Introduction
One of the main goals in ecology is to understand how environmental factors structure animal communities (Guilherme et al. Reference Guilherme, Souza, Franklin, Pequeno, Chagas and Baccaro2019, McGill et al. Reference McGill, Enquist, Weiher and Westoby2006), and researchers have long studied the mechanisms involved (Grinnell Reference Grinnell1917, Levin Reference Levin1992, Sobral & Cianciaruso Reference Sobral and Cianciaruso2016). Niche partitioning, interspecific competition and environmental filtering through the selection of morphological, physiological and behavioural characteristics suitable for species permanence in a given environment (Keddy Reference Keddy1992) play important roles in the distribution of species in a habitat, generating differences in taxonomic, functional and phylogenetic diversity at different spatial and temporal scales (Baccaro et al. Reference Baccaro, Rocha, del Aguila, Schietti, Emilio, Pinto, Lima and Magnusson2013, Carvalho et al. Reference Carvalho, Mustin, Farneda, Castro, Hilário, Martins, Miguel, Xavier and Toledo2021, Guilherme et al. Reference Guilherme, Souza, Franklin, Pequeno, Chagas and Baccaro2019, Poff Reference Poff1997).
The relevance of different environmental characteristics, such as vegetation structure, food availability, terrain elevation and distance to the nearest body of water, has been demonstrated for amphibians and reptiles (Atauri & Lucio Reference Atauri and Lucio2001, Fraga et al. Reference Fraga, Lima and Magnusson2011), birds (Bueno et al. Reference Bueno, Bruno, Pimentel, Sanaiotti and Magnusson2012, Poulsen Reference Poulsen2002), terrestrial mammals (Lees & Peres Reference Lees and Peres2008, Williams et al. Reference Williams, Marsh and Winter2002) and bats (Marciente et al. Reference Marciente, Bobrowiec and Magnusson2015, Pereira et al. Reference Pereira, Capavede, Tavares, Magnusson, Bobrowiec and Baccaro2019, Ramos Pereira et al. Reference Ramos Pereira, Marques, Santana, Santos, Valsecchi, Queiroz, Beja and Palmeirim2009). Bats make a good model for understanding how environmental changes along a gradient affect the distribution of species within a habitat because they have a high number of species, well-known food guilds (López-Baucells et al. Reference López-Baucells, Rocha, Bobrowiec, Bernard, Palmerim and Meyer2016, Reis et al. Reference Reis, Peracchi, Batista, de Lima and Pereira2017), and are very sensitive to environmental variations (Capaverde et al. Reference Capaverde, Pereira, Tavares, Magnusson, Baccaro and Bobrowiec2018, Carvalho et al. Reference Carvalho, Mustin, Farneda, Castro, Hilário, Martins, Miguel, Xavier and Toledo2021).
New World bat assemblages are widely distributed and functionally and taxonomically diverse, increasingly so towards the equator (Bogoni et al. Reference Bogoni, Carvalho-Rocha, Ferraz and Peres2021, Kaufman Reference Kaufman1995, Stevens et al. Reference Stevens, Cox, Strauss and Willig2003). In the tropics, more ecological niches have contributed to diversification of bats into various functional groups, from just one food guild (aerial insectivores) at high latitudes to five (i.e., frugivores, insectivores, nectarivores, carnivores and sanguivores; Kalko et al. Reference Kalko, Handley, Handley, Cody and Smallwood1996a, Stevens & Willig Reference Stevens and Willig2000) closer to the equator. This pattern can be largely explained by the broad ecological and phenotypic radiation of Phyllostomid bats (Monteiro & Nogueira Reference Monteiro and Nogueira2011), the most diverse family in terms of feeding guilds (Schnitzler & Kalko Reference Schnitzler, Kalko, Kunz and Racey1998). However, because of their trophic strategies and physiological and morphological features such as precise thermoregulation and relatively large body size, phyllostomids are limited to lower latitudes (McNab Reference McNab1969). Thus, such features as trophic strategy, physiology and morphology define specific limits for diversity in New World bats, resulting in family-specific latitudinal gradients (Stevens & Willig Reference Stevens and Willig2000).
In the Tropics, bats have a greater diversity of species at the level of local assemblages than any other mammal group (Patterson et al. Reference Patterson, Pacheco and Solari1996, Tavares et al. Reference Tavares, Nobre, Palmuti, Nogueira, Gomes, Marcos, Silva, Farias and Bobrowiec2017). In the Amazon alone, one of the regions with highest diversity of bats in the world (López-Baucells et al. Reference López-Baucells, Rocha, Bobrowiec, Bernard, Palmerim and Meyer2016), approximately 90 species can coexist in a single 25 km2 site (Delgado-Jaramillo et al. Reference Delgado-Jaramillo, Aguiar, Machado and Bernard2020). This diversity of bat species is due to the diversity of microhabitats (e.g., height and foliage structure) found in the Amazon rainforest (Estrada-Villegas et al. Reference Estrada-Villegas, McGill and Kalko2012). Bats, as the only mammals with real flight capability (Norberg & Rayner Reference Norberg and Rayner1987), have the potential to exploit resources in all forest strata. However, the structural complexity of the forest demands functional characteristics from bat species that allow them to use these plant strata (Marinello & Bernard Reference Marinello and Bernard2014). For example, bats that have wings with a large surface area and rounded tips are more adapted to the lower strata of the forest, as such characteristics favour shorter flights and greater maneuverability, allowing greater agility to dodge obstacles in the most obstructed vegetation of the understory (Marinello & Bernard Reference Marinello and Bernard2014). On the other hand, large bats tend to be less agile in more obstructed spaces such as the understorey; however, their long and narrow wings allow for faster and longer distance flights to forage in the forest canopy (Marinello & Bernard Reference Marinello and Bernard2014). As such, the vegetation structure of the forest provides different microhabitats and leads to different uses of the space by different species and bat guilds.
