1. Introduction
Automated written feedback (AWF), often provided by automated writing evaluation (AWE) systems, is gaining increasing popularity in language learning and teaching (e.g. Burstein, Riordan & McCaffrey, Reference Burstein, Riordan, McCaffrey, Yan, Rupp and Foltz2020; M. Li, Reference Li2021; Ranalli & Hegelheimer, Reference Ranalli and Hegelheimer2022). Unlike automated scoring, which is aimed at marking or scoring writing for summative purposes, AWF is applied in formative assessment in pedagogical contexts (Stevenson & Phakiti, Reference Stevenson, Phakiti, Hyland and Hyland2019; Weigle, Reference Weigle2013). This feature renders them specifically appropriate and useful for learning and teaching contexts.
AWEs refer to a type of artificial intelligence (AI) that uses natural language processing algorithms to automatically assess and provide feedback on written language productions. Typical AWE systems include Criterion and Pigai, to name but a few. However, the advancement of technology in different areas such as natural language processing has greatly expanded the scope of systems that can provide AWF. For example, word processors such as Microsoft Word and Google Docs, intelligent tutoring systems like Writing Pal, and the recently developed online writing assistants such as Grammarly have all become research objects in studies of AWF (e.g. Ranalli & Yamashita, Reference Ranalli and Yamashita2022; Strobl et al., Reference Strobl, Ailhaud, Benetos, Devitt, Kruse, Proske and Rapp2019). In addition, with the emergence of generative AI such as ChatGPT and Microsoft Copilot, the potential for AWF to utilize these advanced large language models (LLM) for more accurate and comprehensive feedback is highly anticipated (e.g. Barrot, Reference Barrot2023).
Meanwhile, a line of empirical research has been done to investigate AWF, centering on several strategic areas including validity, user perception, impact, and factors influencing students’ or instructors’ use of AWF (M. Li, Reference Li2021). The validity of AWF has often been examined using the accuracy rate and coverage of feedback types in previous research (e.g. Bai & Hu, Reference Bai and Hu2017; Ranalli, Reference Ranalli2018; Ranalli & Yamashita, Reference Ranalli and Yamashita2022). In addition, a stream of research has explored users’ perception and use of AWF, as well as factors that influence their perception and/or use of AWF (e.g. Jiang, Yu & Wang, Reference Jiang, Yu and Wang2020). The main area of investigation of AWF, perhaps, has been the effect of AWF. For example, a line of studies has found AWF helped increase writing score and performance (e.g. Cheng, Reference Cheng2017; Link, Mehrzad & Rahimi, Reference Link, Mehrzad and Rahimi2022); further, Zhai and Ma (Reference Zhai and Ma2023) did a meta-analysis of 26 studies and found that AWF had a large positive overall effect on the quality of students’ writing. Nevertheless, some studies also found no significant improvements in writing after language learners use AWF (e.g. Saricaoglu, Reference Saricaoglu2019). Despite some AWF research synthesis, such as Fu, Zou, Xie and Cheng (Reference Fu, Zou, Xie and Cheng2022) and Nunes, Cordeiro, Limpo and Castro (Reference Nunes, Cordeiro, Limpo and Castro2022), to our knowledge, no systematic review has been conducted to provide a panoramic description of AWF research integrating all these areas. Thus, the present study sought to fill this gap in knowledge by conducting a systematic review of the current state of AWF research, identifying gaps and limitations, and proposing recommendations for future research directions and pedagogy.
2. Past reviews of AWF
Few synthesis reviews conducted on AWF research have mainly examined the effects of AWF (Fu et al., Reference Fu, Zou, Xie and Cheng2022; Nunes et al., Reference Nunes, Cordeiro, Limpo and Castro2022; Stevenson & Phakiti, Reference Stevenson and Phakiti2014; Strobl et al., Reference Strobl, Ailhaud, Benetos, Devitt, Kruse, Proske and Rapp2019; Zhai & Ma, Reference Zhai and Ma2023). Stevenson and Phakiti (Reference Stevenson and Phakiti2014) examined the effects of AWF in the classroom by reviewing 33 articles, including unpublished papers and book chapters. Their review revealed modest evidence to support the positive effect of AWF on students’ writing. Similarly, Nunes et al. (Reference Nunes, Cordeiro, Limpo and Castro2022) reviewed eight articles that adopted quantitative measures to investigate the effectiveness of AWF on K-12 students and found positive evidence. The study done by Zhai and Ma (Reference Zhai and Ma2023) meta-analyzed 26 studies and, unlike former studies, found large positive overall effects. A more recent review was conducted by Fu et al. (Reference Fu, Zou, Xie and Cheng2022) on the outcomes of AWF. In their paper, Fu et al. examined 48 studies, finding that past researchers focused most of their attention on second language (L2) writing, whereas the first language (L1) writing context was rarely researched. They further reported that AWF can positively impact learners’ writing, but it is not as effective as feedback provided by humans (e.g. teachers and peers). In another study, Strobl et al. (Reference Strobl, Ailhaud, Benetos, Devitt, Kruse, Proske and Rapp2019) examined digital tools used for providing AWF. They found 44 tools that supported writing instruction, but most focused on micro-level features of writing, such as grammar, spelling, and word frequencies. These tools would be suitable for providing feedback on argumentative writing in English, but other writing genres, other languages, and micro-level features, such as structures and rhetorical moves, were underrepresented.
It can be seen that the past reviews predominantly centered on the effects or outcomes of AWF, whereas other areas of AWF studies seem neglected in these reviews. Another point to note is that in the past decade, a multifaceted concept of validity consisting of six validity inferences covering multiple aspects related to AWF studies has been proposed and applied (for details, see Chapelle, Cotos & Lee, Reference Chapelle, Cotos and Lee2015; Kane, Reference Kane1992; Shi & Aryadoust, Reference Shi and Aryadoust2023; Weigle, Reference Weigle2013; Williamson, Xi & Breyer, Reference Williamson, Xi and Breyer2012; Xi, Reference Xi2010). Similarly, in this study, we aim to provide a comprehensive view of existing AWF research by encompassing all relevant areas. By doing so, we hope to offer a more complete understanding of AWF and its various aspects. The research questions of the study are as follows:
-
1. What are the research contexts in AWF studies, in terms of ecological setting, language proficiency level, language environment, educational level, and target language(s)?
-
2. What types of AWF provided by AWF systems were investigated, and in what ways was AWF utilized in these studies?
-
3. How can the published AWF research be characterized in terms of the research design, including genres, participants, time duration, sample size, data source, and research methods?
-
4. What are the foci of investigation in the studies, and what results are found? (While effects of AWF might be one focus of investigation, we anticipate accuracy issues and many other areas will also be among the foci of investigation.)
3. Method
3.1 Data set
The relevant research on AWF was identified in Scopus. Scopus is the “largest abstract and citation database of peer-reviewed literature” (Schotten et al., 2018; p31). We identified the following search items based on the relevant literature (e.g. Stevenson, Reference Stevenson2016; Stevenson & Phakiti, Reference Stevenson and Phakiti2014, Reference Stevenson, Phakiti, Hyland and Hyland2019): automat* writ* evaluation, automat*writ* feedback, automat*essay evaluation, automat*essay feedback, computer*writ* feedback, computer*essay evaluation, computer *essay feedback. We also used some common AWF systems such as MY Access! listed in Strobl et al. (Reference Strobl, Ailhaud, Benetos, Devitt, Kruse, Proske and Rapp2019) as search items. The complete search terms and the screenshot (retrieved on January 2, 2023) of our search and the results are presented in Appendix A in the supplementary material. We set the article type as peer-reviewed journal articles and set the end year at 2022. This preliminary search yielded 375 papers. To meet our research purpose, a further screening process was conducted by the two researchers together.
