1. Introduction
The term cumulative technological culture (CTC) is used to describe how, over time, human populations have gradually accumulated techniques and tools that are too complex to be invented by a single individual (Boyd & Richerson Reference Boyd and Richerson1996; Boyd et al. Reference Boyd, Richerson and Henrich2011; Richerson & Boyd Reference Richerson and Boyd2005; Tomasello Reference Tomasello1999; Tomasello et al. Reference Tomasello, Kruger and Ratner1993), a phenomenon that could be restricted to humans (Boyd & Richerson Reference Boyd and Richerson1996; Galef Reference Galef1992; Tomasello Reference Tomasello1999; but see Boesch & Tomasello Reference Boesch and Tomasello1998; Hunt & Gray Reference Hunt and Gray2003; Whiten et al. Reference Whiten, Horner and Marshall-Pescini2003). CTC has been said to be driven by two engines (Legare & Nielsen Reference Legare and Nielsen2015): imitation (faithful copying of a trait) and innovation (improvement of a trait).Footnote 1 To date, the emphasis has been placed mainly on the imitative component (Boyd & Richerson Reference Boyd and Richerson1985; Dean et al. Reference Dean, Kendal, Schapiro, Thierry and Laland2012; Derex et al. Reference Derex, Godelle and Raymond2013b; Galef Reference Galef1992; Henrich & Gil-White Reference Henrich and Gil-White2001; Lewis & Laland Reference Lewis and Laland2012; Schillinger et al. Reference Schillinger, Mesoudi and Lycett2015; Tennie et al. Reference Tennie, Call and Tomasello2009; Reference Tennie, Call and Tomasello2012; Tomasello Reference Tomasello1999; Tomasello et al. Reference Tomasello, Kruger and Ratner1993; Reference Tomasello, Carpenter, Call, Behne and Moll2005). The rationale is that faithful social transmission can work as a ratchet to prevent slippage backward so that the newly invented technique can be subsequently improved (i.e., ratchet effect; Tomasello Reference Tomasello1999; Tomasello et al. Reference Tomasello, Kruger and Ratner1993). In line with this, the literature has suggested a series of cognitive mechanisms that are oriented toward the social dimension of CTC (e.g., teaching, imitation, theory of mind, and metacognition). The emphasis on the social dimension is obviously justified: Social learningFootnote 2 (whatever it may be) is a far more effective catalyst than asocial learning when it comes to passing on the content of technical information possessed by individuals in a group. However, this does not necessarily imply that CTC originates, first and foremost, in social cognitive skills. A viable alternative explanation is that CTC has emerged because of a non-social cognitive structure that allows humans to acquire and develop this content.
To illustrate this content–structure distinction, let us consider the following assumption: “[H]umans may be smarter than other creatures, but none of us is nearly smart enough to acquire all the information necessary to survive in any single habitat […] We owe our success to our uniquely developed ability to learn from others” (Boyd et al. Reference Boyd, Richerson and Henrich2011, p. 10918). This assumption emphasizes the social dimension of CTC and how the content can be acquired. Let us propose a revised version of this assumption: None of us is nearly smart enough to acquire all the information necessary to survive in any single habitat, but all of us are smart enough to acquire each piece of information – as well as to produce any kind of innovation – necessary to survive in any single habitat. This revised assumption allows us to escape from the social dimension and to focus instead on the non-social, technical dimension.
This assumption has two corollaries. The first is that all humans possess a non-social cognitive structure enabling them to acquire and generate a great – perhaps infinite – amount of technical information (i.e., the content). This is what we call the technical potential. This potential is fundamental for CTC because without it, we would not have observed an almost systematic, and sometimes very fast (e.g., digital technology revolution), increase in accumulated expertise over time. An individual may live in a very rich technical environment (i.e., one with rich content). However, if this individual does not possess the ability (i.e., the necessary cognitive structure) to acquire and improve this content, this rich environment is of no interest and it is unlikely that imitation and innovation will be observed. Our room for technical improvement revealed by CTC is necessarily based on our extensive individual cognitive ability to acquire and improve techniques. The second corollary is that this technical potential is influenced by several factors: non-social environmental opportunities (e.g., seeing lightning striking a tree), social environmental opportunities (e.g., seeing another individual making fire), and idiosyncratic characteristics (e.g., age, personality, and motivation). Thus, even if all humans possess the cognitive skills (i.e., the structure) allowing them to acquire and generate a vast amount of technical information (i.e., the content), the exploitation of this technical potential depends on orthogonal factors (i.e., each individual's specific historical trajectory resulting from non-social and social opportunities as well as idiosyncratic characteristics, see immediately above) and this leads to interindividual variation in terms of technical expertise.
This article aims to lay the foundations for a cognitive approach to CTC. Our working hypothesis is that CTC originates in uniquely human cognitive skills, namely technical-reasoning skills. Technical reasoning is the necessary cognitive structure that enables humans to constantly acquire and develop new techniques (also called mechanical knowledge). In section 2, we will present the theoretical framework of the technical-reasoning hypothesis based on recent advances in neuropsychology and neuroimaging. We will defend the thesis stating that technical-reasoning skills are the ideal underpinning for both imitation and innovation (the so-called dual engines of CTC). Such a thesis represents an epistemological break with the traditional approach, which assumes that imitation and innovation are based on distinct kinds of mechanisms (i.e., social vs. asocial/individual learning, respectively; Boyd et al. Reference Boyd, Richerson and Henrich2011; Dean et al. Reference Dean, Kendal, Schapiro, Thierry and Laland2012; Hernik & Csibra Reference Hernik and Csibra2009; Tennie et al. Reference Tennie, Call and Tomasello2009; Tomasello Reference Tomasello1999), as well as with approaches that reject the potential role of causal understanding (i.e., technical reasoning) in CTC (e.g., Boyd et al. Reference Boyd, Richerson and Henrich2011; Derex et al. Reference Derex, Bonnefon, Boyd and Mesoudi2019). In sections 3 and 4, we will then discuss the idea that technical reasoning is also necessary to represent one's own and other people's technical skills, leading us to revise the theory-of-mind and metacognition hypotheses of CTC. In these three sections, we will point to promising new avenues for interpreting classical issues related to CTC. To sum up, this article offers a unified cognitive approach to CTC in a field that has so far been largely dominated by other disciplines, such as evolutionary biology, mathematics, anthropology, archeology, economics, and philosophy (Heyes Reference Heyes2018).
2. Technical reasoning
2.1. Theoretical framework
Humans are not unique in using and making tools. Many other species also show tool behavior (Bentley-Condit & Smith Reference Bentley-Condit and Smith2010; Shumaker et al. Reference Shumaker, Walkup and Beck2011). For instance, wild chimpanzees can use and learn how to use stones as hammers to crack nuts in a fashion similar to humans (Boesch et al. Reference Boesch, Bombjaková, Boyette and Meier2017; Reference Boesch, Bombjakova, Meier and Mundry2019). Wild chimpanzees and capuchin monkeys can also use tools efficiently. They can select and transport different tools according to the properties of the task (e.g., heavier stone hammers to open fresh nuts vs. lighter stone hammers for dry nuts; Luncz et al. Reference Luncz, Falótico, Pascual-Garrido, Corat, Monsley and Haslam2016; see also Boesch & Boesch Reference Boesch and Boesch1984; Deblauwe et al. Reference Deblauwe, Guislain, Dupain and van Elsacker2006; Schrauf et al. Reference Schrauf, Huber and Visalberghi2008; Sirianni et al. Reference Sirianni, Mundry and Boesch2015; Reference Sirianni, Wittig, Gratton, Mundry, Schuler and Boesch2018; Spagnoletti et al. Reference Spagnoletti, Visalberghi, Ottoni, Izar and Fragaszy2011; Visalberghi et al. Reference Visalberghi, Addessi, Truppa, Spagnoletti, Ottoni, Izar and Fragaszy2009a; Reference Visalberghi, Spagnoletti, Ramos da Silva, Andrade, Ottoni, Izar and Fragaszy2009b; Reference Visalberghi, Sirianni, Fragaszy and Boesch2015). They can plan economical motor actions when performing tool behavior (Liu et al. Reference Liu, Simpson, Izar, Ottoni, Visalberghi and Fragaszy2009; for a review, see Visalberghi et al. Reference Visalberghi, Sirianni, Fragaszy and Boesch2015). In some cases, they can exhibit multifunctional tool use by using the same tool for different tasks (e.g., using sticks as probes to access small preys and also as combs for grooming; Falótico & Ottoni Reference Falótico and Ottoni2014; see also Mannu & Ottoni Reference Mannu and Ottoni2009). New Caledonian crows can also make different types of hook-shaped tools to help them capture their prey (Hunt Reference Hunt1996). In broad terms, a high level of cognitive sophistication can be reflected in nonhuman tool behavior.
However, human tool behavior differs from that known to occur in nonhumans in various respects (Osiurak Reference Osiurak2017). For instance, unlike most tool-using species, we can use not only simple tools that amplify motor actions of the upper limbs (e.g., a stick to extend reach and a rock to increase pounding force), but also complex tools that transform our motor actions into qualitatively different mechanical actions (e.g., a knife to cut and a pencil to write; Frey Reference Frey2007). We are also the only species that exploits “natural” forces (e.g., water, wind, and fire; Shumaker et al. Reference Shumaker, Walkup and Beck2011) and exhibits prospective diagnostic skills (Povinelli & Frey Reference Povinelli and Frey2016) and transfer skills (Martin-Ordas et al. Reference Martin-Ordas, Call and Colmenares2008).Footnote 3 These findings indicate that tool use and making – as well as construction behavior – might be based on distinct cognitive processes in nonhumans and humans. Even if nonhumans – including tool-users – may exhibit signs of causal understanding (Taylor et al. Reference Taylor, Hunt, Medina and Gray2009; van Horik & Emery Reference van Horik and Emery2016), they would not be able to understand unobservable causal properties. By contrast, humans can “reinterpret” the world in terms of unobservable, hypothetical entities such as causal forces (Penn & Povinelli Reference Penn and Povinelli2007; Penn et al. Reference Penn, Holyoak and Povinelli2008b). This non-social cognitive structure might allow us to acquire and develop a vast amount of technical information. This is what we call technical reasoning.
The technical-reasoning hypothesis is akin to previous proposals stressing the uniqueness of humans in terms of causal reasoningFootnote 4 as well as the key role of this kind of reasoning in CTC (e.g., Pinker Reference Pinker2010; Vaesen Reference Vaesen2012; Wolpert Reference Wolpert2003; see also Penn & Povinelli Reference Penn and Povinelli2007; Penn et al. Reference Penn, Holyoak and Povinelli2008b). It nevertheless differs from some of them in positing that technical reasoning is domain-specific and not domain-general (for a similar viewpoint, see Goldenberg Reference Goldenberg2013; Hegarty Reference Hegarty2004; McCloskey Reference McCloskey1983). The technical-reasoning hypothesis is at odds with some of the assumptions of the cultural niche hypothesis (e.g., Boyd et al. Reference Boyd, Richerson and Henrich2011). Although this latter hypothesis does not ignore the role of causal reasoning in CTC (notably in its innovative component), it posits that this kind of reasoning plays a minimal role, because humans commonly learn technical content from social interactions without understanding it. This hypothesis is based on what we call the omniscient view of technical reasoning (see sect. 2.3). Moreover, the corollary of this is that our technical-reasoning skills are a product of cultural transmission and not a species-specific difference-maker from which CTC originates. By contrast, the technical-reasoning hypothesis proposes a Bayesian approach to how technical content is acquired through experience, and suggests that technical reasoning is critical for the learning of technical content from social interactions as well as for improving this content (see sects. 2.2 and 2.4). In this view, technical reasoning is central to CTC.
Technical reasoning can be defined as the ability to reason about physical object properties (Osiurak Reference Osiurak2014; Osiurak & Badets Reference Osiurak and Badets2016; Reference Osiurak and Badets2017; Osiurak et al. Reference Osiurak, Jarry and Le Gall2010; Reference Osiurak2017). It involves the left inferior parietal lobe (and particularly the area PF; Reynaud et al. Reference Reynaud, Lesourd, Navarro and Osiurak2016) and possesses five key characteristics (see Fig. 1):
(1) It is both analogical (i.e., transfer of what is learnt from one situation to another; e.g., using a knife to cut a tomato→using a saw to cut a wooden board) and causal (i.e., predicting the effects on the environment; e.g., the tomato is cut in half). This is a specific form of reasoning directed toward the physical world, which must not be confounded with other forms of non-verbal analogical reasoning, particularly fluid cognition. Indeed, fluid cognition is useful for the development of logical thought, which does not need a deep understanding of physical constraints (e.g., Raven's progressive matrices test). In addition, dissociations can be found between technical reasoning and fluid cognition not only at a behavioral level (e.g., De Oliveira et al. Reference De Oliveira, Reynaud and Osiurak2019), but also at a neural level (left inferior parietal lobe/technical reasoning vs. prefrontal cortex/fluid cognition; Blair Reference Blair2006).
