public or private bodies) of new technologies.
4.3. Engaging with robot developers can generate policy-relevant knowledge
Engaging developers and end-users is instrumental in identifying unregulated and underestimated challenges (e.g., over-time integrative and adaptive systems’ safety, cyber-physical safety, psychological harm) that regulatory frameworks should cover (Fosch-Villaronga et al., Reference Fosch-Villaronga and Drukarch2020). These challenges arose in conversations with developers and are mainly connected with the concerns and the challenges they find while turning a prototype into a marketable device. In this subsection, we introduce the different findings with respect to personal care, rehabilitation, and agricultural robots (Fosch Villaronga and Drukarch, Reference Fosch and Drukarch2021b).
4.3.1. Findings concerning personal care robots
Personal care robot developers experience challenges and inconsistencies regarding ISO 13482:2014. While some developers perceive the standard as easy to follow and valuable as it is, others highlight that it could benefit from better guidance and that while useful, the standard could use different and more concrete examples and measures to be simpler and easier to follow. At the same time, the respondents indicate that the standard is clear concerning its language and layout. They nevertheless see room for improvement in the following sections: (a) safety requirements and protective measures, (b) safety-related control system requirements, and (c) verification and validation.
From the standard-making side, in the exploratory meeting with a representative of the ISO Technical Committee TC299 (Robotics) Working Group 02 on Service Robot Safety standardization, working on the revision of ISO 13482:2014, it became evident that the TC299 sees potential areas for improvement of the standard in its scope and structure. A concrete example is the need to provide further guidance for specific user types. The importance of considering the elderly, children, and pregnant women under ISO 13482:2014 has been pointed out during the LIAISON workshop at the ERF, where participants generally indicated to believe that such consideration is fundamental. In this regard, the standard stated that the Working Group would revise the definition of personal care robots, taking into account concrete users such as children, elderly persons, and pregnant women. However, the 2020 revised standard shows no changes in this respect (Fosch-Villaronga and Drukarch, Reference Fosch-Villaronga and Drukarch2021a).
More specifically, participants indicated that the standard should consider concrete aspects for these different users: cognitive capabilities, different learning ways, safety, different limit values, mental and physical vulnerability, different body dimensions, interaction requirements, mental culture, physical disabilities, interaction with the robot, situation comprehension, body-reaction time, and size physiology. In addition, participants indicated that there should not be a simplification of specific user groups and standard revision within this context. Moreover, within this debate, the importance of adequate training was also stressed, thereby highlighting the need to reconsider the provided training that considers multiple user types.
Concerning the specific definition of personal care robot, LIAISON’s methodology exposed problems associated with a lack of definition for personal care within the standard. Without a defined legal scope, engineers might comply with the wrong instruments (e.g., they might avoid medical legislation) and, therefore, be exposed to sanctions and further responsibilities. The LIAISON survey provided an example directed toward this, where a respondent indicated to be dealing with exoskeletons that fall under the physical assistant robots category but was uncertain as to whether she should follow the Medical Device Regulation (MDR).
Moreover, public responses during the ERF workshops indicate that those robot developers who have experience with ISO 13482:2014 run multiple standards for their devices. They also believe that their robot does not fit into the standard category, and they do not know if their robot is a medical device and have all been confronted with different classifications from public and private policy documents. As a result of incorrect categorization and unclear classification, roboticists might put in place inappropriate safeguards, and users might be put in risky or harmful situations (Fosch-Villaronga, Reference Fosch-Villaronga2019).
In this sense, one of the most notorious confusing categories identified by developers through LIAISON when it comes to healthcare is whether a robot is a medical device or not. While ISO 13482:2014 aimed to bring more clarification to the field, it created many new confusing categories. The focus on personal care robots created an in-between category between service robots and medical devices. This ended up in two standards/categories: those for medical use and well-being and that personal care. However, the MDR states that “devices with both a medical and a non-medical intended purpose shall fulfill the requirements applicable to devices cumulatively with an intended medical purpose and those applicable to devices without an intended medical purpose” on its Art 1.3. This article was meant to avoid treating different devices that presented a similar risk to the user. For instance, colored contact lenses were considered cosmetics while presenting the same risks that contact lenses to replace glasses presented to the human eye. In this regard, the critical question is, to what extent will ISO 13482:2014 provide any room for those robotic devices that flirt the boundary of medical and non-medical.