The first criterion was the relevance of the topic in the identified research: by reading the title and the abstract, we excluded those articles irrelevant to AWF (n = 165). Further screening by rereading the abstracts and the research questions excluded studies that solely focused on automated writing scoring (n = 20). The second criterion was that studies should be empirical in nature (e.g. Zou, Huang & Xie, Reference Zou, Huang and Xie2021), and non-empirical studies such as review articles were excluded (n = 34).
To ensure the quality of research, the last criterion was that the articles should be published in Science Citation Index (SCI) or Social Science Citation Index (SSCI) journals, as these journals are rigorously peer reviewed to ensure the quality of the research (Duman, Orhon & Gedik, Reference Duman, Orhon and Gedik2015); those non-SSCI or non-SCI articles were excluded (n = 72). Therefore, a total of 83 articles (see the supplementary material for the complete list) were finalized for this review. The whole screening process is presented in Figure 1 following the Preferred Reporting Items for Systematic Reviews and Meta-Analyses (PRISMA) flow diagram (Page et al., Reference Page, McKenzie, Bossuyt, Boutron, Hoffman, Mulrow and Moher2021).
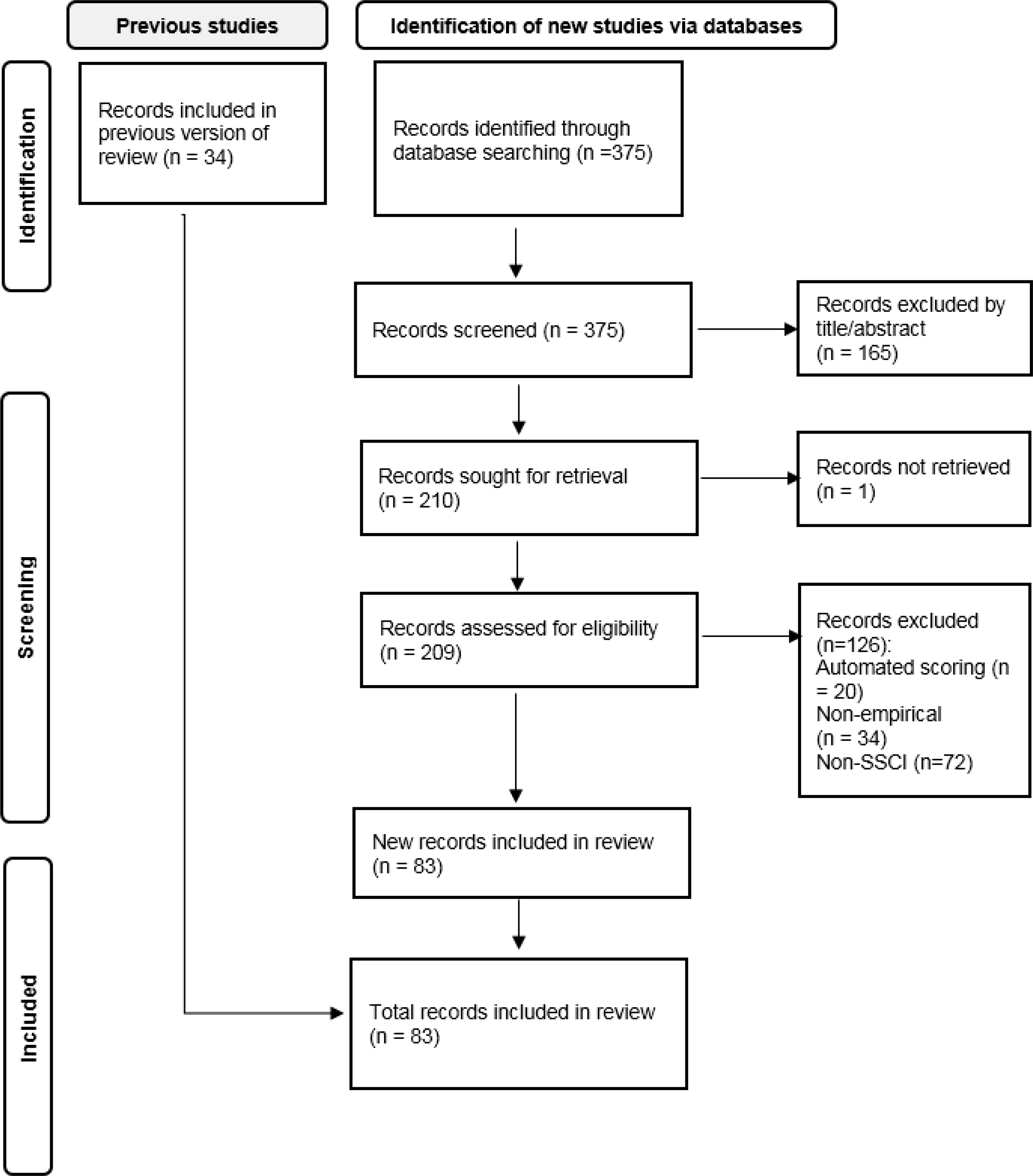
Figure 1. PRISMA flow diagram representing the data selection process
The journals that published these papers are presented in Appendix B in the supplementary material. Together, these papers were published in 40 journals. Figure 2 also displays the trend of publications over the years: a general rising trend of research on AWF is easily noticed, especially in recent years.
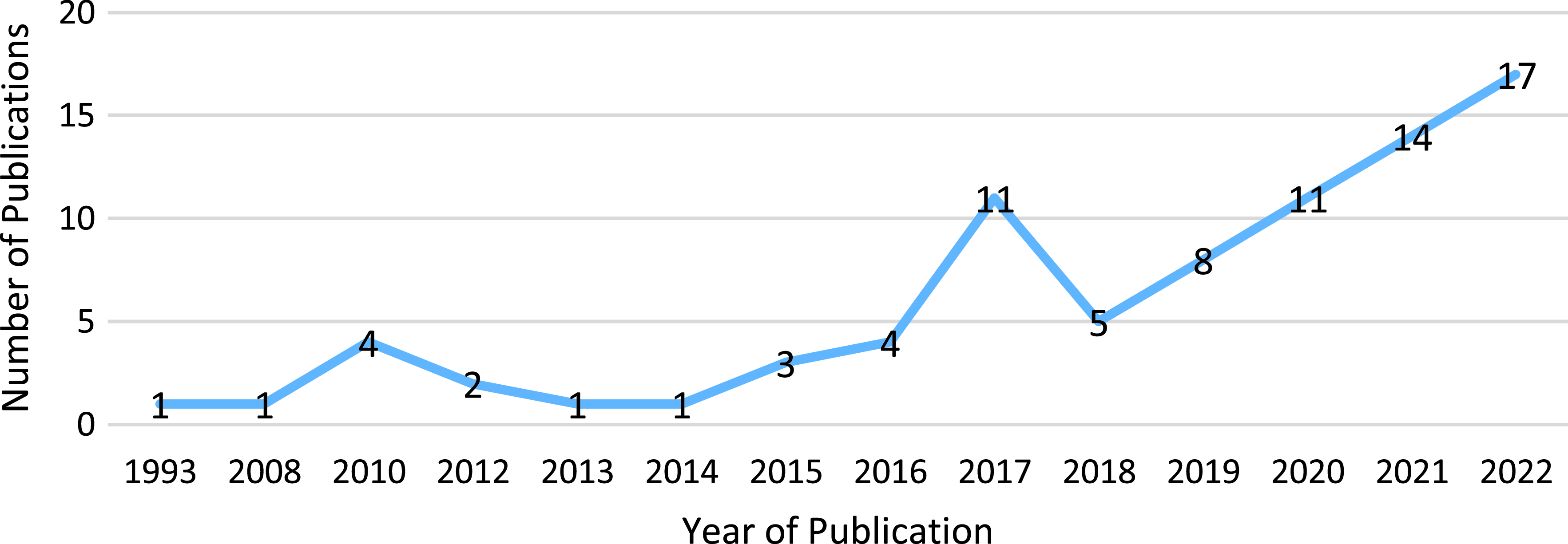
Figure 2. The trend of automated written feedback publications over the years
3.2 Coding
To answer our research questions, we developed a coding scheme that contains four major categories consisting of 17 main variables demonstrated in Appendix C of the supplementary material. To objectively report the results and avoid subjective bias, for the majority of variables, we followed the methods of initial coding and focused coding as used in Shen and Chong (Reference Shen and Chong2023). These two cycle methods were representative of grounded theory (Charmaz, Reference Charmaz2014; Saldaña, Reference Saldaña2016). In our review, the first cycle method we used was initial coding; in this cycle, we directly borrowed words or phrases from each paper that corresponded to each variable. For the variables that required a second cycle method, focused coding was used. During this cycle, we searched for the most frequent or significant codes to develop the most salient categories that make the most analytic sense.
