Honesty repeats itself: comparing manual and automated coding on the veracity cues total details and redundancy
In lie detection experiments, honest and false accounts are commonly compared for verbal cues by analyzing data that is manually coded by humans (Chan & Bull, Reference Chan and Bull2014; Deeb et al., Reference Deeb, Vrij, Hope, Mann, Granhag and Lancaster2017; Leal et al., Reference Leal, Vrij, Mann and Fisher2010). Verbal cues are indicators that are based on the content of speech (Vrij, Granhag, et al., Reference Vrij, Granhag, Ashkenazi, Ganis, Leal and Fisher2022). Recently, researchers started using automation to code data (Dzindolet & Pierce, Reference Dzindolet and Pierce2005; Feldman et al., Reference Feldman, Lian, Kosinski and Stillwell2017; Newman et al., Reference Newman, Pennebaker, Berry and Richards2003), but only a few experiments directly compared manual and automated coding (Bogaard, van der Mark, et al., Reference Bogaard, van der Mark and Meijer2019; Jupe et al., Reference Jupe, Vrij, Leal and Nahari2018; Kleinberg et al., Reference Kleinberg, Nahari, Arntz and Verschuere2017; Kleinberg, Warmelink et al., Reference Kleinberg, Warmelink, Arntz and Verschuere2018; Schutte et al., Reference Schutte, Bogaard, Mac Giolla, Warmelink and Kleinberg2021; Vrij et al., Reference Vrij, Mann, Kristen and Fisher2007). Both manual (Nahari, Reference Nahari2018; O’Connell et al., Reference O’Connell, Carter, Taylor, Vernham and Warmelink2023) and automated (Bond et al., Reference Bond, Holman, Eggert, Speller, Garcia, Mejia, Mcinnes, Ceniceros and Rustige2017; van der Zee et al., Reference van der Zee, Poppe, Havrileck and Baillon2022) coding can distinguish honest from false accounts. While manual coding is dominant in lie detection research, it is often criticized for being subjective (Kleinberg & Verschuere, Reference Kleinberg and Verschuere2021). Hence, automated coding was recommended as an objective alternative (Tomas et al., Reference Tomas, Dodier and Demarchi2022).
In the present paper, we report two experiments. In Experiment 1, we compared manual coding with two coding software programs (Linguistic Inquiry and Word Count [LIWC] and Text Inspector) to test which method performs better at discriminating between honest and false accounts based on the verbal cue “total details.” Experiment 2 was developed based on the findings of Experiment 1. LIWC detected differences in total details to a greater extent than Text Inspector and manual coding in Experiment 1, so in Experiment 2, we examined whether this was caused by the fact that LIWC—but not Text Inspector or manual coding—counts redundant (repeated) words in the same text. We also looked at the type of words that were most redundant.
Theoretical approaches to total details as a verbal veracity cue
Several theoretical approaches have been posited for explaining why truth tellers typically report more details than lie tellers. We elaborate below on the information management approach, the cognitive approach, and the Reality Monitoring (RM) approach. The information management approach postulates that both truth tellers and lie tellers attempt to control their verbal behavior during interviews, but they do this in a different manner (Granhag & Hartwig, Reference Granhag and Hartwig2008). Whereas truth tellers are generally forthcoming, lie tellers are more likely to focus on what information they will provide and on what information they will leave out (Hartwig et al., Reference Hartwig, Granhag, Stromwall and Doering2010). Lie tellers are more concerned than truth tellers about contradicting themselves (Deeb et al., Reference Deeb, Vrij, Leal, Giorgianni, Hypšová and Mann2024; Granhag & Strömwall, Reference Granhag and Strömwall1999; Strömwall & Willén, Reference Strömwall and Willén2011), giving away information that may uncover their lie, and/or failing to remember information they previously reported (Toma & Hancock, Reference Toma and Hancock2012; Vrij et al., Reference Vrij, Mann, Leal and Granhag2010). Thus, lie tellers provide “enough” information to appear honest while refraining from providing information that gives leads to their lie (Hines et al., Reference Hines, CoIweII, Hiscock-Anisman, Garrett, Ansarra and Montalvo2010; McCornack, Reference McCornack1992).
According to the cognitive approach, lie telling is a cognitively engaging process (Vrij et al., Reference Vrij, Granhag, Mann and Leal2011, Reference Vrij, Fisher and Blank2017). Lie tellers have to suppress the truth, to think of and update their lies while responding to the interviewer, to control their verbal and nonverbal behavior, and to observe the interviewer’s behavior to assess if they are providing convincing responses (DePaulo et al., Reference DePaulo, Lindsay, Malone, Muhlenbruck, Charlton and Cooper2003; Vrij, Reference Vrij2008). This exhausts the cognitive resources of lie tellers and makes them provide simple and short accounts (Vrij, Granhag, et al., Reference Vrij, Granhag, Ashkenazi, Ganis, Leal and Fisher2022).
According to the RM approach (Johnson & Raye, Reference Johnson and Raye1981), experienced events contain more perceptual and contextual (spatial and temporal) information than imagined events. Truth tellers report about experienced events, whereas lie tellers report at least partially imagined events (Leins et al., Reference Leins, Fisher and Ross2013; Verigin et al., Reference Verigin, Meijer, Bogaard and Vrij2019). Hence, truth tellers’ accounts should be richer in details than lie tellers’ accounts (Amado et al., Reference Amado, Arce, Farina and Vilarino2016; Bogaard, Colwell, et al., Reference Bogaard, Colwell and Crans2019; DePaulo et al., Reference DePaulo, Lindsay, Malone, Muhlenbruck, Charlton and Cooper2003; Sporer & Sharman, Reference Sporer and Sharman2006). Research has shown that truth tellers not only provide more perceptual and contextual details than lie tellers (Harvey et al., Reference Harvey, Vrij, Leal, Hope and Mann2017; Oberlader et al., Reference Oberlader, Naefgen, Koppehele-Gossel, Quinten, Banse and Schmidt2016) but also other types of details such as person, location, action, temporal, and object (PLATO) details (Deeb, Vrij, Leal, & Mann, Reference Deeb, Vrij, Leal and Mann2021; Deeb et al., Reference Deeb, Vrij, Leal, Mann and Burkhardt2022c; Leal, Vrij, Deeb, et al., Reference Leal, Vrij, Deeb and Jupe2018).
Overall, the theories implicate that truth tellers report more details than lie tellers which was corroborated in previous meta-analyses (Amado et al., Reference Amado, Arce, Farina and Vilarino2016; Gancedo et al., Reference Gancedo, Fariña, Seijo, Vilariño and Arce2021). However, not all researchers reached this conclusion (e.g., Vrij et al., Reference Vrij, Leal, Jupe and Harvey2018). Some factors may moderate the differences between truth tellers and lie tellers on total details, including culture or country in which the data is collected (Western vs. non-Western; Leal, Vrij, Vernham, et al., Reference Leal, Vrij, Vernham, Dalton, Jupe, Nahari and Rozmann2019), interview protocol (experimental vs. control; Bogaard et al., Reference Bogaard, Meijer and Van der Plas2020), metric used (Schutte et al., Reference Schutte, Bogaard, Mac Giolla, Warmelink and Kleinberg2021), coding scheme (Nahari, Reference Nahari2023), and coding method (manual vs automated; Kleinberg, van Toolen, et al., Reference Kleinberg, van der Toolen, Vrij, Arntz and Verschuere2018).
Manual coding in lie detection research
In the present research, we were particularly interested in comparing honest and false accounts derived from interviews in mock forensic settings. The common experimental procedure in these types of experiments is that truth tellers honestly discuss activities they performed (e.g., Leins et al., Reference Leins, Fisher and Vrij2012) or a video footage (e.g., Leal et al., Reference Leal, Vrij, Deeb, Burkhardt, Dabrowna and Fisher2023) they watched, whereas lie tellers make up details about these activities or video footage. The interviewees’ responses are then transcribed and manually coded by human raters for specific verbal cues. The raters would either count the number of verbal cue(s) in the transcripts or rate on a scale the extent to which they think the cue(s) emerged. While the counting method is considered to be more objective than the rating method, it is also more labor-intensive, particularly if the rater examines more than one verbal cue (Nahari, Reference Nahari2016).
For any manual coding method, at least two raters are needed to measure inter-rater reliability. In many instances, inter-rater reliability is not high enough due to the subjectivity in coding between human raters (Tomas et al., Reference Tomas, Dodier and Demarchi2022). Differences between raters frequently lead to a replicability problem in which subsequent research cannot replicate the original results (Kleinberg, Warmelink, et al., Reference Kleinberg, Warmelink, Arntz and Verschuere2018). Thus, some researchers started recommending the use of automated coding as an alternative (Kleinberg & Verschuere, Reference Kleinberg and Verschuere2021; Plotkina et al., Reference Plotkina, Munzel and Pallud2020; Tomas et al., Reference Tomas, Dodier and Demarchi2022).
