Diabetes presents a significant health challenge across the globe, mainly affecting middle-aged and elderly populations( Reference Maki and Phillips 1 ). Type 2 diabetes is associated with fatty liver disease and CVD( Reference Leon and Maddox 2 , Reference Alwahsh, Dwyer and Forbes 3 ). Obesity has been recognised as an important risk factor for insulin resistance and type 2 diabetes( Reference Maki and Phillips 1 ). Numerous studies have searched for effective weight loss interventions that normalise glucose tolerance( Reference Shai, Schwarzfuchs and Henkin 4 , Reference McAuley, Smith and Taylor 5 ).
Excess energy intake and certain dietary habits, such as a high-fat diet and a high-fructose diet, are associated with increased risk of obesity and metabolic disturbance, including type 2 diabetes and fatty liver( Reference Meyer, Kushi and Jacobs 6 – Reference Samocha-Bonet, Campbell and Mori 10 ). Assessing the significance of relative proportions of macronutrient intake on health outcomes has become an active area of research. Recent randomised trials demonstrated more favourable outcomes associated with a low-carbohydrate diet for both weight loss and metabolic parameters as compared with a low-fat diet( Reference Shai, Schwarzfuchs and Henkin 4 , Reference Bazzano, Hu and Reynolds 11 – Reference Foster, Wyatt and Hill 13 ). However, other comparative studies on low-fat diet, low-carbohydrate diet and other types of diets, as well as systematic reviews, have shown inconsistent results( Reference McAuley, Smith and Taylor 5 , Reference Tobias, Chen and Manson 14 – Reference Naude, Schoonees and Senekal 16 ).
One major problem with the classification of diets based on macronutrient proportions is the allocation of complex carbohydrate, dietary fibre and refined carbohydrate into the same category as ‘carbohydrate’. Diets high in fibre, such as the Mediterranean diet, have been associated with improved insulin sensitivity( Reference Shai, Schwarzfuchs and Henkin 4 , Reference Salmeron, Manson and Stampfer 17 ), whereas diets high in refined carbohydrate have been associated with insulin resistance( Reference Barclay, Petocz and McMillan-Price 18 ). Thus, these findings suggest the need to develop a better index to classify diets based on the ‘quality’ of carbohydrate.
East Asians carry increased risk of developing insulin resistance and type 2 diabetes at a lower BMI as compared with Caucasians, partly owing to the tendency to accumulate excess body fat without large increases in BMI( Reference Hsu, Araneta and Kanaya 19 ). Studies on the relationship between dietary factors and insulin resistance are relatively scarce in East Asian populations( Reference Nanri, Mizoue and Noda 20 – Reference Hu, Pan and Malik 22 ). In some studies conducted among Chinese and Japanese, higher consumption of white rice was associated with increased risk of developing type 2 diabetes( Reference Nanri, Mizoue and Noda 20 , Reference Villegas, Liu and Gao 21 ). However, one of these studies did not take into account the effect of dietary fibre in the analysis( Reference Villegas, Liu and Gao 21 ), highlighting the need to investigate the relationship between the quality of carbohydrate and insulin resistance.
In this study, we examined the relationship between the ratio of dietary fibre to carbohydrate intake (fibre:carbohydrate ratio (F:C-R)) and insulin resistance and HbA1c, two strong indicators of future risk of diabetes, in Japanese adult subjects without diabetes participating in a 5-month diet and exercise programme. In addition, we examined the potential modification in association when accounting for individual carrier status of β-3 adrenergic receptor (β3AR) functional gene polymorphism, a locus known to be associated with the risk of obesity( Reference Clement, Vaisse and Manning 23 – Reference Kim-Motoyama, Yasuda and Yamaguchi 25 ). We also investigated the longitudinal relationship between changes in the F:C-R and changes in homoeostasis model of assessment for insulin resistance (HOMA-IR) and HbA1c.