(2) It is based on mechanical knowledge, that is, knowledge about physical principles (intuitive theories or know-how for Pinker Reference Pinker2010; called content above; e.g., the cutting action requires the relative opposition between one thing possessing the properties Sharpness+, Hardness+, and Width− vs. another thing possessing the properties Sharpness−, Hardness−, and Width+; see Osiurak Reference Osiurak2014). This knowledge, which is acquired through experience, is non-declarative. Consequently, when we reason about a physical problem, it is difficult for many of us to make explicit what we understand about the physical principle at work (e.g., just think about how it is difficult to describe a cutting action in physical terms; for a similar viewpoint, see Gatewood Reference Gatewood and Dougherty1985; Wynn & Coolidge Reference Wynn and Coolidge2014). In this way, mechanical knowledge must not be confounded with certain types of semantic knowledge, such as functional knowledge (e.g., van Elk et al. Reference van Elk, van Schie and Bekkering2014) or folk theories (Keller & Keller Reference Keller and Keller1996). A significant body of neuropsychological evidence has confirmed this dissociation, indicating that the ability to use familiar or novel tools, on the one hand, and functional knowledge, on the other, can be impaired independently of one another (e.g., Bartolo et al. Reference Bartolo, Daumüller, Della Sala and Goldenberg2007; Goldenberg & Spatt Reference Goldenberg and Spatt2009; Negri et al. Reference Negri, Lunardelli, Reverberi, Gigli and Rumiati2007; Osiurak et al. Reference Osiurak, Aubin, Allain, Jarry, Richard and Le Gall2008; Reference Osiurak, Jarry, Allain, Aubin, Etcharry-Bouyx, Richard, Bernard and Le Gall2009; Silveri & Ciccarelli Reference Silveri and Ciccarelli2009; for a discussion, see Osiurak & Badets Reference Osiurak and Badets2016). Functional knowledge is also preferentially impaired after damage to the temporal lobes, as in patients with semantic dementia, who can nevertheless have relatively spared tool-use skills because of the integrity of the left inferior parietal lobe (Baumard et al. Reference Baumard, Lesourd, Jarry, Merck, Etcharry-Bouyx, Chauviré, Belliard, Moreaud, Croisile, Osiurak and Le Gall2016; Lesourd et al. Reference Lesourd, Baumard, Jarry, Etcharry-Bouyx, Belliard, Moreaud, Croisile, Chauviré, Granjon, Le Gall and Osiurak2016). In addition, mechanical knowledge is long-term knowledge that supports technical-reasoning skills and which, if present in sufficient quantity, allow humans to progressively develop technical expertise. In a way, any human is an apprentice or a potential expert-in-the-making, “with bits of knowing accumulating inexorably” (Wynn et al. Reference Wynn, Haidle, Lombard, Coolidge, Wynn and Coolidge2017, p. 47). This view is in line with models of expert cognition that stress the key role of long-term memory in expertise (Ericsson & Delaney Reference Ericsson, Delaney, Miyake and Shah1999; Ericsson & Kintsch Reference Ericsson and Kintsch1995; Gobet Reference Gobet2016; for a discussion about the link between expert and technical cognition, see Wynn & Coolidge Reference Wynn and Coolidge2014; Wynn et al. Reference Wynn, Haidle, Lombard, Coolidge, Wynn and Coolidge2017).
(3) The outcome of technical reasoning is a mental simulation of the mechanical action to be performed and this simulation needs to be temporarily maintained to guide the overtly performed action. Technical reasoning allows humans to solve physical problems in everyday life (e.g., to cut a tomato; see Fig. 1). It starts by using mechanical knowledge to find a physical principle that is thought to be (see sect. 2.3) appropriate for solving the targeted physical problem (e.g., cutting) as well as the associated physical object properties (i.e., sharp, and so on). This abstract principle is then transposed to the present situation, which is constrained by certain elements (i.e., the physical properties of the tomato). The individual therefore needs to select a tool that is sharp enough relative to the tomato. During this step, the reasoning ends with the generation of a mental simulation of the mechanical action to be performed (i.e., the motion of the knife on the tomato).
(4) Technical reasoning concerns mechanical actions (i.e., tool–object relationships) and non-motor actions (i.e., hand–tool relationships). Technical reasoning does not deal with the selection and on-line control of the most appropriate motor actions to realize the mentally generated mechanical action. This is the role of the motor-control system, which involves more superior structures of the parietal lobes (e.g., intraparietal sulci; see Osiurak & Badets Reference Osiurak and Badets2017; Reynaud et al. Reference Reynaud, Lesourd, Navarro and Osiurak2016; for a neurocognitive framework of how technical reasoning and the motor-control system can interact, see also Orban & Caruana Reference Orban and Caruana2014; Fig. 1). Importantly, the motor-control system is unaware of the goal of the action (i.e., tool use, tool making, or object transport). If someone has the idea of performing back-and-forth movements with the knife on the tomato, this is the expected effect, which will constrain the motor actions chosen within the motor-control system. Similarly, if someone intends to move an object from one location to another, the expected effect is the motion of the object, which will also constrain the motor actions chosen. In other words, the technical-reasoning hypothesis assumes that no specific tool-use motor program is needed to specify how to manipulate familiar tools (see also Goldenberg Reference Goldenberg2013). In addition, if the goal is not achieved or if the action appears to be ineffective, the process returns to technical reasoning to adapt the mental simulation of the mechanical action to the present situation (e.g., change of the point of incision or orientation of the blade) or to select another tool, for instance (Fig. 1).
(5) Technical reasoning – and the dynamics of the process described above (see also Fig. 1) – is not specific to the use of familiar tools (e.g., a knife), but also concerns any situation in which a physical problem has to be solved, such as when making tools or during construction behavior, or in any situation requiring the understanding of the mechanics of the physical world (e.g., use of novel tools and innovation; Osiurak & Heinke Reference Osiurak and Heinke2018).
The technical-reasoning hypothesis is an alternative to the long-standing tool-use motor-program hypothesis initially developed on the basis of neuropsychological findings (Buxbaum Reference Buxbaum2001; Heilman et al. Reference Heilman, Rothi and Valenstein1982; Rothi et al. Reference Rothi, Ochipa and Heilman1991; van Elk et al. Reference van Elk, van Schie and Bekkering2014). This hypothesis focuses on the key role of motor actions (i.e., hand–tool relationships) in tool use and assumes that the use of familiar tools is based on specific tool-use motor programs, which are also referred to as manipulation or procedural knowledge (e.g., “knowing that a hammer is grasped at the handle and used with a back-and-forth swinging movement”; van Elk et al. Reference van Elk, van Schie and Bekkering2014, p. 237). According to this hypothesis, these motor programs are concerned only with familiar tool use, but not with novel tool use, tool making, or construction. In addition, these motor programs are thought to be stored within the left inferior parietal lobe. Three lines of evidence seem to favor the technical-reasoning hypothesis over the tool-use motor-program hypothesis.
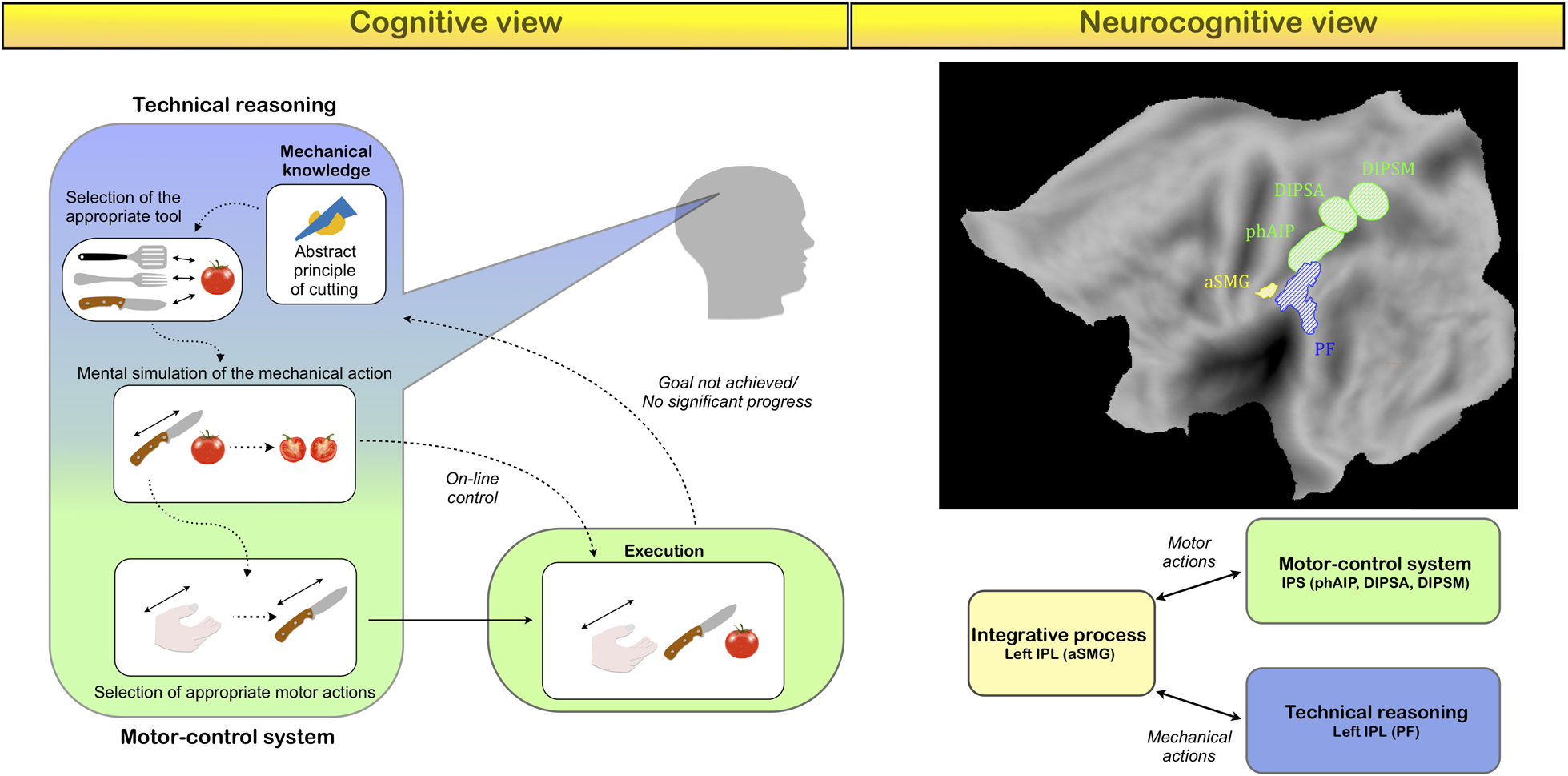
Figure 1. The technical-reasoning hypothesis. The left panel (cognitive view) illustrates the dynamics of technical reasoning (in blue) and its interaction with the motor-control system (in green). This illustration is based on an instance of use of a familiar tool (i.e., a knife). However, technical reasoning is not specific to familiar tool use because it also concerns any situations in which it is necessary to solve a physical problem, such as when making tools or during construction behavior, or in any instances requiring the understanding of the mechanics of the physical world (e.g., use of novel tools and innovation). More details about this dynamic are given in the main text. The right panel (neurocognitive view) corresponds to the neurocognitive model of the technical-reasoning hypothesis. As shown, technical reasoning might mainly involve the cytoarchitectonic area PF within the left inferior parietal lobe (IPL). The motor-control system might instead be supported by more superior parietal structures such as the intraparietal sulcus (IPS; phAIP, putative human anterior IPS; DIPSA, anterior dorsal intraparietal sulcus; DIPSM, medial dorsal intraparietal sulcus). The anterior portion of the left supramarginal gyrus (aSMG) could play a key role by biasing signals to phAIP to favor the selection of the motor actions that best suit the realization of the mechanical action generated by technical reasoning (see Orban & Caruana Reference Orban and Caruana2014).
First, there is a strong link between familiar and novel tool use (e.g., unusual use of familiar tools and mechanical problem-solving including tool making) in left brain-damaged patients, who can exhibit severe difficulties when using familiar tools (Bartolo et al. Reference Bartolo, Daumüller, Della Sala and Goldenberg2007; Goldenberg & Hagmann Reference Goldenberg and Hagmann1998; Goldenberg et al. Reference Goldenberg, Hartmann-Schmid, Sürer, Daumüller and Hermsdörfer2007; Hartmann et al. Reference Hartmann, Goldenberg, Daumüller and Hermsdörfer2005; Heilman et al. Reference Heilman, Maher, Greenwald and Rothi1997; Jarry et al. Reference Jarry, Osiurak, Delafuys, Chauviré, Etcharry-Bouyx and Le Gall2013; Osiurak et al. Reference Osiurak, Jarry, Allain, Aubin, Etcharry-Bouyx, Richard, Bernard and Le Gall2009; see Baumard et al. Reference Baumard, Osiurak, Lesourd and Le Gall2014; for additional evidence in neurodegenerative diseases and normal aging, see also Baumard et al. Reference Baumard, Lesourd, Jarry, Merck, Etcharry-Bouyx, Chauviré, Belliard, Moreaud, Croisile, Osiurak and Le Gall2016; Lesourd et al. Reference Lesourd, Baumard, Jarry, Le Gall and Osiurak2017; Fig. 2A). Second, voxel-based lesion-symptom mapping studies have demonstrated that the same brain structure (the area PF within the left inferior parietal lobe) is systematically damaged in left brain-damaged patients with deficits in both familiar and novel tool use (Goldenberg & Spatt Reference Goldenberg and Spatt2009; Martin et al. Reference Martin, Beume, Kümmerer, Schmidt, Bormann, Dressing, Ludwig, Umarova, Mader, Rijntjes, Kaller and Weiller2016; Salazar-Lopez et al. Reference Salazar-Lopez, Schwaiger and Hermsdörfer2016; Fig. 2B). Taken together, these findings confirm that one and the same neurocognitive process (i.e., technical reasoning, possibly supported by the left area PF) is at work whatever the familiarity of the tool-use activity, thereby ruling out the tool-use motor-program hypothesis, which assumes that only familiar tool use is supported by the left inferior parietal lobe because of the presence of preexisting tool-use motor programs. Third, we conducted a neuroimaging meta-analysis of tool use (Reynaud et al. Reference Reynaud, Lesourd, Navarro and Osiurak2016), including studies in which participants had to focus on the appropriateness of mechanical actions (i.e., tool–object relationships). The results revealed activation of the left area PF (Fig. 2C), confirming that this brain area is involved in the understanding of mechanical actions (i.e., tool–object relationships) but not of motor actions (i.e., hand–tool relationships) as is suggested by the tool-use motor-program hypothesis.