Automated coding in lie detection research
Automated coding is usually conducted via software programs that analyze transcribed interviews through a linguistic approach (Bond et al., Reference Bond, Holman, Eggert, Speller, Garcia, Mejia, Mcinnes, Ceniceros and Rustige2017; Hauch et al., Reference Hauch, Blandón-Gitlin, Masip and Sporer2015). This can be done in different ways, including by providing a count of specific lexical categories (Pennebaker et al., Reference Pennebaker, Boyd, Jordan and Blackburn2015) or by deriving sentence specificity (Kleinberg, Mozes, et al., Reference Kleinberg, Mozes, Arntz and Verschuere2018). Unlike manual coding, automated coding is a faster way of coding large amounts of text. Also, automated coding has been recommended as an alternative to protect against human biases and subjective ratings (Tomas et al., Reference Tomas, Dodier and Demarchi2022).
Automated coding seems to distinguish honest from false accounts well on different verbal cues (Newman et al., Reference Newman, Pennebaker, Berry and Richards2003). It has been suggested that its classification accuracy rate is approximately 70% and similar to manual coding (Bond & Lee, Reference Bond and Lee2005; Mbaziira & Jones, Reference Mbaziira and Jones2016; Tomas et al., Reference Tomas, Dodier and Demarchi2022). However, one meta-analysis (Hauch et al., Reference Hauch, Blandón-Gitlin, Masip and Sporer2015) that compared honest and false accounts when coding software programs were used showed that the effect sizes were small. The authors believed that the small effect sizes could be due to the inability of the software to consider the semantic context, a limitation of automated coding. Further, the authors found that different coding software programs yielded different results, depending on how the software was devised to code verbal cues. Thus, similar to manual coding, coding software programs can produce different conclusions.
Experiments comparing automated and manual coding revealed conflicting results. Whereas some experiments showed that manual coding outperformed automated coding (Vrij et al., Reference Vrij, Mann, Kristen and Fisher2007), others found the opposite effect (Plotkina et al., Reference Plotkina, Munzel and Pallud2020). One experiment (Schutte et al. Reference Schutte, Bogaard, Mac Giolla, Warmelink and Kleinberg2021) that compared automated and manual coding in four different datasets did not find any significant differences between the two coding methods when the same metrics were analyzed. The metrics were either frequency scores (raw number of specific details within an account) or percentage scores (number of specific details compared to the total number of details within an account). Overall, researchers have suggested that the contradictory results across experiments may be moderated by different factors, including manual coding schemes, coding software programs, and metric analyses (Kleinberg et al., Reference Kleinberg, Nahari, Arntz and Verschuere2017, Schutte et al., Reference Schutte, Bogaard, Mac Giolla, Warmelink and Kleinberg2021; Vrij et al., Reference Vrij, Mann, Kristen and Fisher2007).
Experiment 1
In Experiment 1, we aimed to compare manual and automated coding using recently created datasets (see Table 1). We were particularly interested in total details as a veracity cue, because meta-analyses have shown that this cue had a larger magnitude of effect compared to most other tested verbal cues (Amado et al., Reference Amado, Arce, Farina and Vilarino2016; DePaulo et al., Reference DePaulo, Lindsay, Malone, Muhlenbruck, Charlton and Cooper2003). Although this cue has been widely examined, its diagnosticity has yet to be determined when manual and automated coding are directly compared across multiple datasets. We were specifically interested in comparing the manual coding of total details to (a) a software program (LIWC) that is widely researched but that significantly differs from manual coding when coding total details and (b) another software program (Text Inspector) that has not been previously tested but that codes total details similar to manual coding. The automated coding and analyses were carried out in October 2023.
Table 1. Summary of the datasets and conditions included in the present paper
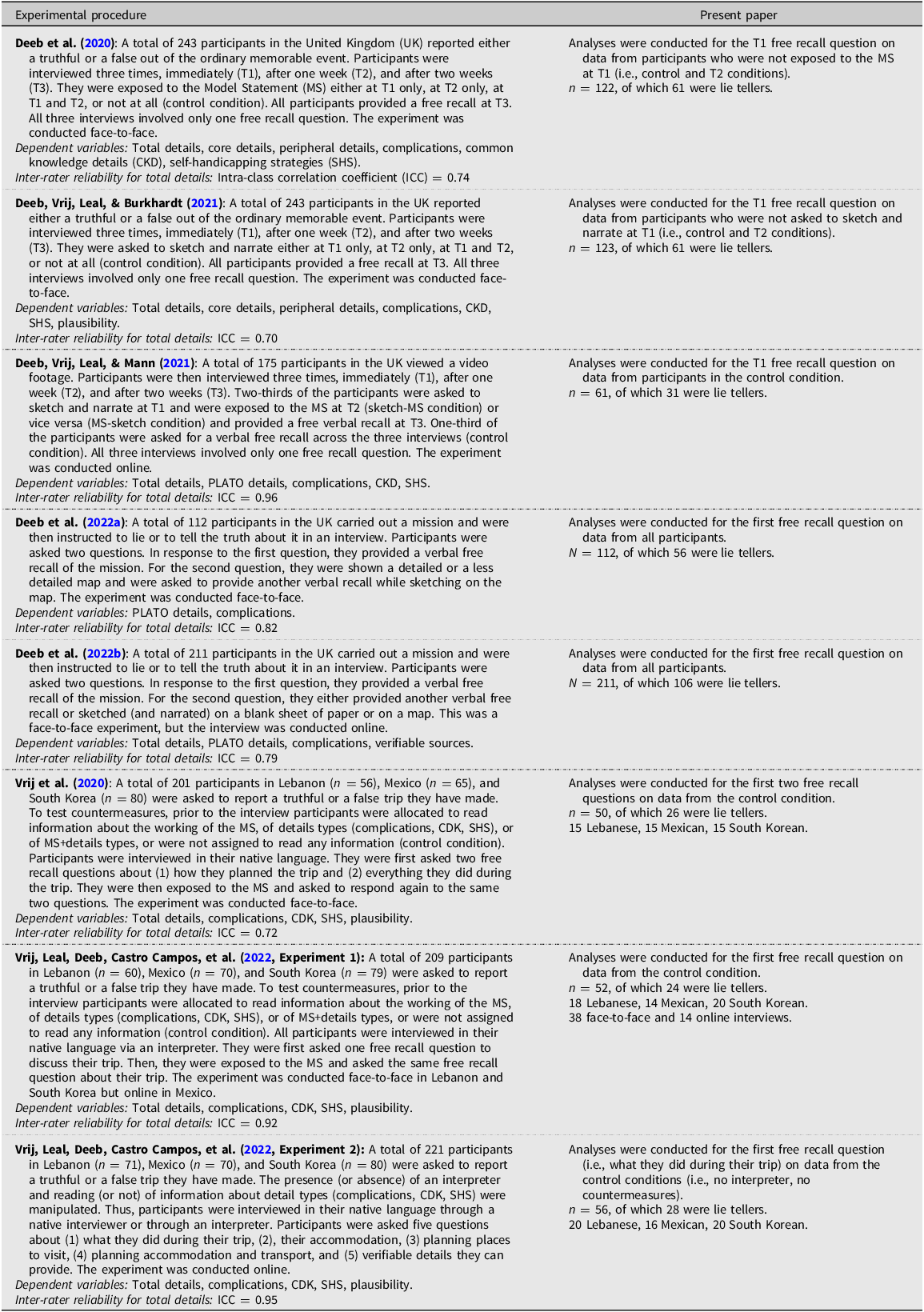
Manual coding of the transcripts
In the original experiments, manual coding schemes differentiated between total details and total word count. Only informative details were coded in a single transcript and the total number of informative details was computed toward the total details score. For example, “It was a side road, I do not remember what the road was called. Uhh but apart from that there was a train station…” includes 24 words, but only the four informative details (underlined) were coded. Across all experiments, redundant words were not coded within a single transcript. That is, if an interviewee mentioned they “walked on the beach and then walked home,” walked would be coded only once as it is a repetition and contains no new information the second time it is mentioned.
The data was manually coded using either an RM coding scheme (Deeb et al., Reference Deeb, Vrij and Leal2020; Deeb, Vrij, Leal, & Burkhardt, Reference Deeb, Vrij, Leal and Burkhardt2021; Vrij, Leal, Deeb, Castro Campos, et al., Reference Vrij, Leal, Deeb, Castro Campos, Fisher, Mann, Jo and Alami2022; Vrij et al., Reference Vrij, Leal, Fisher, Mann, Deeb, Jo, Campos and Hamzeh2020), or a PLATO coding scheme (Deeb et al., Reference Deeb, Vrij, Leal, Fallon, Mann, Luther and Granhag2022a, Reference Deeb, Vrij, Leal, Fallon, Mann, Luther and Granhag2022b; Deeb, Vrij, Leal, & Mann, Reference Deeb, Vrij, Leal and Mann2021). Thus, we included coding scheme as a covariate in the analyses.
The human raters were either one of the authors who had years of experience in coding verbal cues, research assistants who had previously coded verbal veracity cues, or research assistants who had no prior experience with coding cues but were trained. Training always occurred over several sessions. The experienced rater provided the trainee rater with one or more transcripts to code. The experienced rater then provided the trainee rater with feedback for each coded transcript. Afterward, the trainee rater was given another set of transcripts to code. This continued until the rater was able to code the transcripts independently. For all experiments, one rater coded all the transcripts and a second rater coded 15% to 29% of the total number of transcripts for inter-rater reliability purposes.