While ISO 13482:2014 considers the consequences of error in robot autonomous decisions, the wrong autonomous decisions section only states, “a personal care robot that is designed to make autonomous decisions and actions shall be designed to ensure that the wrong decisions and incorrect actions do not cause an unacceptable risk of harm.” However, the standard does not clarify the meaning of an “acceptable risk of harm” and a “non-acceptable risk,” nor does it define the criteria to decide on this. Silence on these matters, however, does not provide a safeguard baseline.
Finally, as a solution, the standard states that “the risk of harm occurring as an effect of incorrect decisions can be lowered either by increasing the reliability of the decision or by limiting the effect of a wrong decision.” This brings about the question whether a broader range of factors should be considered in the standard in this regard. For instance, it is not clear whether the provisions around safety as stipulated in the standard need to be combined with article 22—on automated decision-making, including profiling—of the General Data Protection Regulation (GDPR). While the GDPR seems to have been drafted with computer systems in mind, cyber-physical systems have been primarily disregarded in this regard (Felzmann et al., Reference Felzmann, Fosch-Villaronga, Lutz and Tamò-Larrieux2019).
4.3.2. Findings concerning rehabilitation robots
Before rehabilitation robots can be made commercially available in the EU, manufacturers need to demonstrate that the device is safe. However, the safety validation of rehabilitation robots is complex. Especially when it comes down to straightforward testing procedures that can be used during robot development, information in regulations and standards is rare or scattered across multiple standards. This is partly because rehabilitation robots are relatively new, which reduces the availability of best practices and applicable safety standards.
Moreover, manufacturers of rehabilitation robots should be aware that article 1.6 of the MDR, in essence, states that devices that can also be seen as machinery (such as a robot) should also meet essential health and safety requirements as set out in Annex I of the Machinery Directive. Similarly, there might be standards from other domains which are more specific than the general safety and performance requirements listed in the MDR and can therefore be relevant for rehabilitation robots (e.g., personal care safety standards). However, the user must consider any restrictions or differences between the domains and be aware that the respective standard is not directly applicable (Bessler et al., Reference Bessler, Prange-Lasonder, Schaake, Saenz, Bidard, Fassi, Valori, Lassen and Buurke2021). Moreover, in the EU, the legislation for medical devices applies to rehabilitation robots. When a device complies with relevant so-called harmonized standards, the developer can assume that the device complies with the EU legislation. However, for medical devices, the current applicable harmonized standards are harmonized for the MDD. This means that between May 2021 and May 2024, there probably will be no or just a limited number of harmonized standards that can officially be used to demonstrate conformity with the MDR (Bessler et al., Reference Bessler, Prange-Lasonder, Schaake, Saenz, Bidard, Fassi, Valori, Lassen and Buurke2021). Important to note within this context is that the familiarization with applicable regulations and standards and the process of safety validation take much time, which can be a burden, especially for small to medium enterprises and start-ups.
In addition to the documentation of the system and the risks involved, a validation of the risk mitigation strategies is also required. This validation is defined as a set of actions to evaluate with evidence that a bunch of safety functions meet a group of target conditions (Saenz et al., Reference Saenz, Behrens, Schulenburg, Petersen, Gibaru and Neto2020) and is essentially a measurement to prove that a specific system complies with designated operating conditions characterized by a chosen level of risk. There is currently no guidance from standards on how validation measurements should be executed (Bessler et al., Reference Bessler, Prange-Lasonder, Schaake, Saenz, Bidard, Fassi, Valori, Lassen and Buurke2021). Especially concerning the usefulness of protocolsFootnote 20, the majority of the participants in the LIAISON workshop at the ERF indicated that protocols offer a very clear and valuable tool in guiding them through the validation process.