Figure 2. Evidence for the technical-reasoning hypothesis. (A) The figure depicts the strong link between familiar tool use and novel tool use in left brain-damaged patients, confirming that one and the same cognitive process (i.e., technical reasoning) is at work whatever the familiarity of the task. Each point refers to a study in which both left brain-damaged patients and healthy controls were assessed on both tasks. Patients' deficit is expressed in terms of percentage of impairment as compared to healthy controls (MControls–MPatients). (B) Lesion sites reported in voxel-based lesion-symptom mapping studies investigating familiar tool use and novel tool use in left brain-damaged patients. The area PF within the left inferior parietal lobe is the only brain area identified in all the studies. (C) Key finding of a recent neuroimaging meta-analysis on tool use (Reynaud et al. Reference Reynaud, Lesourd, Navarro and Osiurak2016). The analysis included studies in which healthy participants had to focus on the appropriateness of the mechanical action (tool–object relationship). Results revealed activation of the left area PF (in red in the zoomed picture), suggesting that this area is deeply involved in understanding mechanical actions (i.e., technical reasoning). (D) Key finding from a recent neuroimaging meta-analysis on tool-use observation (Reynaud et al. Reference Reynaud, Navarro, Lesourd and Osiurak2019). The results relate to the contrast between studies in which healthy participants had to observe tool-use actions minus non-tool-use actions. Again, a preferential activation of the left area PF is found (in yellow in the zoomed picture), indicating that people reason technically not only to conceive mechanical actions with tools themselves (aforementioned results) but also when watching others use tools.
More relevant to our purpose is the fact that we recently conducted another neuroimaging meta-analysis on action observation, in which we distinguished between studies focusing on either the observation of non-tool-use actions (e.g., grasping a ball) or the observation of tool-use actions (e.g., using a knife; Reynaud et al. Reference Reynaud, Navarro, Lesourd and Osiurak2019; Fig. 2D). The main difference between these two categories of studies is that the former include only the observation of a motor action (tool–hand relationship), whereas the latter include the observation of both mechanical (tool–object relationship) and motor actions (hand–tool relationship). Our findings indicated that the left area PF is specifically involved in the observation of tool-use actions and, as a result, of mechanical actions performed by others. In broad terms, the same neurocognitive process (i.e., technical-reasoning skills/left area PF) might be at work not only when humans carry out mechanical actions, but also when they watch others doing so.
2.2. Technical reasoning and cumulative technological culture
The focus on the social dimension of CTC has led scientists to consider that distinct cognitive processes are at work during social (e.g., imitation) versus asocial (e.g., trial and error) learning (e.g., Tomasello Reference Tomasello1999; Whiten et al. Reference Whiten, McGuigan, Marshall-Pescini and Hopper2009). We acknowledge that specific cognitive processes can be involved in some instances of social learning because of the social dimension of the situation (see below). However, there is no reason to posit that different cognitive skills are involved in understanding the physical causalities of a situation depending on whether the situation is social or not (for a similar view, see Heyes Reference Heyes2012; Reference Heyes2016). Support for this view comes from the aforementioned neuropsychological and neuroimaging findings that have indicated that the same brain area – the left area PF – is involved not only when humans use tools, solve mechanical problems, sometimes by making novel tools, or focus on mechanical actions, but also when they observe others using tools. Further support comes from a recent series of experimental studies with micro-society paradigms in which participants had to build as high as possible a tower with metal wires (De Oliveira et al. Reference De Oliveira, Reynaud and Osiurak2019; Osiurak et al. Reference Osiurak, De Oliveira, Navarro and Reynaud2020a) or a paper airplane that would fly as far as possible (Osiurak et al. Reference Osiurak, De Oliveira, Navarro, Lesourd, Claidière and Reynaud2016). In these studies, the participants performed the task as members of a chain of 10 participants, each of whom completed the task one after the other. In the observation conditions, the participants could observe other members of the group building an airplane before performing the task themselves. No communication was permitted. In the communication conditions, they could help the subsequent participant by communicating verbally with her or him. The participants' technical-reasoning and theory-of-mind skills were also assessed in additional testing sessions. The results indicated that learners' technical-reasoning skills are the best predictor of cumulative performance over generations irrespective of the kind of social learning studied (i.e., observation and communication). The technical-reasoning hypothesis of CTC represents an epistemological break with the state-of-the-art in various respects.
2.2.1. A dormant, technical potential
The concept of technical potential is close to that of “zone of latent solutions” (Tennie et al. Reference Tennie, Call and Tomasello2009; Reference Tennie, Braun, Premo, McPherron, Haidle, Conard and Bolus2016a; see also Bandini & Tennie Reference Bandini and Tennie2017). “The term ‘latent solution’ refers to a behavior that lies ‘dormant’ or ‘latent’ in an individual until triggered by a particular set of social or environmental cues and sufficient motivation on the part of the learner” (Tennie et al. Reference Tennie, Braun, Premo, McPherron, Haidle, Conard and Bolus2016a, p. 125). In this respect, the zone of latent solutions corresponds to the room for improvement that a given species possesses in light of its cognitive skills. For instance, Tennie et al. (Reference Tennie, Call and Tomasello2009) showed that chimpanzees cannot learn a “loop technique” through social learning, simply because they cannot acquire it on their own (i.e., this technique is beyond their zone of latent solutions). Whiten et al. (Reference Whiten, McGuigan, Marshall-Pescini and Hopper2009) criticized this concept, suggesting that chimpanzees' and humans' very different CTC capacities can be understood only if the task proposed falls within the scope of a species' cognitive skills. We agree with Whiten et al. (Reference Whiten, McGuigan, Marshall-Pescini and Hopper2009) that the study of social learning and culture in any species needs to be based on tasks that are within the scope of the cognitive skills of the species. Many elegant studies have thus shown that chimpanzees, for instance, can develop cultural traditions (Horner et al. Reference Horner, Whiten, Flynn and de Waal2006; Whiten et al. Reference Whiten, Goodall, McGrew, Nishida, Reynolds, Sugiyama, Tutin, Wrangham and Boesch1999; Reference Whiten, Horner and de Waal2005; Reference Whiten, Spiteri, Horner, Bonnie, Lambeth, Schapiro and de Waal2007). However, if we acknowledge that CTC is absent or, at best, minimal in nonhumans, it is reasonable to wonder whether these difficulties might not be because of the fact that the cognitive skills they do possess are too limited to enable them to acquire technical information. In this respect, we posit that CTC emerges in humans because we possess the “dormant” technical potential (i.e., technical-reasoning skills) to acquire and improve on a vast amount of technical information. This provides the room required for technical improvement, which is a key characteristic of CTC.
2.2.2. Imitation and innovation: Two sides of the same coin
The distinction between imitation and innovation is commonly considered to reflect the involvement of distinct forms of learning and, as a result, of cognitive processes (social learning vs. asocial/individual learning including trial and error, respectively; e.g., Tomasello Reference Tomasello1999). Here, we posit that technical-reasoning skills allow humans to extract relevant technical information from a “social demonstration” (imitation), to reject irrelevant information, as well as to generate new ideas to improve the end-product (innovation). In this context, the ability to extract/reject information in an effective way is subject to interindividual differences so that people with good technical-reasoning skills are better at doing this, as evidenced by studies using micro-society paradigms (De Oliveira et al. Reference De Oliveira, Reynaud and Osiurak2019; Osiurak et al. Reference Osiurak, De Oliveira, Navarro, Lesourd, Claidière and Reynaud2016; Reference Osiurak, De Oliveira, Navarro and Reynaud2020a; Reference Osiurak, Cretel, Duhau-Marmon, Fournier, Marignier, De Oliveira, Navarro and Reynaudin press).
2.2.3. An integrative process for social and asocial learning
In the micro-society paradigms employed to investigate CTC in humans, the periods of social transmission and production are generally very short (e.g., 5-min period of social learning and 5-min period of production) and there is no alternation between periods of social and individual learning (e.g., Caldwell & Millen Reference Caldwell and Millen2008; Reference Caldwell and Millen2009; Morgan et al. Reference Morgan, Uomini, Rendell, Chouinard-Thuly, Street, Lewis, Cross, Evans, Kearney, de la Torre, Whiten and Laland2015; Osiurak et al. Reference Osiurak, De Oliveira, Navarro, Lesourd, Claidière and Reynaud2016; Zwirner & Thornton Reference Zwirner and Thornton2015). Indeed, it is very remarkable that cumulative performance can be found in such conditions, because they do not reflect what happens in everyday life, during which people generally alternate between periods of social and individual learning (i.e., exploration and practice; Whiten Reference Whiten2015; Reference Whiten2019b; see also Ingold Reference Ingold1998). After all, we do not assess students just 5 min after a teaching course. The risk is that we may draw conclusions that might be different if more time were given to individuals to digest what they have acquired from social learning. For instance, Morgan et al. (Reference Morgan, Uomini, Rendell, Chouinard-Thuly, Street, Lewis, Cross, Evans, Kearney, de la Torre, Whiten and Laland2015) investigated the efficacy of transmission of stone tool-making skills along the chains of participants in five different social-learning conditions (e.g., reverse engineering, observation, communication, and so on). They found that less information was lost in the communication condition than, for instance, in the reverse-engineering condition. Based on these findings, one might consider that stone tool-making skills can be transmitted only by communication, but not by observation or reverse engineering. For our part, we are not saying that communication is a less effective mode of social transmission than observation or reverse engineering (see below), merely that it remains possible that stone tool-making skills can also be transmitted via observation or reverse engineering if learners can alternate between periods of social and individual learning. Evidence for this comes from the longitudinal study by Putt et al. (Reference Putt, Woods and Franciscus2014), who found no clear difference between a communication condition and an observation condition in the making of symmetric lithic bifaces. Interestingly, these authors noticed that the individuals in the observation condition were able to learn from their mistakes and improve their flake production each week, suggesting a kind of strategy based on reasoned trial and error. Other studies have demonstrated that expertise in stone knapping requires a considerable amount of time (more than 150 h of practice) to progressively acquire a clear technical understanding of the task (Bril et al. Reference Bril, Rein, Nonaka, Wenban-Smith and Dietrich2010; see also Stout et al. Reference Stout, Passingham, Frith, Ape and Chaminade2011; Reference Stout, Rogers, Jaeggi and Semaw2019). In this context, technical-reasoning skills can be viewed as an integrative process that makes it possible to extract information from social learning and to test and improve it via individual learningFootnote 5 using trial-and-error strategies that are not random but reasoned (Vaesen Reference Vaesen2012; see also Derex et al. Reference Derex, Bonnefon, Boyd and Mesoudi2019; Whiten Reference Whiten2019b).Footnote 6 In addition, these reasoned trial-and-error strategies can allow humans (1) to eventually reproduce – and indirectly imitate – a technique that has not been transmitted faithfully during social interaction or (2) to converge toward the same technical invention (i.e., without direct transmission; a kind of virtual imitation) simply because the same reasoning inevitably leads to the emergence of the same technical solution (for a similar view, see the cultural attraction hypothesis; Boyer Reference Boyer2001; Claidière & Sperber Reference Claidière and Sperber2007; Claidière et al. Reference Claidière, Scott-Phillips and Sperber2014a; Morin Reference Morin2015; Reference Morin2016; Sperber Reference Sperber1996; Sperber & Hirschfeld Reference Sperber and Hirschfeld2004).