The inter-rater reliability scores achieved in each dataset are presented in Table 1. Reliability is considered poor for intra-class correlation coefficients (ICCs) less than .40, fair for coefficients between .40 and .59, good for coefficients between .60 and .74, and excellent for coefficients between .75 and 1 (Hallgren, Reference Hallgren2012). The average ICC coefficient across datasets was excellent for total details (ICC = 0.83).
Automated coding of the transcripts
In Experiment 1, we used two software programs for analyzing the data. The first is the Linguistic Inquiry and Word Count software program which is widely used in the lie detection research field (Hauch et al., Reference Hauch, Blandón-Gitlin, Masip and Sporer2015). The second is the Text Inspector software program that to our knowledge was not utilized previously in lie detection research. The inspection of two software programs allowed us to examine potential differences between the programs.
Linguistic Inquiry and Word Count (LIWC) software program
LIWC is a linguistic tool that is psychologically based, analyzing texts for different parts of speech (e.g., pronouns, verbs), psychological constructs (e.g., affect, cognition), and other output variables that constitute more than 90 categories (Pennebaker et al., Reference Pennebaker, Boyd, Jordan and Blackburn2015). Words in analyzed texts are compared to LIWC’s dictionary of linguistic and psychological words and categorized in the corresponding one or more categories (if they fit under more than one category). LIWC is regularly updated and revised based on empirical evidence (e.g., validated emotion rated scales) and other sources (e.g., word extraction software, social media platforms). Its internal consistency as reported by Pennebaker et al. (Reference Pennebaker, Boyd, Jordan and Blackburn2015) is
$\alpha $
= 0.69.
LIWC was developed in 1993 (Francis, 1993) to examine language and expression within the context of health psychology. In 1996, the software was validated using groups of judges who evaluated the extent to which the dictionary of 2000+ words or word stems fit in different categories (Chung & Pennebaker, Reference Chung, Pennebaker and Fiedler2007; Pennebaker et al., Reference Pennebaker, Boyd, Jordan and Blackburn2015). Since then, LIWC has been translated into more than 16 languages. The software has been used by many psychologists, and it has been employed in different cultures and in different areas, including personality psychology, clinical psychology, and lie detection (Addawood et al., Reference Addawood, Badawy, Lerman and Ferrara2019; Newman et al., Reference Newman, Pennebaker, Berry and Richards2003; Pennebaker & Graybeal, Reference Pennebaker and Graybeal2001; Tausczik & Pennebaker, Reference Tausczik and Pennebaker2010).
Unlike manual coding, LIWC does not account for unique words but codes all words regardless of whether or not they are redundant in text. Also, LIWC provides percentage scores except for the total number of words, words per sentence, dictionary words, and punctuations which are presented as frequency scores. In Experiment 1, we used the academic license of LIWC2015 v1.6 and examined LIWC’s total number of words which has the same metric (frequency score) as manual coding and Text Inspector.
LIWC’s founders used it as a lie detection tool and demonstrated its success (Newman et al., Reference Newman, Pennebaker, Berry and Richards2003) which encouraged further lie detection research to utilize the software. It is now the major coding software program tested in lie detection research (e.g., Forsyth & Anglim, Reference Forsyth and Anglim2020; Taylor et al., Reference Taylor, Larner, Conchie and Menacere2017). LIWC can differentiate honest from false accounts based on several parts of speech and constructs (Dzindolet & Pierce, Reference Dzindolet and Pierce2004; Markowitz & Griffin, Reference Markowitz and Griffin2020). However, the diagnosticity of LIWC’s total number of words has yet to be determined. Some researchers found that it was diagnostic with honest accounts including more words than false accounts (Hirschberg et al., Reference Hirschberg, Benus, Brenier, Enos, Friedman, Gilman, Girand, Graciarena, Kathol, Michaelis, Pellom, Stolcke and Shriberg2005; Toma & Hancock, Reference Toma and Hancock2012), whereas others showed the reverse pattern such that false accounts included more words than honest accounts (Bond et al., Reference Bond, Holman, Eggert, Speller, Garcia, Mejia, Mcinnes, Ceniceros and Rustige2017; van der Zee et al., Reference van der Zee, Poppe, Havrileck and Baillon2022). Still, other researchers did not find any significant differences between honest and false accounts on this cue (Bogaard, van der Mark, et al., Reference Bogaard, van der Mark and Meijer2019; Jupe et al., Reference Jupe, Vrij, Leal and Nahari2018; Masip et al., Reference Masip, Bethencourt, Lucas, Segundo and Herrero2012).
Text Inspector software program
Text Inspector is an online language analysis tool that was developed in 2011 to analyze texts for lexical diversity, lexical complexity, and language proficiency (Bax et al., Reference Bax, Nakatsuhara and Waller2019; Weblingua, 2022). In its current form, it can analyze texts for 63 different parts of speech such as articles, verbs, and pronouns and provides the corresponding statistics as frequency scores. Text Inspector is based on empirical evidence in applied linguistics. Since its inception, it has been tested in over 180 countries (Weblingua, 2022). Unlike LIWC, it has not been tested on diverse samples but mostly on student samples. Nonetheless, its data is representative and it has been shown to accurately determine student proficiency levels similar to standardized linguistic tests (Rodríguez, Reference Rodríguez2023). It is regularly updated in line with emerging empirical evidence, and it has scored reliability rates up to 98% (Arslan & Eraslan, Reference Arslan and Erslan2019; Gayed et al., Reference Gayed, Carlon, Oriola and Cross2022). According to Text Inspector’s official website (https://textinspector.com/help/statistics-readability/), the tool is reliable for texts that are longer than 100 words.
Text Inspector is an easy-to-use software that was not tested previously by lie detection researchers. We decided to specifically use it because it can code unique (nonredundant) details similar to manual coding. Text Inspector is also a good alternative to the widely used LIWC as it codes words differently which allowed us to understand lie detection differences between the two coding software programs. The full version of Text Inspector was used for the coding and analyses.
Hypotheses
In line with the majority of previous research, we expected honest accounts to include more total details than false accounts. As the literature shows conflicting results concerning which coding method performs better at lie detection, we did not posit any hypotheses concerning the veracity × coding method interaction effect.
Method
We set several criteria for the inclusion of datasets in our analyses. First, the interviews should have been conducted with only one interviewee. Second, the interviews should have been about a past event as reporting about future events may yield different veracity effects (Sooniste et al., Reference Sooniste, Granhag, Knieps and Vrij2013). Third, the interviews should have included a verbal free recall question at the outset as only this question was used for the analyses to remove the effects of the interview protocol manipulation (see below for more details). Fourth, the relevant paper should have been peer-reviewed and published so that the coded data was readily available for analyses and relevant information on the experiments is accessible for interested readers. Fifth, we were interested in recent data, so only articles published after 2020 were selected. Sixth, the data should have already been manually coded for total details.
All datasets created by the first author and datasets from non-WEIRD samples created by the second author were included in the analyses if they met the above criteria. Including data from non-WEIRD countries in our analyses is an advantage over previous research in which automated and manual coding were compared on transcripts from WEIRD (Western, Educated, Industrialised, Rich, Democratic) samples. There has been an emerging call by researchers in the lie detection field in specific—and in the psychology field in general—to conduct more research in non-WEIRD countries as the majority of psychological research is conducted in WEIRD countries (Denault et al., Reference Denault, Talwar, Plusquellec and Larivière2022; Henrich et al., Reference Henrich, Heine and Norenzayan2010; Vrij et al., Reference Vrij, Leal, Deeb and Fisher2023). Different cultures use different communication modes, and this difference is significant between WEIRD and non-WEIRD countries (Liu, Reference Liu2016). As deception is a communication mode, verbal veracity cues may also differ across countries and cultures (Leal, Vrij, Vernham, et al., Reference Leal, Vrij, Vernham, Dalton, Jupe, Harvey and Nahari2018, 2019; Taylor et al., Reference Taylor, Larner, Conchie, van der Zee, Granhag, Vrij and Verschuere2015) which makes it important to cross-culturally examine manually and automatically coded verbal veracity cues. We thus included the country where the data was collected (i.e., sample’s culture) as a covariate in the analyses.