Methods
Study population
The Nutrition Clinic at Kagawa Nutrition University has a history of providing a diet and exercise programme for over 45 years( Reference Kagawa and Kagawa 26 ). The clinic offers a voluntary 5-month diet and exercise programme semi-annually called the Healthy Diet Course. The participants include not only overweight/obese individuals, but also normal-weight individuals who have the desire to improve the quality of their diets. Participants are recruited through advertisements in television programmes and magazines on health and dieting, the website of the Nutrition Clinic, flyer distribution to participants attending seminars on nutrition at the Nutrition Clinic and annual invitation to individuals who have completed the programme previously via mail. The inclusion criteria for the programme are those aged 20 years or older and the absence of impairment in the knees or hip that limits mobility (walking up and downstairs).
At the beginning and end of the sessions, participants undergo anthropometric measurements and biochemical analyses of blood samples. During the 5-month period, participants are required to attend 12 sessions at the Nutrition Clinic. Each session, usually lasting for 6 h, consists of group sessions, individual nutritional counselling, lectures, cognitive behavioural therapy and exercise regimen. In group sessions, registered dietitians give lectures on food selection and cooking. In individual nutritional counselling, dietitians review participants’ diet records and advise participants to ensure that their meals consist of grains, main dish and side dish, and to increase the amount of vegetables, mushrooms and seaweeds that are rich in dietary fibres. The counselling is conducted based on Dietary Reference Intakes of Japanese People 2010, which recommends males and females at 18 years or older to consume over 19 and 17 g of dietary fibre, respectively( 27 ). At ten of the twelve sessions, participants are provided with lunch, which consists of germ rice, main dish (fish or meat), side dishes (vegetables, mushrooms and/or seaweeds), soup and dessert (fruits). Each lunch contains approximately 2510 kJ (600 kcal) of total energy, 10 g of dietary fibre and <3 g of salt. Participants are also given lectures on lifestyle-related disease and genetics by a medical doctor. Exercise regimen is a 70-min session led by a health fitness programmer, and consists of the use of rubber bands, balance balls, chairs, stretching, squatting and gymnastics that are intended to strengthen the pelvic joint, bones and muscles.
A total of 223 participants were enrolled in the programme between October 2006 and March 2015. Of the 223 participants of both sexes, those missing data on baseline body weight, percentage body fat and energy intake were excluded, resulting in 217 participants with complete baseline data. Of the 217 participants, those with diabetes (n 27) were excluded, leaving 190 participants for our cross-sectional analyses. Participants were considered to have diabetes if they met any of the following criteria: baseline HbA1c of 6·5 % or higher( Reference Kashiwagi 28 , Reference Kharroubi, Darwish and Al-Halaweh 29 ), use of anti-hyperglycaemic medication or prior diagnosis of diabetes. Of the 190 participants, those missing data on body weight, percentage body fat and energy intake at the end of the programme were excluded, leaving 177 participants for the longitudinal analyses. For those who were enrolled in the programme more than once during the period, we used data from the initial enrolment.
This study was conducted according to the guidelines laid down in the Declaration of Helsinki and all procedures involving human subjects/patients were approved by the Institutional Review Board of Kagawa Nutrition University. Written informed consent was obtained from all subjects (n 223).
Anthropometric data
Height was measured to the nearest millimetres by a standiometer (A&D Company, Limited). Weight and percentage body fat were measured by dual X-ray absorptiometry (DEXA) (Osteometer, Toyo Medic). Weight was measured to the nearest 0·1 kg. Body fat mass was calculated by multiplying body weight and percentage body fat as measured by DEXA. Lean body mass was calculated by subtracting body fat mass from body weight( Reference Aloia, Vaswani and Ma 30 ). BMI was calculated by dividing weight in kilograms by height in metres squared.
Dietary and lifestyle data
Each participant completed 3-d diet records at the beginning and at the end of the programme. To improve accuracy, participants were advised to measure the amount of food they ate by weighing each food with a scale. Registered dietitians reviewed each participant’s diet records and interviewed each participant regarding the amount of food consumed to detect missing food or inconsistent reporting. When the reported amount of food items appeared obviously incorrect, dietitians corrected it based on a food database of the food items and confirmed it with the participant. Mean nutrient intakes per day were calculated by entering the diet record data into a diet analysis program (Excel Eiyo-kun, version 6.0; Kenpaku-sha). A ratio of fibre intake to carbohydrate intake (F:C-R) was calculated by dividing fibre intake by total carbohydrate intake and multiplying it by 100.