2.2.4. The basis for innovation
Innovation can be defined as the generation of a novel learned behavior that is not the consequence of social learning or environmental induction (Ramsey et al. Reference Ramsey, Bastian and van Schaik2007). Innovation has been documented in a range of species including nonhuman primates (Reader & Laland Reference Reader and Laland2002). Given that CTC is absent in these species, it has been suggested that innovation alone is not sufficient for CTC (e.g., Dean et al. Reference Dean, Vale, Laland, Flynn and Kendal2014). This view, again, stresses the key role of social, but not of non-social, cognitive skills in CTC (e.g., see Dean et al. Reference Dean, Kendal, Schapiro, Thierry and Laland2012; Herrmann et al. Reference Herrmann, Call, Hernandez-Lloreda, Hare and Tomasello2007; Tomasello Reference Tomasello1999; for a similar view, see also Hernik & Csibra Reference Hernik and Csibra2009). This interpretation, which minimizes the role of innovation in CTC, can nevertheless be questioned if we consider that there are major differences in terms of innovation between humans and nonhumans (see Gruber Reference Gruber2016; Gruber et al. Reference Gruber, Muller, Reynolds, Wrangham and Zuberbühler2011; for evidence indicating difficulties in tool innovation in nonhuman primates). One of these differences concerns the uniquely human ability to combine techniques, as in the case of secondary tool use (i.e., the use of one tool to create another; Shumaker et al. Reference Shumaker, Walkup and Beck2011). Interestingly, modeling work has shown that innovation in humans might primarily result from technical combinations rather than from novel inventions or modifications (Lewis & Laland Reference Lewis and Laland2012). Thus, even if innovation is not unique to humans, humans might be unique in exhibiting combinatory innovation. At a cognitive level, this ability clearly reflects analogical reasoning skills (Vaesen Reference Vaesen2012). For instance, after learning a percussion technique to crack nuts (i.e., acquisition of the mechanical knowledge “percussion”), an individual can transfer it to the context of stone fracture to increase the production of flakes. One of the characteristics of technical reasoning is that it is analogical. Therefore, technical reasoning is an appropriate candidate to account for technical innovation in humansFootnote 7 (for a similar view, see Haidle Reference Haidle2014; Penn et al. Reference Penn, Holyoak and Povinelli2008b; Vaesen Reference Vaesen2012; Wolpert Reference Wolpert2003). Importantly, the analogical component of technical reasoning does not allow humans to systematically generate appropriate new technical solutions. Sometimes – or even often – the transfer of a technique to a new context can lead to failures, which can nevertheless be viewed as inventions at the individual level (for a distinction between technical invention/individual level and innovation/group level, see Wynn & Coolidge Reference Wynn and Coolidge2014). Nevertheless, we assume that this reasoned trial-and-error strategy might be an important engine for innovation, whatever the outcome (innovation or “ineffective inventions”/failures).
2.2.5. Technical reasoning is involved in all forms of social learning
As shown in Table 1, we have identified three main forms of social learning: reverse engineering (indirect), observation (direct), and communication (direct). Any theory that assumes that CTC originates in uniquely human social cognitive skills (e.g., Tomasello et al. Reference Tomasello, Kruger and Ratner1993) must also account for the fact that signs of CTC are also observed in reverse-engineering conditions in which models and learners cannot interact directly. Despite this, studies using micro-society paradigms have reported cumulative performance in such conditions (Caldwell & Millen Reference Caldwell and Millen2009; Derex et al. Reference Derex, Bonnefon, Boyd and Mesoudi2019; Zwirner & Thornton Reference Zwirner and Thornton2015; see also Caldwell et al. Reference Caldwell, Schillinger, Evans and Hopper2012; Reference Caldwell, Renner and Atkinson2018), although the degree of accumulation is generally lower than in observation or communication conditions (e.g., Caldwell & Millen Reference Caldwell and Millen2009). However, it should be remembered that it is already an achievement to observe cumulative performance in such experimental conditions in which there is very limited time and no alternation between periods of social and individual learning. Therefore, if signs of CTC in reverse-engineering conditions are observed in humans, then this suggests that human non-social cognitive skills are sufficient for the emergence of CTC (Vale et al. Reference Vale, Flynn and Kendal2012). Interestingly, in the neuropsychological literature, the idea that people can infer how to use an artifact from its physical structure has already been linked to concepts similar to technical reasoning (e.g., Goldenberg Reference Goldenberg2013; Goldenberg & Hagmann Reference Goldenberg and Hagmann1998; Hodges et al. Reference Hodges, Spatt and Patterson1999; Reference Hodges, Bozeat, Lambon Ralph, Patterson and Spatt2000; Humphreys Reference Humphreys2001). Thus, in line with this proposal, we assume that technical-reasoning skills can be useful in reverse-engineering conditions because they make it possible to infer the making process (see also Vaesen Reference Vaesen2012; Zwirner & Thornton Reference Zwirner and Thornton2015). In this respect, the technical-reasoning hypothesis offers a parsimonious framework for the cognitive study of CTC, suggesting that this reasoning is necessary for the emergence of CTC irrespective of the social-learning conditions (see also Vaesen Reference Vaesen2012). It should be noted, however, that this assumption is consistent with the idea that social cognitive skills could also be involved in direct social-learning conditions, thus making it possible to boost CTC (see below).
Table 1. Behavioral classification of forms of social learning

2.3. Mechanical knowledge is “a kind of magic”
One false belief about technical-reasoning skills is that these skills should lead to a full understanding of any physical situation, permitting the extraction of everything that is relevant from a social interaction but no more than that (Boyd et al. Reference Boyd, Richerson and Henrich2011; Reference Boyd, Richerson, Henrich, Richerson and Christiansen2013; Derex et al. Reference Derex, Bonnefon, Boyd and Mesoudi2019). The following quote provides a good illustration of this: “You see that your uncle's bow shoots farther than yours and notice that it is thicker, but less tapered, and uses a different plait for attaching the sinew. You copy all three traits, even though in reality it was just the plaiting that made the difference” (Boyd et al. Reference Boyd, Richerson and Henrich2011, p. 10922). According to Boyd et al. (Reference Boyd, Richerson and Henrich2011; see also Derex et al. Reference Derex, Bonnefon, Boyd and Mesoudi2019), the fact that humans can also copy irrelevant traits (as illustrated by this quote) is at odds with the idea that technical-reasoning skills are fundamental to CTC – because otherwise humans would copy only the relevant trait (e.g., the plaiting). This omniscient view of technical reasoning is erroneous. It is not because we possess technical-reasoning skills that we are able to spontaneously understand all the dimensions of a physical phenomenon. The reason is that technical reasoning is based on mechanical knowledge, which, similar to any form of knowledge, is a belief but not necessarily true.
This aspect has been elegantly demonstrated by Baillargeon and colleagues, who have shown how the principle of support evolves in early childhood (6 months: Infants consider any amount of contact between the object and the support sufficient for the object to be supported; 6–9 months: Infants expect an object to remain stable if a significant portion of its surface is in contact with the support; 9 months: Infants understand that other features such as the mass distribution of an object is critical to determine whether a support is appropriate or not, and so on; Baillargeon & Hanko-Summers Reference Baillargeon and Hanko-Summers1990; Baillargeon et al. Reference Baillargeon, Needham and DeVos1992; Needham & Baillargeon Reference Needham and Baillargeon1993). These findings indicate that mechanical knowledge can be acquired relatively early in childhood and can continue to grow over time (Remigereau et al. Reference Remigereau, Roy, Costini, Osiurak, Jarry and Le Gall2016), even during adulthood. In this respect, mechanical knowledge is never completely accurate and can be the basis for some “magical” beliefs such as thinking that any amount of contact between an object and a support is sufficient for the object to be supported. Nevertheless, even such magical beliefs can be useful for inferring potential outcomes in the environment (i.e., causal and analogical reasoning, the two dimensions of technical reasoning). However, over time, these inferences can be invalidated through a kind of Bayesian learning, leading the individual to progressively acquire more accurate knowledge about physical phenomena (i.e., technical expertise).
Recently, Derex et al. (Reference Derex, Bonnefon, Boyd and Mesoudi2019) reported a micro-society paradigm in which participants had to improve a physical system (i.e., a wheel that traveled down a 1-m-long inclined track). They found cumulative performance in that the wheel traveled farther in late than in early generations. However, there was no increase over generations in terms of “causal understanding,” which was assessed with an additional decision task in which the participants had to choose the best wheel configurations. For the authors, these findings demonstrate that CTCFootnote 8 can emerge without causal understanding (i.e., technical reasoning). This conclusion is subject to considerable reservations. The authors' main argument was that CTC occurred even though the participants did not develop a full understanding of the physical system, with most of them producing simplistic models related to a salient dimension (i.e., inertia and center of mass) rather than multidimensional causal theories. This is clearly at odds with an omniscient view of technical reasoning. However, it is consistent with the aforementioned idea that mechanical knowledge is the basis for some magical beliefs and, as a result, is not completely accurate. Thus, based on this inaccurate knowledge, the participants were able to produce effective – but not the most effective – solutions and this explains the presence of CTC over generations. It is also consistent with the fact that Derex et al. (Reference Derex, Bonnefon, Boyd and Mesoudi2019) themselves reported signs of causal understanding, stressing that their “participants did not randomly explore the parameter space” (p. 448; i.e., reasoned trial and error, see above; see also Kendal Reference Kendal2019). In broad terms, these findings do not provide clear evidence against the link between CTC and technical-reasoning skills.
2.4. Emulation versus imitation
As shown in Table 1, we suggest a revised classification of the different forms of social learning that diverges from previous proposals (Heyes Reference Heyes1994; Hoppitt & Laland Reference Hoppitt and Laland2013; Whiten & Ham Reference Whiten and Ham1992; Whiten et al. Reference Whiten, Horner and Marshall-Pescini2003; Reference Whiten, McGuigan, Marshall-Pescini and Hopper2009). This new classification is organized around two dimensions, namely the information provided by the model to the learner (i.e., model-oriented dimension) and the amount of information extracted by the learner from the model (i.e., learner-oriented dimension). In the experimental context, these two dimensions can be viewed as the independent variable and the dependent variable, respectively: We experimentally manipulate the amount of information provided by the model to investigate the amount of information extracted by the learner. In this classification, emulation (copying of “results”) and imitation (copying of “actions” and “results”) are distinguished on the basis of the correspondence between the model's behavior and the learner's behavior. In addition, there is no a priori knowledge concerning the model-oriented dimension in that a learner may “imitate” a model even in a reverse-engineering condition if she or he reproduces absolutely all the same actions that are performed by the model. In light of this reservation, a condition should not be considered a priori as imitative or emulative because this presupposes that participants are already ready to copy the different actions (e.g., Morgan et al. Reference Morgan, Uomini, Rendell, Chouinard-Thuly, Street, Lewis, Cross, Evans, Kearney, de la Torre, Whiten and Laland2015; Schillinger et al. Reference Schillinger, Mesoudi and Lycett2015; Zwirner & Thornton Reference Zwirner and Thornton2015). Terms such as “reverse engineering/end-product” or “observation/action” conditions allow us to overcome this classification issue (e.g., Caldwell & Millen Reference Caldwell and Millen2009; Wasielewski Reference Wasielewski2014).
The direct link between imitation and CTC has been repeatedly stressed in the field (Boyd & Richerson Reference Boyd and Richerson1996; Derex et al. Reference Derex, Godelle and Raymond2013b; Galef Reference Galef1992; Henrich & Gil-White Reference Henrich and Gil-White2001; Tennie et al. Reference Tennie, Call and Tomasello2009; Reference Tennie, Call and Tomasello2012; Tomasello Reference Tomasello1999; Tomasello et al. Reference Tomasello, Kruger and Ratner1993; Reference Tomasello, Carpenter, Call, Behne and Moll2005). The critical question, however, is what is imitated? To tackle this question, we propose to distinguish between what we have called mechanical actions (i.e., tool–object relationships) and motor actions (i.e., hand–tool or, more generally, body–tool relationships; also called bodily actions).
The first possibility is to focus on motor actions. This would lead us to consider that humans alone copy the motor actions of the model (e.g., Henrich & Gil-White Reference Henrich and Gil-White2001; Tennie et al. Reference Tennie, Call and Tomasello2009; Tomasello et al. Reference Tomasello, Davis-Dasilva, Camak and Bard1987; see also Köhler Reference Köhler1959; Lotem et al. Reference Lotem, Halpern, Edelman and Kolodny2017). This possibility has two main limitations. First, it implies experimental paradigms in which only one mechanical action is proposed and can be solved using different motor actions, preferentially performed by different body parts (e.g., head vs. hand; see Gergely et al. Reference Gergely, Bekkering and Kiràly2002). However, even with such a simple methodology, scoring issues can arise. For instance, what if a model uses the right hand and the learner the left hand (see Wohlschläger et al. Reference Wohlschläger, Gattis and Bekkering2003)? Is this an instance of imitation or emulation? This methodological issue necessarily grows in importance as the number of mechanical actions increases, thereby limiting the study of the link between imitation and CTC. Second, in line with the ideomotor principle (Brass & Heyes Reference Brass and Heyes2005; Wohlschläger et al. Reference Wohlschläger, Gattis and Bekkering2003; see also Osiurak & Badets Reference Osiurak and Badets2014; Reference Osiurak and Badets2016), the learner can attempt to reproduce the same mechanical action as the model, and then indeed perform the same motor action, simply because this motor action is the most economical for both of them at a biomechanical level (see Claidière et al. Reference Claidière, Smith, Kirby and Fagot2014b for a convergent cultural evolution in baboons based on biomechanical constraints). This is a kind of “sunflower effect”: All the sunflowers follow the sun throughout the course of the day, leading them to perform the same rotation; and yet, sunflowers do not imitate each other. In broad terms, this first possibility, which focuses on motor actions, is hard to demonstrate experimentally and is also partly ruled out by evidence indicating that children reproduce behaviors better when information about the mechanical rather than the motor action is provided (Flynn & Whiten Reference Flynn and Whiten2013). In other words, mechanical actions and non-motor actions might be the vital basis for any social transmission.