A total of seven papers were selected for the analyses. One of the papers (Vrij, Leal, Deeb, Castro Campos, et al., Reference Vrij, Leal, Deeb, Castro Campos, Fisher, Mann, Jo and Alami2022) included two experiments, so the total number of datasets that were analyzed was 8. The total sample analyzed included 787 interviewees. A description of the experiments and the data used is presented in Table 1. All experiments involved a face-to-face or an online oral interview. In two face-to-face experiments (Deeb et al., Reference Deeb, Vrij and Leal2020; Deeb, Vrij, Leal, & Burkhardt, Reference Deeb, Vrij, Leal and Burkhardt2021), participants were asked to report a true or a false out-of-the-ordinary memorable event. In two other face-to-face experiments, participants reported truthfully or falsely a mission they completed in a face-to-face (Deeb et al., Reference Deeb, Vrij, Leal, Fallon, Mann, Luther and Granhag2022a) or online interview (Deeb et al., Reference Deeb, Vrij, Leal, Fallon, Mann, Luther and Granhag2022b). In one online experiment (Deeb, Vrij, Leal, & Mann, Reference Deeb, Vrij, Leal and Mann2021), participants reported truthfully or falsely about a video they watched. In the remaining experiments (Vrij, Leal, Deeb, Castro Campos, et al., Reference Vrij, Leal, Deeb, Castro Campos, Fisher, Mann, Jo and Alami2022; Vrij et al., Reference Vrij, Leal, Fisher, Mann, Deeb, Jo, Campos and Hamzeh2020) in which some of the data was collected online, participants described truthfully or falsely a city trip they had made while or while not talking through an interpreter. The experiments by Vrij et al. (Reference Vrij, Leal, Fisher, Mann, Deeb, Jo, Campos and Hamzeh2020) and Vrij, Leal, Deeb, Castro Campos, et al. (Reference Vrij, Leal, Deeb, Castro Campos, Fisher, Mann, Jo and Alami2022) were the only experiments that were ran with non-WEIRD samples, namely in Lebanon, Mexico, and South Korea. Given that some of the data was collected via an online interview and/or via an interpreter, we added interview modality and interpreter presence as covariates in our analyses.
The original experiments tested different interview protocols (e.g., Model Statement interview technique, sketching and narrating interview technique) and compared them with a control condition which was a verbal free recall in all experiments (see Table 1 for the experimental interview protocol conditions). To be included in the present analyses, participants should have been asked for a free recall at the outset of the interview and should have not been subjected to the experimental interview condition. These exclusions minimized the confounding effects of experimental procedures and manipulations. For all analyses, we used the first free recall question which asked participants to discuss everything they did (or viewed), except for Vrij et al. (Reference Vrij, Leal, Fisher, Mann, Deeb, Jo, Campos and Hamzeh2020) for which we used the first two open-ended questions because participants were asked about their plans for the trip they made in the first question and to discuss everything they did in the second question.
The original datasets were cleaned from fillers (e.g., uhm, err), references to participants’ behaviors (e.g., pausing, smiling), and interviewer’s speech as these were irrelevant to the topic of investigation and/or to the coded cues. In the South Korean transcripts of the Vrij et al. experiments, the transcriber added pronouns to the transcripts to explain what the participants were saying because pronouns do not exist in South Korean language (Liu, Reference Liu2016). We kept the pronouns to ensure that we can compare these transcripts with transcripts from other datasets.
The datasets are publicly shared as noted in the original papers or can be obtained from the original authors. The datasets were coded by various raters, and all were derived from the same lab. None of the datasets was previously analyzed using coding software programs.
Results
We ran a mixed effects model to account for clustering in our data as the data is nested in different datasets (Tate & Pitush, Reference Tate and Pituch2007; West et al., Reference West, Welch and Galecki2006). Our model included veracity (honest, false), coding method (manual, LIWC, Text Inspector), and their interaction as fixed factors. The intercepts of participants and datasets were entered as random factors and also as cluster factors. The coding scheme (RM and PLATO), country (Lebanon, Mexico, South Korea, United Kingdom), interview modality (face-to-face, online), and interpreter presence (present, not present) were treated as covariates. We carried out simple contrasts to compare the coding methods. The analysis was conducted using Jamovi 2.3.18 software and Gamlj package (Gallucci, Reference Gallucci2019).
The variance and the ICC of the random intercepts showed variability in the data, so a mixed model analysis could be carried out on the data. The mixed effects model explained 52.4% of the variance (R2 conditional) and showed significant effects of veracity, F(1, 773.32) = 42.44, p < .001, coding method, F(2, 1570) = 644.55, p < .001, and veracity × coding method, F(2, 1570) = 19.53, p < .001.
The parameter estimates of the fixed factors are shown in Table 2 (also see Figure 1 for an illustration). In line with our hypothesis, honest accounts included significantly more total details than false accounts. Both LIWC and Text Inspector coding resulted in more total details than manual coding, but the estimates and t-values were larger for LIWC coding.
Table 2. Fixed effects parameters for total details as a function of veracity and coding method
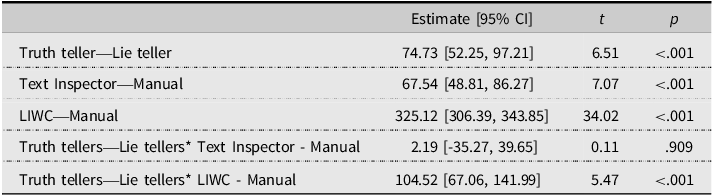
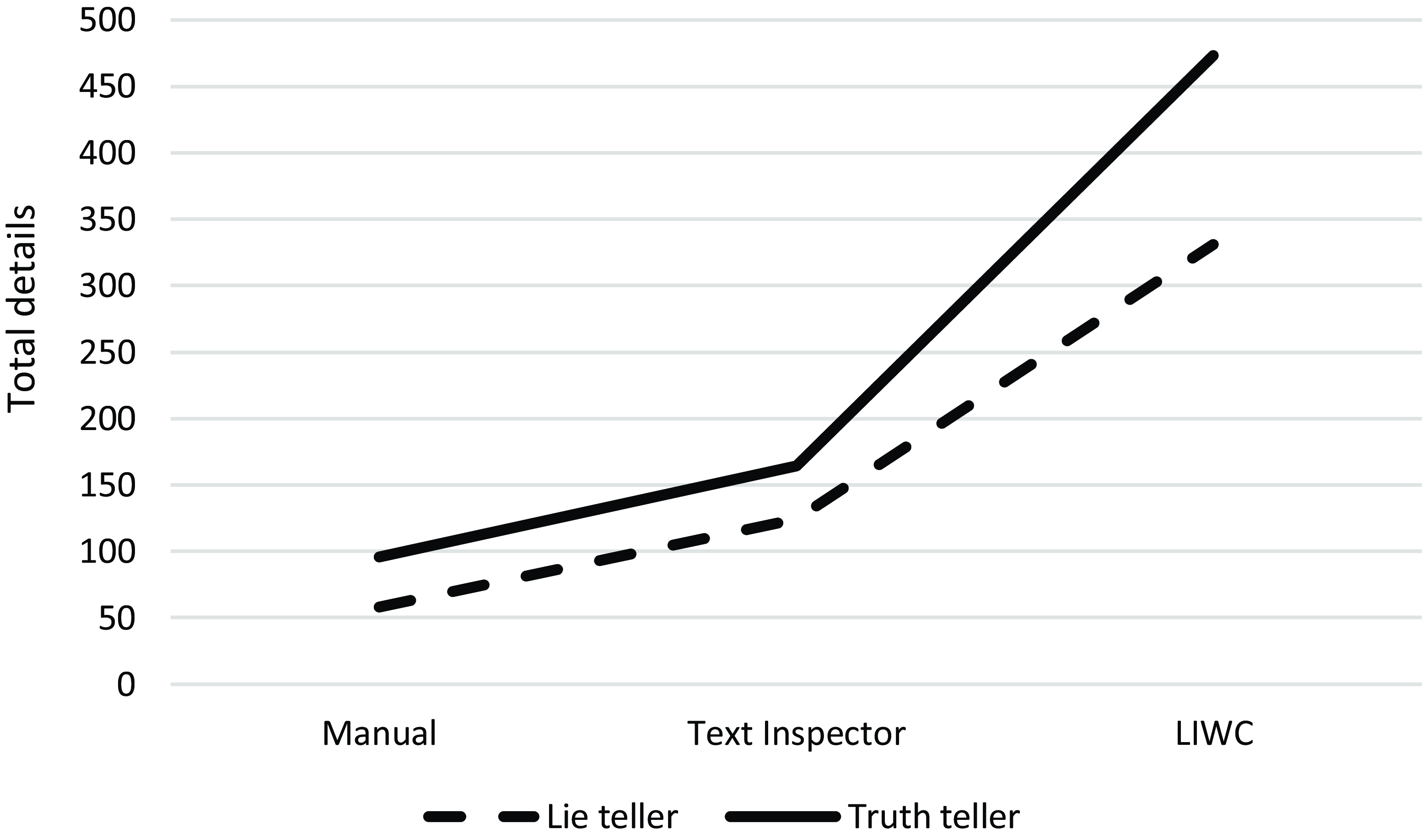
Figure 1. Simple effects for total details as a function of veracity and coding method.
For the interaction effect, veracity differences emerged for LIWC coding, but not for Text Inspector coding, compared to manual coding. To dissect this finding, we ran simple effects. As shown in Table 3, all the coding methods could differentiate honest from false accounts, but LIWC coding showed the largest estimate and significance. The estimates and t-values for manual coding and Text Inspector were almost similar.