In the Amazon rainforest, terrain elevation and vegetation structure are already well documented as predictors of bat community structure (Bobrowiec & Tavares Reference Bobrowiec and Tavares2017, Carvalho et al. Reference Carvalho, Gomes, Castro, Martins, Esbérard and Mustin2018, Marques et al. Reference Marques, Ramos Pereira and Palmeirim2012, Marciente et al. Reference Marciente, Bobrowiec and Magnusson2015, Silva Reference Silva2012). However, the studies showing these links have been carried out comparing very different habitat types: terra firme versus floodplain (Bobrowiec et al. Reference Bobrowiec, Rosa, Gazarini and Haugaasen2014), terra firme versus savannas versus campinaranas (Carvalho et al. Reference Carvalho, Gomes, Castro, Martins, Esbérard and Mustin2018) and terra firme versus floodplain versus igapó (Ramos Pereira et al. Reference Ramos Pereira, Marques and Palmeirim2010). As such, observed changes in species composition are abrupt due to more pronounced differences in the terrain elevation and the vegetation composition and structure between habitats. However, it is not well known how bat, or indeed animal assemblages, responds to finer changes in environmental conditions within each habitat (Capaverde et al. Reference Capaverde, Pereira, Tavares, Magnusson, Baccaro and Bobrowiec2018, Tews et al. Reference Tews, Brose, Grimm, Tielbörger, Wichmann, Schwager and Jeltsch2003). It is possible that more subtle variations of the same vegetation type can also influence the diversity and composition of bats at a local scale (Capaverde et al. Reference Capaverde, Pereira, Tavares, Magnusson, Baccaro and Bobrowiec2018, Estrada-Villegas et al. Reference Estrada-Villegas, McGill and Kalko2012, Ober & Hayes Reference Ober and Hayes2008). Furthermore, there is a spatial bias in studies carried out on the bat fauna of the Amazon, with most located along the Amazon River channel in areas of easy access, close to large urban centers such as Manaus, Belém and Santarém (Delgado-Jaramillo et al. Reference Delgado-Jaramillo, Aguiar, Machado and Bernard2020). Finally, there is also a clear knowledge gap regarding the natural and evolutionary history and biotic and abiotic interactions of bat fauna in the Amazon (Crane et al. Reference Crane, Silva, Matthew and George2022, Hortal, et al. Reference Hortal, Bello, Diniz-Filho, Lewinsohn, Lobo and Ladle2015), with few studies analyzing the influence of vegetation structure, terrain elevation and proximity to water bodies on aspects of the richness and taxonomic composition of bat assemblages (e.g., Capaverde et al. Reference Capaverde, Pereira, Tavares, Magnusson, Baccaro and Bobrowiec2018, Marciente et al. Reference Marciente, Bobrowiec and Magnusson2015, Pereira et al. Reference Pereira, Capavede, Tavares, Magnusson, Bobrowiec and Baccaro2019).
To date, no study has simultaneously assessed the taxonomic, functional and phylogenetic dimensions of bat diversity in a continuous forest gradient in the Amazon. Multidimensional approaches that incorporate species diversity, functional traits and phylogenetic trees can increase the detectability of ecological patterns (McGill et al. Reference McGill, Enquist, Weiher and Westoby2006) because the different components of diversity can capture different variations in the community. The mechanisms that give rise to variations in species richness may not be the same ones responsible for the variation in functional and phylogenetic characteristics (Cisneros et al. Reference Cisneros, Burgio, Dreiss, Klingbeil, Patterson, Presley and Willig2014). For example, Blanchet et al. (Reference Blanchet, Helmus, Brosse and Grenouillet2014) demonstrated that the mean annual water temperature and the distance of the river’s upstream–downstream gradient negatively affected the phylogenetic diversity of fish, while these same predictors positively affected species richness. Graça et al. (Reference Graça, Morais, Franklin, Pequeno, Souza and Bueno2016) demonstrated that the relationship between the diversity of tropical butterflies and birds was evident when assessing the variation in butterfly body size (functional component), but when analyzing species composition (taxonomic component), there was no relationship. Therefore, the use of functional and phylogenetic approaches, together with the taxonomic approach, provide more robust information on the mechanisms that determine the variation in diversity along environmental gradients (Freitas & Mantovani Reference Freitas and Mantovani2017, Tucker et al. Reference Tucker, Cadotte, Carvalho, Davies, Ferrier, Fritz, Grenyer, Helmus, Jin, Mooers, Pavoine, Purschke, Redding, Rosauer, Winter and Mazel2017).
In this study, we aim to distinguish how vegetation structure (vegetation clutter, canopy height and basal area), terrain elevation and distance to the nearest watercourse affect the three dimensions (taxonomic, functional and phylogenetic) of α-diversity (richness and Simpson’s diversity) and the predominance of some functional traits of the bat assemblage (phyllostomid and mormoopid bats) in a protected area terra firme forest area in the northeastern Brazilian Amazon. We expect vegetation clutter to be a physical barrier at the understory level for bats (Marciente et al. Reference Marciente, Bobrowiec and Magnusson2015), negatively affecting all dimensions of α-diversity. Basal area is an indicator of perches, shelter and food availability (Aguirre et al. Reference Aguirre, Lens and Matthysen2003, Carbone et al. Reference Carbone, Czimczik, Keenan, Murakami, Pederson, Schaberg, Xu and Richardson2013, Minor & Kobe Reference Minor and Kobe2019). Therefore, we expect that a higher basal area index will positively impact on the dimensions of α-diversity. Tropical forests show considerable vertical variation from soil to canopy in terms of vegetable stratification (Clark et al. Reference Clark, Olivas, Oberbauer, Clark and Ryan2008), temperature, humidity and light (Parker et al. Reference Parker, Lowman and Nadkarni1995), and this complexity of environmental factors stimulates different strategies to take advantage of available resources (Bazzaz Reference Bazzaz1975). Therefore, considering that vegetation structure is an important factor in the availability of shelter and food, we expect an increase in the dimensions of α-diversity in the higher parts of the terrain. In addition, soils are sandier, waterlogged and poorly oxygenated in areas close to water bodies, which limits the growth of many plant species, affecting the diversity of resources such as food and shelter for bats in these areas (Costa et al. Reference Costa, Magnusson and Luizao2005, Toledo et al. Reference Toledo, Castilho, Magnusson and Nascimento2017). Due to these conditions, we expect a positive relationship between the distance from the nearest watercourse and the dimensions of α-diversity.
