Econometrics occupies an influential place in the study of political economy. Common arguments put forward are that econometrics is more rigorous than qualitative approaches, uses “objective” data, and yields clearer, quantifiable results about relationships and effects. Yet, in practice, econometric research in many areas of the social sciences struggles to offer definite answers to important policy questions.
We illustrate this problem using the case of social policy in Latin America. This is a central area of concern for both social scientists and policymakers. From an analytical perspective, the evolution of social policy tells us much about how societies operate and how they resolve class struggles. From a policy perspective, the level and allocation of social spending has major implications for poverty, inequality, and human development more generally.
Following previous studies in OECD countries, the literature on Latin America has explored the role of economic and political drivers in determining social spending in the region. Different studies have rightly emphasized the importance of democracy and the need to consider social insurance, social assistance, and health care separately. Yet, overall, econometric results have been inconclusive: for example, leftist parties, trade openness, or urbanization have a positive, neutral, or negative effect on social spending, depending on the studies.
The fact that different papers using different data and methods produce ambivalent answers is not surprising: it is what motivates new studies on the same topic in the first place. We should obviously expect variation in research design and methodology to lead to a diversity of results. However, while some differences in results should be expected as a consequence of research design and methodology, the lack of significant agreement and the existence of important contradictions should not. Like Bird (Reference Bird2007), we understand scientific progress in the following simple terms: after some time of research, there should be more common knowledge and understanding than before. This does not currently seem the case in the study of the determinants of public social spending in Latin America. Hence we need to understand better where differences come from and why different studies do not converge to clear understandings. To promote knowledge accumulation, research fields should find a fair balance between promoting research creativity and avoiding unnecessary differences in research designs.
Econometric research practices in applied fields have been criticized before.Footnote 1 In contrast to these contributions, we do not focus on providing a detailed critique of specific practices or proposing better alternatives. Rather, our goal is to suggest reasons for inconclusive quantitative evidence, where econometric mistakes are only one aspect of the story. In many instances, even when econometric tools are used in a technically and theoretically satisfactory way, there remains scope for ambiguities. We hope to enable a better understanding of some limitations of quantitative contributions in applied political economy research, leading to constructive discussions about ways forward.
To do so, we review the most relevant articles published in the last two decades on the determinants of social spending in Latin America. We argue that data limitations and a variety of econometric problems related to panel studies (e.g., unobserved country characteristics, dependencies across units and time, endogeneity issues) have forced authors to make a series of technical decisions with both analytical and empirical implications. As a result, comparing results across studies and building consensus have proven particularly hard.
We focus on three kinds of decisions. First, we highlight two methodological choices with analytical implications: whether to use levels of social spending or rates of change, and whether to study differences between countries or within each country over time. Second, we consider problems with data—including levels of government used and the periods considered—and the different ways in which they have been resolved. Third, we also discuss more complex econometric problems (e.g., model specification, the characteristics of the error terms and endogeneity) and the biases that different choices can introduce in the results.
Given the limitations of econometric analysis, at the end of the article we call for the complementary use of qualitative and quantitative research. This goal goes beyond the use of mixed methods—a technique several of the authors studying social policy in Latin America have already done (e.g., Huber and Stephens Reference Huber and Stephens2012; Kaufman and Segura-Ubiergo Reference Kaufman and Segura-Ubiergo2001)—and refers to the importance of having more conversations between qualitative and quantitative researchers about theory, data, and the causal stories we want to develop.
What we know about determinants of public social spending in Latin America
The study of social policy occupies a central role in political economy (Amenta Reference Amenta, Mahoney and Rueschemeyer2003). As Doyle (Reference Doyle2018, 1) puts it, “given that distributional battles lie at the heart of politics, it is perhaps not a great surprise that one of the most researched areas of political science involves work on social policy and social spending.” How much different countries spend on social policy and how they shape their welfare states tells us much about their institutions, class balance, and economic prospects (Amenta Reference Amenta, Mahoney and Rueschemeyer2003; Mkandawire Reference Mkandawire2006). The level (and composition) of social spending is also fundamental for poverty reduction and the promotion of more equitable societies. Given the region’s high levels of inequality, reaching agreements on the determinants of social policy and identifying the best ways to expand social spending in the future is particularly important in the Latin American context (Sánchez-Ancochea Reference Sánchez-Ancochea2020).
