INTRODUCTION
Multidrug-resistant tuberculosis (MDR TB), or TB resistant to the two potent anti-mycobacterial agents isoniazid and rifampin, has become an international problem as treatment efforts have expanded over the last several decades [1]. The emergence of M. tuberculosis strains resistant to second-line drugs has been a subject of more recent concern. Extensively drug-resistant (XDR) TB, a form of MDR TB resistant to not only isoniazid and rifampin, but also any fluoroquinolone and at least one of three injectable second-line drugs, has resulted in an epidemic in the large province of KwaZulu-Natal, South Africa [2, Reference Moodley3]. Increased identification of the disease has also generated attention in a number of other countries performing surveillance studies [4]. In this review, we discuss the emergence of XDR TB. We discuss the transmission dynamics of drug-resistant TB strains in South Africa, and how HIV co-infection and transmission in enclosed spaces play key roles in propagating the disease. We then compare potential control strategies for XDR TB using mathematical models of TB transmission, which shed light on both helpful and potentially dangerous responses to the emergence of XDR TB.
The burden of MDR and XDR TB
The reported incidence of MDR TB among new TB cases is highly variable among different regions, ranging from 0% in some countries of Western Europe, to ~4% in Rwanda, to over 20% in some Eastern European states [4]. However, comparison between regions is hampered by heterogeneities in the surveillance infrastructure required to detect drug resistance. Resistant cases are generally thought of in terms of three categories of resistance development: (1) acquired resistance; (2) amplified resistance; and (3) primary resistance [1]. Incomplete suppression of bacteria through chemotherapy can lead to the development of new mutations. Acquired resistance is defined by the development of such mutations as a result of patient non-adherence to therapy [5]. Countries with very poor rates of follow-up to ensure patients complete therapy have found that over half of their TB patients that have been previously exposed to therapy have MDR TB [4]. However, when therapy is improperly delivered, patients may experience MDR TB even if they are adherent to therapy [1, Reference Farmer6]. Such amplified resistance occurs when M. tuberculosis is not suppressed, because it is already resistant to one or a few drugs in the prescribed regimen; the bacteria develops resistance to increasing numbers of drugs in the regimen because a potent combination therapy was not prescribed to prevent persistence of the bacteria and avert the opportunity for bacteria to propagate with new mutations [Reference Connolly, Edelstein and Ramakrishnan7].
Primary transmission of MDR TB (i.e. transmission of already resistant M. tuberculosis) was previously considered an isolated phenomenon compared to acquired and amplified resistance [Reference Dye and Espinal8]. Classical evolutionary theory suggested that the mutations that confer drug resistance will produce a fitness ‘cost’, thought to reduce the transmissibility of M. tuberculosis [Reference Cohen, Sommers and Murray9]. Recent epidemiological and laboratory-based studies, however, indicate that such fitness costs are highly heterogeneous, depending both upon the host and environmental contexts, as well as on the specific mutations acquired by the bacteria [Reference Cohen, Sommers and Murray9–Reference Dye12]. Compensatory mutations that confer increasing fitness have been identified among drug-resistant strains [Reference Sherman13]. Hence, while some authors have concluded that MDR TB will ‘remain a localized problem’ [Reference Dye and Espinal8], others have indicated that limited transmission cannot be assumed [Reference Cohen and Murray14]. Whether or not drug-resistant TB strains are less transmissible than drug-susceptible strains, MDR TB patients often experience prolonged infectiousness associated with delayed identification and ineffective therapy [Reference Farmer6]. These two factors will resonate in our discussion of XDR TB.
XDR TB was first defined as resistance to isoniazid and rifampin (MDR) and at least three of the six main classes of second-line anti-TB drugs (aminoglycosides, polypeptides, fluoroquinolones, thioamides, cycloserine, and para-aminosalicyclic acid) [Reference Shah15]. More recently, however, the definition was changed to correspond to more reliable drug-susceptibility tests and with poorer treatment outcomes. XDR TB is now defined as resistance to isoniazid and rifampin, as well as any fluoroquinolone and at least one of three injectable second-line anti-TB drugs (capreomycin, kanamicin, and amikacin) [16]. Under the former definition, the U.S. Centers for Disease Control and Prevention (CDC) and the World Health Organization (WHO) conducted a study of the prevalence of XDR TB among isolates collected from every region of the world through the network of supranational TB reference laboratories. Among 17 690 isolates from the period 2000–2004, 3520 were MDR isolates, of which 347 (~10% of MDR isolates) were XDR [Reference Shah15]. The prevalence of MDR TB in this sample is expected to be much higher than in populations generally as a result of sampling bias (since suspected cases are referred to supranational laboratories), but the proportion of XDR among all MDR cases indicated that XDR TB was a serious concern, and that XDR cases appeared in all regions of the world. Distinguishing amplified, acquired and primary transmission as the mechanisms of resistance was not possible, and is generally very difficult. Standard WHO surveys record whether patients are new TB patients (not previously having been exposed to therapy, a potential indicator of primary transmission) or re-treated TB patients (who may have acquired or amplified resistance from prior therapy) [4]. In the CDC/WHO XDR TB study, sufficient information was not available from which to determine how the recorded XDR TB cases arose [Reference Shah15].