Regarding the functional traits of the bat assemblage, we expect higher values for mean body mass and wing morphology (relative wing loading and wing aspect ratio) in environments with fewer obstacles, which tend to have less vegetation clutter and understory obstruction, a lower basal area, and a higher canopy. We also expect higher mean values for body mass, wing aspect ratio and relative wing loading in lower areas and closer to the nearest watercourse due to less vegetation clutter that favors bat navigation (Ober & Hayes Reference Ober and Hayes2008, Sleep & Brigham Reference Sleep and Brigham2003). Animalivorous bats have lower values for aspect and relative wing loading (Marinello & Bernard Reference Marinello and Bernard2014), and these characteristics allow them to better exploit environments with more obstacles. Therefore, we expect a higher average weight of bats of this trophic level in areas with greater vegetation cluttering, basal area, and lower canopy height and in higher areas and further away from watercourses, where vegetation is more obstructed (Oliveira et al. Reference Oliveira, Marciente, Magnusson and Bobrowiec2015). On the other hand, phytophagous bats that are more generalists will have higher average weight in less complex environments, less elevated areas and closer to the nearest watercourse, due to the greater abundance of fruits in these areas (Marques et al. Reference Marques, Ramos Pereira and Palmeirim2012).
Methods
Study area
This study was carried out in the Floresta Nacional do Amapá (FLONA of Amapá), a sustainable use protected area located in the extreme northeast of the Brazilian Amazon. The FLONA of Amapá has an area of 459,800 ha (Figure 1 – ICMBio 2016). The region’s climate is hot and humid, with temperatures ranging from 24 to 28 °C, and annual precipitation ranging between 2,300 and 2,900 mm (Oliveira et al. Reference Oliveira, Cunha, Jesus, Barreto, Cunha, Souza and Cunha2010, Reference Oliveira, Canani, Barreto and Cunha2020). The wet season, which lasts from February to May, concentrates 70% of the total annual precipitation, which can exceed 500 mm/month. The dry season (September to November) concentrates 30% of annual precipitation and is characterised by <250 mm/month (Oliveira et al. Reference Oliveira, Cunha, Jesus, Barreto, Cunha, Souza and Cunha2010, Reference Oliveira, Canani, Barreto and Cunha2020).

Figure 1. Location of the study area in the state of Amapá, Brazil (a), southern part of the Amapá National Forest (b), highlighting the 15 sampling points (black squared) in the permanent plots (solid line) of the Biodiversity Research Program (c).
Most of the FLONA of Amapá is located at elevations between 50 and 160 m a.s.l (ICMBio 2016); however, in the northwest and east portions, the elevation varies from 200 m a.s.l. The phytophysiognomy of the FLONA of Amapá is heterogeneous, and the main vegetation types are dense alluvial forest and dense ombrophilous forest (ICMBio 2016).
Bat sampling
Sampling was carried out in 15 permanent plots installed in a system of trails established by the Brazilian Program for Research in Biodiversity (PPBio; https://ppbio.inpa.gov.br/ – Figure 1). PPBio uses the RAPELD method (in Portuguese, RAP = rapid assessment protocol; PELD = long-term ecological survey) for sampling different biological groups (Magnusson et al. Reference Magnusson, Lima, Luizão, Luizão, Costa, Castilho and Kinupp2005). The trail system consists of 6 trails installed along the east-west gradient and 6 trails along the north-south gradient, covering a total area of 25 km2. The trails allow access to 30 permanent plots systematically distributed at 1 km intervals along the east-west trails (Magnusson et al. Reference Magnusson, Braga-Neto, Pezzini, Baccaro, Bergallo, Penha, Rodrigues, Verdade, Lima, Albernaz, Hero, Lawson, Castilho, Drucker, Franklin, Mendonça, Costa, Galdino, Castley, Zuanon, do Vale, dos Santos, Luizão, Cintra, Barbosa, Lisboa, Koblitz, da Cunha and Pontes2013). The plots are 250 m long and follow the ground-level relief, thus minimising internal variations in soil and topography. The 15 plots were chosen to ensure the greatest variation in topography (elevation) and vegetation structure (e.g., diameter and height of trees) and distance to the nearest watercourse (Figure 1).
We visited each plot twice during the dry season, between November 15 and December 2, 2017, and once in the rainy season, between February 27 and March 7, 2018. During each visit (sampling night), we captured bats using eight mist nets (12 × 3 m in size and 14 mm mesh) per plot. The nets were set up 15 minutes before sunset (∼18h:00) at ground level and remained open for 6 hours (∼00h:00), being inspected every 15 minutes (Carvalho et al. Reference Carvalho, Norris and Michalski2016). The sampling effort was calculated following recommendations by Straube and Bianconi (Reference Straube and Bianconi2002), totalling 5.184 m2.h per plot and 77.760 m2.h across all plots.
After removing bats from the mist net, we measured their forearm size with a caliper and body mass with a spring scale (Pesola brand with an accuracy of ±0.3%). Subsequently, bats were identified in the field with the help of taxonomic keys and descriptions (Lim & Engstrom Reference Lim and Engstrom2001, López-Baucells et al. Reference López-Baucells, Rocha, Bobrowiec, Bernard, Palmerim and Meyer2016, Reis et al. Reference Reis, Fregonezi, Peracchi and Shibatta2013, Reference Reis, Peracchi, Batista, de Lima and Pereira2017). Captured individuals were marked with a collar with individual identification (Esbérard & Daemon Reference Esbérard and Daemon1999) and later released. We restricted our analyses to bats of the Phyllostomidae family and the aerial insectivore Pteronotus sp. (family Mormoopidae) because bats from other families are not commonly captured in ground-level mist nets (Kalko et al. Reference Kalko, Handley, Handley, Cody and Smallwood1996a).
Forest structure
We estimated the vegetation clutter using the point-of-intercept sampling method (Dias-Terceiro et al. Reference Dias-Terceiro, Kaefer, de Fraga, de Araújo, Simões and Lima2015). This method consists of quantifying the number of direct contacts with leaves and branches on a 1.5 m long stick held vertically to 50 cm above the ground (Bobrowiec & Tavares Reference Bobrowiec and Tavares2017, Dias-Terceiro et al. Reference Dias-Terceiro, Kaefer, de Fraga, de Araújo, Simões and Lima2015). We counted the number of points that touched the stick every 2 m in a 100 m stretch located on the central track of the plot and in the same portion where the mist nets were installed. This procedure to estimate the vegetation clutter was carried out in parallel to and 1 m from the mist nets in order to minimise interferences caused by the removal of vegetation when the nets were placed. Thus, the vegetation clutter in each plot was represented by the total number of contacts with the stick over the 100 m.