The literature on the determinants of social policy first developed in the context of developed countries and emphasized the role of economic liberalization—inspired by Karl Polanyi’s ideas on protective countermovements in The Great Transformation—as well as trade unions and political parties (e.g., Cameron Reference Cameron1978; Garrett Reference Garrett2001; Hicks and Swank Reference Hicks and Swank1992; Katzenstein Reference Katzenstein1985; Korpi Reference Korpi1978). In the mid-1990s, this literature was expanded to consider the contradictory impacts of globalization.
As a result of successive waves of research, we can identify three main hypotheses: the trade and globalization hypothesis, the modernization hypothesis, and the politics hypothesis. After finding robust and positive partial correlations between trade openness and government expenditure, Rodrik (Reference Rodrik1998) stimulated an intensive debate on the relationship between the two. In his view, government expenditure is a risk compensation mechanism for citizens in open economies (compensation hypothesis), an argument that seems particularly valid for OECD countries (Doyle Reference Doyle2018). In contrast, Garrett (Reference Garrett2001) argued that when capital mobility is large, increases in trade could lead to pressures for a smaller rather than a bigger government (efficiency hypothesis). According to Wibbels (Reference Wibbels2006), the efficiency hypothesis may be particularly relevant to developing countries, which are capital-constrained and face more obstacles to borrowing. While these initial contributions were concerned with overall government expenditure, the debate later focused on social spending and its components.
The modernization hypothesis assumes a positive link between the level of social spending and GDP per capita. As countries become more developed, both social demands and social needs increase, thus resulting in the expansion of social services. This hypothesis, developed around Wagner’s Law on public spending, has received attention in the context of OECD countries (see, e.g., Williamson and Fleming Reference Williamson and Fleming1977). Drawing on this argument, some authors have also argued that a growing urban population may drive higher spending, as urbanization comes together with industrialization and labor organization (Avelino, Brown, and Hunter Reference Avelino, Brown and Hunter2005).Footnote 2
Various studies highlight the role of political institutions and party ideology. The literature on OECD countries focused on the contributions of left-wing parties, trade unions, and various institutional arrangements to higher social spending (Hicks and Swank Reference Hicks and Swank1992; Huber, Ragin, and Stephens Reference Huber, Ragin and Stephens1993; Huber and Stephens Reference Huber, Stephens and Pierson2001). There was less attention to the role of democracy because it was considered “the only game in town” in the developed world.
Since the early 2000s, the study of the determinants of social spending has extended to Latin America. The region constitutes an excellent case for researchers interested in “building on extant theory and developing mid-range theories of welfare state development across regions” (Huber, Mustillo, and Stephens Reference Huber, Mustillo and Stephens2008, 420). It has stronger welfare institutions and higher levels of spending than other developing countries. At the same time, it is poorer and more institutionally diverse than the United States or Europe. There are thus reasons to treat social policy in Latin America as a unique subject of research with its own explanatory variables (Doyle Reference Doyle2018).
For this exploration, we juxtapose the results obtained by six studies. The selection criteria were as follows. We aimed for articles that study the determinants of public social spending in Latin American in light of the three main hypotheses—the trade and globalization hypothesis, the modernization hypothesis, and the politics hypothesis—and that were published after 2000. Studies before the 2000s are less sophisticated econometrically and have more data limitations and were therefore excluded. Further, because we want to evaluate papers that “talk to each other” explicitly, we excluded research that focuses on other political economy variables and processes, such as Doyle’s (Reference Doyle2015) excellent work on the impact of remittances. We decided to focus on Latin America exclusively and avoid cross-national studies (such as Haggard and Kaufman Reference Haggard and Kaufman2004 or Wibbels Reference Wibbels2006) because some of the determinants of social policy are likely to be region-specific, depending on particular histories, institutions, and economic models. This decision allowed us to focus on a smaller number of studies, making comparison easier. Finally, we did not include two influential books (Huber and Stephens Reference Huber and Stephens2012; Segura-Ubiergo Reference Segura-Ubiergo2007) because their econometric chapters are extensions of two of the articles we review. To the best of our knowledge, there exist six original articles that fulfill our criteria (see table 1). In any event, our aim is not to provide a full review of all this literature but to illustrate a number of problems arising in it, using these articles as an illustration. There is no reason to assume that including additional articles would change our evaluation.