The first category is research context, which contains five variables: ecological setting, language proficiency level, language environment, educational level, and target language. The next category is the description of AWF, comprising the name of AWF systems, and the feedback focus, and ways of using AWF. With regard to the feedback focus, we delineated three categories of feedback based on Shintani, Ellis and Suzuki (Reference Shintani, Ellis and Suzuki2014): form-focused feedback, meaning-focused feedback, and comprehensive feedback. Form-focused feedback centers on language, whereas meaning-focused feedback is about content, as well as other aspects related to content. A combination of these two variables was coded as comprehensive feedback. Concerning the ways of using AWF, we developed 13 types. For instance, when AWF was used to compare with human feedback, the study would be coded as “AWF vis-à-vis human feedback,” and when AWF and human feedback were combined, we would code it as “AWF + human feedback.” Meanwhile, the order of the combination was used to indicate the order of using such feedback.
In the third category, the research design comprised six variables, namely, genres of writing, participants, sample size, time duration, data source, and research methodologies. The last category is made up of three variables: research questions, foci of investigation, and results. For the foci of investigation variable, first we used initial coding to find all research questions and their corresponding results, and then we looked for similarities from the collected data of these two variables and conducted focused coding; through two rounds of focused coding, we identified common categories that surfaced and settled the wording of each subcategory through discussion and with reference to the relevant studies by Chapelle et al. (Reference Chapelle, Cotos and Lee2015), Fu et al. (Reference Fu, Zou, Xie and Cheng2022), and M. Li (Reference Li2021). After three rounds of coding, we identified three primary categories that consisted of 20 subcategories.
In line with Shi and Aryadoust (Reference Shi and Aryadoust2023), the unit of analysis for coding is one study. In our data set, 81 papers each contained one study, and two papers each contained two studies, so we have 85 studies for coding. To ensure reliability, we first selected 20 varied papers and analyzed them together using initial coding and focused coding; after three rounds of discussion, we developed the initial coding scheme. Based on the coding scheme, the first author coded the rest of the articles twice with an interval of six months. The intra-coder reliability was calculated using Cohen’s kappa, the value of which for each variable was above 0.90. All coding was checked by the second author, and the differences were resolved through discussion.
4. Results
4.1 Research contexts
Table 1 presents the research contexts in AWF studies. The first variable concerns ecological settings. Thirty-six studies (42.4%) were conducted in writing classes, and language classes were the second most frequently investigated context. It is noticeable that content classes that focused on subjects other than language or writing were the third most examined context. Regarding language proficiency level, the majority of studies (n = 57) did not state the information about the learners’ language proficiency level. Among the rest of the studies that reported the language proficiency levels, 14 studies examined users at intermediate level, while the remaining studies examined users of two or more different proficiency levels. Concerning the language environment, over half of the studies (n = 45) investigated the foreign language context, followed by the L1 (n = 15) and L2 (n = 15) context. In addition, nine studies looked into a mix of L1 and L2. In terms of the education level, 82.4% of all studies were conducted at the tertiary level, whereas only 15 studies were carried out in K-12 education. For the last variable, the target language of 82 studies was English, and the rest of the studies (n = 3) examined German.
Table 1. Research contexts of automated written feedback studies
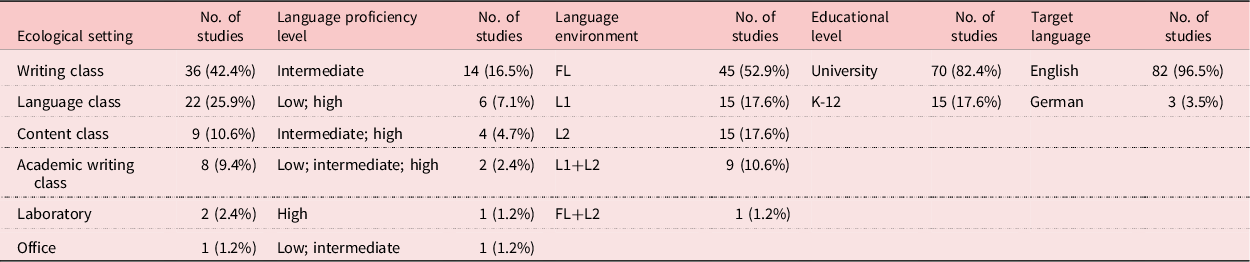
Note. Seven studies did not specify their ecological settings and 57 studies did not specify language proficiency level. FL = foreign language; L1 = first language; L2 = second language.
4.2 AWF system, feedback focus, and ways of using AWF
Table 2 presents the systems that provide AWF on writing. In total, 31 different AWF systems were identified. Most of the systems are conventional AI-based AWE systems such as Pigai (n = 18, 21.2%), which is the most frequently studied system, followed by Criterion (n = 16, 18.8%), and Grammarly (n = 9, 10.6%). In addition, 21 systems were studied only once. It is also noted that intelligent tutoring systems such as Writing Pal (n = 3) and word processors like Microsoft Word Office (n = 1) were also investigated as AWF systems.