Basal area (BA) and canopy height data were obtained through a vegetation structure inventory carried out previously in accordance with the protocol established by PPBio (Castilho et al. Reference Castilho, Schietti, Freitas, Araújo, Coelho, Magnusson and Costa2014). Trees with diameter at breast height (DBH) ≥1 cm and <30 cm were inventoried within the plots in an area of 2 × 250 m and trees with a DBH ≥ 30 cm were sampled in an area of 40 × 250 m. DBH was measured at 1.3 m above ground level or at 50 cm above tabular roots or trunk irregularities. The basal area of the plot was calculated by adding the basal area values derived from the DBHi of each tree, using the following equation:

where BA is the basal area in m2. The calculation was performed for trees with 1 ≤ DBH < 30 cm and DBH ≥ 30 cm separately (due to sampling in differently sized areas), and the basal areas were multiplied by 20 and 1 (respectively), then added together to provide an estimate per hectare.
A laser hypsometer (TruPulse® 360) was used to measure the total height (from the base of the trunk to the top of the crown) of 1.156 trees (1 – 109 cm DBH) selected in 5 plots. The height calculation was performed using the tangent method (Larjavaara & Muller-Landau Reference Larjavaara and Muller-Landau2013), using the distance and the lower and upper angles of the tree in relation to the operator. The relationship between total height and DBH was adjusted using the Michaelis–Menten equation to generate an allometric model capable of predicting height using the following equation:

Equation 2 was then used to estimate the total height of the inventoried trees, and the mean height was calculated to represent the canopy height of the plot as a function of DBH.
Terrain elevation and distance to the nearest watercourse
We used the geographic coordinates of the initial part of each plot to determine its position and thus obtain the elevation through a digital elevation model (DEM) from the Topodata project available at http://www.webmapit.com.br/inpe/topodata/, which is derived from the Shuttle Radar Topography Mission – STRM. The distances to the nearest watercourse were measured from the location of the plots and the network of river channels generated with the DEM data. Using Quantum GIS 3.6.3 software and data on river channel networks (Fill sinks modules), we calculated the shortest distance from the central point of each plot to the closest point of the river channel.
Functional traits
We chose four functional traits that have previously been shown to be correlated with the distribution of bats in tropical forests, namely: body mass, trophic level, relative wing loading and wing aspect ratio (Castillo-Figueroa & Pérez-Torres Reference Castillo-Figueroa and Pérez-Torres2021, Cisneros et al. Reference Cisneros, Burgio, Dreiss, Klingbeil, Patterson, Presley and Willig2014, Marciente et al. Reference Marciente, Bobrowiec and Magnusson2015). The trait data were obtained based on data collected during the manipulation of bats in the field and data from the literature.
Bat body mass may be a determining factor in habitat use (Hanspach et al. Reference Hanspach, Fischer, Ikin, Stott and Law2012, Ramos Pereira et al. Reference Ramos Pereira, Marques, Santana, Santos, Valsecchi, Queiroz, Beja and Palmeirim2009). Large, heavier bats, such as Artibeus lituratus and Phyllostomus hastatus, prefer to forage on larger fruits that occur in the tree canopy, whereas small bats like Carollia spp. prefer smaller fruits that are available in the lower forest strata (Kalko et al. Reference Kalko, Herre and Handley1996b, Marques et al. Reference Marques, Ramos Pereira and Palmeirim2012, Rex et al. Reference Rex, Michener, Kunz and Voigt2011, Shanahan & Compton Reference Shanahan and Compton2001, Voigt Reference Voigt2010). Body mass was determined as the mean of the body mass of adult individuals of each species captured in this study.
Both the relative wing loading (division of body mass by the wing area) and wing aspect ratio (division of the square of the wingspan by the wing area) directly influence the maneuverability, agility and flight speed of bats (Marinello & Bernard Reference Marinello and Bernard2014), being important predictors of habitat use in foraging (Muller et al. Reference Muller, Mehr, Bassler, Fenton, Hothorn, Pretzsch, Klemmt and Brandl2012, Norberg & Rayner Reference Norberg and Rayner1987). Bats that have a low relative loading and a lower wing aspect ratio are better able to forage in places with more obstacles (Marinello & Bernard Reference Marinello and Bernard2014, Silva Reference Silva2012). Species with these characteristics have wings capable of producing the high curvature necessary to maintain lift in low-speed flights, favoring success in environments with higher levels of obstruction (Stockwell Reference Stockwell2001). Bats that have a high relative wing loading and a high wing aspect ratio have constant high-speed flights and low-energy costs (Norberg & Rayner Reference Norberg and Rayner1987). These characteristics favor flights in less obstructed environments and over long distances in search of food (Norberg & Rayner Reference Norberg and Rayner1987). Values for relative loading and wing aspect were obtained from Marinello & Bernard (Reference Marinello and Bernard2014).
Resource availability is a factor in the distribution of individuals of all animals trophic guilds, with individuals seeking areas with better chances of finding available food (Capaverde et al. Reference Capaverde, Pereira, Tavares, Magnusson, Baccaro and Bobrowiec2018, Marinello & Bernard Reference Marinello and Bernard2014, Marciente et al. Reference Marciente, Bobrowiec and Magnusson2015, Oliveira et al. Reference Oliveira, Marciente, Magnusson and Bobrowiec2015, Silva Reference Silva2012). We classified bats into two trophic guilds: animalivorous, which includes insectivorous, carnivorous and hematophagous bats, and phytophagous, which includes frugivorous and nectarivorous bats (Giannini & Kalko Reference Giannini and Kalko2004, Schnitzler et al. Reference Schnitzler, Moss and Denzinger2003).