The transmission dynamics of XDR TB in rural KwaZulu-Natal
In 2006, concerns about the XDR TB problem were amplified by the report of an unusually high prevalence of XDR TB in a rural area of South Africa [Reference Gandhi17, Reference Gandhi18]. The report revealed a large number of XDR TB cases in the region of Tugela Ferry, part of the KwaZulu-Natal province that has a high incidence of TB (over 1000/100 000) and a high rate of treatment default (at least 15% of patients not completing their course of treatment) [19, 20]. While the prevalence of MDR TB among new TB cases was 1·7% in a 2001 survey [Reference Weyer21], an analysis at one hospital in Tugela Ferry from January 2005 to March 2006 revealed that 39% of 475 patients with culture-confirmed TB had MDR TB and 6% had XDR TB [Reference Gandhi17, Reference Gandhi18].
Two key factors in this report were of most interest. The first was that a majority of the XDR TB patients in this cohort had never been treated for TB, and none had received the second-line drugs to which they were resistant. Primary transmission of XDR TB was suspected to play a key role in explaining the large number of cases accumulating over a brief period, potentially as a result of nosocomial (hospital-based) transmission [Reference Basu and Galvani22, Reference Basu23]. This suspicion was supported by later investigations that revealed hospitalization to be a key risk factor for XDR TB (odds ratio of 3·7 of having XDR TB if hospitalized in the past 2 years, in a case-control study of 57 drug-susceptible, 52 MDR and 61 XDR TB patients [Reference Andrews24]). As in most resource-poor settings, hospitals in this region lack TB isolation facilities; hospital wards are congregate settings where several dozen persons are housed in a single, generally over-crowded room with limited ventilation and few if any respiratory masks for patients. Among a group of patients in whom multiple sputum smears were available (n=17), all were exogenously re-infected or super-infected with XDR TB, having had a non-XDR drug-susceptibility profile initially and XDR TB after hospitalization, without being given the second-line drugs to which XDR TB was resistant [Reference Gandhi25]. This further supports the idea that nosocomial primary transmission could fuel XDR TB incidence.
In addition to concerns about primary transmission, a second factor of concern was that all of the XDR TB patients who were tested for HIV were found to be HIV-positive, having a very low median CD4 cell count of 63 cells/mm3 (indicating severely immunocompromised). The survival rate among these patients was abysmal, as they survived a median time of only 16 days from the time of sputum collection, and 70% died within 30 days from that time [Reference Gandhi18]. The report from Tugela Ferry reflected a collision of TB and HIV epidemics. HIV co-infection accelerates the progression of TB, increasing the likelihood of active disease after infection [Reference Havlir and Barnes26]. Patients co-infected with HIV also experience a higher risk of exogenous TB re-infection and relapse after TB therapy [Reference Havlir and Barnes26]. Thirty-nine percent of antenatal clinic patients test positive for HIV in the KwaZulu-Natal province, but antiretroviral therapy – which appears to significantly reduce the risk for active TB – has not been received by many (~7%) [27, 28]. While some scientists have hypothesized that immunosuppression associated with HIV could allow for less-fit drug-resistant strains to emerge and propagate widely [Reference Dye12], whether HIV is truly an independent risk factor for MDR TB has been debated as a result of conflicting data [Reference Wells29].
Regardless of the fitness debate, it is not a novel phenomenon to observe primary transmission (particularly nosocomial transmission) of drug-resistant TB among HIV-infected patients. Indeed, the high prevalence of XDR TB in KwaZulu-Natal reflects a large and disastrous manifestation of an all-too-familiar phenomenon that has occurred in many countries over the past few decades. MDR TB outbreaks have been witnessed in hospitals and prisons on all continents. The total number of cases in those outbreaks ranged from a few dozen to over 100, and more than 90% of MDR infected persons were HIV-infected in all cases, with median survival times ranging from 4 to 16 weeks [Reference Narvskaya30–Reference Fischl42]. Therefore, the events in Tugela Ferry can be seen to represent a larger and more severe manifestation of a familiar phenomenon. Since the initial Tugela Ferry report, over 50 independent health facilities have reported more than 600 cases of XDR TB from around the KwaZulu-Natal province [Reference Moodley3].