Table 2. Automated written feedback systems
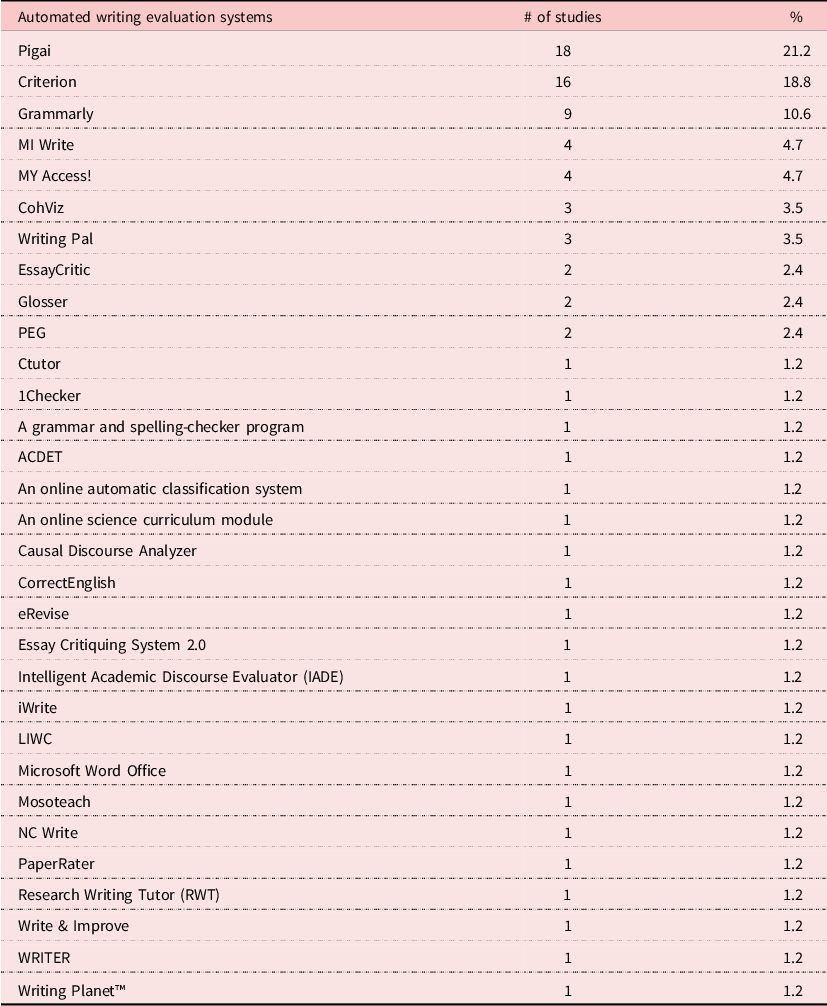
Note. One study (Chapelle et al., Reference Chapelle, Cotos and Lee2015) examined two systems; two studies did not specify the name of the systems.
The feedback focus on the reviewed studies is presented in Appendix D in the supplementary material. As can be observed, over half of the published research (57.6%) was mainly concerned with form-focused feedback, utilizing 12 distinct AWF systems to provide this feedback. Nineteen studies (22.4%) did not distinguish between different types of AWF, and used it the way it is provided by 10 different AWF systems. The remaining 15 studies explored meaning-focused feedback by 11 AWF systems. It is also noted that some AWF systems, such as Criterion, offered the capability to control feedback focus and were represented in multiple subcategories.
Table 3 shows the application methods of AWF in previous research. Overall, 14 patterns in using AWF were identified. Most research focused solely on AWF (n = 55), followed by the comparison of AWF with teacher feedback (n = 10) and with peer feedback (n = 4). In addition, nine studies also investigated the combination of AWF and human feedback, such as teachers and peers. Integrating AWF with certain pedagogies also appeared in several studies. It is also noted that when integrating AWF with other types of feedback, most studies followed the order of AWF first, except for three studies where teacher feedback was provided to students first.
Table 3. Methods of using automated written feedback (AWF)
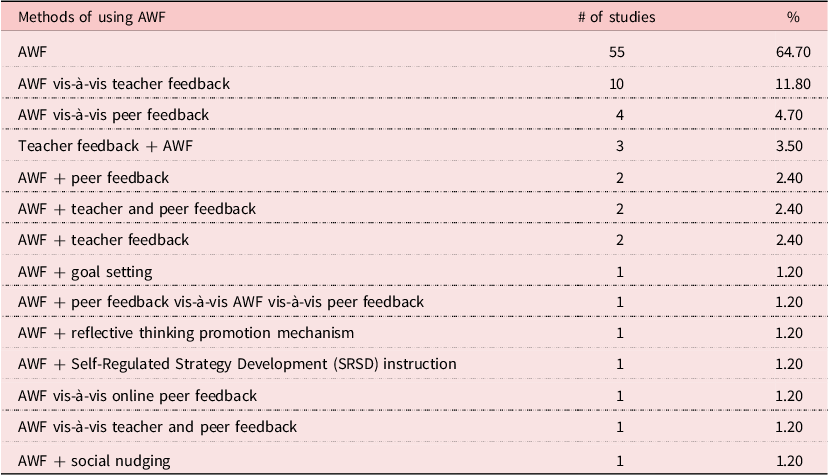
4.3 Research design
The results of the research design analysis are displayed in Appendix E in the supplementary material. The first variable of interest is writing genres: overall, six different types of genres were investigated, with the genre of essays being the most frequent type (n = 59, 69.4%). Nevertheless, 18 studies did not specify the genres of writing, and the rest of the studies examined varied genres, such as research articles (n = 3), reports (n = 1), etc. With respect to participants, learners were the main subject of the studies (n = 73, 85.9%), followed by the studies recruiting both learners and teachers as participants (n = 7). Other studies had a more diversified composition of participants (n = 2). The next variable is the sample size; we noted that 31 of all studies had a large sample size, over 101 participants, while the number of participants in 12 studies was very small, with less than 10 participants. In terms of duration, nearly 50% of the studies were completed over 10 weeks, while 42.9% of the studies did not indicate their time span. On the other hand, 12.9% of the studies were conducted within two to five weeks, while 11.4% were completed within a time span of six to nine weeks. A mere 5.7% (four studies) were conducted in less than two weeks.
The data source used in the studies is further presented in Figure 3, which represents a variety of data used in the AWF studies. The largest number of studies included participants’ writing as the only or one of the data sources (n = 67), while surveys (n = 42) and interviews (n = 29) were the next most frequently used data source. Additionally, though not common, 13 other types of data were also used in these studies, such as think-aloud (n = 5), observation (n = 4), and stimulated recall (n = 4).