4.3.3. Findings concerning agriculture robots
ISO 18497:2018 specifies principles for designing highly automated aspects of large autonomous machines and vehicles used for agricultural field operations but fails to include small autonomous agricultural robots into its scope. A regulatory framework for small autonomous agricultural robots is yet to be created. A valid question raised within this context relates to the definition the standard attributes to a highly automated agricultural machine—does this definition also encompass agricultural robotic devices? The insights provided by participants in the LIAISON workshop at the ERF led to interesting findings, with some participants believing that agricultural robots fall within the scope of this definition, and others thinking that they do not (see Figure 2).

Figure 2. Do you think that robots fit into the definition of agricultural machinery?
Engagement with DIH-AgROBOfood’s standards work package leader led to the finding that no safety standard addresses agricultural robots during a rapidly advancing field. As a result, it will become necessary to specifically take this type of robotic field into account in the standard revision or create a standard tailored explicitly to agricultural robots.
On a European level, the Machinery Directive 2006/42/CE is the reference text on the regulation of equipment and machinery, including for agriculture. To observe these requirements, European and international norms and standards (EN and ISO) are applied. However, the emergence of agricultural robots has led to new functionalities and new applications and therefore unknown risks, which must be understood to best comply with the Machinery Directive. Compatibility with the automation of agricultural functions is not always apparent. The Directive stipulates that a machine must not make unexpected movements near a person. This calls into question the automated process that enables a robot to take over on start-up. Other discrepancies between text and practice include operator responsibility, mainly where the operator acts remotely.Footnote 21 Such operators are not always present with the robot but nevertheless may remain legally responsible for the safety of operations and must, therefore, be able to place the machine in safe mode at all times.
A key lesson learned from interacting with the agricultural community is that with risk analysis and the performance of tests and adjustments in the design phase, the key is developing reliable, safe machines within a regulatory context that is ill-suited and inaccurate.
Therefore, within agriculture, harmonized standards from other sectors are applied analogously with agricultural robotics. On the remaining points, robot developers explain the risk analysis conducted and set out the solutions implemented in response, thereby demonstrating the resulting level of performance.
Finally, the involved DIH also stress this need for diverse stakeholder involvement. For instance, engagement with DIH AgROBOfood has presented the need for robot developers to pay attention to ethical, legal, and many other issues to determine if a robot will survive in a practical setting. Since agricultural robots barely interact with humans (at least not directly as in personal care or rehabilitation), the community is still not ready to engage with the ethical, legal, and societal (ELS) aspect community yet.
4.3.4. Cross-domain challenges
Safety standards are characterized by a 5-yearly revision, allowing for an evaluation of the adequacy of the relevant standard(s). Concerning the 5-yearly period for revision, the respondents to the LIAISON surveys and participants in the LIAISON workshop at the ERF presented clearly divided opinions on whether this time frame is too long. Out of a pool of 15 respondents, 40% indicated that this time frame is too long, while 60% disagreed with that opinion. These results were complemented with arguments from the workshop participants, stating that whether the 5-yearly revision is too long depends on the domain to which the standard relates—is the domain settled or still in the early stages of development? Moreover, it was argued that in some disciplines, there are still too few experts active in ISO, making it impossible to shorten the revision time frame. In addition, it takes time to gain sufficient experience in a particular domain to assess the adequacy of standards properly; revision should not be based on “single-case experiences.” Moreover, it was argued that standards are supposed to offer a reliable framework for safety. By updating standards more frequently, we might risk undermining the reliability and dependability of standards.
While each of the investigated standards is concerned with physical safety requirements, the legislative system includes many other fundamental rights to be protected, for example, (a) health, safety, consumer, and environmental regulations; (b) liability; (c) IP; (d) privacy and data protection; and (e) capacity to perform legal transactions (Leenes et al., Reference Leenes, Palmerini, Koops, Bertolini, Salvini and Lucivero2017). Concerning the adequacy of standards, the involved participants of the LIAISON workshop at the ERF believed that standards should shift from mono-impact to multi-impact, including factors related to ethics, environmental sustainability, liability, accountability, privacy, and data protection, and psychological aspects. As such, it is clear that robots, to be safe, need to comply with the safety requirements set by private standards and include other aspects highlighted within the law to ensure that the rights and protection of the user are not compromised.