We assessed the validity of self-reported diet records at baseline through the Goldberg method( Reference Black 31 ). Specifically, we compared the group mean of reported energy intake with the BMR ratio (EI:BMR) and the 95 % lower or upper confidence limit calculated by the following formula: PAL×exp{sd×[(S/100)/√n]}, where PAL is the mean physical activity level for the study group, sd −2 for the 95 % lower confidence limit and +2 for the 95 % upper confidence limit, and n the number of subjects in the study( Reference Black 31 ). S refers to the factor that takes into consideration the variation in intake, BMR and energy requirement, and is given by the following formula: S=[(CVwEI)2/d+(CVwB)2+(CVtP)2]1/2, where CVwEI is the within-subject CV in energy intake, d the number of days of diet records, CVwB the CV of repeated BMR measurements or the precision of estimated compared with measured BMR and CVtP the total variation in PAL( Reference Black 31 ). For our calculation, we set PAL as 1·55, which is the value used by the World Health Organization for ‘light’ activity( Reference Black 31 ). We used the following CV values (CVwEI 23 %, CVwB 8·5 %, CVtP 15 %) as applied in a previous Japanese study( Reference Murakami 32 ). As for BMR, we used the weight-adjusted predictive equation given in the Dietary Reference Intakes for Japanese, 2010( Reference Miyake, Tanaka and Ohkawara 33 ). The absence of under-reporting or over-reporting is suggested by an EI:BMR within the range of the 95 % lower and upper confidence limits( Reference Black 31 ).
Average number of steps per day was the mean of steps taken a day for 7 d as measured by a pedometer (Tanita). The measurement was done during the 1st week of the programme and at the end of the programme.
Biochemical profile and blood pressure data
Fasting blood samples were collected after overnight fasting at the beginning and end of the programme. Blood samples were sent to LSI Medience Corporation for the analyses of biochemical profile. Complete blood counts, serum levels of electrolytes, liver enzymes, glucose, insulin, HbA1c, total cholesterol, LDL-cholesterol, HDL-cholesterol and TAG were measured. HOMA-IR was calculated by the following formula: (fasting plasma insulin×fasting plasma glucose (mg/dl))/405 ((fasting plasma insulin×fasting plasma glucose (mmol/l))/22·5)( Reference Matthews, Hosker and Rudenski 34 ).
Blood pressure was measured in the seated position with the arm at the level of the heart using a TM-2665P Automatic Tabletop Blood Pressure Monitor (A&D Medical) at the beginning and end of the programme.
Genetic polymorphism data
β3AR are mainly expressed on adipose cells and play an important role in lipolysis and thermogenesis( Reference Clement, Vaisse and Manning 23 ). Participants were genotyped for β3AR gene by PaGE Science Co., Ltd, because the variant of β3AR (Trp64Arg) has been associated with higher BMI( Reference Mitchell, Blangero and Comuzzie 24, Reference Kim-Motoyama, Yasuda and Yamaguchi 25 ) and increased capacity to gain weight( Reference Clement, Vaisse and Manning 23 ). Those who had at least one Trp64Arg allele (rs4994) were considered carriers.
Statistical analysis
For the cross-sectional descriptive analyses of the baseline data, we divided the subjects into two groups using the 50th percentile value of the F:C-R as a cut-off and labelled the groups as high- and low-ratio groups. This was used in a bivariate analysis to compare mean values of anthropometric, metabolic and dietary parameters using the Student’s t test, and proportion of female participants, carriers of β3AR polymorphism and individuals taking medications for dyslipidaemia and hypertension using the χ 2 test.
Next, we used multivariable regression to investigate the independent association between the F:C-R and HOMA-IR after controlling for potential confounders. HOMA-IR was log-transformed and initial selection of variables into the multivariable model was based on clinical hypotheses regarding factors thought to affect HOMA-IR, as well as consideration of the association results from the bivariate analyses. The same covariates were considered in the analysis of the F:C-R and HbA1c. For the longitudinal analysis, we evaluated the relationship between absolute changes in F:C-R and changes in HOMA-IR or HbA1c using multivariable linear regression adjusting for potential confounders. We ran the model including change in the F:C-R, age, sex and variables that showed suggestive associations (P-value<0·20) in the unadjusted analyses. Variables were excluded in a step-wise manner if they did not influence the F:C-R association. We presented coefficients, 95 % CI and P-values for each variable.