This leads us to a second possibility: the focus has to be on mechanical actions (Whiten et al. Reference Whiten, McGuigan, Marshall-Pescini and Hopper2009). Therefore, a learner can be considered to be imitating a model if she or he reproduces the same mechanical action as the model. A good way to explore this possibility is to use a “two-action” paradigm consisting of offering the choice between two mechanical actions that can be used to achieve the same goal (e.g., Horner & Whiten Reference Horner and Whiten2005; Horner et al. Reference Horner, Whiten, Flynn and de Waal2006; Whiten et al. Reference Whiten, Horner and de Waal2005). A significant body of evidence has been gathered using this paradigm and clearly demonstrates that nonhumans can not only exhibit imitation by reproducing the same mechanical action as that performed by a model, but also establish this as a tradition within their group (Bonnie et al. Reference Bonnie, Horner, Whiten and de Waal2007; Whiten et al. Reference Whiten, Horner and de Waal2005; for review, see Whiten & van de Waal Reference Whiten and van de Waal2017). In other words, these results invalidate the idea that (1) only humans can imitate and (2) CTC is necessarily based on imitation. More interestingly, it has also been shown that both humans and nonhumans can exhibit flexibility by alternating between imitation and emulation (e.g., Horner & Whiten Reference Horner and Whiten2005). More generally, these findings question the idea that humans are imitators and nonhumans are – at best – emulators (Whiten et al. Reference Whiten, McGuigan, Marshall-Pescini and Hopper2009; see also Caldwell et al. Reference Caldwell, Schillinger, Evans and Hopper2012; Dunstone & Caldwell Reference Dunstone and Caldwell2018).
A third possibility needs to be considered. In nonhumans, imitation generally concerns one mechanical action (e.g., using either a slide or a lift method to open a door, Horner et al. Reference Horner, Whiten, Flynn and de Waal2006; making a composite tool from two components, Price et al. Reference Price, Lambeth, Schapiro and Whiten2009) or a sequence of independent mechanical actions (hereafter called sequential mechanical actions; e.g., twisting a bolt, spinning a pin, and turning a handle; Whiten Reference Whiten1998), but not a combination of interdependent mechanical actions (hereafter called combined mechanical actions) that make it possible to achieve a technical goal (e.g., making a simple spear by producing a flake tool to cut down a tree and carve the spear, Haidle Reference Haidle2010). By contrast, in humans, micro-society paradigms have shown that cumulative performance can emerge over generations even when participants have to build complex artifacts by means of combined mechanical actions (e.g., Caldwell & Millen Reference Caldwell and Millen2009; Osiurak et al. Reference Osiurak, De Oliveira, Navarro, Lesourd, Claidière and Reynaud2016; Zwirner & Thornton Reference Zwirner and Thornton2015). Thus, the main difference between humans and nonhumans may lie in the ability to learn such combined mechanical actions from a model (for a similar view, see Whiten et al. Reference Whiten, Horner and Marshall-Pescini2003).
Some cognitive archeologists have suggested that the complexification of human technology over time might be explained by a specific enhancement of working memory (Wynn & Coolidge Reference Wynn, Coolidge, Mellars, Boyle, Bar-Yosef and Stringer2007; see also Haidle Reference Haidle2010; Reference Haidle2014). In line with this idea, it can be hypothesized that humans might be able to learn combined mechanical actions from models because of their enhanced working-memory skills. This hypothesis does not explain the qualitative difference between sequential and combined mechanical actions. Being able to maintain multiple mechanical actions in memory for a period of time is cognitively different from being able to combine them into a purposeful chain of interdependent mechanical actions. Furthermore, working memory is not a cognitive mechanism that is used to generate content, but instead temporarily stores content that is processed by other cognitive mechanisms (see Osiurak Reference Osiurak2017). Therefore, another hypothesis is that nonhumans might have difficulties understanding the physical principles underlying mechanical actions because of their lack of technical-reasoning skills. This would mean that they can, at best, imitate sequential mechanical actions but not combined mechanical actions. By contrast, the human ability to understand mechanical actions could enable us to store these more easily in working memory and thus permit us to reproduce the combined mechanical actions viewed in a model. The corollary is that the degree of technical expertise (i.e., the amount of mechanical knowledge possessed by an individual) modulates the level of copying, as shown in other domains such as chess where masters can quickly recall a very high number of “logical” chess configurations (e.g., Chase & Simon Reference Chase and Simon1973). If we adopt this viewpoint then the degree of technical expertise is not a bias for CTC that needs to be controlled (e.g., Derex et al. Reference Derex, Bonnefon, Boyd and Mesoudi2019; Reindle & Tennie Reference Reindle and Tennie2018; Tennie et al. Reference Tennie, Call and Tomasello2012), but rather a key aspect to be studied.
In addition, the fact that technical-reasoning skills can modulate the amount of information extracted in the case of combined mechanical actions can also explain why imitation and emulation are also flexible in humans, sometimes leading to innovation (for a similar view concerning technical expertise based on long-term working memory models, see Wynn & Coolidge Reference Wynn and Coolidge2014). To illustrate this, imagine Einstein explaining to you his theory of relativity. If you possess absolutely no knowledge about physics and mathematics, you may be able to reproduce at best 1% of his theory, even though you make a considerable effort to maintain a lot of information in working memory. If you are a physics graduate student, you may reproduce 20%. However, if you are the new Einstein, you may reproduce 80%, the remaining 20% corresponding to the improvement you make to the theory (i.e., innovation). However, the fact remains that if someone asks you to explain the entirety of the theory, you will be able to do so.
In sum, this third possibility, which focuses on combined mechanical actions, offers a revised view of the distinction between emulation and imitation, thereby departing from the idea that copying in humans is both process- and product-oriented because we alone are able to infer the mental states of others and represent others' actions as intentional (e.g., Tennie et al. Reference Tennie, Call and Tomasello2009; Reference Tennie, Call and Tomasello2012; Tomasello et al. Reference Tomasello, Carpenter, Call, Behne and Moll2005; for a similar view, see Csibra & Gergely Reference Csibra, Gergely, Munakata and Johnson2006; Reference Csibra and Gergely2009). Indeed, it is one thing to have the intention to imitate but another to be able to do so. After all, even if you intend to reproduce what Einstein is explaining, you may still copy no more that 1% if you are a novice in physics. Therefore, beyond the question of whether humans alone can imitate or whether imitation is the basis for CTC, a more critical question is whether humans are able to copy a great number of mechanical actions without possessing the technical ability to implement them. Our answer is that they probably do not.
2.5. Interim conclusion
Non-social cognitive skills are commonly excluded from the discussion about the origins of CTC. The technical-reasoning hypothesis offers a viable alternative to this view by assuming that CTC could not emerge without a specific technical potential based on non-social cognitive skills that allow us to reason about our physical world. These skills are involved in (1) both asocial/individual and social learning, (2) imitation and innovation, (3) combinations of mechanical actions, and (4) can develop over time, leading to interindividual differences in terms of technical expertise. In this perspective, social learning is not the cause, but a catalyst of CTC, enabling humans to acquire technical information more quickly than through asocial learning. In the next two sections, we will discuss the potential role of two – apparently – distinct cognitive skills (i.e., theory-of-mind and metacognitive skills) that can also contribute to CTC by facilitating social learning but without being necessary for its emergence.
3. Theory of mind
3.1. Theoretical framework
Theory of mind refers to the ability to attribute mental states to oneself and others (Bruner Reference Bruner1983; Harris Reference Harris and Whiten1991; Mead Reference Mead1934; Piaget Reference Piaget1932; Premack Reference Premack, Byrne and Whiten1988; Tomasello et al. Reference Tomasello, Kruger and Ratner1993; Whiten Reference Whiten1991).Footnote 9 The fact that this ability also concerns one's own mental states has led some authors to question the cognitive independence between theory-of-mind and metacognitive skills (e.g., Carruthers Reference Carruthers2009; Dunstone & Caldwell Reference Dunstone and Caldwell2018; Flavell Reference Flavell2000; Shea et al. Reference Shea, Boldt, Bang, Yeung, Heyes and Frith2014; Tomasello et al. Reference Tomasello, Kruger and Ratner1993). We will address this relationship in more detail below. It has been suggested that CTC may originate in uniquely human theory-of-mind skills (i.e., the theory-of-mind hypothesis of CTC; Boyd & Richerson Reference Boyd and Richerson1996; Herrmann et al. Reference Herrmann, Call, Hernandez-Lloreda, Hare and Tomasello2007; Tomasello Reference Tomasello1999; Tomasello & Call Reference Tomasello and Call1997; Tomasello et al. Reference Tomasello, Kruger and Ratner1993; Reference Tomasello, Carpenter, Call, Behne and Moll2005).Footnote 10 There are four main hypothesized manifestations of this involvement. The first is imitation. According to the theory-of-mind hypothesis, humans imitate others because their theory-of-mind skills might help them to “conceive” that others behave intentionally and, as a result, that they can be a source of information (see also Csibra & Gergely Reference Csibra and Gergely2009). Support for this idea comes initially from studies indicating that humans imitate whereas nonhumans emulate (e.g., Nagell et al. Reference Nagell, Olguin and Tomasello1993; Tomasello et al. Reference Tomasello, Davis-Dasilva, Camak and Bard1987). However, as stressed above, the humans/imitation versus nonhumans/emulation distinction is controversial and we will therefore not discuss it further (but see below for the question of overimitation in children). The other three manifestations are: cooperation, teaching, and opacity. In the next sections, we address these manifestations in turn.
3.2. Cooperation
Resource-sharing-based division of labor characterizes all human cultures (Gibson Reference Gibson, Gibson and Ingold1993; Ingold Reference Ingold, Gibson and Ingold1993; Reynolds Reference Reynolds, Gibson and Ingold1993; Whiten & Erdal Reference Whiten and Erdal2012). This may concern, for instance, foraging (hunting–gathering) or childcare (Whiten & Erdal Reference Whiten and Erdal2012). This cooperative dimension may be unique to humans, with nonhumans generally being more engaged in competition during their social interactions (Moll & Tomasello Reference Moll and Tomasello2007; Tennie et al. Reference Tennie, Jensen and Call2016b). The theory-of-mind hypothesis of CTC offers a theoretical framework for a narrower definition of cooperation as “shared or joint cooperative activities” during which participants (1) share a joint goal, (2) take on reciprocal and complementary roles to reach this joint goal, and (3) are motivated to help one another accomplish their roles if needed (Moll & Tomasello Reference Moll and Tomasello2007). A corollary is that working together allows individuals to pool their knowledge, thereby offering (4) the opportunity for separate solutions to be combined (Tomasello Reference Tomasello1999; Tomasello & Call Reference Tomasello and Call1997; see also Dean et al. Reference Dean, Vale, Laland, Flynn and Kendal2014). Indeed, theory-of-mind skills might be a key factor favoring the development of cooperation in humans. This can explain how two individuals can share a joint goal and be motivated to help one another (points 1 and 3 above, respectively). However, the question is whether theory-of-mind skills are sufficient to support the emergence of complementary roles (point 2 above) as well as the combination of solutions (point 4 above).
Let us begin with the question of complementary roles. Cooperation can be defined in different ways depending on the role played by each participant (for a classification, see Boesch & Boesch Reference Boesch and Boesch1989). In this respect, a joint cooperative activity is an instance of collaboration (Boesch & Boesch Reference Boesch and Boesch1989), also called heterotechnic cooperation (Reynolds Reference Reynolds, Gibson and Ingold1993), in that the different participants do not perform similar actions in time and space (i.e., coordination) but different and complementary actions. More rudimentary forms of cooperation have been documented in nonhumans (see Boesch Reference Boesch2002). However, although heterotechnic cooperation may be characteristic of all human cultures, evidence in favor of such forms of cooperation is rare in nonhumans (Boesch Reference Boesch2002; Boesch & Boesch Reference Boesch and Boesch1989; Boesch & Boesch-Achermann Reference Boesch and Boesch-Achermann2000) and subject to debate (see Moll & Tomasello Reference Moll and Tomasello2007). Heterotechnic cooperation can clearly contribute to CTC by allowing humans to engage in and develop effective technical activities they could not perform alone. Imagine, for instance, a group of seven individuals intending to lift a heavy piece of wood to strengthen the top of a cabin. To do so, they use a pulley technique. The piece of wood is tied with a rope and the rope passes over a strong branch situated above the cabin. Five individuals are needed to pull the rope to lift the piece of wood. Two additional individuals are also needed (1) to direct the piece of wood toward the top of the cabin using a pole and (2) to take hold of it and attach it to the roof. The theory-of-mind hypothesis can explain the motivation that these individuals have to act cooperatively, but not how the idea of dividing this activity into different mechanical actions has arisen.
Our assumption is that this type of cooperative activity is based on a combination of mechanical actions (e.g., tying and pulley) intended to solve a physical problem. It is necessarily the ability to conceive these different mechanical actions (through technical reasoning) that can transform any cooperative activity into a heterotechnic cooperative activity. Additionally, the cooperation between humans is anecdotal here. A workhorse or a tractor could have pulled the rope so that even a single individual could have solved this problem alone and our example of heterotechnic cooperation could simply be motivated by necessity because of the available technical resources (i.e., no workhorse or no tractor). This also leads us to address another aspect, which is that humans can also be viewed by their conspecifics as technical resources (e.g., workforce) in the same way as nonhumans. The history of mankind has clearly demonstrated that humans can, unfortunately, exploit nonhuman animals and other humans (e.g., slavery and colonization) and sometimes use them as technical resources to build exceptional constructions (e.g., Egyptian pyramids).