Table 3. Simple effects for total details as a function of veracity and coding method
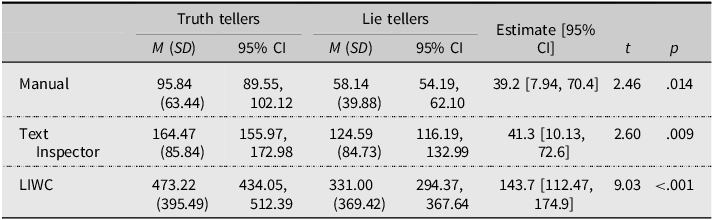
To test the model’s classification accuracy, we experimented with three classification methods: linear discriminant analysis, XGBoost, and random forests. We decided to use statistical and machine learning classification methods to understand if classification accuracy differs according to the learning model. We trained a different model for each coding method to ensure a fair comparison of the classification capability of each method and to remove any information leakage between the different methods if we train a joint model. We used 10-fold cross-validation to evaluate classification accuracy.
We ran separate analyses with and without the covariates (coding scheme, country, interview modality, and interpreter presence). We set veracity as the grouping variable and total details as the independent variable. The results are shown in Table 4. The analyses with and without the covariates showed similar results. The average accuracy rate across all three classification methods was highest for manual coding followed by Text Inspector and LIWC. Among the classification methods, the differences in accuracy were small, but the random forest classifier showed the best results (64.4%–65.3%).
Table 4. Classification accuracy for each coding method based on total details using linear discriminant analysis, XGBoost classifier, and random forest classifier
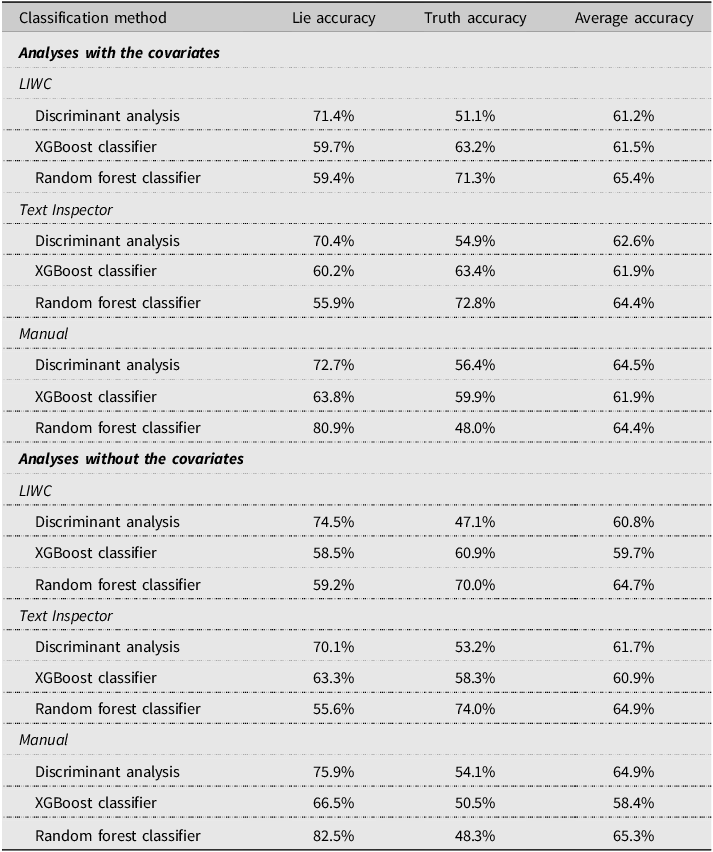
Note: The covariates are coding scheme, country, interview modality, and interpreter presence.
We evaluated the feature importance of the model using the random forest classifier which showed the highest accuracy. We trained a random forest using all the data (total details, coding method, coding scheme, country, interview modality, and interpreter presence) for each coding method separately and calculated the decrease in impurity within each decision tree. Table 5 shows that for all coding methods, total details was the most important feature followed by country.
Table 5. Feature importance of the model in Experiment 1
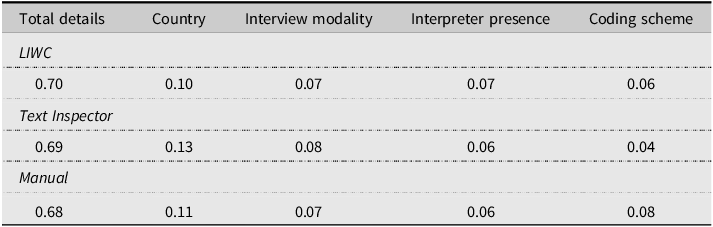
Discussion
We predicted that honest accounts will include more total details than false accounts, and this prediction was supported. The finding aligns with previous research showing that total details is a valid veracity cue and that a larger number of details is evident in honest accounts (Amado et al., Reference Amado, Arce, Farina and Vilarino2016; Colwell et al., Reference Colwell, Hiscock-Anisman, Memon, Taylor and Prewett2007). The mixed effects analysis also revealed that among all three coding methods, LIWC showed the highest estimates and differences between honest and false accounts. Text Inspector and manual coding showed comparable performance. We further found that all three coding methods could significantly classify truth tellers and lie tellers with very small differences between them. The LIWC classification accuracy rates were the lowest (62.7% in the analysis with the covariates) and those of manual coding were the highest (63.6% in the analysis with the covariates), but the three coding methods showed similar lie detection performance. Further, the feature importance model revealed that total details contributed substantially to the model compared to the covariates (country, interview modality, interpreter presence, and coding scheme) which corresponds with previous research showing total details to be a diagnostic verbal cue.
We also found that LIWC coding yielded the highest number of total details. This finding can be expected given that LIWC codes all words in an account (including redundant words), whereas Text Inspector and manual coding involve the coding of only unique (non-redundant) words. The overall findings suggest that researchers should consider the software program used when comparing manual and automated coding, but there is no coding method that can always be superior to the other.
Experiment 2
LIWC resulted in more pronounced veracity differences than Text Inspector and manual coding in Experiment 1. A main difference between LIWC and the other two coding methods is that LIWC counted redundant details, whereas the other two methods only counted nonredundant (unique) details. It could thus be that honest accounts included more redundant words than false accounts and that LIWC picked up this difference (although this difference did not seem to enhance lie detection accuracy). However, the data in Experiment 1 cannot inform us on whether honest accounts were more redundant, so we put this question to test in Experiment 2.
In previous research, redundancy was either examined under the construct of lexical diversity (i.e., unique words in text; e.g., Fuller et al., Reference Fuller, Biros, Burgoon and Nunamaker2013) or as a cue on its own (e.g., Chen et al., Reference Chen, Ita Levitan, Levine, Mandic and Hirschberg2020). The findings were generally inconsistent: Compared to false accounts, honest accounts were either more redundant (Burgoon, Reference Burgoon2018), less redundant (Davis et al., Reference Davis, Markus, Walters, Vorus and Connors2005; DePaulo et al., Reference DePaulo, Lindsay, Malone, Muhlenbruck, Charlton and Cooper2003; Hauch et al., Reference Hauch, Blandón-Gitlin, Masip and Sporer2015; Mbaziira & Jones, Reference Mbaziira and Jones2016; Zhou et al., Reference Zhou, Twitchell, Qin, Burgoon and Nunamaker2003), or equally redundant (Chen et al., Reference Chen, Ita Levitan, Levine, Mandic and Hirschberg2020; Dunbar et al., Reference Dunbar, Burgoon, Chen, Wang, Ge, Huang and Nunamaker2023; Duran et al., Reference Duran, Hall, McCarthy and McNamara2010; Zhou, Reference Zhou2005). One potential reason for this inconsistency is that redundancy was measured differently across experiments. Whereas some researchers computed it as the ratio of unique details to total details (Burgoon, Reference Burgoon2018; Dunbar et al., Reference Dunbar, Burgoon, Chen, Wang, Ge, Huang and Nunamaker2023), other researchers computed it as the ratio of total function words to total sentences (Zhou et al., Reference Zhou, Twitchell, Qin, Burgoon and Nunamaker2003), the total number of redundant consecutive words (Chen et al., Reference Chen, Ita Levitan, Levine, Mandic and Hirschberg2020), the total number of redundant nouns (Duran et al., Reference Duran, Hall, McCarthy and McNamara2010), the total number of redundant words in adjacent sentences (Davis et al., Reference Davis, Markus, Walters, Vorus and Connors2005), or the redundant words or phrases that are part of non-ah speech disturbances (DePaulo et al., Reference DePaulo, Lindsay, Malone, Muhlenbruck, Charlton and Cooper2003). This suggests some measurements were liberal (e.g., measuring all redundant components in text), whereas others were more restrictive (e.g., measuring only adjacent text for redundancy).
The inconsistent pattern of the findings can be explained by different theoretical frameworks. False accounts can become less redundant than honest accounts when lie tellers have prepared for their account (Dunbar et al., Reference Dunbar, Burgoon, Chen, Wang, Ge, Huang and Nunamaker2023) or are given time to interact with the interviewer (Zhou et al., Reference Zhou, Burgoon, Twitchell, Qin and Nunamaker2004). That would help lie tellers to report longer accounts than they would have otherwise done which increases diversity in their accounts. In contrast, truth tellers who take their credibility for granted do not usually prepare for the interview and would not intentionally plan a diverse account as lie tellers do (Chan & Bull, Reference Chan and Bull2014; Granhag & Hartwig, Reference Granhag and Hartwig2008; Vrij et al., Reference Vrij, Mann, Leal and Granhag2010).