The current cross-domain nature of robotics raises a dilemma for roboticists that many other users of the Machinery Directive and related harmonized standards do not encounter. This arises from the fact that the standards focusing on the safety of collaborative robotics are domain-specific, and it is not always clear to a roboticist which standards are applicable to their system. Currently, these standards covering different domains are not synchronized and can have conflicting requirements. This can lead to uncertainty, mainly when robots are used in new fields (such as agriculture) or for multiple domains (i.e., an exoskeleton used for medical purposes or to support workers in manufacturing) (Bessler et al., Reference Bessler, Prange-Lasonder, Schaake, Saenz, Bidard, Fassi, Valori, Lassen and Buurke2021).
A key cross-domain finding is that robots and AI technologies can impact humans beyond physical safety. Traditionally, the definition of safety has been interpreted to exclusively apply to risks that have a physical impact on persons’ safety, such as, among others, mechanical or chemical risks. However, the current understanding is that integrating AI in cyber-physical systems such as robots, thus increasing interconnectivity with several devices and cloud services and influencing the growing human–robot interaction, challenges how safety is currently conceptualized rather narrowly (Martinetti et al., Reference Martinetti, Chemweno, Nizamis and Fosch-Villaronga2021). Thus, to address safety comprehensively, AI demands a broader understanding of safety, extending beyond physical interaction, but covering aspects such as cybersecurity (Fosch-Villaronga and Mahler, Reference Fosch-Villaronga and Mahler2021) and mental health. Moreover, the expanding use of machine learning techniques will more frequently demand evolving safety mechanisms to safeguard the substantial modifications over time as robots embed more AI features. In this sense, the different dimensions of the concept of safety, including interaction (physical and social), psychosocial, cybersecurity, temporal, and societal, need to be considered for robot development (Martinetti et al., Reference Martinetti, Chemweno, Nizamis and Fosch-Villaronga2021). Revisiting these dimensions may help, on the one side, policy and standard makers redefine the concept of safety in light of robots and AI’s increasing capabilities, including human–robot interactions, cybersecurity, and machine learning, and, on the other hand, robot developers integrate more aspects into their designs to make these robots genuinely safe to use.
A final cross-domain challenge relates to the autonomy levels of robots. The levels of autonomy define the robot’s progressive ability to perform particular functions independently. In other words, “autonomy” refers to a robot’s “ability to execute specific tasks based on current state and sensing without human intervention.” For the automotive industry, the Society of Automotive Engineers (SAE, 2018) established automation levels to clarify the progressive development of automotive technology that would, at some point, remove the human from the driving equation. However, no universal standards have been defined for progressive autonomy levels for personal care, rehabilitation, or agricultural robots.
Yang et al. (Reference Yang, Cambias, Cleary, Daimler, Drake, Dupont and Taylor2017) proposed a generic six-layered model for medical robots’ autonomy levels depicting a spectrum ranging from no autonomy (level 0) to full autonomy (level 5) to bridge this gap in the medical field. The effort is a significant step toward bringing more clarity to the field, especially with respect to the roles and responsibilities resulting from increased robot autonomy. Still, the model needs more detailing on how it applies to specific types of medical robots. Robots’ embodiment and capabilities differ vastly across surgical, physically/socially assistive, or agricultural contexts, and the involved human–robot interaction is also very distinctive (Fosch-Villaronga et al., Reference Fosch-Villaronga, Khanna, Drukarch and Custers2021).