The same rationale can be applied to combinations of solutions. Although engaging in cooperative activities can lead to the sharing of technical solutions and, potentially, new combinations of solutions, theory-of-mind skills do not adequately account for the integration of these solutions within a coherent representation of mechanical actions (see above for a discussion of combination in the context of imitation and innovation). In sum, although theory-of-mind skills could favor cooperation, technical-reasoning skills remain necessary to transform this cooperation into heterotechnic cooperation or to use it to produce new combinations of solutions.
3.3. Teaching
Teaching can be broadly defined as behavior that facilitates learning in others (Kline Reference Kline2015; Thornton & Raihani Reference Thornton and Raihani2008). In recent years, evidence has accumulated for teaching behavior in nonhumans (e.g., Franks & Richardson Reference Franks and Richardson2006; Thornton & McAuliffe Reference Thornton and McAuliffe2006). Although these instances correspond to more rudimentary forms of teaching (e.g., scaffolding; Csibra Reference Csibra2007), they do not satisfy the strict definition of “direct active teaching,” during which an individual engages in teaching as a consequence of her or his own belief (which may or may not be substantiated) about the likely effect on the learner (Caldwell et al. Reference Caldwell, Renner and Atkinson2018; Caro & Hauser Reference Caro and Hauser1992; Csibra & Gergely Reference Csibra, Gergely, Munakata and Johnson2006; Kline Reference Kline2015). To date, no clear examples of active direct teaching have been identified in nonhumans (Csibra Reference Csibra2007; Galef Reference Galef1998; Kline Reference Kline2015; Tennie et al. Reference Tennie, Call and Tomasello2009; but see Boesch Reference Boesch1991). For this reason, the term teaching will be used in the following to refer to direct active teaching. Similar to imitation, it has been suggested that teaching is a precursor of CTC (Tennie et al. Reference Tennie, Braun, Premo, McPherron, Haidle, Conard and Bolus2016a; Tomasello Reference Tomasello1999; Tomasello et al. Reference Tomasello, Kruger and Ratner1993; see also Dean et al. Reference Dean, Kendal, Schapiro, Thierry and Laland2012; Reference Dean, Vale, Laland, Flynn and Kendal2014). Indeed, it can be hypothesized that theory-of-mind skills might be critical for forming a representation of what others understand about a given task. Based on this, an individual can become a teacher by orienting the attention of another individual (i.e., the learner) toward relevant information and providing appropriate feedback during the task (Csibra & Gergely Reference Csibra, Gergely, Munakata and Johnson2006; Dunstone & Caldwell Reference Dunstone and Caldwell2018). Strictly speaking, teaching can occur in both communication and observation conditions (Table 1).
However, studies that have included observation conditions have not generally reported data indicating that the model is clearly engaged in teaching (e.g., by slowing down the demonstration). By contrast, the use of verbal information in communication conditions makes it easier to see that the model is engaged in teaching based on her or his hypothesized theory-of-mind skills. The idea of a direct link between CTC and teaching is supported by a significant body of evidence acquired using micro-society paradigms, which has shown that cumulative performance is better (or that less information is lost over generations; Morgan et al. Reference Morgan, Uomini, Rendell, Chouinard-Thuly, Street, Lewis, Cross, Evans, Kearney, de la Torre, Whiten and Laland2015) in communication conditions than in observation or reverse-engineering conditions (Caldwell & Millen Reference Caldwell and Millen2009; De Oliveira et al. Reference De Oliveira, Reynaud and Osiurak2019; Osiurak et al. Reference Osiurak, De Oliveira, Navarro, Lesourd, Claidière and Reynaud2016; Zwirner & Thornton Reference Zwirner and Thornton2015; see also Caldwell et al. Reference Caldwell, Renner and Atkinson2018). Despite these findings, the teaching/theory-of-mind hypothesis remains unsatisfactory as an explanation of CTC for several reasons.
First, even if communication conditions favor CTC in micro-society paradigms, CTC can also be found in observation conditions and, more problematically, in reverse-engineering conditions, in which models and learners do not interact at all. Although the complexity of the technical information that could be transmitted in such conditions may be limited, the fact remains that less complex forms of CTC could emerge without theory-of-mind skills. Consequently, given that technical-reasoning skills may be involved in all forms of social learning within a technical context, CTC could instead originate from these skills whereas nevertheless still being boosted by the different contributions of theory-of-mind skills (i.e., cooperation and teaching; for a similar view, see Caldwell et al. Reference Caldwell, Renner and Atkinson2018). Second, the impact of teaching on CTC might also be overestimated in that the ethnological record of hunter–gatherer cultures reports that teaching plays a minimal role compared to observational learning (Hewlett et al. Reference Hewlett, Fouts, Boyette and Hewlett2011; MacDonald Reference MacDonald2007). Third, the idea that teaching is critical to CTC also implies that teachers are reliable sources of information for learners. However, this depends on several factors, such as the teacher's theory-of-mind skills, which are necessarily subject to interindividual differences in the same way as any other cognitive skills. In other words, even if a teacher is motivated to orient the learner's attention toward relevant information, this orientation can be untimely and slow down the learning process by preventing the learner from focusing on other aspects of the task that are actually more relevant to her or him (Putt et al. Reference Putt, Woods and Franciscus2014; for a similar conclusion in a written communication condition, see Derex et al. Reference Derex, Bonnefon, Boyd and Mesoudi2019). Fourth, recent evidence from micro-society paradigms indicates that learners' technical-reasoning skills are a better predictor of cumulative performance than teachers' theory-of-mind skills not only in observation but also in communication conditions (De Oliveira et al. Reference De Oliveira, Reynaud and Osiurak2019; Osiurak et al. Reference Osiurak, De Oliveira, Navarro, Lesourd, Claidière and Reynaud2016; Reference Osiurak, De Oliveira, Navarro and Reynaud2020a). Even if teaching can facilitate the transmission of technical information, what a learner can acquire remains first and foremost determined by her or his technical-reasoning skills.
Although teaching certainly remains a very effective way of transmitting information, it might nevertheless not be the necessary condition for CTC. Interestingly, teaching could play a key role in certain specific situations in which the learner lacks information. This can be the case when someone attempts to reproduce a tool whose making process is opaque (see below). More generally, teachers' theory-of-mind skills can be particularly relevant – and even necessary – when the learner and the teacher cannot directly share information about a specific technical task (i.e., nothing-is-present situations; see Osiurak et al. Reference Osiurak, De Oliveira, Navarro and Reynaud2020a). For instance, imagine a teacher explaining to a learner how to build a shelter in the absence of any raw material. In this situation, the teacher has to guide the learner through the building process using only her or his words. Theory-of-mind skills might play a critical role here in allowing the teacher to form an accurate representation of what the learner already knows as well as in permitting the use of terms that are comprehensible to the learner.
We recently tested this hypothesis in a micro-society paradigm that included a communication condition in which learners and teachers could communicate without sharing visual information (i.e., they were sitting on two chairs positioned back to back; Osiurak et al. Reference Osiurak, De Oliveira, Navarro and Reynaud2020a). We confirmed the hypothesis by observing that teachers' theory-of-mind skills were the best predictor of cumulative performance, along with learners' technical-reasoning skills. These findings indicate that teaching could help boost CTC by freeing humans from the need to be in concrete situations (i.e., presence of materials and demonstration) to acquire and transmit new technical information. It should be noted that this supportive role is far from unimportant given the adaptive value of being able to transmit information in the absence of concrete situations. However, this boosting role might be systematically conditioned by learners' technical-reasoning skills.
3.4. Opacity
The terms opacity/transparency refer to the amount of information that an individual can extract from scrutinizing an artifact. An artifact is transparent if it provides adequate information about how it is built (e.g., a simple spear), and opaque if not (e.g., a spear with a split-based bone point).Footnote 11 Given the lack of information directly provided by opaque artifacts, it has been repeatedly argued that more complex forms of social learning (e.g., imitation and teaching) might be required to transmit this (Boyd & Richerson Reference Boyd and Richerson1985; Caldwell & Millen Reference Caldwell and Millen2009; Caldwell et al. Reference Caldwell, Renner and Atkinson2018; Csibra & Gergely Reference Csibra, Gergely, Munakata and Johnson2006; Reference Csibra and Gergely2011; Derex et al. Reference Derex, Godelle and Raymond2013b; Schillinger et al. Reference Schillinger, Mesoudi and Lycett2015; Tennie et al. Reference Tennie, Call and Tomasello2012; Wasielewski Reference Wasielewski2014). Support for this view comes from studies indicating that the building of transparent artifacts can be transmitted through many forms of social learning, including reverse engineering, whereas the building of opaque artifacts can be transmitted in communication and observation conditions, but to a lesser extent in reverse-engineering conditions (e.g., Caldwell et al. Reference Caldwell, Renner and Atkinson2018; Derex et al. Reference Derex, Godelle and Raymond2013b; Morgan et al. Reference Morgan, Uomini, Rendell, Chouinard-Thuly, Street, Lewis, Cross, Evans, Kearney, de la Torre, Whiten and Laland2015; Wasielewski Reference Wasielewski2014). The theory-of-mind hypothesis of CTC provides an appropriate framework for interpreting these findings in that teachers' theory-of-mind skills might play a critical role when the learner lacks information, for example in nothing-is-present situations or when an artifact is opaque. Nevertheless, this hypothesis might place exaggerated emphasis on what the teacher can provide (based on theory-of-mind skills) compared to what the learner can understand (based on technical-reasoning skills). More specifically, the successful transmission of an opaque artifact may depend first and foremost on the learner's technical expertise, meaning that a technical expert might be able to reproduce an opaque artifact in all social-learning conditions (i.e., reverse engineering, observation, and communication), whereas an individual with little technical expertise might encounter difficulties whatever the condition. Let us discuss this aspect in more detail.
The notions of opacity/transparency commonly refer to an essential property of an artifact. An artifact is either opaque or transparent, and this is true for any individual. In line with James (1890/Reference James2007), we propose an epistemological shift by considering that “there is no property that is absolutely essential to any one thing” (see also Osiurak et al. Reference Osiurak, Jarry and Le Gall2010). The corollary is that the opacity of an artifact is phenomenologically based on the learner's technical expertise. For instance, it has been suggested that CTC was observed in a reverse-engineering condition in which the task required the participants to make a paper airplane, that is, a transparent artifact (e.g., Derex et al. Reference Derex, Godelle and Raymond2013b). Our experience with this task has taught us that many participants can find it difficult to reproduce the folding sequence by observation or to extract it by scrutinizing airplanes built by their predecessors. In other words, the assumption that the paper-airplane building task is fundamentally a transparent task for any individual is questionable. Given the link we found between cumulative performance and learners' technical-reasoning skills in such a task (Osiurak et al. Reference Osiurak, De Oliveira, Navarro, Lesourd, Claidière and Reynaud2016), this leads us to consider that the level of technical expertise can make the artifact transparent for some participants and opaque for others. It would therefore be interesting to propose a similar task to origami experts. Because of their expertise in folding techniques, all such artifacts should be transparent and they should achieve cumulative performance whatever the social-learning condition.
In broad terms, the major issue an individual faces when attempting to reproduce an artifact is to extract information from it. We posit that this extraction can be mediated by two factors. The first is the level of technical expertise, which can make an artifact opaque for some individuals, but transparent for others. The second is the information provided by the model. In cases where learners possess little technical expertise that is relevant to the task, teachers' theory-of-mind skills may help to compensate for this lack. This learner-centered perspective diverges from the position according to which the level of expertise is viewed as a confound in the study of CTC (Derex et al. Reference Derex, Bonnefon, Boyd and Mesoudi2019; Reindle & Tennie Reference Reindle and Tennie2018; Tennie et al. Reference Tennie, Call and Tomasello2012). Taken to its logical conclusion, this position implies that CTC should be studied only with inexperienced participants who have no previous knowledge about the task. This position is difficult to sustain because it would exclude the ethnological record on hunter–gatherer cultures in which individuals are never inexperienced. In addition, it suggests that the best way to explore CTC would be to study only children (Reindle & Tennie Reference Reindle and Tennie2018), presupposing that they have no prior knowledge about the task – which is always difficult to demonstrate.
The motivation for this methodological choice is also debatable because it is more than likely that the innovative component of CTC does not develop in children but in adults, who are necessarily not inexperienced. In line with this idea, Reindle and Tennie (Reference Reindle and Tennie2018) failed to obtain cumulative performance in a micro-society paradigm with children. Nevertheless, they observed the presence of cultural lineages in the different transmission chains, a finding very similar to those obtained in nonhumans. This study is instructive in showing that it is precisely by controlling for the so-called confound (i.e., technical reasoning) that CTC, and its innovative component in particular, can be suppressed. The technical-reasoning hypothesis offers a clear interpretation for these findings. Children do not possess a level of technical expertise that is sufficient to allow them to detect irrelevant information in a model and this makes it difficult for them to generate more effective solutions. Even if their level of technical-reasoning skills can allow them to reproduce a solution (even in reverse-engineering conditions; Reindle et al. Reference Reindle, Apperly, Beck and Tennie2017), their innovative skills remain limited (Beck et al. Reference Beck, Apperly, Chappell, Guthrie and Cutting2011; Cutting et al. Reference Cutting, Apperly, Chappell and Beck2014). In sum, technical-reasoning skills are not a confound, but perhaps the key cognitive skills explaining CTC and its innovative component. This is an aspect that has commonly been overlooked in the literature (for a similar viewpoint, see Caldwell et al. Reference Caldwell, Renner and Atkinson2018).