Another argument for the inconsistent pattern of the findings is that false accounts can become more redundant than honest accounts, because lie tellers prefer to keep their accounts simple (Vrij et al., Reference Vrij, Mann, Leal and Granhag2010; Vrij, Granhag, et al., Reference Vrij, Granhag, Ashkenazi, Ganis, Leal and Fisher2022), so they tend to repeat information rather than add new information (Alison et al., Reference Alison, Alison, Noone, Elntib, Waring and Christiansen2014; Deeb et al., Reference Deeb, Vrij, Leal, Giorgianni, Hypšová and Mann2024). Further, in an interview, lie tellers produce information on a follow-along basis as the account develops because they fabricate rather than retrieve information from memory (Duran et al., Reference Duran, Hall, McCarthy and McNamara2010). Hence, there is less possibility of producing new information and thus lie tellers default to a more redundant account. It can also be argued that lie tellers do not have the creativity to improvise a text with diverse wording (Vrij et al., Reference Vrij, Palena, Leal and Caso2021). In contrast, truth tellers can demonstrate more lexical diversity, because they have experienced the event and information is retrieved from memory at the global level so new information is continuously developed (Duran et al., Reference Duran, Hall, McCarthy and McNamara2010). Thus, truth tellers can be more specific in their accounts by including more perceptual and contextual details (Masip et al., Reference Masip, Sporer, Garrido and Herrero2005) without having to use redundant words.
Given these conflicting theoretical explanations, we tested redundancy in Experiment 2. Based on the findings from Experiment 1, we expected honest accounts to be more redundant than false accounts. We also explored which types of words are the most redundant. If, for example, content words (i.e., core structures of a sentence such as nouns and verbs) are particularly redundant, then speakers may be more focused on the content (semantics) of the message. However, if function words (e.g., conjunctions, prepositions) are particularly redundant, the focus would mostly be on the grammatical structure (syntax) of the message.
Method
The same eight datasets as in Experiment 1 were used in Experiment 2. SpaCy software program (https://spacy.io/) was employed to count redundant words in text. SpaCY is a library for the Python programming language that analyses texts based on pretrained language pipelines. A SpaCy pipeline has multiple components which utilize a base artificial intelligence model for natural language processing (NLP) tasks such as part of speech tagging, named entity recognition, and lemmatization. The analyses were carried out with the SpaCy English language transformer pipeline based on the RoBERTa model, which is an enhanced model of the original BERT language model (Liu et al., Reference Liu, Ott, Goyal, Du, Joshi, Chen, Levy, Lewis, Zettlemoyer and Stoyanov2019).
The software tokenized each transcript into separate words. Punctuation marks and spaces were skipped. For each token, the software searched for the word lemma based on a set of rules and the word’s part of speech and dependency. Lemmatization is the process of reducing words to their normalized form by grouping together different inflected forms of the same word (Khyani et al., Reference Khyani, Siddhartha, Niveditha and Divya2021; Plisson et al., Reference Plisson, Lavrac and Mladenic2004). For example, in the context of going somewhere, “going” and “went” would both be lemmatized to “go” and allocated to the same group. Thus, where these three words are mentioned by the same participant in a single transcript, the software would count them as three redundant words.
Results
To account for the length of each transcript (see Schutte et al., Reference Schutte, Bogaard, Mac Giolla, Warmelink and Kleinberg2021), we computed a redundancy ratio score by dividing the total number of redundant words by the total number of words in each transcript. A mixed effects model revealed that there was no variability in the data, so we conducted a one-way univariate analysis of variance with veracity (honest, false) as factor, redundancy ratio as dependent variable, and datasets (all eight), country (Lebanon, Mexico, South Korea, United Kingdom), interview modality (face-to-face, online), and interpreter presence (present, not present) as covariates. A significant effect of veracity emerged, F(1, 781) = 30.58, p < .001,
$\eta 2$
= .04 (see Figure 2). Honest accounts (M = 0.68, SD = 0.09, 95% CI [0.67, 0.69]) were more redundant than false accounts (M = 0.64, SD = 0.10, 95% CI [0.63, 0.65]), d = 0.42 (95% CI [0.28, 0.56]). This result supported our hypothesis.
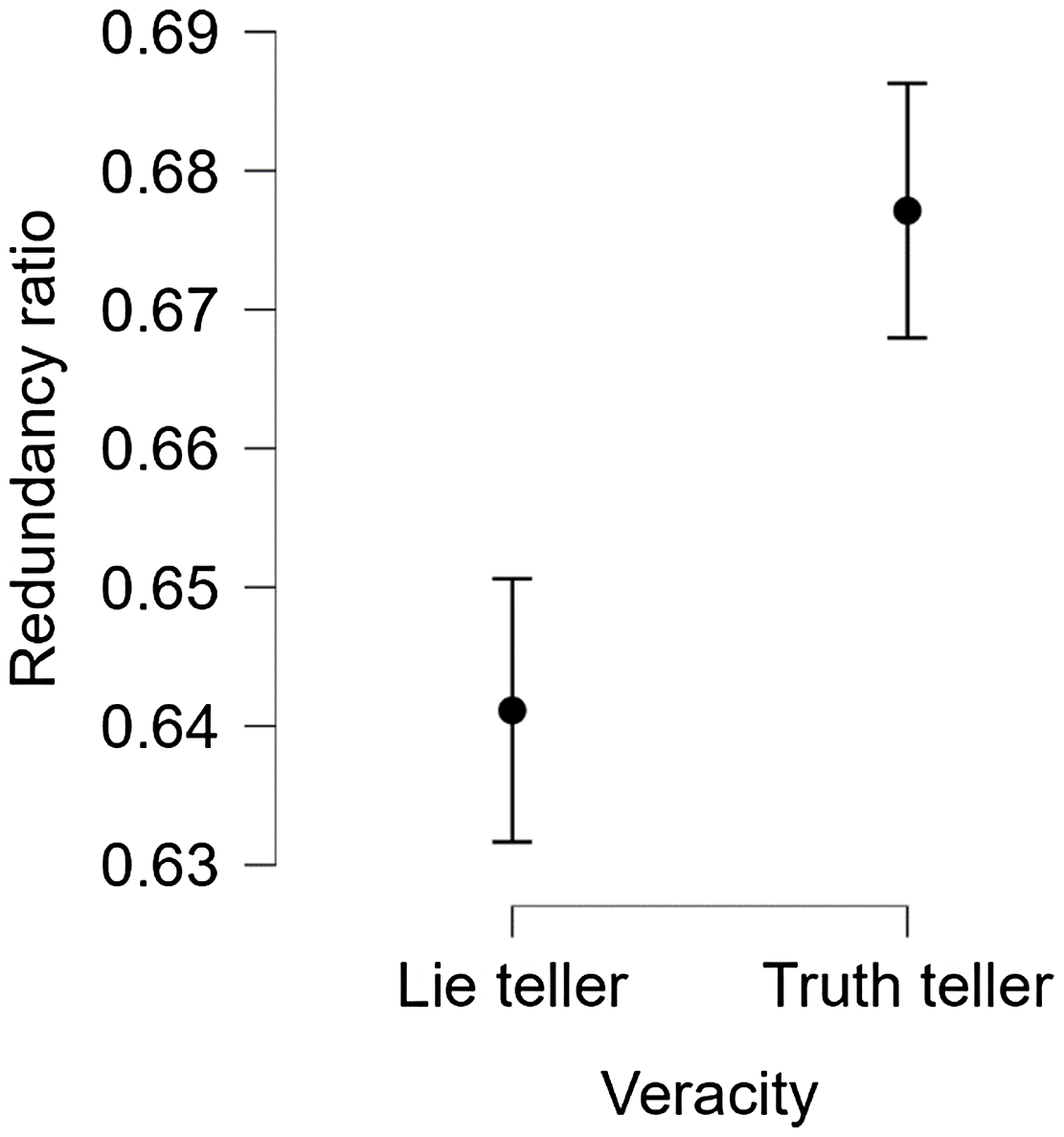
Figure 2. Means of the redundancy ratio as a function of veracity.
To explore which types of words were most redundant across participants, we further scrutinized the dataset. There were 3,233 redundant words with the most redundant word repeated 14,511 times. A total of 2,999 words were repeated less than 100 times, 200 words were repeated more than 100 times, and 34 words were repeated more than 1,000 times. We decided to analyze the 34 words that were repeated more than 1,000 times for two reasons. First, to have a better understanding of what types of words were most redundant, we needed to limit the number of interpreted words, and 34 words seemed enough for this purpose. Second, there was a significant gap in the times that these words were repeated. The least redundant word among these 34 words was repeated 1,146 times versus 14,511 times for the most redundant word. It thus made sense to include these 34 words rather than the words that were repeated less than 1,000 times as including the latter would further increase this gap.
We extracted the 34 most redundant words and subjected them to a t-test with veracity as factor. To control for multiple comparisons, we applied a strict p-value of less than .001 (two-sided). The redundant words that yielded significant differences between honest and false accounts are shown in Table 6. These redundant words are generally function words.