Table 1. Countries and years analyzed in reviewed studies.
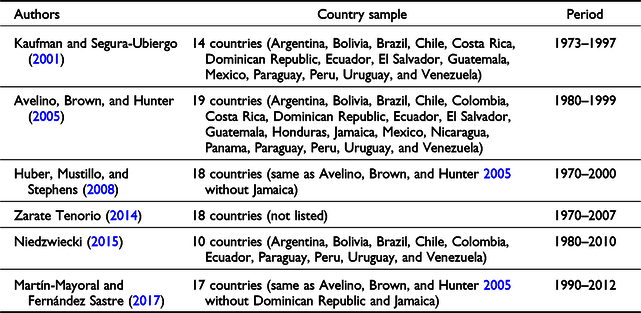
Trade and globalization
Much of the global cross-country literature argues in favor of the efficiency hypothesis, finding that trade and capital account liberalization reduce a country’s ability to tax and spend (Wibbels Reference Wibbels2006; Wibbels and Arce Reference Wibbels and Arce2003). Several studies on Latin America confirm this emerging consensus. For example, Kaufman and Segura-Ubiergo (Reference Kaufman and Segura-Ubiergo2001) find that trade openness has a negative impact on total social spending and social security but no effect on health and education. Niedzwiecki (Reference Niedzwiecki2015) also finds negative effects.
Yet this result is by no means undisputed. In fact, Avelino, Brown, and Hunter (Reference Avelino, Brown and Hunter2005) find a positive effect on social insurance and education, but not on health. Huber, Mustillo, and Stephens (Reference Huber, Mustillo and Stephens2008) find no statistically significant links between various types of social spending and trade openness. Zarate Tenorio (Reference Tenorio2014) confirms that neither levels nor changes of trade openness are related to public social spending. The evidence thus tends to confirm the negative impact of globalization—a result that also make sense theoretically given Latin America’s high level of dependence—but even here there are doubts.
Modernization
The evidence on the significance of the modernization hypothesis in the Latin American context is mixed. The impact of GDP per capita on social spending is contradictory and inconsistent across studies. For example, in Huber, Mustillo, and Stephens (Reference Huber, Mustillo and Stephens2008), GDP per capita has a positive but small impact on health and education, but none on overall social security and welfare—opposite to what Zarate Tenorio (Reference Tenorio2014) finds. For social security spending, results vary from no statistical significance to positive and even negative impacts. In the latter case, some differences may stem from different foci: for example, negative relationships over time (Avelino, Brown, and Hunter Reference Avelino, Brown and Hunter2005; Niedzwiecki Reference Niedzwiecki2015) do not contradict the absence of a positive relationship in cross-country comparison. But contradictions still remain. Two studies estimate levels and changes separately and still find opposite results (Kaufman and Segura-Ubiergo Reference Kaufman and Segura-Ubiergo2001; Zarate Tenorio Reference Tenorio2014).
Politics
In contrast to the literature on the OECD, Latin Americanists pay particular attention to the role of democracy, which is expected to exert a positive role in social policy (Martínez Franzoni and Sánchez-Ancochea Reference Martínez Franzoni and Sánchez-Ancochea2016). Elections force political parties to compete for votes among poor and middle-income groups, most of which benefit from higher spending. Democratic institutions also open new space for social contestation and social demands through mass media.
In the reviewed studies, democracy has a positive effect on the level of social spending but not on changes from year to year. This is evident when comparing, for example, Huber, Mustillo, and Stephens (Reference Huber, Mustillo and Stephens2008) with Kaufman and Segura-Ubiergo (Reference Kaufman and Segura-Ubiergo2001). The first study focuses on explaining the levels of social spending as percentage of GDP and finds that the number of accumulated years of democracy is positively related to spending in social insurance and health plus education, a result confirmed by Niedzwiecki (Reference Niedzwiecki2015), as far as within-country developments are concerned. In contrast, Kaufman and Segura-Ubiergo (Reference Kaufman and Segura-Ubiergo2001)—like Zarate Tenorio (Reference Tenorio2014) and Martín-Mayoral and Fernández Sastre (Reference Martín-Mayoral and Fernández Sastre2017)—find no impact or even negative effects of democracy on social spending, depending on specifications.