Additionally for each model, we tested the presence of multicollinearity by checking variance inflation factors (VIFs) and excluded variables with high VIF until all included variables had VIF of <5. We performed all statistical analyses in STATA 13 (StataCorp LP) and Microsoft Excel. We considered a two-tailed P<0·05 as statistically significant.
Results
The cross-sectional analyses were performed on 190 participants (180 female and ten male; aged 21–78 years) based on baseline data, and the longitudinal evaluations included a large subset of 177 participants (168 female and nine male) who also had complete follow-up data. Regarding the validity of self-reported diet records, we found the EI:BMR to be within the range of the 95 % lower and upper confidence limits, suggesting the absence of under-reporting or over-reporting as a group.
Median baseline F:C-R among the participants was 6·38. Table 1 shows the means of demographic, anthropometric, metabolic and dietary variables in the high F:C-R group (above median value) and low-ratio group (below median value). Compared with the low F:C-R group, the high-ratio group showed significantly lower mean body weight (61·9 v. 66·4 kg, P=0·004) and lean body mass (40·1 v. 42·8 kg, P=0·002). A similar relationship was observed for body fat mass (P=0·054). The high F:C-R group also showed lower mean fasting serum insulin levels (6·5 v. 8·6 μU/ml, P<0·001) and HOMA-IR (1·5 v. 2·0, P=0·002). Mean HbA1c level also appeared lower in the high F:C-R group as compared with the low F:C-R group, which approached statistical significance (5·6 v. 5·7 %, P=0·081). The high F:C-R group had significantly higher mean intake of protein (73·5 v. 68·5 g, P=0·026), grams of protein intake per kg of standard weight (1·4 v. 1·2 g/kg standard weight, P=0·002) and percentage energy from protein (16·6 v. 15·0 %, P<0·001) than did the low-ratio group. Fibre intake was also significantly greater in the high-ratio group as compared with the low-ratio group (19·3 v. 12·8 g, P<0·001), but the two groups showed similar mean total carbohydrate intake (242·4 v. 247·3 g, P=0·513) and fat intake (55·1 v. 56·0 g, P=0·654). As for energy intakes by food groups, the high F:C-R group had significantly greater energy intakes from fish, beans, green/yellow vegetables, plain-coloured vegetables, mushrooms and seaweeds, and fruits, and lower energy intakes from grains and oil food as compared with the low F:C-R group.
Table 1 Baseline characteristics of study participants divided by fibre:carbohydrate ratio (F:C-RFootnote *) (Mean values with their standard errors; numbers and percentages)
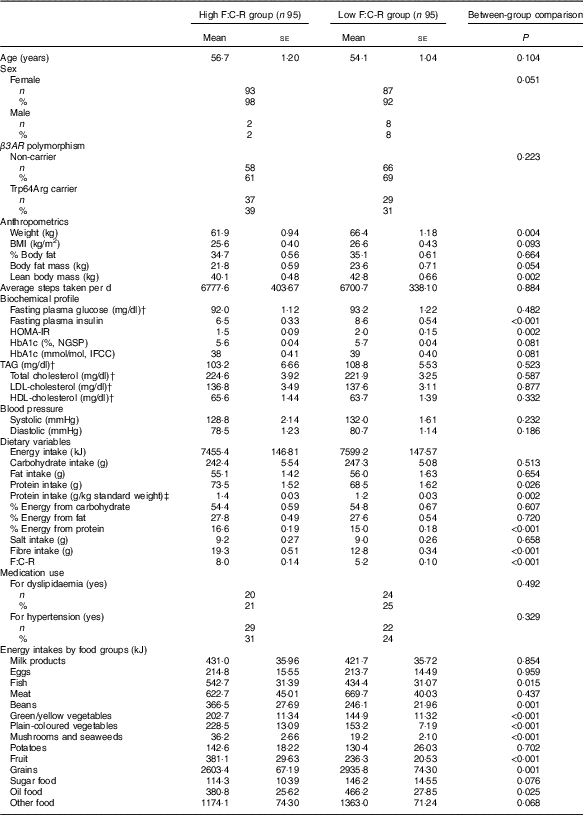
β3AR, β-3 adrenergic receptor; HOMA-IR, homoeostasis model of assessment for insulin resistance; NGSP, National Glycohemoglobin Standardization Program; IFCC, International Federation of Clinical Chemistry and Laboratory Medicine.