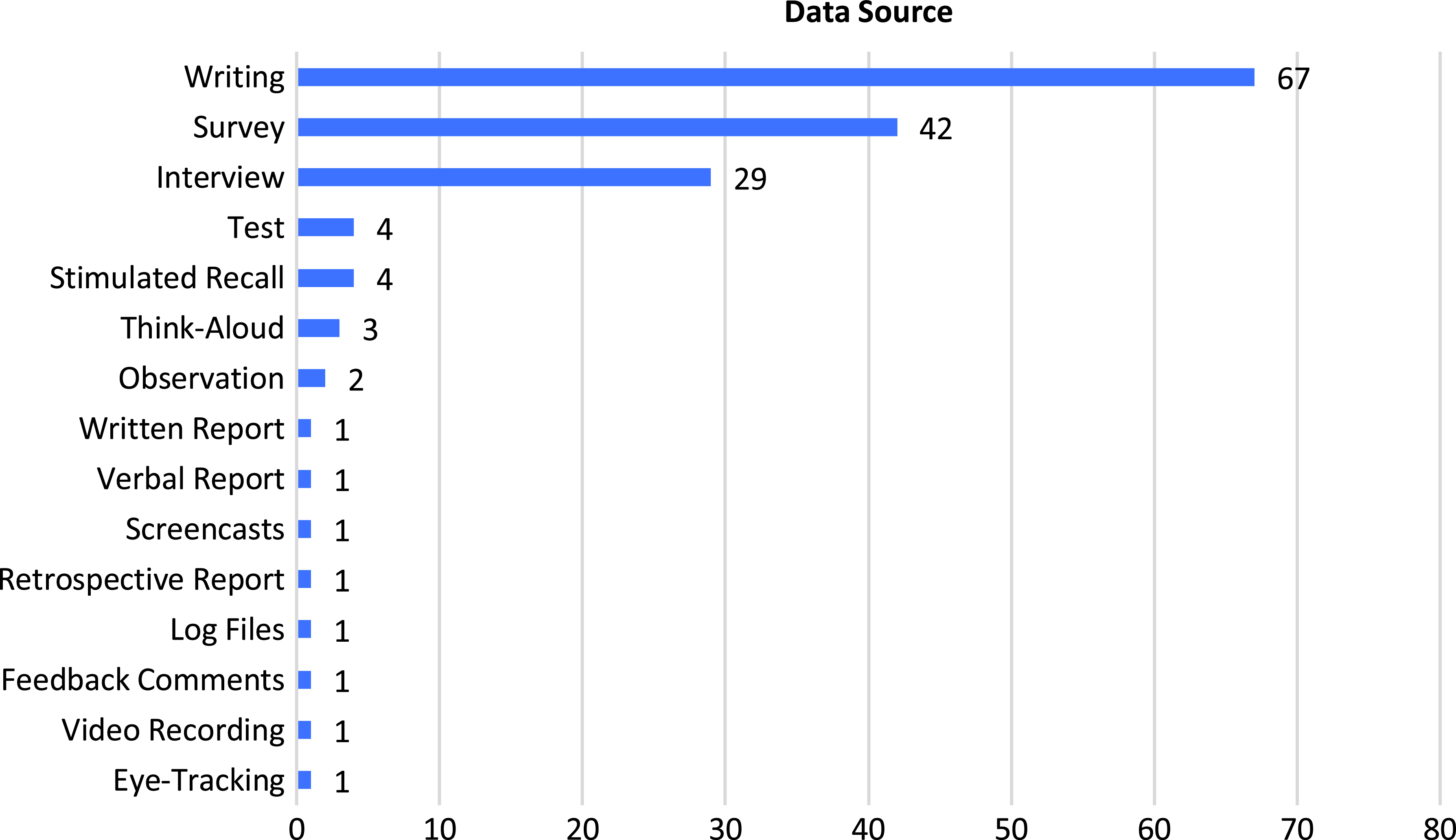
Figure 3. Data source of automated written feedback studies.Note. Thirty-three studies employed only one type of data, whereas the remaining 52 studies used two or more types of data.
The methodologies used in each study, as presented in Figure 4, were also vast and varied. In total, we identified six categories of research methods adopted in the studies. The largest number of studies (n = 34, 40%) adopted mixed methods, followed by quantitative methods (n = 22, 26%). The qualitative method was also used in 13 studies, and one study adopted action research. The rest of the studies explicitly stated the experimental design, with 11 studies using quasi-experiment, and four studies using experimental design.
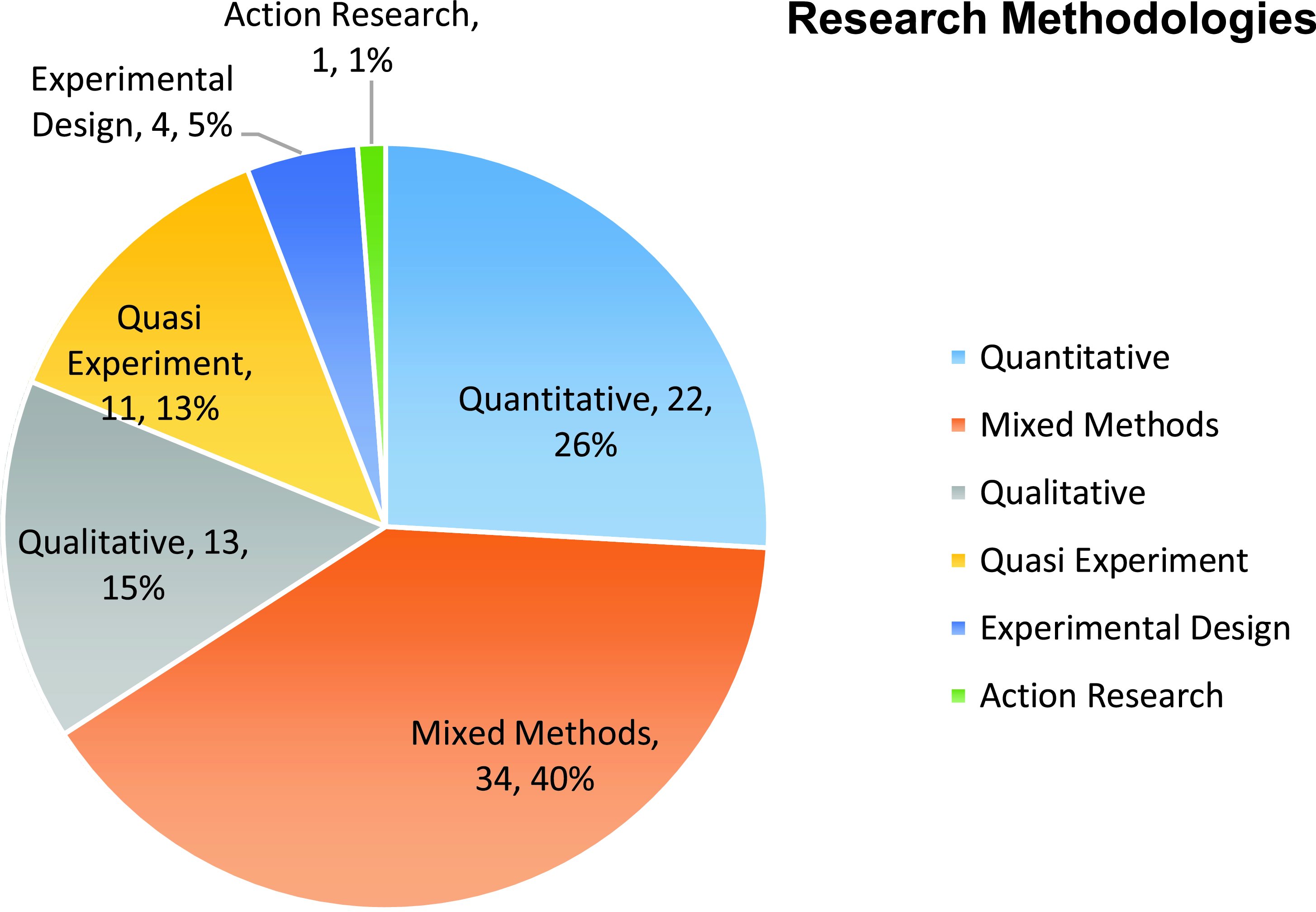
Figure 4. Research methodologies of automated written feedback studies
4.4 Foci of investigation and study results
The foci of investigation and the results of each study are presented in Table 4. As mentioned, three main categories were delineated: the performance of AWF; perceptions, uses, engagement with AWF and their influencing factors; as well as the effects of AWF. Overall, significantly more studies were conducted on perceptions, uses, engagement with AWF and their influencing factors as well as on the effects of AWF than the performance of AWF. Because of space constraints, relevant studies are cited only in the tables.