All these econometric works on Latin America make useful references to each other and provide new understandings of the determinants of social spending. Authors read, cite, and engage with previous works. Yet the accumulation of knowledge—important to devise future research agendas and make policy recommendations—has been limited. As reflected in table 2, the six studies provided a diversity of interesting results, but few clear consensuses.
Table 2. Overview of results of six different studies.
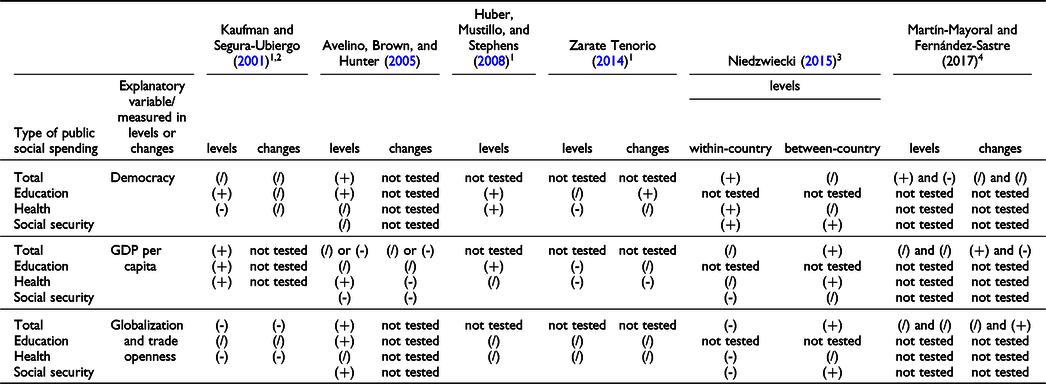
Results: (+) = positive and statistically significant coefficient; (-) = negative and statistically significant coefficient; (/) = no statistically significant coefficient.
1 Health and education spending estimated jointly.
2 Results are reported where spending per capita is the dependent variable.
3 Reported results are those that use separate estimators for within-country and between-country variance (Niedzwiecki Reference Niedzwiecki2015, 23, table 8).
4 Results reported are from system GMM estimator. Results refer to two different periods that were analyzed comparatively: 1990–2000 and 2000–2012.
This body of research constitutes a clear example of our broader claim about the problems of applied econometric research to provide definite conclusions. In the rest of the article we try to explain why the results are contradictory. Some contradictions can be explained by differences in key analytical decisions, which are not always sufficiently acknowledged. Other differences are related to data. A final set of factors has to do with the econometric techniques used.
Diversity of analytical approaches
Let us begin with two kinds of decisions that are often discussed in technical terms but have significant analytical implications. First, the determinants of rates of change in social spending are likely different from those affecting levels of spending. It is thus not surprising that studies that use one or the other end up with different results. Second, the use of country fixed effects (FE), which has become standard to account for unobserved heterogeneity between countries, also affects what researchers analyze and should have more influence on the way they interpret their results.
Levels versus changes
Some of the studies reviewed here focus on the level of social spending, while others concentrate on changes over time. Likewise, several independent variables are sometimes used in levels and other times in changes. The decision about levels or changes is often presented in technical terms, that is, as a way to solve various econometric problems such as serial correlations in the error terms (Martín-Mayoral and Fernández Sastre Reference Martín-Mayoral and Fernández Sastre2017), while theoretical implications are often only superficially explored. However, the use of levels or changes implies different research questions, the answers to which are not easily compared.