3.5. Interim conclusion
The emphasis on the social dimension of CTC has led researchers to assume that social cognitive skills are the precursor of CTC. Teachers' theory-of-mind skills can favor cooperation or play a boosting role in situations where learners lack information (e.g., nothing-is-present situations or situations in which the process used to make an artifact is opaque for the learner). Nevertheless, the emergence of heterotechnic cooperation or the amount of information that can be extracted from social transmission is fundamentally dependent on (learners') technical-reasoning skills. The learner-centered perspective defended here has the merit of refining previous proposals based on a teacher-centered perspective. For instance, Csibra and Gergely (Reference Csibra, Gergely, Munakata and Johnson2006) stressed that teaching (or pedagogy) is characterized by the explicit manifestation of generalizable knowledge by a teacher, a key aspect that could distinguish active direct teaching in humans from other forms of teaching in nonhumans (see Csibra Reference Csibra2007). However, even if a teacher can help a learner to generalize knowledge, the ability to generalize does not depend on the teacher's cognitive architecture but is intrinsic to that of the learner. We therefore suggest that although theory-of-mind skills can favor the transmission of technical information, the potential to acquire, generalize (i.e., analogical reasoning), and improve it is orthogonal to theory of mind because it is based on technical-reasoning skills.
This leads us to propose the virtuous circle hypothesis of CTC, which is intended to solve the question of how a learner can improve her or his technical-reasoning skills if, during each learning phase, the role of the teacher is minimal (Osiurak et al. Reference Osiurak, De Oliveira, Navarro and Reynaud2020a). The idea is as follows. First, models – and teachers – remain fundamental for social transmission. Even if they do not possess outstanding theory-of-mind skills, they still remain a potential source of information, so that everyone can learn even when taught by poor teachers. Second, the role of teachers might be limited to helping learners reach the upper limit of what they can learn in a given situation, an idea very similar to the concept of proximal developmental area (Vygotsky Reference Vygotsky1978). In the mid-/long-term, this may result in a progressive increase in learners' technical-reasoning skills, thus favoring the emergence of CTC.Footnote 12 Consequently, with regard to the claim that technical expertise is a confound and necessarily depends on previous social transmission (thereby emphasizing the social dimension of CTC), the virtual circle hypothesis holds that: (1) This is certainly right (although not systematic because people can also learn from asocial learning) and (2) without technical reasoning, the progressive accumulation of mechanical knowledge in a single individual via social transmission – i.e., the necessary condition for CTC – could not occur.
4. Metacognition
4.1. Theoretical framework
Metacognition can be broadly defined as cognition about cognition (Flavell Reference Flavell2000; Shea et al. Reference Shea, Boldt, Bang, Yeung, Heyes and Frith2014). Initially, Flavell (Reference Flavell1979) distinguished between metacognitive knowledge (i.e., knowledge about variables that can affect the course and outcome of a cognitive activity) and metacognitive experience (i.e., cognitive experiences that accompany and pertain to any cognitive activity). The former includes knowledge about one's own but also others' cognitive capacities, thus establishing a clear link with theory of mind. The latter belongs to the personal level and, as a result, cannot be directly linked to what others can experience – except, perhaps, in the form of a kind of inferential mechanism. More recently, Shea et al. (Reference Shea, Boldt, Bang, Yeung, Heyes and Frith2014) proposed a revised version of this framework based on two systems referred to as System 1 and System 2 (Heyes Reference Heyes2016; see also Dunstone & Caldwell Reference Dunstone and Caldwell2018). System 1 is very similar to Flavell's concept of metacognitive experience. It does not appear to be specific to humans. System 2 is responsible for forming interpersonal representations about what oneself and others know in a given situation. This second system is hypothesized to be unique to humans (for a similar view, see Gruber et al. Reference Gruber, Zuberbühler, Clément and van Schaik2015), and could differ from theory of mind in that it relates primarily to one's own cognitive processes and is the preferred basis for communication during cooperative activities as well as teachingFootnote 13 (Shea et al. Reference Shea, Boldt, Bang, Yeung, Heyes and Frith2014). In this regard, the metacognition hypothesis of CTC suggests that System 2 (simply referred to as metacognition below) provides the main underpinning for social-learning strategies (i.e., when and whom to copy), providing an adaptive value for humans in terms of CTC (Heyes Reference Heyes2016; Reference Heyes2018). This hypothesis provides an interesting cognitive framework for exploring key questions about social-learning strategies (i.e., transmission biases, flexibility, and overimitation) that we will discuss in turn below.
4.2. Transmission biases
Humans live in groups, thus giving them the opportunity to learn from many conspecifics. Evidence shows that group size is critical for CTC in that the presence of a high number of models is beneficial for the stability of a trait as well as for innovation through the combination of solutions produced by the different models (Derex & Boyd Reference Derex and Boyd2015; Derex et al. Reference Derex, Beugin, Godelle and Raymond2013a; Kemp & Mesoudi Reference Kemp and Mesoudi2014; Muthukrishna et al. Reference Muthukrishna, Shulman, Vasilescu and Henrich2014). Other evidence has been reported, highlighting an absence of or even an inverse relationship between population size and cumulative performance (Caldwell & Millen Reference Caldwell and Millen2010; Collard et al. Reference Collard, Kemery and Banks2005; Reference Collard, Vaesen, Cosgrove and Roebroeks2016; Fay et al. Reference Fay, De Kleine, Walker and Caldwell2019; Vaesen et al. Reference Vaesen, Collard, Cosgrove and Roebroeks2016). This discrepancy indicates that deciding whom to copy is a complicated task (Fay et al. Reference Fay, De Kleine, Walker and Caldwell2019). One possibility is that humans copy random things from random people. This form of transmission is called unbiased copying (e.g., individuals have a 60% probability of copying a trait that 60% of people possess; Boyd & Richerson Reference Boyd and Richerson1985). However, this kind of transmission is not adaptive (Boyd & Richerson Reference Boyd and Richerson1985; Giraldeau et al. Reference Giraldeau, Valone and Templeton2002; Rogers Reference Rogers1988) and does not account satisfactorily for the diffusion of innovation (Rogers Reference Rogers1995).
Consequently, another more likely possibility is that social transmission is necessarily biased by a certain number of rules, such as the prestige biasFootnote 14 or the conformist bias (Henrich Reference Henrich2001; Henrich & Gil-White Reference Henrich and Gil-White2001; see also Atkinson et al. Reference Atkinson, O'Brien and Mesoudi2012; Morgan et al. Reference Morgan, Rendell, Ehn, Hoppitt and Laland2012; Osiurak et al., Reference Osiurak, Cretel, Duhau-Marmon, Fournier, Marignier, De Oliveira, Navarro and Reynaudin press). For a cognitive scientist, the key question is to determine the cognitive skills underlying these kinds of bias. As pointed out by Heyes (Reference Heyes2018), interpretations in terms of cognitive processes are rare, because research on social-learning strategies has been dominated by disciplines concerned with what individuals do, not what they think (for a similar viewpoint, see Gruber Reference Gruber2016). Nevertheless, some interpretations have been proposed. For instance, Henrich and Gil-White (Reference Henrich and Gil-White2001) suggested that the prestige bias necessarily has its roots in the uniquely human ability of infocopying (a concept similar to imitation). Indeed, emulation leads individuals to focus only on the result and not on the process. The corollary is that if an individual only focuses on the result, she or he is unable to detect, among a number of different models, those who can achieve the expected result the most efficiently (i.e., the prestigious individual). This emphasis on imitation is consistent with the theory-of-mind hypothesis of CTC, even if the purpose differs. Whatever the case may be, this interpretation is difficult to support for the same reasons as evoked above: Nonhumans can exhibit imitation but not CTC. More problematically, the evidence indicates that conformist and prestige biases can also be observed in nonhumans (e.g., Horner et al. Reference Horner, Whiten, Flynn and de Waal2006; Reference Horner, Proctor, Bonnie, Whiten and de Waal2010; Kendal et al. Reference Kendal, Hopper, Whiten, Brosnan, Lambeth, Schapiro and Hoppitt2015; Whiten et al. Reference Whiten, Horner and de Waal2005). Another interpretation is that these biases may favor CTC by allowing humans to learn complex technologies more quickly from their conspecifics via technical reasoning. The distinction between humans and nonhumans would reside more in the complexity of the transmitted technique (see sect. 2.4) than in the kind of social-learning strategies employed (for a similar view, see Whiten et al. Reference Whiten, Horner and Marshall-Pescini2003).
4.3. Flexibility
There are many forms of social-learning strategies that can be distinguished depending on when (e.g., copy-when-uncertain and copy-when-unsatisfied) and whom (e.g., copy-the-majority and copy-successful-individuals, i.e., two strategies that lead to the above-mentioned conformist and prestige biases, respectively) to copy (Kendal et al. Reference Kendal, Boogert, Rendell, Laland, Webster and Jones2018; Laland Reference Laland2004). Models have shown that populations of flexible learners who switch between social and asocial learning at key moments outperform populations of purely social or purely asocial learners (Ehn & Laland Reference Ehn and Laland2012; Enquist et al. Reference Enquist, Eriksson and Ghirlanda2007; Rendell et al. Reference Rendell, Boyd, Cownden, Enquist, Eriksson, Feldman, Fogarty, Ghirlanda, Lillicrap and Laland2010). In line with this finding, it has been suggested that flexibility not only between social and asocial learning but also in social-learning strategies might be particularly adaptive and critical for CTC (Galef Reference Galef1995; Heyes Reference Heyes2016; Kendal et al. Reference Kendal, Coolen, van Bergen and Laland2005; Reference Kendal, Boogert, Rendell, Laland, Webster and Jones2018; Laland Reference Laland2004). Henrich and Gil-White (Reference Henrich and Gil-White2001) had already suggested that humans are default infocopiers (i.e., imitators) who try first to learn from others instead of “reinventing the wheel” and then seeking improvements via asocial learning. The metacognition hypothesis of CTC posits that this flexibility is based on the ability to form explicit strategies about when and whom to copy (Heyes Reference Heyes2016; Reference Heyes2018; Shea et al. Reference Shea, Boldt, Bang, Yeung, Heyes and Frith2014). Even if evidence is still needed to demonstrate that this flexibility is unique to humans (Kendal et al. Reference Kendal, Boogert, Rendell, Laland, Webster and Jones2018), this hypothesis is theoretically viable within a limited scope. We say “within a limited scope” because it provides an adequate account of the “when” and “who” strategies. By accessing one's own cognitive activity, an individual can explicitly decide to learn from others because she or he is uncertain or unsatisfied about her or his performance (the “when” strategies). By representing others' cognitive skills, the same individual can also explicitly decide to learn from successful individuals (the “who” strategy). Nevertheless, this hypothesis does not specify the domain of cognition targeted.
Metacognition is cognition about cognition. Therefore, given that we are concerned with technical skills, all these strategies – whether explicit or not – are necessarily based on a technical, cognitive content, and it seems most probable that this content takes the form of technical-reasoning skills. To some extent, this is consistent with Henrich and Gil-White's (Reference Henrich and Gil-White2001) proposal according to which social-learning strategies cannot be fully effective if individuals cannot accurately represent others' skills. When we observe someone else who is able to fix shelves very quickly by using a series of effective mechanical actions, the prestige we attribute to this individual results from our appraisal of her or his technical-reasoning skills, which differ from our own. In other words, the quality of our metacognitive representations could depend to a very great extent on the content of the targeted knowledge, with the result that the main difference between humans and nonhumans might not relate to metacognition but rather to the quality of the content processed by metacognition (i.e., technical-reasoning skills).
4.4. Overimitation
Overimitation refers to the imitation of actions that are causally irrelevant to the achievement of a goal (Lyons et al. Reference Lyons, Young and Keil2007; Reference Lyons, Damrosch, Lin, Macris and Keil2011; see also Nagell et al. Reference Nagell, Olguin and Tomasello1993). This phenomenon has aroused interest in the CTC research community because of the commonly made link between imitation and CTC (Legare & Nielsen Reference Legare and Nielsen2015; Shipton & Nielsen Reference Shipton and Nielsen2015). Evidence indicates a trend toward increasing overimitation with age, with most 2-year-olds exhibiting emulation, and most 5-year-olds exhibiting overimitation (Horner & Whiten Reference Horner and Whiten2005; McGuigan & Whiten Reference McGuigan and Whiten2009; McGuigan et al. Reference McGuigan, Whiten, Flynn and Horner2007; Nielsen & Tomaselli Reference Nielsen and Tomaselli2010). Overimitation might be even greater in adults (McGuigan Reference McGuigan2012; McGuigan et al. Reference McGuigan, Makinson and Whiten2011; see also Caldwell et al. Reference Caldwell, Schillinger, Evans and Hopper2012). A potential interpretation of this phenomenon is that humans may ontogenetically develop specific social-learning strategies, progressively leading them to copy prestigious and competent individuals (i.e., prestige bias; McGuigan Reference McGuigan2012). In line with this idea, a significant body of evidence has indicated that children can exhibit two classes of biases, the first being to display a preference for the information provided by a familiar caregiver versus a stranger and the second being to display a preference for someone who has proven to be a reliable source of information in the past (Harris & Corriveau Reference Harris and Corriveau2011). The corollary is that children may prefer to learn from individuals who provide reliable information and care, namely parents (Hewlett et al. Reference Hewlett, Fouts, Boyette and Hewlett2011). However, because their parents may be less accurate and reliable than other individuals, children may progressively opt for more accurate informants, leading to a shift from vertical to oblique or horizontal transmission (Henrich & Broesch Reference Henrich and Broesch2011; see also Harris & Corriveau Reference Harris and Corriveau2011). This perspective can explain why the aforementioned presence of overimitation found in dyadic interactions (one model: an adult; one learner: a child) tends to disappear in micro-society paradigms in which children interact with one another (e.g., Flynn Reference Flynn2008).