Alpha diversity
We quantified the taxonomic, functional and phylogenetic richness and diversity of bat assemblages sampled in each plot using Hill numbers (Chao et al. Reference Chao, Chiu and Jost2014a, Hill Reference Hill1973). Hill numbers are defined by the q parameter, which reflects their respective sensitivity to relative species abundances, facilitating data comparison (Chiu & Chao Reference Chiu and Chao2014, Hill Reference Hill1973, Roswell et al. Reference Roswell, Dushoff and Winfree2021). Thus, as the q parameter increases, the diversity index becomes more sensitive to common species and less dependent on rare species (Chiu & Chao Reference Chiu and Chao2014). For Hill numbers, q = 0 equals species richness, as species abundances are not considered, and for q = 2, dominant species receive greater weight than rare species, representing the inverse of the Simpson dominance index (Chiu & Chao Reference Chiu and Chao2014, Daly et al. Reference Daly, Baetens and De Baets2018, Li Reference Li2018a). Thus, for each plot, the taxonomic, functional and phylogenetic richness and diversity were quantified considering the variation of the parameter q (q = 0, q= 2), through the “hillR” R package (Li Reference Li2018b). Specifically, the taxonomic richness (q= 0) of each plot was estimated by constructing interpolated and extrapolated species accumulation curves using the “iNEXT” package in R (Chao et al. Reference Chao, Gotelli, Hsieh, Sander, Ma, Colwell and Ellison2014b, Hsieh et al. Reference Hsieh, Ma and Chao2016). Comparisons were made with a sample size of 15 individuals, which is triple the size of the smallest sample. For functional diversity, Hill numbers incorporate functional distances between pairs of species using the functional characteristics of the species (Chiu & Chao Reference Chiu and Chao2014, Chao et al. Reference Chao, Chiu, Villéger, Sun, Thorn, Lin, Chiang and Sherwin2019). For phylogenetic diversity, Hill numbers incorporate a phylogenetic tree (Li Reference Li2018a), and we used a tree derived from the most current phylogeny (Upham et al. Reference Upham, Esselstyn and Jetz2019).
Community weighted indexes
The use of indices weighted by the relative abundances of the species allows for an evaluation of the changes in the mean values of the characteristics of the assemblage/community (Lavorel et al. Reference Lavorel, Grigulis, McIntyre, Williams, Garden, Dorrough, Berman, Quétier, Thébault and Bonis2008). We used the community weighted mean (CWM), which is the predominance of functional traits of the bat assemblages of each plot using a weighted average of the functional trait for the community. The CWM allows access to the average value of functional traits for the community (in our case, the bat assemblage of each plot), weighting this value by the relative abundances of the species in the sample in question (Lavorel et al. Reference Lavorel, Grigulis, McIntyre, Williams, Garden, Dorrough, Berman, Quétier, Thébault and Bonis2008). We estimate the CWM through the BAT’ R package (Cardoso et al. Reference Cardoso, Mammola, Rigal and Carvalho2021).
Data analysis
We evaluated the effect of vegetation clutter, terrain elevation, distance to nearest watercourse, basal area and canopy height on each of the richness and diversity dimensions: taxonomic, functional and phylogenetic and on CWM. First, we tested for the existence of multicollinearity between the predictor variables using variance inflation factors (VIFs). All predictors showed inflation results below 4, indicating that collinearity was not affecting the estimates (Chatterjee & Hadi Reference Chatterjee and Hadi2006).
Posteriorly, using generalised linear models (GLM, Gaussian family), we selected models separately for each q parameter (q = 0, q = 2) of richness, diversity and for CWM, with all possible combinations of predictor variables. Model residuals were tested for normality (Shapiro Wilks, p ≥ 0.05) and homoscedasticity (Breusch–Pagan test, p ≥ 0.05). Based on this, we had to perform two transformations so that the residuals of the models followed a normal distribution: (1) a square root transformation of the distance to the nearest watercourse in the selection of models for CWM of the animalivorous and phytophagous trophic levels; and (2) a square transformation of mean body mass response variable in models.
To identify the predictor variables with greater explanatory support for the response variables, the generated models were selected considering the Akaike information criterion, corrected for small samples (AICc – corrected Akaike Information Criterion). The best model was considered to be the one with the lowest value of AICc (Burnham & Anderson Reference Burnham and Anderson1998), and models that presented ΔAICc values <2 were considered to also be well supported. Subsequently, we calculated the parameter estimates, importance and the unconditional mean of the regression coefficients and their standard errors for each predictor variable, based on all models with ΔAICc < 2. The parameter estimates of the predictor variables were only calculated for the model selections that did not have the null model as the “best” model (i.e., the one with greatest explanatory support – ΔAICc = 0). These estimates and model selection were made using the “Multi-model inference” package (MuMIn), version 1.40.0 (Barton Reference Barton2018). We also confirmed the absence of spatial autocorrelation for the residuals of all models using Moran’s I (Dale & Fortin Reference Dale and Fortin2014). All packages mentioned above were loaded in the R program, version 3.6.1 (R Development Core Team 2019).
Results
Diversity trends and environmental features
We captured 279 individuals belonging to 28 bat species of two families. The most captured species were Rhinophylla pumilio (46 individuals), Artibeus planirostris (44), Artibeus lituratus (31) and Pteronotus sp. (31). The species R. pumilio and A. planirostris represented 32% of all captured bats. Phytophagous bats were more captured (199 captures of 16 species) than were animalivorous bats (80 captures of 12 species – (Supplementary Material – Table S1)).
The plots covered an understory obstruction gradient between 513 and 2.378 touches (1.354.53 ± 636.62; mean ± SD), while basal area ranged from 5.46 to 18.5 m2/ha (12 .51 ± 3.32 m2/ha; mean ± SD), and the canopy height varied from 10.69 to 15.26 m (12.38 ± 1.45; mean ± SD. Supplementary Material – Table S1). The terrain elevation varied in altitude from 99 to 149 m (123.6 ± 13.70 m; mean ± SD). The distance to the stream ranged from 37.48 to 671.46 m (307.11 ± 160.32 m; mean ± SD. Supplementary Material – Table S2).
Environmental factors driving richness and alpha diversity components
Considering the five predictor variables used for model selection, distance to the nearest watercourse was the most important predictor (0.64) for taxonomic richness (q = 0), followed by elevation (0.36). Taxonomic richness increased with distance to the nearest watercourse and with elevation (Figure 2; Table 1). Taxonomic and functional diversity also increased with distance to the nearest watercourse (q = 2; Figure 2; Table 1), this being the most important predictor for taxonomic (0.62) and functional diversity (0.83).