While initial reports suggested that nearly all XDR TB cases were among persons with HIV or among health-care workers, increasing numbers of patients who are confirmed HIV-negative and had no prior health system exposure are being found (including children), suggesting that an initially nosocomial outbreak may be expanding into a larger community-level epidemic [Reference Shah43]. HIV-infected patients, having the shortest incubation periods after infection, may appear first as ‘canaries in the mine shaft’ for a larger epidemic [Reference Gandhi25]. XDR TB has also been reported in every other province of South Africa [44]. In KwaZulu-Natal, a common KZN strain (F15/LAM4/KZN) has been identified in the majority of cases [Reference Pillay and Sturm45]. The strain emerged in an XDR form since at least 2001, as a strategy to avoid drug-susceptibility testing and standardize therapy for all MDR TB cases was adopted (to simplify therapy delivery) [Reference Pillay and Sturm45]. It has been argued that this strategy probably amplified XDR TB resistance, since patients with resistance to one or a few drugs experienced amplified resistance while being unknowingly treated with inadequate drug regimens [Reference Sturm46]. In other words, acquired and amplified resistance may have generated the strain that was then spread further by primary transmission.
Limiting XDR TB transmission
Among the potential responses to the XDR TB problem in KwaZulu-Natal, the proposal to institute quarantine has been a subject of major discussion and debate [Reference Singh, Upshur and Padayatchi47]. Indeed, quarantine has been a central topic of media reports regarding XDR TB since the Tugela Ferry report [48, Reference Dugger49]. However, one must proceed with caution when considering the best manner to reduce the transmission of XDR TB. Infection control studies suggest that isolation is highly effective, but these studies have assumed individual isolation of patients, not merely the removal of patients from the community setting [Reference Nardell and Friedman50]. Two conflicting sets of proposals have emerged in South Africa as a result of the debate concerning quarantine, and the discussion around nosocomial transmission; each proposal relies on different interpretations about the transmission dynamics of the disease and the operational constraints involved in controlling XDR TB.
On one hand, proponents of a quarantine or mass isolation approach have suggested that detaining XDR TB suspects in central facilities could reduce community-based transmission and maintain TB treatment in the hands of treatment specialists [51]. Group hospitalization for long periods of time has been the dominant approach for the treatment of MDR and XDR TB patients to date. A number of infection control measures have been proposed to reduce potential nosocomial transmission of XDR TB [Reference Bock52].
Many concerns have been raised about group quarantine, however. Most patients are infected for weeks or months, and experience the largest part of their infectious periods in the community [Reference Corbett53]; hence, quarantine is unlikely to sufficiently curtail transmission. Furthermore, drug-susceptibility testing takes at least a month to return results to the testing site from central laboratories, and most ‘suspects’ (patients having previously defaulted from therapy, or having persistent symptoms or worsening chest X-ray on a standard TB treatment course [54]) do not have XDR TB, but will be susceptible to super-infection with XDR TB in congregate wards. It is unclear how much impact can be realistically achieved through hospital-based infection control measures alone, particularly in wards where several dozen patients share common rooms. Super-infection with XDR TB has been observed to be a major source of new XDR TB infections in Tugela Ferry [Reference Gandhi25]. Patients who are quarantined as suspected MDR or XDR TB patients are then super-infected with XDR TB while awaiting their drug-susceptibility results. The quarantine approach, in other words, appears to have backfired as a result of slow diagnosis and nosocomial transmission in congregate wards. Since partial immunity from prior infections appears to be weakened during active disease [Reference Behr55], and may in part be strain-specific [Reference Lopez56–Reference Manca59], the risk of super-infection is very high. Finally, patient refusal to accept long-term hospitalization in poor conditions (hot, stuffy containment units far from home) has resulted in default and ‘escape’ from quarantine [48]. One patient was shot by hospital security guards during a protest against hospital conditions [54], reflecting a severe communication breakdown between public health authorities and patients, an antagonistic rather than cooperative approach to public health, and violations of basic human rights and patient rights.
As an alternative strategy, it may be possible to harness the advantages of isolation facilities through a patient-centred approach that limits the risk of nosocomial transmission and reduces patient non-adherence or default from therapy. We can arrive at such a strategy by viewing the key operational barriers to effective patient treatment in resource-limited settings. In South Africa, most TB patients live in poverty, and the lost wages and transportation expenses required to visit centralized health facilities (often very far from work or home) are a significant barrier to prompt diagnosis [60]. After being infectious for prolonged periods, TB patients will experience worsened symptoms and finally appear to health facilities. If properly diagnosed with TB (which is often more difficult to diagnose in HIV-infected patients, as a result of uncommon disease manifestations [Reference Havlir and Barnes26]), patients usually do not receive drug-susceptibility testing. Rather, given the large volume of TB patients appearing at most health facilities, drug-susceptibility testing is reserved for patients who have persistent symptoms after a standardized first-line TB treatment regimen. Therefore, patients experiencing primary MDR and XDR TB will be given ineffective standard TB treatment [Reference Farmer6].