To account for the developmental trajectory of overimitation, McGuigan (Reference McGuigan2012) suggested that young children may lack the cognitive “sophistication or motivation” needed to overimitate, thereby drawing a parallel between the emergence of overimitation and the development of social, cognitive skills. This proposal is consistent with the metacognition hypothesis of CTC in that children may progressively develop social-learning strategies based on their ability to accurately represent what they know, what others know, as well as whether what others know is reliable. However, to be complete, this hypothesis needs to specify the aspect of cognition at which these metacognitive skills are directed. As mentioned above, we assume that metacognitive skills necessarily work in concert with technical-reasoning skills, which are the only appropriate candidate for explaining how an individual can form representations about her or his own and others' technical skills. In other words, social-learning strategies such as the prestige bias cannot be the result of reflecting on cognitive skills in the abstract, but must instead be the result of thought focused on specific cognitive skills. In this perspective, the technical-reasoning hypothesis of CTC offers a revised interpretation of overimitation by stressing that this phenomenon could not occur in the absence of technical-reasoning skills. This hypothesis could be tested in left brain-damaged patients with tool-use disorders. The prediction is that these patients might not exhibit certain social-learning strategies (e.g., a prestige bias) because of their inability to anticipate the effectiveness of their mechanical actions as well as of those performed by others.
4.5. Interim conclusion
The metacognition hypothesis of CTC is based on the parallel between the apparently uniquely human ability to exhibit complex metacognition and the fact that CTC is present only in humans. As we have emphasized, this parallel needs to be proven. Nevertheless, this hypothesis offers a viable theoretical framework in which to interpret social-learning strategies, such as the conformist bias or the prestige bias. Nevertheless, it is subject to the same limitations as the theory-of-mind hypothesis. First, it cannot explain why signs of CTC are observed in reverse-engineering conditions in which social transmission is not direct. This observation is inconsistent with the metacognition hypothesis of CTC, which assumes that some strategies can be developed based on the detection of the most frequent variants or the variants proposed by prestigious and competent individuals, namely information that is lacking in reverse-engineering conditions. Second, metacognition is cognition about cognition. However, this hypothesis tends to overlook the domain of cognition in question and therefore offers an incomplete view of how metacognitive skills might operate. In the face of these limitations, we propose a revised interpretation according to which technical-reasoning skills can play a key role by providing humans with useful content for representing not only their own but also others' technical skills. In line with this, we recently demonstrated with a closed-group micro-society paradigm that participants tend to progressively copy the most prestigious individual of the group, that is to say the one with the best technical-reasoning skills (Osiurak et al., Reference Osiurak, Cretel, Duhau-Marmon, Fournier, Marignier, De Oliveira, Navarro and Reynaudin press). In other words, this study shows a direct link between social-learning strategies – and potentially metacognitive skills – and technical-reasoning skills.
5. Conclusion
CTC is both a social and technological phenomenon. By focusing on the social dimension, most accounts of CTC have tended to minimize the technical dimension and the potential influence of non-social cognitive skills, perhaps failing to see the elephant in the room. Here, we offer a cognitive view that contrasts with these accounts by assuming that CTC originates in uniquely human technical-reasoning skills. In broad terms, we assume that technical reasoning is a difference-maker that has allowed humans to become cumulative and this to a greater extent than in other species, thereby explaining why this phenomenon is unique to humans. This view acknowledges the important role played by social-learning mechanisms, which are crucial in that they enrich the technical content. As illustrated in our example of someone improving upon Einsteinian physics, social learning is undoubtedly a mechanism through which an incredible amount of information can be transmitted, and the technical solution that is created is novel only up to a point. Nevertheless, humans are not “passive” technical learners because they can also transform the acquired technique into new technical solutions. In this way, the technical-reasoning hypothesis is akin to the cultural attraction hypothesis, which stresses the key role of individual cognition in cultural transmission (e.g., Sperber Reference Sperber1996; Sperber & Hirschfeld Reference Sperber and Hirschfeld2004).
The technical-reasoning hypothesis of CTC also does not rule out the potential boosting role played by other cognitive skills, such as theory-of-mind or metacognitive skills. The former might be particularly useful when information is lacking and the latter might help in the selection of reliable models. Nevertheless, they are not necessary conditions for the emergence of CTC. In addition, one outstanding question is whether they should be considered as cognitively distinct or not, given the clear apparent link they share at a theoretical level. They also share other features, such as their close relationships with language skills, also considered as another potential factor of CTC (Pinker Reference Pinker2010; see also Dean et al. Reference Dean, Vale, Laland, Flynn and Kendal2014; Whiten & Erdal Reference Whiten and Erdal2012). Some authors have also stressed potential interactions with future-planning skills (Vale et al. Reference Vale, Flynn and Kendal2012). The question is whether all these cognitive processes are different labels for a single cognitive capacity (or even module), which consists of thinking about oneself or others in different spaces and times. At a phylogenetic level, we can hypothesize that technical-reasoning skills might have played a key role in the development of these skills, a viewpoint that resembles the cognitive niche hypothesis (Pinker Reference Pinker2010; Tooby & DeVore Reference Tooby, DeVore and Kinzey1987; see also Osiurak Reference Osiurak2017). In other words, the sophistication of hominin technology could have led to cognitive adaptations, such as the emergence of theory-of-mind skills.Footnote 15 However, the small but important difference here is that the level of sophistication of these other cognitive “modules” could not have been reached without technical-reasoning skills.
This leads us to address an overlooked, yet fundamental, question concerning CTC: Why do we continue to improve our technologies? Given that CTC is characterized by constant innovation, research on the topic should attempt to address the question of why we seem to want to constantly improve our technologies. One potential answer is that early hominin tool use could have been developed by necessity in response to environmental pressures (i.e., survival; Boesch & Tomasello Reference Boesch and Tomasello1998; Wynn Reference Wynn, Gibson and Ingold1993). This hypothesis has also been proposed for nonhumans, but has received no empirical support (e.g., Koops et al. Reference Koops, Visalberghi and van Schaik2014; Sanz & Morgan Reference Sanz and Morgan2013; but see Gruber Reference Gruber2016). However, this does not explain why we continue to make tools that have no apparent benefits for survival (e.g., TV remote control).Footnote 16
Another answer consists of focusing not on external incentives but rather on the intrinsic characteristics of the cognitive system. In this Lorentzian view, the answer is simple: We constantly improve our tools because we generate our own physical problems through technical-reasoning skills. As extensively discussed above, technical-reasoning skills allow us to produce technical solutions that increase our effectiveness and efficiency. In this way, they are a generator of solutions. However, our technical solutions are never fully effective/efficient, even if they can give us the illusion of constantly having to do less. It is not sufficient to think of an environmental effect (e.g., switching on the TV) to make it happen: motor actions are always necessary (pressing a button). There is therefore a gap here between the benefits provided by any technical solution and its ultimate goal (i.e., appearance of an environmental effect without any motor action). This gap represents a self-generated problem space, leading humans to find more effective/efficient solutions, as illustrated by the fantasies regarding teleportation and telekinesis that are present in human cultures (Hubert & Mauss Reference Hubert and Mauss1902; see Osiurak Reference Osiurak2017; Osiurak et al. Reference Osiurak, Jarry and Le Gall2010). It should be noted that some of these fantasies almost become reality. We can take the train to transport ourselves without moving (teleportation) or use a crane to move objects far too heavy for us (telekinesis). However, the fact that we are far from fulfilling them – and that we will certainly never fulfill them –, provides an increasing room for technical improvement. In this respect, the two sides of technical reasoning (i.e., as a generator of both problems and solutions) form an ideal, theoretical framework in which to understand the emergence and the cognitive origins of CTC in humans.
To conclude, we emphasize the implications of the technical-reasoning hypothesis for our understanding of CTC. To date, this hypothesis has received support from neuropsychological and neuroimaging research. It has recently been applied to the study of CTC by means of micro-society paradigms, which are useful for investigating the role of individuals' cognitive skills in CTC or comparing the quality of social transmission in different social-learning conditions (for a discussion of the strengths and limitations of micro-society paradigms, see Caldwell et al. Reference Caldwell, Atkinson, Blakey, Dunstone, Kean, Mackintosh, Renner and Wilks2019). These paradigms also allow us to collect empirical data that can be compared to theoretical data generated by modeling approaches to test their predictions. However, they also suffer from the limitation that they do not strictly reflect how technical transmission occurs in everyday life, such as in the case of an apprenticeship, in which the apprentice alternates between periods of social learning and individual learning and in which teaching – and particularly verbal teaching – might play a minor role (Gatewood Reference Gatewood and Dougherty1985; see also Wynn & Coolidge Reference Wynn and Coolidge2014). Therefore, we recommend that future micro-society studies introduce this temporal component (i.e., alternation between social and individual learning) to be more faithful to what really happens in technical transmission situations.
One related point concerns the undoubted interest of the technical-reasoning hypothesis for cognitive archeology or neuroarcheology, two disciplines that aim to investigate stone knapping using experimental psychology or neuroscientific methods, respectively. Some attempts have been made to do this and have emphasized the role of certain parietal structures in stone knapping (Hecht et al. Reference Hecht, Gutman, Khreisheh, Taylor, Kilner, Faisal, Bradley, Chaminade and Stout2015; Stout & Chaminade Reference Stout and Chaminade2007; Stout et al. Reference Stout, Passingham, Frith, Ape and Chaminade2011; see also Stout & Hecht Reference Stout and Hecht2017). This research is valuable because of the groundbreaking nature of its methodology. However, it does not provide a model of technical cognition that can be applied to other disciplines (Wynn et al. Reference Wynn, Haidle, Lombard, Coolidge, Wynn and Coolidge2017), in particular because it originates in classical neuropsychological models that ignore the important contribution of reasoning in tool behavior (see sect. 2.1). In this respect, the technical-reasoning hypothesis offers an original theoretical framework that can contribute to our insight into the importance of the understanding of functional parameters (i.e., physical principles) in stone knapping when compared to motor procedures (e.g., Bril et al. Reference Bril, Rein, Nonaka, Wenban-Smith and Dietrich2010).
Finally, we have to acknowledge that the technical-reasoning hypothesis is still a new hypothesis, which has been developed by only a few researchers. This is surprising if we consider how dramatically our technical skills have modified the surface of the Earth. Nevertheless, well-known handbooks of cognitive psychology or the cognitive sciences contain no sections on technical cognition. Considerable effort is therefore needed to develop a comprehensive, computational model that could be useful for modeling work, and in particular for testing the predictions of this hypothesis versus those derived from other hypotheses of CTC.
Acknowledgments
We thank Tim Pownall for the English proofreading of this manuscript. This work was supported by grants from ANR (Agence Nationale pour la Recherche; Project “Cognition and tool-use economy” ECOTOOL; ANR-14-CE30-0015-01), and was performed within the framework of the LABEX CORTEX (ANR-11-LABX-0042) of Université de Lyon, within the program “Investissements d'Avenir” (ANR-11-IDEX-0007) operated by the French National Research Agency (ANR).
Target article
The elephant in the room: What matters cognitively in cumulative technological culture
Related commentaries (26)
A cognitive approach to cumulative technological culture is useful and necessary but only if it also applies to other species
A cognitive developmental approach is essential to understanding cumulative technological culture
A cognitive transition underlying both technological and social aspects of cumulative culture
A little too technical: The threat of intellectualising technical reasoning
A long view of cumulative technological culture
A theory limited in scope and evidence
Causal learning in CTC: Adaptive and collaborative
Chimpanzees' technical reasoning: Taking fieldwork and ontogeny seriously
How will we find the elephant in the room?
Human tool cognition relies on teleology
Implications for technological reserve development in advancing age, cognitive impairment, and dementia
Missing in action: Tool use is action based
New Caledonian crows afford invaluable comparative insights into human cumulative technological culture
Putting social cognitive mechanisms back into cumulative technological culture: Social interactions serve as a mechanism for children's early knowledge acquisition
Refining our understanding of the “elephant in the room”
Shared intentionality shapes humans' technical know-how
Supporting the weight of the elephant in the room: Technical intelligence propped up by social cognition and language
Taking into account the wider evolutionary context of cumulative cultural evolution
Technical reasoning alone does not take humans this far
The blind men and the elephant: What is missing cognitively in the study of cumulative technological evolution
The crow in the room: New Caledonian crows offer insight into the necessary and sufficient conditions for cumulative cultural evolution
The social side of innovation
The technical reasoning hypothesis does not rule out the potential key roles of imitation and working memory for CTC
Tools as “petrified memes”: A duality
What matters emotionally: The importance of pride for cumulative culture
Where does the elephant come from? The evolution of causal cognition is the key
Author response
The elephant in the China shop: When technical reasoning meets cumulative technological culture