Figure 2. Relationship between the variables selected as the best predictors for taxonomic richness (q = 0 – a, b), taxonomic diversity (q = 2 – c), and functional diversity (q = 2 – d) in a community of phyllostomid and mormoopid bats in the terra firme forest of the northern Brazilian Amazon. The straight line represents the model estimate and the gray area the 95% confidence interval.
Table 1. Estimates of parameters, standard error and unconditional regression coefficient (SE) of the set of models with ΔAICc < 2 related to predictor variables with taxonomic richness (q = 0), taxonomic diversity (q = 2), functional diversity (q = 2), and with the CWM of the mean body mass. The predictors that enter the models are distance from the nearest watercourse – DNW; elevation – ELE; basal area – BA; and vegetation clutter – VCL.

No predictor variable explained phylogenetic richness (q = 0; Supplementary Material – Table S7). For functional richness (q = 0) and phylogenetic diversity (q = 2), the null model had the greatest explanatory support (Supplementary Material – Tables S5 and S8).
Environmental factors that drive the predominance of functional traits
For CWM, the null model had the greatest explanatory support in all cases except body mass (ΔAICc < 2 – Supplementary Material – Table S9-S13). For this functional trait, basal area and vegetation clutter had greater explanatory support, with basal area being present in two of the best selected models (Supplementary Material – Table S9). The basal area was more important (0.76), followed by vegetation clutter (0.29), to predict the variation in mean body mass. However, only the relationship between basal area and mean body mass was significant (p = 0.05 – Table 1). Thus, areas occupied by trees with greater basal area tend to also be occupied by bat species with higher mean body mass (Figure 3).

Figure 3. Relationship between the mean body mass of phyllostomid and mormoopid bat species (measured using the community weighted mean – CWM) and basal area in a terra firme forest in the northeastern Brazilian Amazon. The straight line represents the model estimate and the gray area the 95% confidence interval.
Discussion
We found that taxonomic richness was positively influenced by the distance to the nearest watercourse and terrain elevation (Figure 4). In addition, taxonomic and functional diversity were positively influenced by the distance to the nearest watercourse. Across the Amazon basin, terrain elevation and distance to water bodies have been shown to be important predictors of the richness and taxonomic composition assemblages of bats (Bobrowiec & Tavares Reference Bobrowiec and Tavares2017, Capaverde et al. Reference Capaverde, Pereira, Tavares, Magnusson, Baccaro and Bobrowiec2018), terrestrial mammals (Kinap et al. Reference Kinap, Nagy-Reis, Bobrowiec, Gordo and Spironello2021, Michalski et al. Reference Michalski, Norris, de Oliveira and Michalski2015), understory birds (Bueno et al. Reference Bueno, Bruno, Pimentel, Sanaiotti and Magnusson2012, Cintra & Naka Reference Cintra and Naka2012, Oliveira et al. Reference Oliveira, Almeida, Florencio, Pinho, Oliveira, Ligeiro and Rodrigues2019), amphibians and reptiles (Fraga et al. Reference Fraga, Lima and Magnusson2011, Rojas-Ahumada et al. Reference Rojas-Ahumada, Landeiro and Menin2012, Tavares et al. Reference Tavares, Morcatty, Zuanon and Magnusson2019) and plants (Drucker et al. Reference Drucker, Costa and Magnusson2008, Schietti et al. Reference Schietti, Emilio, Rennó, Drucker, Costa, Nogueira, Baccaro, Figueiredo, Castilho, Kinupp, Guillaumet, Garcia, Lima and Magnusson2014). Therefore, our results show that, as occurs in the southwest and central Amazon, variations in terrain elevation and distance to waterbodies are crucial for structuring bat assemblages at small scales in terra firme forests in the northeastern Brazilian Amazon.

Figure 4. Schematic illustration of the main results regarding the alpha diversity of bats (different types of colours). Higher and more distant areas of waterbodies are richer in species (Hill numbers q = 0). Furthermore, the bat assemblage is more taxonomically and functionally diverse (Hill numbers q = 2) in higher areas and further away from waterbodies.
We found that the taxonomic richness of bats increases with terrain elevation. Subtle variations (up to 100 m a.s.l.) in terrain elevation have been shown to be fundamental for the structuring of bat assemblages (Bobrowiec & Tavares Reference Bobrowiec and Tavares2017, Capaverde et al. Reference Capaverde, Pereira, Tavares, Magnusson, Baccaro and Bobrowiec2018), as terrain elevation has a direct influence on the hydrological and edaphic characteristics of the soil, playing an important role in determining the composition of the plant assemblage (Costa et al. Reference Costa, Guillaumet, Lima and Pereira2008, Schietti et al. Reference Schietti, Emilio, Rennó, Drucker, Costa, Nogueira, Baccaro, Figueiredo, Castilho, Kinupp, Guillaumet, Garcia, Lima and Magnusson2014). For example, in the central Amazon, the richness and abundance of palm trees and shrubs (Kinupp & Magnusson Reference Kinupp and Magnusson2005, Rodrigues et al. Reference Rodrigues, Cintra, Castilho, Pereira and Pimentel2014) and the structure of woody vegetation (Castilho et al. Reference Castilho, Magnusson, de Araújo, Luizao, Luizao, Lima and Higuchi2006) tend to increase with elevation of the terrain. In addition, tall trees (>70 m) are more frequently found at higher elevations (>100 m a.s.l.) in Amazonian forests (Gorgens et al. Reference Gorgens, Nunes, Jackson, Coomes, Keller, Reis, Valbuena, Rosette, Almeida, Gimenez, Cantinho, Motta, Assis, Pereira, Spanner, Higuchi and Ometto2021). More complex forest provides more niches and different ways to harness environmental resources, greater availability of food, shelter and nesting sites (Bazzaz Reference Bazzaz1975, Tews et al. Reference Tews, Brose, Grimm, Tielbörger, Wichmann, Schwager and Jeltsch2003). Thus, forests in higher elevation areas of the Amazon lowlands tend to have a greater frequency of large trees, which produce greater structural complexity, increasing the number of available niches and allowing more bat species to coexist.