* F:C-R was calculated by dividing fibre intake by total carbohydrate intake and multiplying it by 100.
† To convert glucose in mg/dl to mmol/l, multiply by 0·0555; to convert TAG in mg/dl to mmol/l, multiply by 0·0113; to convert cholesterol in mg/dl to mmol/l, multiply by 0·0259.
‡ A standard weight for each participant was calculated by multiplying the square of height in metres by 22.
Table 2 shows results of the multivariable linear regression analysis evaluating the association between F:C-R and log HOMA-IR and HbA1c levels at baseline, adjusting for age, sex, β3AR polymorphism, body fat mass, lean body mass, average number of steps taken and intakes of total energy, carbohydrate, fat and protein. F:C-R was not associated with log HOMA-IR (P=0·214), whereas sex, β3AR polymorphism and body fat mass were significantly associated with log HOMA-IR. In the multivariable analysis of baseline HbA1c level, F:C-R was not significantly associated with HbA1c (P=0·615), whereas increasing age was positively associated with HbA1c (P<0·001).
Table 2 Coefficients of variables included in the multiple linear regression models for baseline log homoeostasis model of assessment for insulin resistance (HOMA-IR) and HbA1cFootnote * (Coefficients and 95 % confidence intervals)
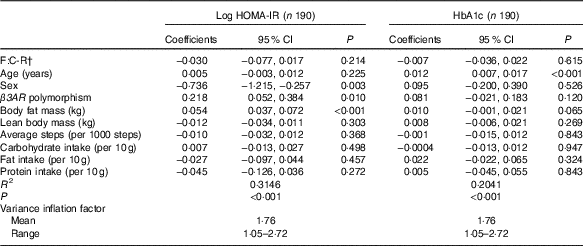
F:C-R, fibre to carbohydrate ratio; β3AR, β-3 adrenergic receptor.
* Baseline energy intake was included in the original model; however, the variable was excluded from the final model owing to multicollinearity.
† F:C-R was calculated by dividing fibre intake by total carbohydrate intake and multiplying it by 100.
Online Supplementary Table S1 shows changes in energy intakes by food groups across the 5-month study period. On average, participants increased their energy intakes from beans, green/yellow vegetables, plain-coloured vegetables, mushrooms and seaweeds, potato and fruits, whereas their energy intakes from milk, egg, fish, meat, grain, sugar food, oil food and other food decreased.
Table 3 presents results of the analysis evaluating factors associated with changes in HOMA-IR. The unadjusted analysis showed that increases in the F:C-R during the 5-month follow-up was associated with a reduction in HOMA-IR (P=0·009). Adjustment for other covariates attenuated the association (P=0·093). The covariates included age, sex, β3AR polymorphism, baseline measures (HOMA-IR, body fat mass, lean body mass, average number of steps taken, intakes of total energy, carbohydrate, fat, protein and F:C-R) and changes in average number of steps taken, change in intakes of total energy, carbohydrate, fat and protein, and percentage changes in body fat mass and lean body mass. Inclusion of percentage change in body fat mass, a hypothesised intermediate in the causal pathway, in the model greatly attenuated the association between the F:C-R change and HOMA-IR change (P=0·515).
Table 3 Coefficients of variables included in the multiple linear regression models for changes in homoeostasis model of assessment for insulin resistance (HOMA-IR)

F:C-R, fibre:carbohydrate ratio; β3AR, β-3 adrenergic receptor.
* The adjusted model initially included F:C-R change, age, sex and variables with a P-value<0·20 in the unadjusted analysis. Percentage change in body fat mass was excluded because it is considered to be in the pathway of F:C-R change leading to HOMA-IR change. Change in average steps, sex and baseline lean body mass were excluded from the model owing to the minimal influence on F:C-R change.
† F:C-R was calculated by dividing fibre intake by total carbohydrate intake and multiplying it by 100.