Table 6. T-test results for redundant words that significantly differentiated truth tellers and lie tellers
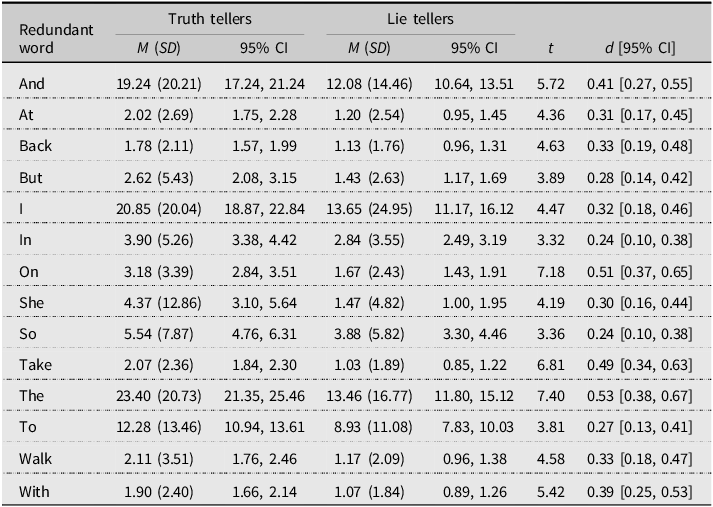
Note: For all redundant words, p < .001.
To explore if looking at the verbal cue redundancy enhances lie detection, we performed a discriminant analysis, XGBoost, and random forests with veracity as the grouping variable and redundancy ratio as the independent variable. We ran separate analyses with and without the covariates (country, interview modality, and interpreter presence). We used 10-fold cross-validation to evaluate classification accuracy. The results are shown in Table 7. The analyses with and without the covariates showed similar results. The average accuracy rate was highest for the discriminant analysis (59%) and lowest for the XGBoost classifier (51.4%-52.2%).
Table 7. Classification accuracy based on redundancy ratio using linear discriminant analysis, XGBoost classifier, and random forest classifier
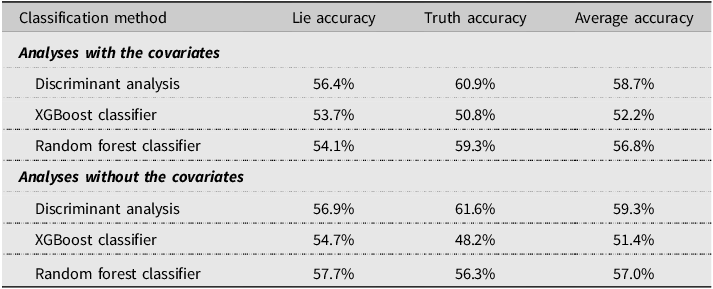
Note: The covariates are country, interview modality, and interpreter presence.
We evaluated the feature importance of the model using all the data (redundancy ratio, country, interview modality, and interpreter presence). Redundancy ratio was the most important feature (0.780) followed by country (0.107), interview modality (0.057), and interpreter presence (0.056).
Discussion
In line with our hypothesis, honest accounts were more redundant than false accounts, and this difference can be detected with approximately 59% accuracy. This finding suggests that accounting for redundancy in manual and automated coding can enhance detecting honest and false accounts. The most frequent redundant words were function words that do not have imperative lexical meaning. Function words are relevant to the grammatical structure of an account that make it look coherent (Afroz et al., Reference Afroz, Brennan and Greenstadt2012). Examples of function words include conjunctions (and, but), determiners (a, the), prepositions (on, at), personal pronouns (they, she), and modal verbs (should, might). While function words are not related to content, they are considered closely linked to psychological and social processes, including deceptive communication as they are less evident in false accounts than in honest accounts (Chung & Pennebaker, Reference Chung, Pennebaker and Fiedler2007).
There are several explanations for our findings. First, truth tellers provide more coherent, logical, and chronological accounts than lie tellers (Vrij, Reference Vrij2005). It thus makes sense that truth tellers would repeat function words to make the message flow and content more comprehensive to the interviewer (Zhou et al., Reference Zhou, Burgoon, Twitchell, Qin and Nunamaker2004). In contrast, lie tellers produce more ambiguous and vague accounts (DePaulo et al., Reference DePaulo, Lindsay, Malone, Muhlenbruck, Charlton and Cooper2003) so that their messages do not appear clear and/or coherent. This should result in fewer redundant function words that link sentences together.
Second, in the original experiments, lie tellers self-reported preparing for the interviews more than truth tellers. While lie tellers kept their accounts shorter and simpler as we found in Experiment 1, preparing for the interview may have enhanced their lexical diversity and helped them avoid appearing redundant (Dunbar et al., Reference Dunbar, Burgoon, Chen, Wang, Ge, Huang and Nunamaker2023). In contrast, truth tellers did not prepare for the interviews but reported from memory (Hartwig et al., Reference Hartwig, Granhag and Strömwall2007; Vrij et al., Reference Vrij, Leal, Granhag, Mann, Fisher, Hillman and Sperry2009). Truth tellers’ focus was thus on maintaining a continuous flow of communication for establishing a coherent account which requires the inclusion of redundant language (Amado et al., Reference Amado, Arce, Farina and Vilarino2016; Zhou et al., Reference Zhou, Burgoon, Twitchell, Qin and Nunamaker2004).
Third, Zhou et al. (Reference Zhou, Burgoon, Twitchell, Qin and Nunamaker2004) argued that lie tellers can become less redundant when they are given time to interact with the receiver of their message. Given that our datasets were collected via interviews which are considered rich media venues (Zhou, Reference Zhou2005), the level of interaction was high which may have allowed lie tellers to produce a more diverse account than they would in less rich mediums (e.g., emails; Zhou et al., Reference Zhou, Twitchell, Qin, Burgoon and Nunamaker2003).
General discussion
In Experiment 1, we showed that honest accounts were more detailed than false accounts. This finding is consistent with previous meta-analyses that found that compared to other verbal cues, total details was the most diagnostic cue with the largest magnitude (Amado et al., Reference Amado, Arce, Farina and Vilarino2016; DePaulo et al., Reference DePaulo, Lindsay, Malone, Muhlenbruck, Charlton and Cooper2003; Gancedo et al., Reference Gancedo, Fariña, Seijo, Vilariño and Arce2021). Given that we reached the same conclusion using a large number of participants, this increases our confidence in total details as a diagnostic cue (Lakens & Evers, Reference Lakens and Evers2014).
The theoretical approaches to deception, namely the information management approach, the cognitive approach, and the RM approach, can shed light unto this finding. Lie telling is a cognitively demanding task given that lie tellers typically have to fabricate at least some of the reported information (Vrij, Reference Vrij2008). At the same time, lie tellers want to appear cooperative and convincing so that their lie is believed (Granhag et al., Reference Granhag, Vrij and Verschuere2015). This leads to lie tellers calculating what information they should disclose and what information they should avoid reporting. They thus strive to provide accounts that are simple to reduce cognitive demands and to avoid potentially contradicting themselves (Deeb et al., Reference Deeb, Vrij, Leal, Giorgianni, Hypšová and Mann2024; Vrij et al., Reference Vrij, Fisher and Blank2017). Ultimately, lie tellers provide fewer details than truth tellers.
We found that the automated coding of total details can capture differences between truth tellers and lie tellers to a larger extent than manual coding, although this may depend on the software program used. More specifically, LIWC seemed to detect these differences more than Text Inspector. This finding aligns with previous research demonstrating that various software programs perform differently which can affect lie detection (Ceballos Delgado et al., Reference Ceballos Delgado, Glisson, Shashidhar, McDonald, Grispos and Benton2021; Kleinberg et al., Reference Kleinberg, Nahari, Arntz and Verschuere2017). In Experiment 2, we showed that LIWC’s performance may have been driven by its analysis of redundant words in text which makes it more sensitive for detecting differences between honest and false accounts. These findings suggest that it may be possible for software programs other than LIWC that also account for redundancy to effectively differentiate honest and false accounts. This question can be put to test by future research.
While LIWC showed larger veracity differences in reported details than Text Inspector and manual coding, the classification accuracy rate of the three coding methods were similar and all in the 60%–65% range. We expect the manual coding classification rate to increase up to 75% when human judges are asked to specifically look for total details. We base this prediction on a previous meta-analysis which has shown that when judges are asked to look for specific verbal cues that received empirical evidence, lie detection accuracy increases (Mac Giolla & Luke, Reference Mac Giolla and Luke2021).
In Experiment 2, honest accounts were more redundant than false accounts and the veracity groups could be accurately classified via automated coding based on this verbal cue. While the direction of the differences between honest and false accounts on redundancy contradicts some previous research (DePaulo et al., Reference DePaulo, Lindsay, Malone, Muhlenbruck, Charlton and Cooper2003; Mbaziira & Jones, Reference Mbaziira and Jones2016; Zhou et al., Reference Zhou, Twitchell, Qin, Burgoon and Nunamaker2003), it is consistent with other findings (Burgoon, Reference Burgoon2018; Zhou et al., Reference Zhou, Burgoon, Twitchell, Qin and Nunamaker2004). The nonconverging findings are likely the result of the redundancy cue being measured differently across experiments. In the present research, we used a simplified measure of redundancy that takes into account repeated lemmatizations in text and that accounts for the length of the account by calculating a percentage score (see Schutte et al., Reference Schutte, Bogaard, Mac Giolla, Warmelink and Kleinberg2021).