If MDR and XDR TB patients survive the ineffective treatment course (which takes several months), they will usually continue to experience TB symptoms. Many clinicians in South Africa will add one drug to the regimen (an outdated ‘re-treatment’ approach of adding an extra drug to a failing regimen, which was once believed to improve outcomes); an abundance of evidence now suggests that this is an ineffective approach, although many countries still adopt it as a cost-saving measure to limit the use of drug-susceptibility testing and second-line drugs [Reference Espinal61, Reference Espinal62]. As opposed to individually tailoring a new regimen based on drug-susceptibility test results, the re-treatment strategy potentially amplifies resistance through inadequate bacterial suppression [1]. The four-drug-resistant XDR TB strain in Tugela Ferry has amplified into a predominantly six-drug-resistant strain over the course of just 2 years as a result of the re-treatment strategy [Reference Moll63]. If MDR and XDR TB patients are alive and able to complete the several months of ineffective therapy, clinicians may finally perform drug-susceptibility testing if the patients return to the clinic. Results of testing will be received after another month or longer, and meanwhile patients will be told to travel to distant (usually urban) specialized hospitals for group quarantine. Every stage in this process contributes to the mortality of patients and to non-adherence to prolonged (usually ineffective) therapy with significant side-effects and personal expense to the patient.
The operational barriers to effective care reveal an alternative structure for TB treatment delivery. In many resource-limited settings, care has been delivered effectively through a more decentralized approach [Reference Mitnick64]. Contacts of MDR or XDR TB patients are actively pursued in the community and tested with rapid drug-susceptibility tests, then administered therapy in the community near their homes. Trailers or park homes can be used as readily available but relatively inexpensive and hospitable individual isolation facilities for the period during which patients are infectious [Reference Basu65]. Home-care nurses deliver therapy close to or in patient homes, and if appropriate therapy is delivered, such patients can rapidly become non-infectious [Reference Iseman66]. While some patients will be too ill to receive therapy at home, many community-based programmes have instituted therapy by housing patients in well-ventilated facilities separated from other family members but close to home [54]. Since trailers may be limited in availability in settings with many MDR or XDR TB patients, a few programmes have built separate rooms near the patients home, while training household members in appropriate mask use and infection control [54]. This increases retention (by permitting masked visitation with family members) and reduces default from therapy, while being relatively inexpensive in comparison to hospitalization in tertiary care facilities [54]. While family members may in theory be exposed to XDR TB, the community-based approach would still reduce their exposure time since this therapy would result in rapid suppression of bacterial loads among treated patients, and the current hospital-based method results in delayed diagnosis and long waiting times to therapy that permit prolonged infectiousness.
While the community-based, decentralized approach would seem to be an improvement in theory, it must be rigorously evaluated. Some physicians have argued against the approach, suggesting that it risks amplifying community-based transmission in an effort to prevent hospitalization and reduce the risk of nosocomial exposures [51]. On the other hand, incarceration of patients rather than community-based detection and treatment may frustrate case detection, as people may avoid health centres and thereby remain infectious in the community rather than seeking treatment. Therefore we need to compare these approaches directly to one another. What can we expect from these alternative proposals for controlling XDR TB? What strategies are most likely to improve outcomes while minimizing perverse amplification of XDR TB by the health system?
Simulating control strategies
Because direct comparison of alternative treatment approaches is difficult and sometimes unethical in human populations, mathematical models have been used to understand the transmission dynamics of drug-resistant TB and the consequences of adopting alternative strategies to control the disease. We can address several questions related to XDR TB transmission in South Africa using mathematical models. First, we can evaluate the transmission dynamics of disease in hospital ward settings [Reference Basu65]. This can reveal how likely it is for patients to be infected with XDR TB given typical conditions in South African hospital wards, and how infection control strategies may impact upon the risk of infection. Next, we can evaluate the dynamics of an XDR TB epidemic, and examine how likely nosocomial epidemics are to spread into the community. Finally, we can compare the group quarantine and decentralized community-based approaches to controlling the disease, in order to examine what approaches might best reduce future XDR TB incidence while minimizing potentially perverse effects of health-care delivery strategies.