The lower value of taxonomic and functional diversity found for areas closer to watercourses may be due to the greater dominance of the frugivores Rhinophylla pumilio, Artibeus planirostris, Artibeus lituratus and the insectivore Pteronotus sp. In these regions, these species represented 54% of the total captures. Soils are more sandy and poorly oxygenated in regions close to waterbodies, which limits the growth of many plant species in these regions (Costa et al. Reference Costa, Magnusson and Luizao2005, Pansonato et al. Reference Pansonato, Costa, Castilho, Carvalho and Zuquim2012, Rodrigues et al. Reference Rodrigues, Cintra, Castilho, Pereira and Pimentel2014, Toledo et al. Reference Toledo, Castilho, Magnusson and Nascimento2017). However, in the Amazon region, several plant species, including pioneers such as Ficus, Cecropia and Vismia, manage to persist under these conditions (Ferreira Reference Ferreira2000, Salo et al. Reference Salo, Kalliola, Häkkinen, Mäkinen, Niemelä, Puhakka and Coley1986). Bats of the genera Artibeus and Rhinophylla are known for their strong associations with fruits of plants of these genera (Andrade et al. Reference Andrade, Thies, Rogeri, Kalko and Mello2013, Giannini & Kalko Reference Giannini and Kalko2004). In the FLONA of Amapá, in the present study, we observed a higher occurrence of Ficus spp., including fruiting individuals, closer to the watercourses. In addition, natural erosion, and subsequent sediment deposition due to river dynamics, forms sandbanks and creates habitats of primary succession on riverbanks (Peixoto et al. Reference Peixoto, Nelson and Wittmann2009). In these environments, there is less competition for solar radiation, and the water table is closer, which allows plants adapted to these conditions to allocate more energy to fruit production (Van Schaik et al. Reference Van Schaik, Terborgh and Wright1993). Consequently, plants of the genus Ficus, Cecropia, Piper and Vismia on riverbanks offer a greater abundance of fruit than plants of these genera in the forest interior (Marques et al. Reference Marques, Ramos Pereira and Palmeirim2012). In addition to this fruit abundance for frugivorous bats, habitats close to watercourses have a significant influence on insect biomass and represent an important foraging habitat for many insectivorous bats (Fukui et al. Reference Fukui, Murakami, Nakano and Aoi2006, Holloway & Barclay Reference Holloway and Barclay2000, Hagen & Sabo Reference Hagen and Sabo2011, Reference Hagen and Sabo2014). Thus, the abundance of food for the bats R. pumilio, A. planirostris, A. lituratus and Pteronotus sp. in areas close to the watercourses may have contributed to the increase in the number of individuals of these species, reducing the Simpson diversity values (q = 2), as this index is more sensitive to dominant species (Chiu & Chao Reference Chiu and Chao2014).
In addition to the increased dominance of a few species close to the watercourses, the decrease in taxonomic richness may be one of the factors responsible for the lower taxonomic diversity in these locations. The conditions that favor the dominance of some species (e.g., high abundance of some food resources, less dense undergrowth) close to watercourses may inhibit the existence of other species in these areas (Oliveira et al. Reference Oliveira, Marciente, Magnusson and Bobrowiec2015), reducing the taxonomic richness. Locations away from watercourses may not have a great abundance of certain resources, but they may contain a greater diversity of them, contemplating a greater number of bat species.
Contrary to our expectations, only one functional trait of the bat assemblages was influenced by environmental variables. The mean body mass weighted by abundance was higher in areas that had a larger basal area (Figure 5). Larger trees have a greater ability to acquire and store nutrients and carbohydrates (Carbone et al. Reference Carbone, Czimczik, Keenan, Murakami, Pederson, Schaberg, Xu and Richardson2013) and tend to produce more fruits (Minor & Kobe Reference Minor and Kobe2019). Thus, this greater availability of fruits reflects in a greater biomass of primary consumers, such as bats (Ramos Pereira et al. Reference Ramos Pereira, Marques, Santana, Santos, Valsecchi, Queiroz, Beja and Palmeirim2009). In addition, bat size can be an important and limiting criterion in habitat use (Hanspach et al. Reference Hanspach, Fischer, Ikin, Stott and Law2012, Stockwell Reference Stockwell2001). For example, locomotion through flight requires bat species to expend high rates of energy during foraging (Norberg & Rayner Reference Norberg and Rayner1987, Voigt & Holderied Reference Voigt and Holderied2012), and larger bats have a higher absolute energy demand (Winter & Von Helversen Reference Winter and Von Helversen1998). Therefore, larger bats are more favored when foraging in areas where the trees have a larger basal area, as the energy costs incurred by the flight activity are offset by the greater availability of food in these areas.