Table 4. Foci of investigation and results
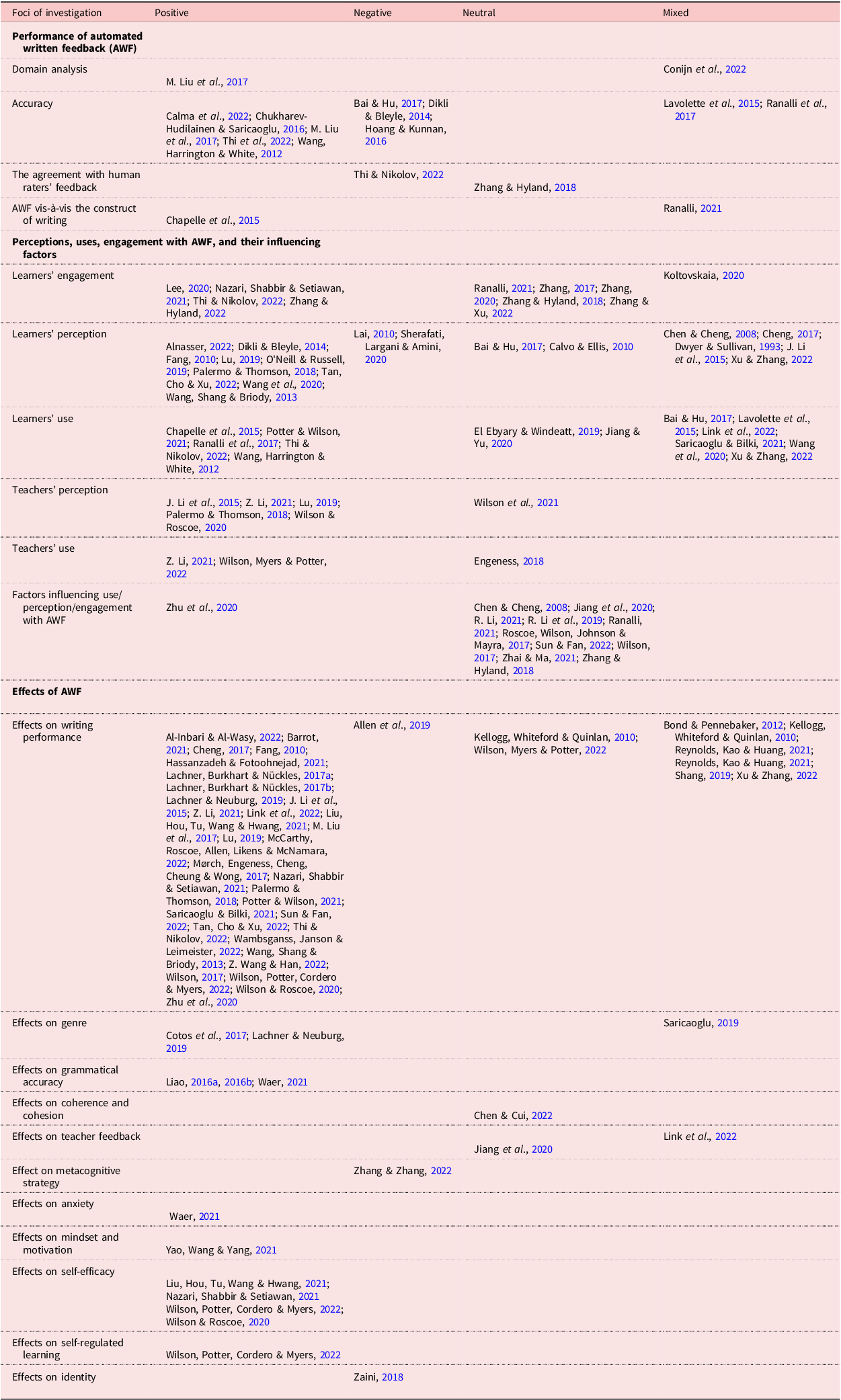
Regarding the first category, four sub-areas were identified. The first area was domain analysis, which refers to whether the domain of AWF matches the targeted writing construct as indicated in authentic writing tasks (Chapelle et al., Reference Chapelle, Cotos and Lee2015). One study (M. Liu, Li, Xu & Liu, Reference Liu, Li, Xu and Liu2017) showed positive results, and the other study (Conijn et al., Reference Conijn, Martinez-Maldonado, Knight, Buckingham Shum, Van Waes and van Zaanen2022) investigated five groups of stakeholders and found that learners were interested in lower-level behavioral constructs, while teachers, writing researchers, and professional development staff focused on higher-level cognitive and pedagogical constructs such as critical thinking. Accuracy and the issue of agreement of AWF with human raters’ feedback were the main foci of investigation in this category. Specifically, five studies provided positive evidence concerning the accuracy of AWF, with the accuracy rate ranging from 0.41 to 0.985. In the meantime, five studies showed negative results in this respect, with the accuracy rate being as low as or less than 50%. Two studies provided mixed results, with the accuracy of some types of errors being satisfactory and the accuracy of other types being low. Three studies that measured agreement between AWF and human raters’ feedback discussed the construct represented by AWF; specifically, two studies showed that compared to teacher feedback that covered both language and content issues, AWF covered fewer features of writing, while one study (Zhang & Hyland, Reference Zhang and Hyland2018) found that both types of feedback had their own strengths.
Perceptions, uses, engagement with AWF and their influencing factors constituted the second focus of the investigation. The concept of engagement was examined in nine studies. Although no studies returned negative results, the study done by Koltovskaia (Reference Koltovskaia2020) found that learners have different levels of cognitive engagement when engaging with AWF. Learners’ perception of AWF was the main interest of a number of studies. Eleven studies reported positive perception by learners, while only two had negative findings. Interestingly, these two studies found that learners preferred feedback from human raters, whether they were teachers or peers. Two studies produced neutral results, which indicated that learners are cognizant of the strengths and weaknesses of both AWF and human rater feedback, while five other studies yielded mixed results; that is, students held positive attitudes towards some aspects or some types of AWF such as feedback on grammar, but expressed negative perceptions towards other aspects or types of AWF like content-related feedback (e.g. Li, Link & Hegelheimer, Reference Li, Link and Hegelheimer2015). A similar pattern also emerged in the use of AWF by learners, where positive (n = 5), neutral (n = 2), and mixed (n = 6) results were found across different studies. In contrast, in terms of teachers’ perception and teachers’ use, most studies showed that teachers were positive about AWF and were willing to integrate AWF into teaching. No mixed or negative results concerning teachers’ perception and teachers’ use were reported.
Factors that might influence perception, uses, and engagement with AWF comprised the last subcategory in this area. A myriad of influencing factors was identified. For example, Zhu, Liu and Lee (Reference Zhu, Liu and Lee2020) found that higher initial scores and contextualized feedback positively impacted such interaction, and vice versa. In addition, the instructor’s willingness to apply AWF (e.g. Jiang et al., Reference Jiang, Yu and Wang2020), perceived trust in AWF (e.g. Ranalli, Reference Ranalli2021; Zhai & Ma, Reference Zhai and Ma2021), perceived ease of use (R. Li, Reference Li2021), and self-efficacy (e.g. Li et al., Reference Li, Meng, Tian, Zhang, Ni and Xiao2019) are all found to play a role in users’ interaction with AWF.