Spending levels tell us something about the level of public welfare and allow comparisons across countries or with other types of government spending. Researchers might be interested in understanding how, for example, a particular shock (such as a commodity boom) affects year-to-year changes in public social spending. Unfortunately, these differences are often not considered, and levels or changes are sometimes mixed up. For example, Kaufman and Segura-Ubiergo (Reference Kaufman and Segura-Ubiergo2001, 557) discuss the efficiency and compensation hypothesis of globalization in terms of levels, arguing that “in OECD countries this hypothesis is supported by studies that show a very strong empirical association between economic openness, large public sectors, and generous welfare systems.” Their assumption is thus that more open countries spend more on social spending, which is quite different than saying that opening up the economy will lead to year-to-year expansion of social programs. Yet they then estimate a model where the dependent variable is changes in social spending.
There is a similar problem in the case of the independent variables: Should we assume that the variance of social spending across countries is determined by the same variables than changes across time? The answer is obviously no. As Huber, Mustillo, and Stephens (Reference Huber, Mustillo and Stephens2008, 421) explain for the case of political variables, “we would not expect one year of democracy or of dominance of one political tendency or another in the legislature and/or the executive to make a major difference in the formation of social policy.” There are no reasons to assume that an improvement in the quality of democracy will result in an immediate expansion of social spending. More competitive elections or better electoral tribunals will lead to more attention to voters’ preference and can result in more redistributive policies, but the process will take years to materialize. Equally, GDP per capita is likely to affect social spending over the long run (because the amount spent by the government will depend on the overall resources available), but its influence on annual changes is less clear. Economic growth may have a larger effect in changes in social spending than in its level. As a result, the various studies reviewed are less comparable than initially thought: small changes in the equation used (e.g., introducing differences) can have major implications in the results and in their interpretation.
Differences between and within countries
To account for unobservable country-level characteristics and avoid biased estimators, most studies introduce country FE. As the term fixed effects is understood differently in different contexts (Wooldridge Reference Wooldridge2010; Kropko and Kubinec Reference Kropko and Kubinec2020), we clarify that we use it to refer to a model with case-specific intercepts. Such a model can be thought of as containing dummy variables for each country. Coefficients estimated using country FE reflect the over-time impact of each independent variable averaged across countries. The estimator can be derived via the means-centering approach (e.g., Wooldridge Reference Wooldridge2010) or the (equivalent) data subsetting approach (Kropko and Kubinec Reference Kropko and Kubinec2020), both of which lend themselves to intuitive exposition. In the former, the FE estimator subtracts the mean across observations within each country so that the remaining variation comes only from variations within each country over time. In the latter, the regression is first performed country-wise (i.e., all variation takes place over time), before the FE coefficient is calculated as a weighted average of all country-specific coefficients. Both approaches imply that the results of regression analysis with country FE should be interpreted as the effect of changes in the explanatory variable on the dependent variable within countries over time. In contrast, they tell us nothing about differences in social policy between countries, because this dimension of the variation in the cross-sectional data has been removed.Footnote 3
The decision to implement country FE is most often driven by the desire to address unobserved heterogeneity in panel data (see also next section), and not by the authors’ wish to concentrate their analysis on within-country developments.Footnote 4 For example, in discussing the role of political regimes, Martín-Mayoral and Fernández Sastre (Reference Martín-Mayoral and Fernández Sastre2017, 7) wonder “whether authoritarian or democratic regimes have different levels of social spending,” even though their analysis will not allow them to say anything about this. Avelino, Brown, and Hunter (Reference Avelino, Brown and Hunter2005, 628) present their hypotheses in a similar way, as comparative statements about different countries at a certain moment in time, which cannot be evaluated through an econometric technique that considers changes within countries over time.
Diversity of sources
The second set of factors that we deem responsible for diverse and partly contradictory results of the literature refers to data. Here we focus our discussion on the dependent variable but also make a few remarks about independent variables.