Table 4 shows the regression coefficients for variables included in the multivariable models for changes in HbA1c. Increases in the F:C-R during the 5-month follow-up was associated with a reduction in HbA1c (P<0·001), even after adjusting for potential confounders, including age, sex, β3AR polymorphism, baseline measures (HbA1c, body fat mass, lean body mass, average number of steps taken, intakes of total energy, carbohydrate, fat and protein, and F:C-R) and changes in average number of steps taken; change in intakes of total energy, carbohydrate, fat and protein; and percentage changes in body fat mass and lean body mass. In addition, a higher baseline HbA1c level was associated with greater decreases in HbA1c in the multivariable model (P<0·001).
Table 4 Coefficients of variables included in the multiple linear regression models for changes in HbA1c

F:C-R, fibre:carbohydrate ratio; ; β3AR, β-3 adrenergic receptor.
* The adjusted model initially included F:C-R change, age, sex and variables with a P-value <0·20 in the unadjusted analysis. Percentage change in body fat mass was excluded because it is considered to be in the pathway of F:C-R change leading to HbA1c change. Change in fat intake, sex, baseline body fat mass and lean body mass were excluded from the model owing to the minimal influence on F:C-R change.
† F:C-R was calculated by dividing fibre intake by total carbohydrate intake and multiplying it by 100.
Because β3AR polymorphism was significantly associated with log-transformed baseline HOMA-IR, we evaluated the presence of effect modification (statistical interaction) by the polymorphism. The interaction model (including a term representing the product of the baseline F:C-R and carrier status of the β3AR polymorphism) adjusting for potential confounders approached statistical significance (P=0·093), suggesting potential heterogeneity in effect of F:C-R on HOMA-IR by β3AR polymorphism carrier status. In stratified multivariable analyses, the baseline F:C-R was not significantly associated with baseline log HOMA-IR (coefficient=0·0141, P=0·647) in non-carrier participants; however, in participants carrying the polymorphism, F:C-R was inversely associated with log HOMA-IR (coefficient=−0·0970, P=0·018).
Discussion
To the best of our knowledge, no past studies have specifically investigated the relationship between F:C-R and measures of glucose tolerance. We observed, in a cross-sectional analysis, that individuals with a higher ratio of fibre to carbohydrate intake had significantly lower HOMA-IR as compared with those with a lower ratio. Interestingly, the higher-ratio group and the lower-ratio group had very similar mean carbohydrate and fat intakes, as well as percentage energy from these nutrients; however, the two groups had significantly different HOMA-IR levels. This finding may suggest the presence of factors other than relative proportions of macronutrients that significantly affect insulin resistance.
We also demonstrated an inverse association between changes in F:C-R and changes in HbA1c after adjusting for potential confounders. The result suggests that, on average, participants with greater increase in the F:C-R over the 5-month period had greater reduction in HbA1c. Our results are supported by previous studies showing an association between higher fibre intake and lower risk of incident diabetes( Reference Meyer, Kushi and Jacobs 6 , Reference Schulze, Liu and Rimm 7 ). The analysis of HOMA-IR showed a similar association, which was not, however, statistically significant after adjustment for potential confounders. The disparity in association could be attributed to the possibility that an increase in F:C-R may result in reduced postprandial glucose levels, which are more strongly correlated with HbA1c levels as compared with fasting plasma glucose levels( Reference Ketema and Kibret 35 ). Some studies have shown that soluble dietary fibre can help reduce postprandial glucose levels potentially through slowing the rate of glucose absorption in the intestine( Reference Jenkins, Wolever and Leads 36 , Reference Jenkins, Goff and Leeds 37 ). Increase in F:C-R may have had smaller effects on fasting plasma glucose levels, which were used to calculate HOMA-IR, over the study period.