In the category of the effects of AWF, 11 sub-areas were identified. Among these areas, the effect of AWF on writing performance was examined by the highest number of studies (n = 38), with most of them (n = 30) proving the positive effects except one study by Allen, Likens and McNamara (Reference Allen, Likens and McNamara2019), in which no impact was found for AWF. In addition to the overall performance, subconstructs of writing such as genres, grammatical accuracy, coherence, and cohesion were examined in 12 studies, seven of which yielded positive results. Moreover, the impact of AWF on other aspects related to writing such as strategy, and psychological states including self-efficacy, and motivation was also inspected in nine studies, especially in the last two years, of which, seven showed positive effects.
5. Discussion
This study examined 85 studies in 83 papers on AWF. It aimed to answer four research questions concerning research context, description of AWF, research design, foci of investigation, and results. It also aimed to provide a bigger picture of past AWF research to propose future AWF-related research.
5.1 Research question 1
The first research question dealt with research contexts. Our results showed that AWF was mainly studied in language and writing classes at the tertiary level, with English being the target language, and that the majority of studies did not report language proficiency level. Nevertheless, the heterogeneity of research contexts was demonstrated, which also echoes Stevenson and Phakiti’s (Reference Stevenson and Phakiti2014) review. For instance, it is noticed that AWF applied to a wider ecological setting such as content classes of subjects other than language. This heterogeneity indicates a widespread interest in AWF, on the one hand, and warrants the need for careful consideration of the writing construct, on the other hand (Aryadoust, Reference Aryadoust2023), as any variation in delineating the writing construct could significantly impact research results. As a result, even minor changes in the research context could lead to vastly different findings, even if other variables remained consistent (Shi & Aryadoust, Reference Shi and Aryadoust2023).
5.2 Research question 2
The second research question dealt with AWF systems, feedback focus, and the way of using AWF. It was found that a wide range of AWF systems have been applied and that AWF providers are not confined to AWE systems, although they are still the dominant provider of AWF. Other systems capable of providing AWF such as word processors (e.g. Microsoft Word) and online writing assistance (e.g. Grammarly) are also gaining traction in research. All this suggests a broader view and definition of AWF systems.
In terms of feedback focus, we found that the majority of AWF studies focused on form-related feedback, and fewer studies provided meaning-related feedback. This may be partly due to the limited affordances of the AWF systems examined. As the writing construct is rather complex (Vojak, Kline, Cope, McCarthey & Kalantzis, Reference Vojak, Kline, Cope, McCarthey and Kalantzis2011; Weigle, Reference Weigle2013), it appears that most AWF provided by AWE systems is unable to represent the entire construct of writing, and therefore relying solely on AWF in language learning would likely result in an incomplete evaluation of students’ writing abilities. This could also lead to underrepresenting writing for language learners who are in need of personalized feedback and guidance in areas beyond surface-level errors, such as content organization, coherence, and style. Furthermore, as will be discussed, the integration of generative AI such as ChatGPT and GPT-4 in AWF research offers exciting opportunities for more in-depth and nuanced feedback that transcends surface error correction and addresses higher-order writing skills.
Regarding the ways of using AWF, 14 patterns were identified, with most studies investigating AWF in isolation, highlighting the predominant interest in how AWF performs without other interventions. This attention also signals queries about whether AWF aligns with or closely mirrors human feedback in writing. Nevertheless, 16 studies examined AWF in comparison with human feedback, which might provide more substantial evidence regarding the utility and benefits of using AWF compared with human-generated feedback. Other studies combined AWF and human raters’ feedback or learning strategies. The varied ways of utilizing AWF prompt us to reflect on the role of AWF as a complement to teaching or a replacement. Specifically, it is important to investigate the extent of the efficacy of AWF in language learning and whether AWF alone, particularly that provided by generative AI such as ChatGPT, can provide sufficient feedback for learners to improve their writing skills. These are crucial questions to consider in designing future studies and developing effective approaches to AWF implementation in language learning.
5.3 Research question 3
Regarding the research design, our findings further validate the heterogeneous nature of AWF, as showcased in the first research question. The first variable investigated under this heading is the genres of writing, as one of the important features of writing constructs. Our result showed that a range of genres were examined in the previous AWF research, with essays being the most frequently examined type. Although some AWF systems were designed for specific genres (e.g. Research Writing Tutor [RWT]; see Cotos, Link & Huffman, Reference Cotos, Link and Huffman2017), most AWF systems did not specify whether they are suitable for all genres or just certain types of genres, which, if not taken into consideration, could impact the results of teaching and research. It is plausible that prior to the advent of LLMs, which are foundational machine learning models utilized to process, understand, and generate natural language, existing AWF systems were genre specific and/or limited to specific writing prompts. However, this postulation requires verification, as there is a dearth of research that examines the historical development of AWF systems. Concerning the participants, it is clear that learners were the main users of the AWF systems, followed by teachers. It is interesting that some studies expanded the scope of the study to participants such as workplace representatives (e.g. Conijn et al., Reference Conijn, Martinez-Maldonado, Knight, Buckingham Shum, Van Waes and van Zaanen2022; Zaini, Reference Zaini2018), indicating that AWF is not confined to educational settings. The data source and the methodology together imply that researchers have approached AWF from many possible angles. However, although mixed methods and quantitative methods were the common ways, the number of studies adopting an experimental design or quasi-experimental design was relatively small. According to Marsden and Torgerson (Reference Marsden and Torgerson2012), experimental and quasi-experimental designs enable researchers to draw more unambiguous conclusions concerning the potentially causal relationship between the variables of interest, so it is advisable to promote the utilization of these two designs in future AWF research.
5.4 Research question 4
Our fourth research question examined the foci of investigation and results of previous AWE research. Unlike the previous reviews that focused on learning outcomes or the effects of AWF (e.g. Fu et al., Reference Fu, Zou, Xie and Cheng2022; Nunes et al., Reference Nunes, Cordeiro, Limpo and Castro2022), our review provides a comprehensive view of research related to AWF. Three main areas surfaced: the performance of AWF; perceptions, uses, and engagement with AWF and their influencing factors; as well as the effects of AWF. Within each area, several subcategories were identified. Though seemingly varied and loosely related, these areas were interwoven from the perspective of argument-based validity (Chapelle et al., Reference Chapelle, Cotos and Lee2015; Kane, Reference Kane1992). Argument-based validity (ABV) regards issues of validity not as separate, but as a unitary concept that consists of validation of interpretations and uses of assessment instruments and scores (Kane, Reference Kane1992). Domain analysis is the first inference in this argument-based validation framework (Chapelle et al., Reference Chapelle, Cotos and Lee2015) – that is, whether the writing assessment task represents writing tasks in the target language domain. Our results showed that only two studies explicitly investigated the target language domain (Conijn et al., Reference Conijn, Martinez-Maldonado, Knight, Buckingham Shum, Van Waes and van Zaanen2022; M. Liu et al., Reference Liu, Li, Xu and Liu2017). This might partly be due to our exclusion of non-empirical studies in the review. Nevertheless, the importance of domain analysis lies in the identification of language knowledge, skills, and abilities that are needed for test design (Im, Shin & Cheng, Reference Im, Shin and Cheng2019), and in our context, the design of AWF. In light of the importance of domain analysis, it is suggested that further research be conducted to expand domain definition and specification in AWF studies. The results of a robust domain analysis can also be utilized to examine the efficacy of generative AI across all the identified domains and determine where AI can best complement human feedback in the AWF process.