Data sources and measurement: Public social spending
Some of the differences in the results may be due to the use of different data sources. Avelino, Brown, and Hunter (Reference Avelino, Brown and Hunter2005) and Martín-Mayoral and Fernández Sastre (Reference Martín-Mayoral and Fernández Sastre2017) use Economic Commission of Latin America and the Caribbean (ECLAC) data, while Kaufman and Segura-Ubiergo (Reference Kaufman and Segura-Ubiergo2001) rely on information from the International Monetary Fund (IMF). Zarate Tenorio (Reference Tenorio2014) and Niedzwiecki (Reference Niedzwiecki2015) use the Latin American Welfare Dataset elaborated by Evelyne Huber and John D. Stephens, which is currently available in its 2014 version.Footnote 5 This database combines data for social security and welfare spending, and education and health spending, from different ECLAC and IMF sources.Footnote 6
The level of government included in each database is different. As Kaufman and Segura-Ubiergo (Reference Kaufman and Segura-Ubiergo2001) indicate, the IMF data is available at central government level only. As to ECLAC data, levels of coverage have changed over time. ECLAC statistics for the period 1980–1990 (based on Cominetti and Ruiz Reference Cominetti and Ruiz1998) covers spending by the central government, with a few exceptions. Brazil is the only country where general government spending is available, while the cases of Argentina and El Salvador include nonfinancial public-sector spending (Cominetti and Ruiz Reference Cominetti and Ruiz1998, 24–25).
Since 1991, ECLAC data appears in its annual flagship report, Social Panorama of Latin America, and the institutional coverage still varies by country. Bolivia (1996–2014), Brazil (2000–2015), Colombia (2009–2015), Cuba (1996–2015), and Peru (1999–2015) only report information for the central government. Nonfinancial public-sector spending, which comprises spending for the central government, subnational governments, and nonfinancial public corporations, is available for El Salvador (2002–2015) and Mexico (1990–2015); public-sector spending is available for Costa Rica (1987–2015) and Peru (1999–2015).
The use of central government spending is particularly problematic in countries with federal structures, where social spending is decentralized. In Brazil, for example, the federal government spent less than 60 percent of public social spending, while state governments and municipalities were responsible for 23 percent and 20 percent, respectively (ECLAC 2006, 127). Additionally, since the beginning of our period of study, many Latin American countries have undergone decentralization reforms. As a result, even in nonfederal countries like Bolivia or Colombia, subnational governments account for over 70 percent of public spending in education and about 50 percent in health (Brosio and Jiménez Reference Brosio, Jiménez, Brosio and Juan2012). Not surprisingly, using data from different levels of government can lead to erroneous conclusions (Martínez and Paz Collinao Reference Martínez and Paz Collinao2010, 26).