Interestingly, we also observed evidence that the Trp64Arg polymorphism may modify the relationship between the F:C-R and baseline HOMA-IR. The ratio was inversely associated with log HOMA-IR at baseline in carriers. The variant of the β3AR gene (Trp64Arg) has been considered one candidate locus that increases susceptibility to obesity and insulin resistance( Reference Clement, Vaisse and Manning 23 , Reference Walston, Silver and Bogardus 38 , Reference Walston, Silver and Hilfiker 39 ). Some studies showed association of the variant with higher BMI( Reference Mitchell, Blangero and Comuzzie 24 , Reference Kim-Motoyama, Yasuda and Yamaguchi 25 ), increased capacity to gain weight( Reference Clement, Vaisse and Manning 23 ) and early onset of type 2 diabetes mellitus( Reference Walston, Silver and Bogardus 38 , Reference Walston, Silver and Hilfiker 39 ), although not all studies support the association( Reference Gagnon, Mauriege and Roy 40 , Reference Elbein, Hoffman and Barrett 41 ). These studies did not account for dietary factors in their analyses( Reference Clement, Vaisse and Manning 23 – Reference Kim-Motoyama, Yasuda and Yamaguchi 25 , Reference Walston, Silver and Bogardus 38 – Reference Elbein, Hoffman and Barrett 41 ). Further investigation is warranted to evaluate whether carriers of this polymorphism may gain weight and develop insulin resistance more easily as compared with non-carriers if their F:C-R are low.
Our findings have significant implications on the dietary interventions aimed at type 2 diabetes prevention. Although results from some randomised trials favour low-carbohydrate diets as compared with high-carbohydrate diets( Reference Shai, Schwarzfuchs and Henkin 4 , Reference Bazzano, Hu and Reynolds 11 – Reference Foster, Wyatt and Hill 13 ), our findings raise the possibility that a high-carbohydrate diet may be a feasible dietary strategy for diabetes prevention as long as F:C-R is maintained at a high level, as was the case for traditional Asian diets( Reference Hsu, Lau and Matsumoto 42 ). This possibility is important because diets relatively high in carbohydrate with a high F:C-R may be more sustainable than low-carbohydrate diets, which were shown to have higher attrition rates in some randomised trials lasting 1 year or longer( Reference Shai, Schwarzfuchs and Henkin 4 , Reference Iqbal, Vetter and Moore 43 ). Furthermore, a meta-analysis of observational studies showed increased risk of all-cause mortality in subjects on low-carbohydrate diets( Reference Noto, Goto and Tsujimoto 44 ). Long-term large-scale prospective studies are warranted to investigate the combined effects of different relative proportions of macronutrients and F:C-R on weight, metabolic profile and dropout rates.
Taking a ratio of fibre to carbohydrate intake may have an advantage over simply measuring the intake of fibre in terms of predicting the level of insulin resistance. Two individuals with equal intake of dietary fibre could have markedly different intakes of refined sugars and total energy, which likely affects their risk of developing insulin resistance( Reference Barclay, Petocz and McMillan-Price 18 ). F:C-R may differentiate such individuals and enable clinicians or dietitians to identify those at increased risk of metabolic disturbance. Given the scarcity of studies comparing the effect of diets with equal amount of fibre and different levels of total carbohydrate intakes, this topic warrants further investigation.
Among the fibre-containing food groups, the high F:C-R group had significantly greater energy intakes from beans, green/yellow vegetables, plain-coloured vegetables, mushrooms and seaweeds and fruits, as compared with the low F:C-R group. Frequently consumed foods for each of these food groups were as follows – (1) beans: tofu (firm tofu, silken tofu, fried tofu), natto (fermented soyabeans); (2) green/yellow vegetables: carrot, spinach, tomato, pumpkin, broccoli; (3) plain-coloured vegetables: daikon radish, onion, cabbage, Chinese cabbage, cucumber; (4) mushrooms and seaweeds: shimeji, shiitake, enokitake, wakame (sea mustard), hijiki; (5) fruits: banana, apple, mandarin. Taking the average F:C-R of these foods based on a Japanese food composition book (Excel Eiyo-kun, version 8.0) showed that mushrooms and seaweeds and beans had F:C-R above 50, and green/yellow vegetables and plain-coloured vegetables had F:C-R above 30. Thus, recommending foods from these food groups is likely to lead to practical dietary advice that can increase the F:C-R of a person’s diet.