The issue of accuracy pertains to the evaluation and generalization inferences – that is, whether the AWF is an accurate representation of writers’ performance on parallel writing tasks. What we found was a mix of results in this respect; that is, for some AWF providers, positive results were found (e.g. Calma, Cotronei-Baird & Chia, Reference Calma, Cotronei-Baird and Chia2022; Chukharev-Hudilainen & Saricaoglu, Reference Chukharev-Hudilainen and Saricaoglu2016), while for others, negative results attenuated this inference (e.g. Bai & Hu, Reference Bai and Hu2017; Dikli & Bleyle, Reference Dikli and Bleyle2014). Another compromising piece of evidence was that for some AWF providers, such as Criterion, mixed results were produced in different studies (Lavolette, Polio & Kahng, Reference Lavolette, Polio and Kahng2015; Ranalli, Link & Chukharev-Hudilainen, Reference Ranalli, Link and Chukharev-Hudilainen2017). All this suggests that the accuracy of AWF deserves continuous research attention, particularly because in the ABV framework, low-level inferences function as a premise to high-level inferences. To move up to the next inference without validating a former one would be unjustified and can undermine interpretations and uses of the feedback generated by AWF systems.
In addition, there are some data pertaining to the higher-level duo inferences of explanation and extrapolation. The data draw mainly on the comparison of AWF and writing constructs (explanation) and the agreement between human raters’ feedback and AWF (extrapolation). That is, based on the tenets of ABV, AWF should resonate with the construct of interest and human raters’ feedback (Chapelle et al., Reference Chapelle, Cotos and Lee2015). No conclusive evidence was found to support the effectiveness of AWF in comparison to teacher feedback, indicating that AWF and teacher feedback might not be addressing the same aspects of the writing construct. This suggests that AWF may hardly be the equivalent of or replacement for human raters’ feedback. Therefore, for users to choose the appropriate AWF providers, developers of AWF systems should endeavor to disclose the nature and content of the training data. This will result in better compatibility between the educational goals of writing programs and AWF systems.
The next two categories – perceptions, uses, engagement with AWF and their influencing factors, as well as the effects of AWF – belonged to the utilization inference, which concerns whether AWF systems provide meaningful information and positive consequences. As mentioned, if a lower-level inference is not robust, the strength of upper-level inferences would inevitably suffer. So it is not surprising to see a mix of results both in perception, uses, and engagement with AWF and its effects. The inconclusive findings of the study would encourage researchers to continuously explore how learners and teachers perceive, use, or engage with AWF, as well as investigate the factors that impact the effectiveness of AWF.
Regarding the effect of AWF, most studies reported positive results in both general writing performance and different subcategories such as grammatical accuracy. Our findings resonate with the results of previous meta-analysis research that reported positive effects of AWF on writing (e.g. R. Li, Reference Li2023; Mohsen, Reference Mohsen2022). Our review also surfaced increasing research interest in the effects of AWF on constructs related to writing such as anxiety, self-efficacy, and motivation in accordance with Hayes’s (Reference Hayes, Levy and Ransdell1996) model of writing (e.g. Wilson & Roscoe, Reference Wilson and Roscoe2020), indicating the effects of AWF are multidimensional. Accordingly, future research should continue to explore these areas and provide a more comprehensive view of the effects of AWF.
6. Conclusion
With the aim of providing a comprehensive synthesis of AWF research to inform future research, we reviewed 83 papers comprising 85 studies and presented our results in four main areas: research contexts; AWF systems, feedback focus, and ways of using AWF; the research design; and foci of investigation and results.
It was found that although AWF was mainly studied in language and writing classes at the tertiary level, with English being the target language, the heterogeneous nature of AWF research was demonstrated by diverse ecological settings, and language environments in which it was studied. This heterogeneity was also demonstrated in the results of the second and third research questions. That is, it was found that a wide range of AWE systems and ways of using AWF have been applied; three types of AWF were investigated, with form-related feedback being the most frequently examined; and varied research designs were also found in terms of genres of writing, participants, sample size, time duration, data source, and research methods. Looking at it from a different perspective, we argue that this indicates the difference and complexity of the construct of writing for different subgroups from heterogeneous contexts. Meanwhile, three main foci of investigation were observable in the data: the performance of AWF; perceptions, uses, and engagement with AWF and their influencing factors; as well as the effects of AWF. By comparison, limited research was done in the area of validating AWF. For the results, we identified positive, negative, neutral, and mixed results in all three main foci of investigation. Specifically, less conclusive results were found in validating AWF compared to those favoring the other two areas.
We grounded our findings within the argument-based validation framework (Chapelle et al., Reference Chapelle, Cotos and Lee2015; Kane, Reference Kane1992), based on which several suggestions can be offered. First, AWF systems may delineate the application scope with reference to the types of users, the language levels these systems are targeted at, and the genres of writing they are designed for. Second, both AWF providers and researchers may continue to examine the accuracy of AWF, as well as the agreement between AWF and human raters’ feedback, especially teacher feedback. Third, it is suggested that AWF systems clearly state the interpretations and uses of AWF; for instance, under what circumstance will the provided AWF be viewed as the replacement of or the supplement to human feedback? Finally, although the effect of AWF on writing continues to be a thriving research area, effects on other writing-related factors such as self-efficacy, self-regulated strategies, and factors that influence perception, use, or engagement with AWF also merit future research (Aryadoust, Reference Aryadoust2016; Hayes, Reference Hayes, Levy and Ransdell1996).
This review is not without limitations. A limitation is that the articles reviewed were empirical studies indexed in SSCI peer-reviewed journals, which may have restricted the scope of the review. Another limitation is that our review only included papers published till 2022. The advent of transformer-based generative AI systems like ChatGPT promises more personalized feedback. This innovation has garnered great interest, with a fresh body of research emerging this year. Such advancements should be considered in subsequent research endeavors. Nevertheless, unlike past reviews that mainly examined the effects of AWF, the study presented a broader view of AWEs. It is hoped that the study will serve as a useful guide for future AWF research and practice, and the indictments of AWF as identified in the study will be addressed in rigorously designed studies.
Supplementary material
To view supplementary material referred to in this article, please visit https://doi.org/10.1017/S0958344023000265
Acknowledgements
We would like to acknowledge the support from the Teaching Reform Research Project of Yantai University (No. jyxm2022065). We would also like to thank the anonymous reviewers of the journal for their insightful reviews, which helped us strengthen our arguments and improve the manuscript.
Ethical statement and competing interests
All the studies collected for the research can be accessed online. The authors declare no competing interests.
About the authors
Huawei Shi is an English language instructor at Yantai University, China, and currently a PhD student at the Chinese University of Hong Kong. His research interests include technology-enhanced language learning, and language assessment. His work has appeared in journals such as Education and Information Technologies.
Vahid Aryadoust is an associate professor of language assessment at the National Institute of Education of Nanyang Technological University, Singapore; an honorary associate professor at the Institute of Education (IOE) of UCL, London; and a visiting professor at Xi’an Jiaotong University, China. His areas of interest include the application of generative AI and computational methods in language assessment, meta-analysis, as well as sensor technologies such as eye tracking, brain imaging, and GSR in language assessment. He has published his research in Applied Linguistics, Language Testing, System, Computer Assisted Language Learning, Current Psychology, International Journal of Listening, Language Assessment Quarterly, Assessing Writing, Educational Assessment, Educational Psychology, etc.
Author ORCIDs
Huawei Shi, https://orcid.org/0000-0001-7872-3047
Vahid Aryadoust, https://orcid.org/0000-0001-6960-2489