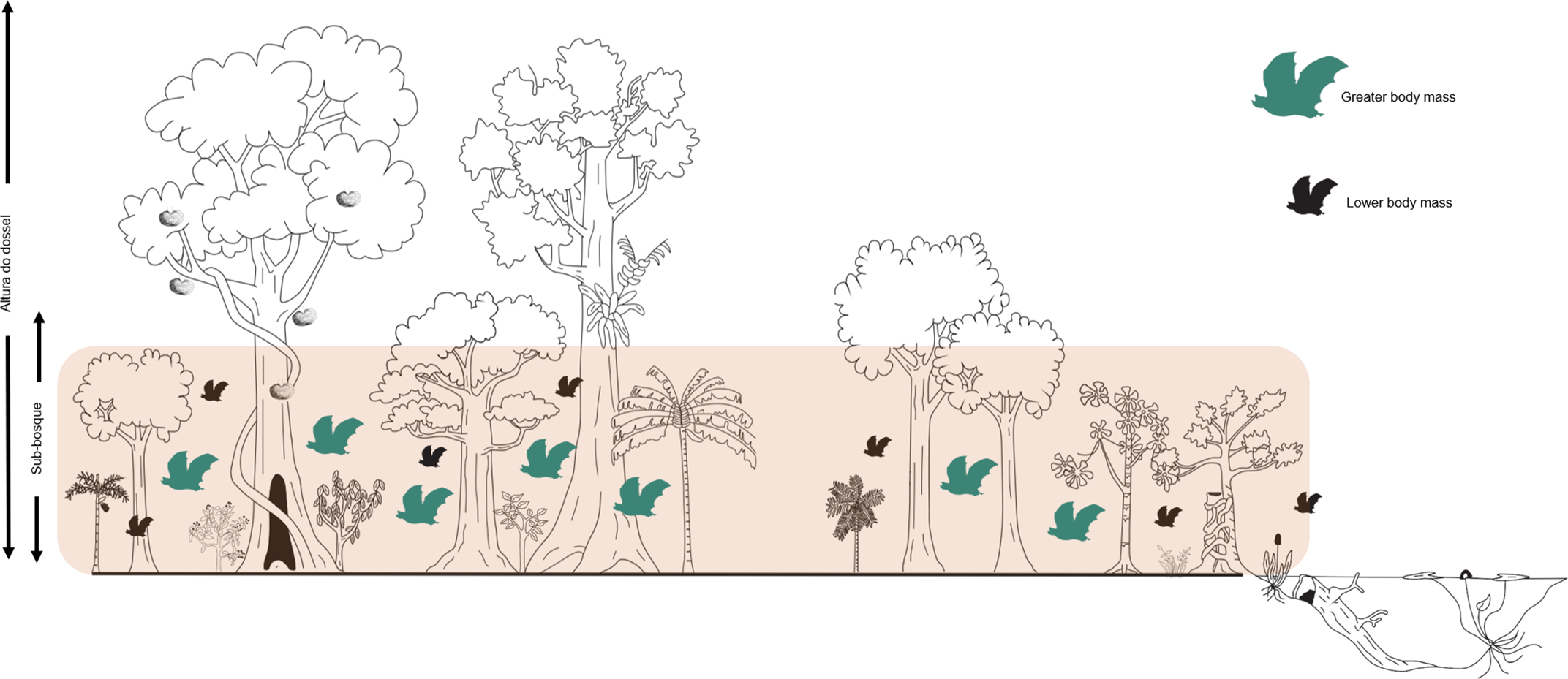
Figure 5. Schematic illustration showing the relationship of mean body mass of bat species (measured using community weighted mean – CWM) with basal area in a terra firme forest in the northeastern Brazilian Amazonia. In the forest, bats with higher mean body mass (in green) were much more favored in areas with a higher basal area index (pink band delimitation).
Despite the low number of captures, compared to other studies carried out in terra firme forests (e.g., Capaverde et al. Reference Capaverde, Pereira, Tavares, Magnusson, Baccaro and Bobrowiec2018, Carvalho et al. Reference Carvalho, Mustin, Farneda, Castro, Hilário, Martins, Miguel, Xavier and Toledo2021, Marciente et al. Reference Marciente, Bobrowiec and Magnusson2015), our multidimensional approach proved to be efficient to understand the effect of environmental filters on the distribution of bats in this Amazon ecosystem. Overall, our study supports the hypothesis that variations in environmental factors in the same forest habitat are sufficient to structure bat assemblages. Specifically, we show that increasing distance to the nearest watercourse and small variations (<50 m a.s.l) in elevation exert a positive effect on the taxonomic and functional richness and diversity of bats in terra firme forest. Furthermore, only the average body mass stood out among the functional traits of the bat assemblage (CWM), showing an association with the basal area of the forest.
Implications for conservation
Our study demonstrated that taxonomic and functional diversity increase with distance (up to 600 m) to the nearest watercourse. Brazilian environmental legislation requires that the vegetation along watercourses is protected in Areas of Permanent Preservation. However, this legislation only protects 30 m from the riparian strip in streams <10 m wide (Brasil 2012). Therefore, if we consider our results, the minimum strip of 30 m left on each side of the streams may not be sufficient for the preservation of different species of bats, and consequently, of the ecosystem services provided by the group (e.g., seed dispersal).
We also found a positive relationship between the mean body mass of bats and the basal area of trees, which could be a variable to be considered in future management plans for selective logging. Due to the increase in human population, and with it the increased demand for timber resources (Kraxner et al. Reference Kraxner, Nordström, Havlík, Gusti, Mosnier, Frank, Valin, Fritz, Fuss, Kindermann, McCallum, Khabarov, Böttcher, See, Aoki, Schimid, Máthé and Obersteiner2013), tropical forests are being degraded at an alarming rate by selective logging (Asner et al. Reference Asner, Knapp, Broadbent, Oliveira, Keller and Silva2005, Hansen et al. Reference Hansen, Potapov, Moore, Hancher, Turubanova, Tyukavina, Thau, Stehman, Goetz, Loveland, Kommareddy, Egorov, Chini, Justice and Townshend2013, Lindquist et al. Reference Lindquist, D’Annunzio, Gerrand, MacDicken, Achard, Beuchle, Brink, Eva, Mayaux, San-Miguel-Ayanz and Stibig2012). In Brazil, the current government has tried to make the bidding model and contracts for forest concessions more flexible to make the process faster and more attractive (Menegassi Reference Menegassi2021). For example, the FLONA of Amapá started a process for logging in an area of 226.000 hectares, which corresponds to almost half of this protected area (Serviço Florestal Brasileiro 2019, 2020). Therefore, it is necessary to promote forest management plans that prioritise the preservation of biological and physical elements such as large trees, which are the focus of current exploration, as well as hollow and dead trees, which are crucial for the supply of food, perches, and shelters for the bat fauna.
Supplementary material
For supplementary material accompanying this paper visit https://doi.org/10.1017/S0266467422000438
Acknowledgements
MMF and BSX were supported, respectively, by master and doctoral scholarships from the Coordenação de Aperfeiçoamento de Pessoal de Nível Superior (CAPES), Brazil. WDC was supported by post-doctoral (PNPD/CAPES) scholarships of the Coordenação de Aperfeiçoamento de Pessoal de Nível Superior (CAPES), Brazil, until early 2020. Currently, WDC is supported by ‘Ayudas Maria Zambrano’ (CA3/RSUE/2021-00197) funded by the Spanish Ministry of Universities. We thank Gustavo Silveira, Cledinaldo Marques, Cremilson Marques, Raimundão and Dona Vita for their help in sampling and logistics for carrying out activities in the field. We also thank Instituto Chico Mendes de Conservação da Biodiversidade (ICMBio) for the logistical help and permissions to carry out this study. We thank Karen Mustin and Hernani Oliveira for the English review of this manuscript. We also thank the reviewers and editors who criticized this manuscript and helped to improve it.
Conflict of interest
The author(s) declare none.