Figure 1 illustrates the differences in the values of spending and its change over time for countries for which more than one level is available from ECLAC data. For example, in Argentina there was a drop in nonfinancial public-sector spending around 2007, but no changes in central government spending. There are also marked differences in Brazil, where the evolution of general government spending and central government spending has been different over the whole period. Comparing spending at different levels thus poses problems for both cross-country and within-country comparisons.
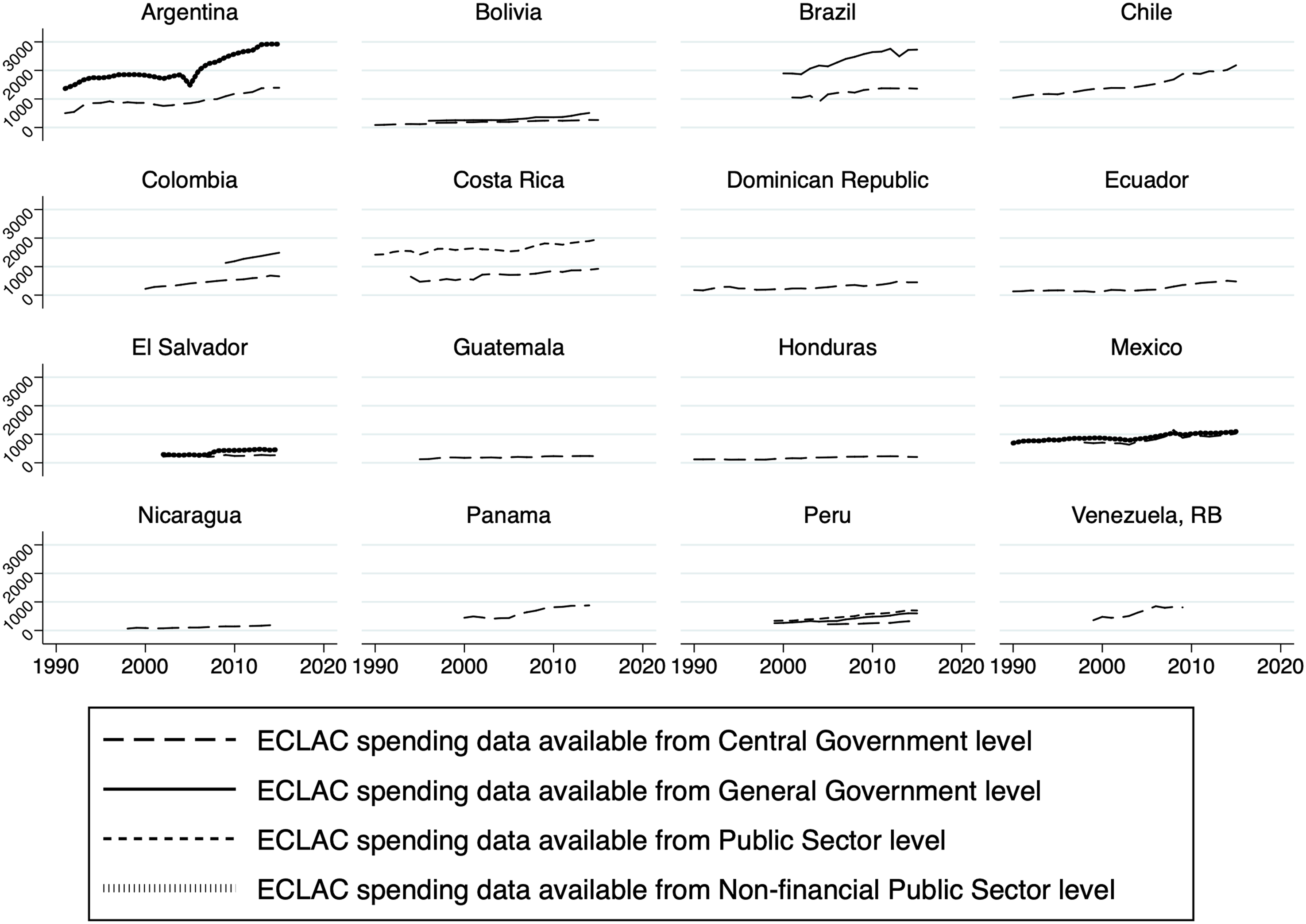
Figure 1. ECLAC public social spending data 1990–2015, by different levels of government spending.
With the Latin American Welfare Dataset, Huber and Stephens make an outstanding effort to construct the most comprehensive database possible. To do so, they combine four different ECLAC sources with IMF data. Unfortunately, by merging data from these different series, their information may end up combining central government spending at the beginning of the period with more encompassing levels later on.
How should researchers deal with this problem? If at all possible, we should only use data that fully reflects a country’s effort in social policy. This would require considering information for all levels of government in federal and highly decentralized countries. We should also change sources depending on the circumstances: for example, for a country like Bolivia, it may be fine to use central government data prior to the late 1980s but better to use data on the whole public sector for more recent periods.
More broadly, this problem calls for a new approach to the construction of databases by international institutions. At the moment, there is a lot of information available for some indicators—for example, each international institution seems to have its own information on pensions—and not enough on others. Ideally, the World Bank, ECLAC, and other institutions should collaborate to produce the most comprehensive historical data possible on various social spending indicators (see Martínez Franzoni and Sánchez-Ancochea Reference Martínez Franzoni and Sánchez-Ancochea2018 for a similar plea in the case of indicators on universalism). Working together and pooling resources, a better database could be easily built, improving quantitative (and qualitative) research significantly.
Data sources and measurement: Explanatory variables
Some of the key explanatory variables are also measured in different ways and come from a variety of sources. Consider, for example, the case of trade. Niedzwiecki (Reference Niedzwiecki2015) and some others use trade openness data from the World Bank’s World Development Indicators. In contrast, Avelino, Brown, and Hunter (Reference Avelino, Brown and Hunter2005) argue that measures of trade based on real exchange rates underestimate the size of some economies; they propose a trade measure based on purchasing power parity instead.