Our study has several strengths. First, a ratio of dietary fibre to carbohydrate intake may be easy for lay individuals who are not trained in dietetics to use. People can imagine that foods high in fibre, such as vegetables, legumes and brown rice, increase the ratio, whereas refined grains, sugary snacks and sweetened beverages increase total carbohydrate intake without increasing fibre intake, thus lowering the ratio. Thinking of how each food they consume changes their diet’s overall F:C-R can help them design dietary regimens with favourable ratios that may improve their glucose tolerance. Second, our ability to investigate the association between longitudinal changes in F:C-R and glucose tolerance addressed the temporality assumption in causal inference and provided added confidence in the results. Third, the quality of dietary data obtained in this study is likely to be high because participants were encouraged to weigh the amount of food using a scale, and registered dietitians interviewed each participant regarding the amount of foods consumed and the seasonings used in order to improve the accuracy of the diet records. Moreover, we evaluated the validity of self-reported diet records by the Goldberg method( Reference Black 31 ), which suggested the absence of under-reporting or over-reporting.
Our study also had limitations. First, the duration of the diet and exercise programme was 5 months. We cannot conclude whether our results are applicable for a longer period of time, but expect that dietary changes maintained beyond this period would provide sustained or additional improvements in insulin resistance parameters. Second, most of the participants were middle-aged or elderly Japanese women without diabetes. Thus, the findings from this study may not be extrapolated to other racial/ethnic groups or male subjects who are affected with diabetes. Whether a high F:C-R diet would help to improve glucose tolerance in patients with type 2 diabetes is uncertain and cannot be concluded from the study. However, our findings are important because research on patients without type 2 diabetes has been scarce. Third, it is possible that the 3-d diet records provided by the participants may not reflect their usual dietary intake; however, because the purpose of this self-initiated voluntary 5-month programme was to improve their dietary habit, it is unlikely that they intentionally overestimated or underestimated their food intakes, as suggested by the validity evaluation using the Goldberg method( Reference Black 31 ). Fourth, the actual amount of physical activity that participants engaged might not have been well captured by average number of steps taken per day, which was included in our analyses. Because physical activity has been shown to be associated with lower risk of incident type 2 diabetes( Reference Hu, Leitzmann and Stampfer 45 – Reference Honda, Kuwahara and Nakagawa 47 ), more accurate measures of physical activity would have improved the quality of our study. Fifth, intakes of simple carbohydrates, such as glucose and fructose, were not separately measured in this study. Because differential effects of these carbohydrates on metabolic disturbances have been demonstrated( Reference Stanhope, Schwarz and Keim 48 ), this topic merits further investigation in future studies. Last, although we considered a comprehensive breadth of potential confounders, like most observational studies, we cannot completely rule out the influence of residual confounding and/or unknown confounders. These limitations, however, are unlikely to undermine the significance of a F:C-R in diabetes prevention given supportive evidence derived from two different measures of glucose tolerance, the strength of associations with HbA1c and confirmation by longitudinal analyses in the context of previous supportive scientific literature.
Conclusion
We found that an increase in F:C-R was associated with a reduction in HbA1c in Japanese subjects enrolled in a 5-month diet and exercise programme. Our findings suggest the possibility that a relatively high-carbohydrate diet can be a viable strategy for diabetes prevention if a F:C-R is maintained at a high level. The relationship among relative proportions of macronutrients, F:C-R and insulin resistance merits further investigation. Overall, our findings strongly suggest the need to take into account the quality of carbohydrate, or a ratio of fibre to carbohydrate intake, in strategies aimed at type 2 diabetes prevention in East Asian populations.
Acknowledgements
The authors extend sincere appreciation to all staff members of the Nutrition Clinic for running the programme and collecting data.
This research received no specific grant from any funding agency or commercial or not-for-profit sectors.
N. M. and Akira T. formulated the research questions and designed the study; N. M., C. K., Airi T., K. K. and Akira T. carried out the study; N. M., C. K., Airi T., K. K., M. A., K. U. and Akira T. analysed data; C. K., Airi T., K. K., M. A., K. Y. U. and Akira T. revised critically for important intellectual content; Akira T. had primary responsibility for final content. All authors read and approved the final manuscript.
The authors declare that there are no conflicts of interest.
Supplementary material
For supplementary material/s referred to in this article, please visit https://doi.org/10.1017/S0007114517003725