4.4. A bridging the gap through interdisciplinary cooperation
The results gained from the survey on the usefulness of the LIAISON model and the interactive sessions at the ERF are very revealing. While 28% of the 27 respondents believe that currently there is no link between robot development and policy/standard making, 66% believe that such a link does exist but that this link is either too complex and lacks openness, merely far too complex or only exists between robot development and policy/standard making (Fosch Villaronga and Drukarch, Reference Fosch Villaronga and Drukarch2021c). A small 7% of respondents believed that such a link already exists between robot development and policy/standard making.

Figure 3. Is a link between robot development and policy/standard making missing?
More specifically, in response to the question of whether a link between robot development and policymaking is currently missing, a range of responses was provided, namely (a) yes, currently there is no such a link between robot development and policy/standard making (28%); (b) no, there is already such a link between robot development and policy/standard making, but it is too complex (38%); (c) no, there is already such a link between robot development and policy/standard making, but it is too complex and lacks openness (21%); (d) no, but the link is only between developers and standard organizations (7%); and (e) no, there is already such a link between robot development and policy/standard making (7%) (Fosch Villaronga and Drukarch, Reference Fosch Villaronga and Drukarch2021c).
It is here where the LIAISON model aims to bridge the gap between robot governance and development by fostering interdisciplinary cooperation. The results gained from the survey on the usefulness of the LIAISON model and the interactive sessions at the ERF indicate that for the regulatory approach proposed through LIAISON to be valuable and effective, a diverse group of stakeholders should be involved (Fosch Villaronga and Drukarch, Reference Fosch Villaronga and Drukarch2021c). These stakeholders include robot developers, manufacturers, policymakers, standardization organizations, legal scholars, and ethicists. The involved DIH also stress this need for diverse stakeholder involvement. For instance, engagement with DIH AgROBOfood has presented the need for robot developers to pay attention to ethical, legal, and many other issues to determine if a robot will survive in a practical setting.
In addition, the exploratory meeting with standard makers clarified the value of the LIAISON model in liaising standardization activities and robot development. More specifically, during one of the policy and standard-making institutional meetings, a representative of ISO Technical Committee TC299 (Robotics) Working Group 02 on Service Robot Safety standardization stressed establishing a relationship of cooperation between ISO/TC299/WG2 and the LIAISON model could be very useful and valuable. On the one hand, ISO/TC299/WG2 could provide LIAISON with the necessary input from standard making. On the other hand, looking at its goal, putting into practice the LIAISON model could offer WG2 the relevant knowledge on inconsistencies and gaps in ISO 13482:2014 from the perspective of robot developers (Fosch Villaronga and Drukarch, Reference Fosch Villaronga and Drukarch2021d).
Moreover, the results obtained through the survey on the usefulness of the LIAISON model and the interactive sessions at the ERF also indicate that there is a serious need for cooperation between (a) central policymakers and standardization institutes; (b) major standardization institutes (ISO, BSI, CENELEC); and (c) user group initiatives and policy/standard makers.
4.5. Lack of legal comprehension
Robot manufacturers deal with many different legal frameworks, including standards and regulations. While compliance tools like the H2020 COVR Toolkit can help in this respect, the platform leaves room for desire. The results gained from the survey on the usefulness of the LIAISON model and the interactive sessions at the ERF indicate an overall lack in legal comprehension among robot developers, thereby adding emphasis to the first finding of a clear missing link between robot developers and policymakers. More specifically, the obtained data highlight this on three points: (a) experience with standards, (b) knowledge about the difference between public and private policymaking, and (c) experience applying standards. Out of the 33 respondents, 23% indicated to have never used a standard before, against 77% who suggested having experience with standards (Fosch Villaronga and Drukarch, Reference Fosch Villaronga and Drukarch2021c). At the same time, while all respondents—based on a pool of 15 respondents—indicated being aware of and understanding the difference between standards and the law, approximately 33% showed to be still confused regarding this difference.
Interestingly, with regard to respondents’ experience with standards, based on a pool of 26 respondents, we were presented with a variety of responses, namely: (a) run multiple standards for my devices (70%); (b) my robot does not fit into the standard category (45%); (c) do not know if my robot is a medical device (40%), and (d) do not know the difference between standard and regulation (10%).