Also unlike previous research on redundancy, all included datasets were rich media venues (oral interviews) that were conducted in a forensic context. Thus, at least in forensic interview contexts where free recalls are requested, redundancy may be diagnostic of truth telling. However, we have to be cautious when interpreting these findings as the effect size was medium. While such an effect size is practically significant for lie detection, a large effect size is usually preferred because it would make the veracity differences very noticeable to the naked eye (Cohen, Reference Cohen1992). These results can be ameliorated if interviewers look at multiple verbal cues at the same time (Deeb et al., Reference Deeb, Vrij, Leal, Giorgianni, Hypšová and Mann2024; Hartwig & Bond, Reference Hartwig and Bond2011; Vrij, Hartwig, et al., Reference Vrij, Hartwig and Granhag2019). Based on the present results, and as total details and redundancy could accurately classify honest and false accounts above chance levels, interviewers can look at both verbal cues to enhance lie detection accuracy.
We specifically found that the most redundant words were function words. While function words constitute less than 0.04% of the English vocabulary, they account for half of the words used in daily communications (Chung & Pennebaker, Reference Chung, Pennebaker and Fiedler2007). It is reassuring to find that words referring to syntax can be diagnostic similar to words referring to semantics (Afroz et al., Reference Afroz, Brennan and Greenstadt2012; Newman et al., Reference Newman, Pennebaker, Berry and Richards2003). However, we cannot infer from the data why function words were repeated more than content words. Future research can examine this through the use of metacognitive questions, whereby truth tellers and lie tellers are asked how they think about and use function words in their preparations and in their actual accounts.
Limitations and future research implications
We analyzed data from free recall (control) questions only. That meant that we only used passive interview protocols to reach our conclusions (Vrij, Reference Vrij2008). We did not code how honest and deceptive language changes as a function of asking different questions. We wanted to standardize the analyses and see how truth tellers and lie tellers respond to questions in a neutral context (i.e., when the interviewer is not actively asking questions that would increase differences between truth tellers and lie tellers). In the original experiments, the experimental interview questions (e.g., Model Statement; sketching while narrating) yielded more significant differences between honest and false accounts than the free recall question. We would thus expect more veracity differences to emerge when the interview protocol is manipulated. Future research can compare manual and automated coding on passive (free recall) versus active (experimental) questions.
Relevant to the above, our analyses are based on responses to one free recall question. In real life, interviews are usually longer and involve more specific questions (Griffiths & Milne, Reference Griffiths, Milne and Williamson2006; Oxburgh et al., Reference Oxburgh, Myklebust and Grant2010). There are also instances where a suspect may refuse to respond to questions (Moston et al., Reference Moston, Stephenson and Williamson1992). Thus, our results cannot generalize to all contexts and are limited to free recalls. We encourage researchers to compare manual and automated coding on other types of questions such as probing questions (Hartwig et al., Reference Hartwig, Granhag, Stromwall, Wolf, Vrij and Hjelmsäter2011).
The analyses were limited to eight datasets collected in the same lab. Our research questions can be tested on more datasets by different labs and also in different countries. While our research involved the recruitment of participants from non-WEIRD countries, it is fundamental to recruit participants from different cultures as that may yield different results (Leal, Vrij, Vernham, et al., 2019; Taylor et al., Reference Taylor, Larner, Conchie and Menacere2017). Further, automated coding has yet to be tested on datasets in real-life forensic interviews where stakes are usually higher and may differ from stakes in laboratory settings. Whereas some research suggests that higher stakes affect differences in honest and false accounts (ten Brinke & Porter, Reference ten Brinke, Porter, Cooper, Griesel and Ternes2013), a meta-analysis showed null effects (Hartwig & Bond, Reference Hartwig and Bond2014). It is worth examining if and how suspects would change their language when they know that an automated system will be used to assess their accounts.
We compared manual and automated coding on one veracity cue (total details) in Experiment 1. Other cues that reflect richness within an account (e.g., person details, location details) can also be assessed. LIWC does not code these details in the same manner as manual coding. For example, pronouns, names, and people descriptions are coded as person details in PLATO manual coding schemes, but LIWC has different categories related to people (e.g., pronouns, social processes, body parts, etc.). The coding process would become subjective if the researcher has to decide on which LIWC categories to include under “person details” in the analysis. Other sophisticated software programs may be more appropriate for coding these details. For example, SpaCy can code “person” entities in a manner that is comparable to manual coding and can also account for redundant and non-redundant entities. When a software program already has a specific entity (category), researchers from different labs can use that same entity which creates a more standardized coding scheme across experiments and allows for a more proper comparison between outputs (Nahari & Vrij, Reference Nahari and Vrij2015). Such software programs may also result in a higher accuracy rate than the more commonly used LIWC (Duran et al., Reference Duran, Hall, McCarthy and McNamara2010; Kleinberg, Mozes, et al., Reference Kleinberg, Mozes, Arntz and Verschuere2018; Kleinberg et al., Reference Kleinberg, Nahari, Arntz and Verschuere2017).
We further encourage the testing of other stylometric features. A major advantage of automated coding is that it allows for more sophisticated coding (e.g., by examining patterns in language or by coding multiple cues simultaneously) that humans are not capable of doing (Chung & Pennebaker, Reference Chung, Pennebaker and Fiedler2007; Hauch et al., Reference Hauch, Blandón-Gitlin, Masip and Sporer2015). Future research can look at features that were not widely examined in automated lie detection research but that have shown promising results, including sentence structure (Dykstra et al., Reference Dykstra, Lyon and Evans2022), average sentence and word length (Afroz et al., Reference Afroz, Brennan and Greenstadt2012; Zhou et al., Reference Zhou, Burgoon, Twitchell, Qin and Nunamaker2004), and word concreteness (Kleinberg et al., Reference Kleinberg, van der Vegt and Arntz2019).
We found that automated coding can differentiate honest and false accounts on the verbal cues total details and redundancy. While we reported the advantages of automated coding and while we acknowledge that many advancements have been incorporated on coding software programs to enhance lie detection, automated coding has its own limitations (Tomas et al., Reference Tomas, Dodier and Demarchi2022). First, although automated coding can examine content to a certain extent (such as words with similar meanings), it cannot accurately capture the context of an account such as its plausibility and predictability which may explain the conflicting results between different software programs (Hauch et al., Reference Hauch, Blandón-Gitlin, Masip and Sporer2015; Mann et al., Reference Mann, Vrij, Deeb and Leal2023). Second, it cannot differentiate words used in different contexts (Chung & Pennebaker, Reference Chung, Pennebaker and Fiedler2007). For example, the word “lie” has different meanings in “She is lying to me” versus “She is lying on the floor.” Third, while automated coding is more objective than manual coding, it is still subjective as different software programs include different libraries and dictionaries which varies their lie detection accuracy. Fourth, while automated coding has been recommended as an objective alternative to manual coding, it can still be biased as it was originally developed by humans and the output often requires human interpretation which is often bias- and error-prone (Jupe & Keatley, Reference Jupe and Keatley2020; Kassin et al., Reference Kassin, Dror and Kukucka2013). Fifth, overreliance on automation can lead to erroneous decision-making. In applied forensic settings, interviewers may start basing their decisions solely on the automation output rather than on the overall evidence they have acquired which may lead to guilty suspects being judged as innocent or vice versa (Kleinberg & Verschuere, Reference Kleinberg and Verschuere2021; Tomas et al., Reference Tomas, Dodier and Demarchi2022). Sixth, automated coding cannot be used in all contexts and at all times. For example, patrol officers who interview people in the field or on the spot do not have access to computerized venues, Also, in combat and military contexts, automated coding software may not be accessible.
Conclusions
The replicability crisis has taken its toll on the psychology field, so it is important to standardize procedures that yield robust and replicable results (Pashler & Wagenmakers, Reference Pashler and Wagenmakers2012; Tomas et al., Reference Tomas, Dodier and Demarchi2022). For lie detection research, coding is a very important aspect of assessing accounts, and the subjectivity in coding which in many cases yields low inter-rater reliability scores is an obstacle for replicable results. Thus, automated coding has been suggested as a solution to this problem while at the same time allowing for a faster assessment of accounts than manual coding.
In the present research, we showed that automated software programs can indeed detect differences between honest and false free recalls on total details and redundant details, but the extent to which these differences are captured varies depending on the program used. In addition, automated coding performance was similar to manual coding when classifying truths and lies, at least in the tested context. The overall results thus implicate that both manual and automated coding could be implemented for lie detection purposes. Where time resources are limited, technology that automatically transcribes an interviewee’s free recall, coupled with automated coding of total details and redundancy, can be used.
Replication package
This article was co-authored by Gerges Dib in his personal capacity. The views expressed in this article are his own and do not necessarily reflect those of Amazon.com, Inc.
The material, data, and analyses are available in the repository of the University of Portsmouth at https://doi.org/10.17029/73bf0f42-b599-4c36-81b7-0c87befb795f.
Competing interests
The authors declare none.