The transmission dynamics of infection in enclosed settings such as hospital wards has been commonly evaluated using the Wells–Riley equation, a model of airborne transmission [Reference Wells67, Reference Riley, Murphy and Riley68]. We can derive this equation, and a more extensive dynamic version of it, to examine XDR TB control. The Wells–Riley model is derived by defining a ‘quanta’ as a unit of airborne droplet nuclei. Suppose an individual's pulmonary ventilation rate is κ and the production of M. tuberculosis quanta for each of I infectious persons in the room is q per unit time. If the room has volume V and ventilation rate A, then the number of quanta inhaled by a susceptible individual will be the concentration of quanta in the air (qI/VA) multiplied by the volume of air inhaled by the person over the time period t(κt). Poisson's law of small numbers (the probability of a number of occurrences of a rare event with many opportunities) can be used to define the expected number of new infections in this situation. The chances of escaping an infection, using the Poisson approximation, are e−(qIκt/VA). Hence, the probability of infection is 1 minus this quantity, and the number of new infections in a room with S susceptible persons can be estimated as S(1−e−qIκt/VA), known as the Wells–Riley equation [Reference Riley, Murphy and Riley68].
In the years since its proposal, the Wells–Riley model has provided a number of critical insights. Perhaps most importantly, it has allowed estimates of the potential impact of different degrees of infectiousness among TB patients and different infection control interventions. Estimates of the number of infectious quanta produced by TB patients [Reference Escombe69–Reference Riley and Nardell72], typically through experiments in which guinea pigs are infected in hospital wards fitted with animal cages, have revealed that the infectiousness of patients can be highly varied in different settings and among human hosts [Reference Escombe69] (Fig. 1). Some persons are far more infectious than others; inadequate treatment, having acid-fast bacilli on a sputum smear, experiencing laryngeal TB, or having an active cough are all related to higher degrees of infectiousness [Reference Iseman66, Reference Escombe69, Reference Kawai73]. Inadequate therapy also prolongs the duration of infectiousness [Reference Iseman66, Reference Kawai73]. The Wells–Riley model provides insights into what the risks of XDR TB infection can be among South Africans hospitalized in congregate TB wards. As shown in Figure 1, the risk of infection is quite high upon sustained exposure to infectious patients. In typical South African hospitals, the duration of in-patient admission and potential exposure to XDR TB-infected patients on congregate wards is ~22 days [Reference Andrews24], and the Wells–Riley model suggests that the risk of nosocomial transmission is probably extremely high even across a variety of infectious quanta rates potentially produced by XDR TB patients.

Fig. 1. Results of the Wells–Riley model, showing the proportion of susceptibles infected by a single infectious TB case over different durations of exposure. Note that in the context of XDR TB epidemic in South Africa, average hospital admission times in congregate wards were ~22 days [Reference Andrews24]. Three simulations are shown, using common parameter values (κ=0·48 m3/h [Reference Noakes75]; VA=402 m3/h, an average among mechanically ventilated rooms [Reference Escombe78]), where the number of infectious quanta is varied from the Riley's estimate among HIV-negative TB in-patients (q=1·3, [Reference Riley70]) to Escombe's estimate among HIV-positive TB in-patients (q=8·2 [Reference Escombe69]) to Nardell's estimate for an untreated TB patient (q=13 [Reference Nardell71]).
As with all models, these simulations must be viewed as approximations in the context of the model's assumptions. The Wells–Riley model assumes steady-state levels of infectious quanta in a room (complete air mixing). A recent modification of this model to describe non-steady-state conditions was proposed by Gammaitoni & Nucci [Reference Gammaitoni and Nucci74], who described both the rate of change of total quanta in a space (Q) and the rate of change of the number of susceptible persons in the ward over time, using the following differential equations:


This model reduces to the Wells–Riley equation in the steady-state case. We can modify the model through a series of simple algebraic manipulations (see Appendix) to suit a general framework for simulating nosocomial epidemics [Reference Noakes75], and for simulating the course of epidemics where both nosocomial and community-based transmission occur [Reference Basu65]. As shown in Figure 2, the model reveals that an initially nosocomial outbreak can lead to eventual community-based transmission, even when the transmission fitness of XDR TB strains is very low in comparison to drug-susceptible strains. Nosocomial outbreaks may not, in other words, remain confined to hospital wards. The movement of infected persons into and out of TB wards leads to community-based transmission. But a key question is whether a group quarantine approach rather than community-based detection and treatment would be sufficient to curtail this occurrence.