It was indicated that smaller and younger companies often lack the necessary knowledge and understanding concerning the applicable legal frameworks and the difference between private and public policymaking within this context. Thus, especially for new robot manufacturers, a clarification of the existing legal landscape and the applicable legal framework would help in reducing the complexity in robot legal compliance. Moreover, various meetings with the DIH DIH-HERO and DIH AgROBOfood have indicated this confusion among their community and the lack of legal comprehension. For this reason, these DIH stressed the value that the LIAISON model could also offer and the valuable insights that their community could provide policymakers in this respect. This has led to the organization of domain-specific webinars at a later stage in the LIAISON project.
5. Conclusion
Based on the belief that the regulatory cycle is truly closed when it starts again upon the identification of new challenges, LIAISON puts the theoretical model of a dynamic, iterative regulatory process into practice, aiming to channel robot policy development from a bottom-up perspective toward a hybrid top-down/bottom-up model, leaving the door open for future modifications. As such, LIAISON aims to clarify what regulatory actions policymakers have to take to provide compliance guidance, explain unclear concepts or uncertain applicability domains to improve legal certainty, and inform future regulatory developments for robot technology use and development at the European, National, Regional, or Municipal level. Moreover, by explicitly shedding light on the standardization activities in the abovementioned domains, LIAISON has created awareness about the barriers to access for robot developers and other relevant stakeholders concerning such activities.
Overall, the LIAISON model has proven to be a valuable tool to facilitate effective robot governance, as indicated by relevant stakeholders, because of its all-encompassing nature. Possible avenues for expansion relate to active involvement in standardization organizations, focus on harmonization activities, and legal and educational participation in DIH to create more legal awareness among the involved communities of robot developers. A platform based on the mechanism proposed by LIAISON could be thus beneficial in improving the communication between all stakeholders involved in the development and regulation (be it through public or private bodies) of robot technologies.
The results presented in this paper highlight the importance of and need for active stakeholder involvement in robot governance. However, currently, the link between robot development and policymaking is complex, and it lacks openness, transparency, and free access. Access to standardization activities is not always accessible due to high costs, and there is a lack of clarity concerning public policymaking activities and their relation to private standard making. This requires a reconsideration of how (public) policy/standard makers engage with stakeholders in the normative process.
Moreover, the above results have indicated the need to seek active participation of affected parties (e.g., NGOs, user group initiatives, e.g., patients organizations and consumer networks, and other interested groups). These parties should not only be involved at the end of the development and policy/standard-making chain. They form an integral part of the process and should be engaged in these activities from an early stage to provide input and feedback that will consider the needs and concerns of the wider public.
In the long-term, the expected project results may complement the existing knowledge on the “ethical, legal, and societal (ELS)” considerations in relation to robotics by providing clarity on how to address pressing but still uncovered safety challenges raised by robots, and represent a practical, valuable tool to advance social goals in a robotized environment. With the necessary adaptations, LIAISON’s dynamic model also has the potential to be used in the regulation of different technologies, particularly those whose fast-paced development and unknown impact make them harder to regulate. Overall, taking interactions in policymaking seriously will provide a solid basis for designing safer technologies, safeguarding users’ rights, and improving the overall safety and quality delivered by such systems.
Acknowledgments
An earlier version of this paper was presented at the 2021 Data for Policy Conference. The authors wish to thank the participants for the useful feedback and discussion.
Funding Statement
This paper is part of the H2020 COVR Project that has received funding from the European Union’s Horizon 2020 research and innovation program (grant agreement No. 779966). The funder had no role in study design, data collection and analysis, decision to publish, or preparation of the manuscript.
Competing Interests
The authors declare no competing interests exist.
Author Contributions
Conceptualization: H.D. and E.F-V.; Writing original draft: H.D., C.C., and E.F-V. All authors approved the final submitted draft.
Data Availability Statement
Replication data and code can be found in https://www.universiteitleiden.nl/en/research/research-projects/law/liaison.
Comments
No Comments have been published for this article.