Fig. 2. Results of a simple simulation model of XDR TB, incorporating both community and nosocomial transmission (see Appendix). In this simulation, an initially nosocomial outbreak can lead to eventual community-based transmission, even though this model assumes the transmission fitness of the XDR TB strain is only 35% that of drug-susceptible strains (an average among MDR TB strains [Reference Dye and Espinal8]). The parameters in the simulation are: κ=0·48 m3/h [Reference Noakes75]; VA=402 m3/h (78); q=1·3 [Reference Riley70]; 20% HIV prevalence [27], and 40 in-patient beds [Reference Basu65], and the resulting cumulative incidence curve is of similar rate and magnitude to the observed XDR TB epidemic in KwaZulu-Natal [Reference Vella89].
To answer this question, we must incorporate the capacity of group quarantine facilities into the model, and also include the likely impact of infection control interventions that are currently being implemented in congregate settings. As discussed further in the Appendix, the operations research approach known as ‘queuing theory’ can be incorporated into epidemic models to evaluate how resource-constrained systems deal with high demand [Reference Kleinrock76]. Queuing systems are sets of equations that keep track of how people move among different environments in the context of limited capacity (e.g. how many people queue for a particular service); in the context of epidemics, queuing effectively occurs for limited hospital beds or by placing demand on limited health-care staff. Within the hospital ward, a number of different administrative, environmental and personal protective infection control measures can also be instituted to simulate improvements in congregate wards [Reference Basu65]. These include respiratory masks, improvements in ventilation, and rapid identification and isolation of XDR TB suspects. However, if we simulate the infection control interventions and group quarantine strategy proposed by the South African government at present (Fig. 3) [51], we see that perversity could occur because the risks of nosocomial transmission in expanded congregate wards remains very high in light of the demand placed on hospitals and the number of persons that are admitted to congregate settings. In contrast, community-based care decreases XDR TB incidence in this model, as a result of rapid diagnosis and reduction in infectious periods with effective therapy, outweighing the potential perversity of increased community-based incidence.

Fig. 3. The risk of nosocomial super-infection by XDR TB can outweigh the benefit of reduced community-based transmission when sufficient numbers of people are quarantined together on multi-person (congregate) TB wards. Most South African health facilities lack individual isolation units, hence quarantine is enforced in congregate settings. The degree to which such perversity will occur will depend on the congregate ward conditions and community contact rates, which are generally difficult to estimate. In this simulation using baseline parameters from KwaZulu-Natal, South Africa (see Appendix; which uses the same parameters as Fig. 2), not allowing suspects to be discharged from congregate in-patient wards and increasing ward capacity (employing the parameters described in a recent South African government strategy document [51]) increased the incidence of XDR TB. Providing community-based therapy (with the parameters specified in Mitnick et al. [Reference Mitnick64]) reduced the incidence of XDR TB, as slight increases to community-based risk were outweighed by large decreases in nosocomial risk.
Infection control measures must be instituted in congregate wards, but to be effective, infection control should consider radically altering the congregate ward system rather than simply implementing one or a few infection control measures. Comprehensive infection control packages have been described in detail elsewhere [Reference Nardell and Friedman50, Reference Bock52, Reference Jensen77]; the common theme in the infection control literature is that no single intervention can be expected to completely eliminate the risk of TB transmission in enclosed spaces. Natural ventilation, for example, may achieve improved air circulation levels (e.g. through opening windows and installing mesh screens in the bottoms of doors), while mechanical ventilation systems are often poorly maintained [Reference Escombe78]. However, patients may not benefit from natural ventilation when wind speeds are low or when windows are closed during cold winter months; hence mechanical systems may be necessary in many settings, and would ideally be implemented in the form of individual negative-pressure rooms, which will require time to construct (while community-based therapy may be more rapidly implemented). Similarly, personal respirator use is subject to adherence and face-seal leakage [Reference Lee, Slavcev and Nicas79], and little data indicate how long a single respirator can be used effectively, which is of relevance in settings with constrained budgets and health-care personnel who work in hot environments. Cough hygiene (having patients cover their mouths, or spit outdoors when sputum is required for testing) remains a difficult intervention to empirically evaluate, but may also reduce nosocomial transmission [Reference Gandhi25]. High-efficiency particulate air (HEPA) filters offer another form of ventilation-based control, but require substantial air movement [Reference Nardell and Friedman50]. On the other hand, ultraviolet germicidal irradiation (UVGI) has shown considerable efficacy in a number of experiments, but is often avoided due to concerns (often misconceptions) about safety and utility [Reference Nardell and Friedman50]. While the details of these various infection control options are beyond the scope of this review, the implications for XDR TB control operations are that a complete infection control programme is more appropriate to implement that a single intervention. Individual facilities should consult an infection control engineer to design and facilitate an infection control package. Facilities facing the resource constraints of poverty are often those that are most likely to have problems accessing this expertise and controlling nosocomial transmission, and while simple measures may be possible to implement at even very resource-poor facilities, the prospect of community-based detection and treatment remains crucial to consider. Previous studies suggest that even comprehensive infection control packages are insufficient to prevent XDR TB transmission in the context of delayed identification and poor delivery of effective treatment regimens [Reference Basu65].
CONCLUSIONS
Excessive reliance on the hospital, and crowding of HIV-infected persons in congregate settings such as hospital wards or antiretroviral treatment clinics, may lead to conditions that can fuel nosocomial transmission and justify the idea of increasing case detection and treatment in the community. The numerous MDR TB outbreaks in nosocomial settings of resource-wealthy nations suggest that infection control efforts are not merely a matter of resource availability, but also of detailed and thorough implementation that often requires significant political will to institute [Reference Tokars80, Reference Moro81]. On the other hand, it will require extensive and careful training to allow community health workers to provide complex therapies in the community environment, and will require significant resources to build sufficient isolation facilities and monitor patients so that they can receive appropriate care (including in-patient care when necessary) [Reference Mitnick64, Reference Rich82].
Our conclusions are, however, tempered by a number of caveats. Our knowledge of XDR TB remains preliminary. Surveillance is inherently biased towards health-care facilities, hence nosocomial transmission may be over-emphasized at sites with limited community-based surveillance infrastructure. Community-based transmission can be particularly important in the case in South Africa, where the mining industry fuels seasonal migration among provinces and across borders [Reference Posel and Casal83]. Migration is believed to be significant to the spread of HIV and TB in South Africa [Reference Crush84, Reference Williams, Banks and Parker85]. Indeed, the incidence of TB has been reported to be higher than 3000/100 000 at some mines [Reference Anglogold86], and the attendant risk of silicosis increases the risk of active TB among this group [Reference Williams, Banks and Parker85]. Even outside of mines, extensive use of kombis (small mini-buses where many people are packed together for extended travel and daily commutes) are also sites for concern; these mini-taxis form the basis of public transport in many poor areas of the world, and are often colloquially referred to as ‘TB taxis’ in which people sit in close proximity to one another on a daily basis. The importance of migration and transmission along migration routes cannot be understated when evaluating the potential for regional spread of XDR TB. Migration is likely to have played a role in XDR TB emergence in Tugela Ferry. The hospital in Tugela Ferry where XDR TB was identified does not have access to the second-line drugs to which the observed XDR TB patients were resistant; therefore, initial cases probably migrated from other areas. Whether migrant or not, transportation of patients to clinics or the use of mobile health-care delivery teams to bring effective care to rural and poor patients will be an important challenge to address. Determining the optimal strategies for these treatment approaches will be an important subject of future research.
The realities of TB treatment operations reveal that the traditional separation of acquired and amplified resistance from primary transmission is indeed a false dichotomy. All of these types of resistance are important, and result from the same limitations in health system capacity. Acquired and amplified resistance can generate the resistance that is later transmitted nosocomially or in other enclosed environments such as schools, homes, or public transportation vehicles. Acquired and amplified resistance result from poor follow-up and limited use of drug-susceptibility testing and pharmacological treatments – a sign of both poor or limited training and inadequate systems to deliver effective treatment. To be truly ‘directly observed’, therapeutic efforts must address the problem of patients lost to follow-up, who often require extensive treatment support and consideration for their economic burdens (e.g. through transportation vouchers, or home-based care). The problem of inadequate health systems is also manifested by primary transmission of XDR TB in facilities that contribute to nosocomial transmission, and where infection control strategies are not thoroughly implemented. Fortunately, a number of recent advances have led to the broader availability of effective therapies for MDR and XDR TB in resource-limited settings [Reference Gupta87], and to the invention of diagnostic techniques that more rapidly detect drug-susceptibility profiles in patients in resource-limited settings [Reference Moore88]. Implementing both detection and treatment measures in these settings remains a key challenge, and will require a close consideration of the operational burdens of disease on the health system, and the burdens placed upon patients expected to complete therapy.
APPENDIX
The Gammaitoni & Nucci model [Reference Gammaitoni and Nucci74] has been previously transformed by Noakes et al. [Reference Noakes75] into a SIR (susceptible–infected–recovered) compartmental form used typically for modeling infectious disease epidemics.
Using the equations from the main text, if we assume a steady-state ventilation rate and continuous quanta production from infectious persons (dQ/dt=0), then Q=qI/A. The rate of infection is then dS/dt=−(κq/VA)IS. The quantity (κq/VA)I is typically referred to as the force of infection (λ) [Reference Basu65, Reference Anderson and May90], where I is the proportion of total infected persons with active TB (T) who are infectious (proportion f of T).
If we were to use a standard simple model of TB pathogenesis based on prior models [Reference Blower91], then we could describe the typical epidemic behavior of TB using just a few equations:



where In this simplistic formulation (Fig. A1), Π births occur per unit time and the background (non-TB) mortality rate is μ while the TB mortality rate is μT. A portion of susceptibles S(t) are infected at rate λ from which a portion p undergo primary progressive disease [rapid progression to active disease T(t)] while (1−p) achieve latency [L(t)]. The rate of reactivation from latency is v, but a portion x are susceptible to primary progression upon exogenous re-infection [rate λ(t)]. Active TB patients may be detected (rate d) and a portion k are cured, while natural self-cure can occur at rate n. We use the typical WHO rubric of defining cure as regression to latency [Reference Dye92], from which reactivation and re-infection can occur. Typical parameter values are described in Table A1. Note the time dependence indicator (t) is removed from the above equations to improve legibility.

Fig. A1. Flow diagram for the simple model of TB transmission.
Table A1. Typical parameter values used to describe TB pathogenesis
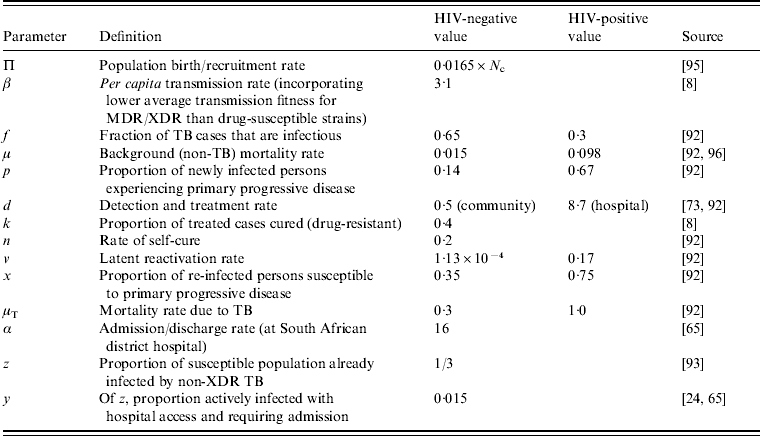
The model described here can be subcategorized into HIV-specific groups [Reference Basu65], or one can describe the average population dynamics by describing the HIV prevalence in the simulated community and taking the weighted average of the HIV-negative and HIV-positive parameters (main text). (All rates are in units of 1/year.)
Suppose we were to use this formulation to simulate a XDR TB epidemic. A few complexities should be added to generate a simple model of nosocomial and community-based transmission of XDR TB. The above model does not account for a portion z of people susceptible to XDR TB but infected with other strains, who may harbour partial immunity to new infections. In high-prevalence regions, and certainly on TB hospital wards, a large portion of the population may have non-XDR TB disease. Among the group z, a portion x are susceptible if we use the typical assumption that partial immunity is strain-independent (an optimistic case). Therefore, the presence of other disease can be resolved by multiplying the rate of infection λ by (1−z+zx)S [Reference Dye and Williams93].
Furthermore, if we used the above formulation to simulate a XDR TB epidemic, then we fail to account for the fact that transmission can occur either in the hospital or in the community. The above λ describes nosocomial transmission, but people who become infected in the community (at contact rate β) can enter the hospital as a result of the symptoms experienced in the community for either non-XDR or XDR TB. They may also become nosocomially infected and then be discharged from the hospital before experiencing active TB. This can be resolved by using one set of equations to describe each of two environments (community and hospital), and adding a queuing system to connect the two environments. A queuing system is a simple expression that keeps track of movement between the two settings based on the availability of beds in the hospital [Reference Basu65, Reference Kaplan, Craft and Wein94]. Suppose we have a facility with b beds and T admitted patients. Then b−T is the number of available beds, and if the typical length of stay is 1/α, then α is the rate of admission/discharge to/from those beds. If a total of D persons with active disease are available to be admitted at a given time, then the number admitted will be α∗min(D, b−T). For a subgroup D i among D, admittance will be α∗min(D i, D i∗(b−T)/D)=α∗D i∗min(1, (b−T)/D), since either the hospital has capacity to admit all such persons, or the relevant fraction of persons for whom beds are available will be admitted.
Using this simple formulation, we can arrive at a basic model of XDR TB epidemics that incorporates the potential for both community-based and nosocomial transmission, where the subscripts c or h refer to populations in the community [population size N c(t)] or hospital [population size N h(t)], respectively:






where



DECLARATION OF INTEREST
None.