Democracy has also been measured in two ways: as a yearly binary dummy variable and as the number of years of democracy that a country has accumulated over time. Avelino, Brown, and Hunter (Reference Avelino, Brown and Hunter2005) rely on a binary distinction between democratic and authoritarian regimes, checking for robustness with continuous Polity data. They motivate their choice by explaining that they understand democracy as “fundamentally distinguishable” from other regimes, apparently implying that this distinction can be properly expressed by a yearly dummy. Huber, Mustillo, and Stephens (Reference Huber, Mustillo and Stephens2008), in contrast, rely on cumulative years of democracy from 1945 onward and argue that this measure is able to express the “strength of the democratic record,” likely implying that democracies become stronger, more stable, or more impactful over time. Kaufman and Segura-Ubiergo (Reference Kaufman and Segura-Ubiergo2001), Martín-Mayoral and Fernández Sastre (Reference Martín-Mayoral and Fernández Sastre2017), and Zarate Tenorio (Reference Tenorio2014) use binary measures as yearly dummies, while Niedzwiecki (Reference Niedzwiecki2015) uses cumulative years of democracy based on a binary measure; these four articles only state their choice without motivating it theoretically.
The use of these distinct measures of democracy imply different theoretical understandings of the role of democracy for public social spending. Even though it is not always clearly expressed, it seems that authors who use an annual score of democracy assume that the political system has an immediate impact on policy variables, while those that use accumulated years of democracy have a more complex understanding of how institutions work. Note also that besides the two common measures used by our authors, there exist other, less minimalist measures of democracy, which may convey different information still.Footnote 7
While we do not wish to identify any one measure as superior, we emphasize that any measure chosen should be a good fit for the theoretical mechanism under study. The use of different measures of democracy, as well as the use of more complex measures, clearly comes at a cost—the lack of direct comparability. Yet it may nevertheless enhance our understanding of the role played by democratic institutions for public social spending as long as authors motivate their choices clearly and carefully discuss implications of their results with a view to the measure used.
Periods of analysis
Another difference that should be mentioned refers to periods of analysis (see table 1). Decisions on the period of analysis are likely to exert a major influence on the results but receive insufficient attention. Martín-Mayoral and Fernández Sastre (Reference Martín-Mayoral and Fernández Sastre2017) conduct separate regressions for two different periods and obtain different results. This is not surprising: variables like commodity exports likely had a different impact on public social spending during the commodity boom of the 2000s than at other times. In fact, studies in other areas have shown that the impact of political processes varies significantly depending on the period under study. For example, Schmitt (Reference Schmitt2016) shows how periodization changes empirical results about partisan effects on policy.
Theoretically, it is clear that studies conducted on a specific time series should not be used to extrapolate beyond the period of the sample, but this is sometimes forgotten. Moreover, it would be useful to motivate the choice of time periods not only on the basis of data availability but on theoretical grounds. Here, econometric theory does not come with a user’s guide: the choice of periods under study needs to be based on case knowledge. Otherwise, the analysis could produce results that only hold for a subperiod, mask results from one period that do not hold for others, and so forth.Footnote 8
Decisions on econometric techniques
All studies we discuss in this article use time-series cross-section (TSCS) data. TSCS data come along with a number of features that contradict basic assumptions from the canonical ordinary least squares (OLS) model. This gives rise to a set of problems, among them unobserved heterogeneity; nonstationarity; endogeneity; and serial correlation, heteroscedasticity, and contemporaneous correlation of the error terms. The articles we review follow two general strategies to resolve some of these issues (see table 3): one group uses OLS panel estimators with country FE, and a second group uses error-correction models (ECM).
Table 3. Overview of estimation techniques used in six different studies.
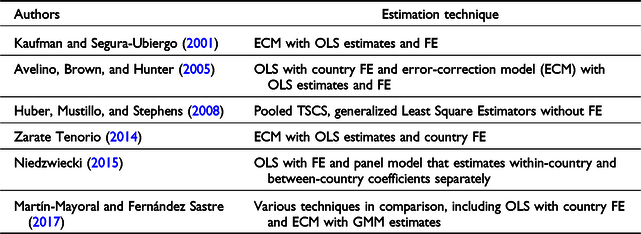
Unobserved heterogeneity
Unobserved heterogeneity is probably the primary reason to introduce country FE. Unobserved heterogeneity occurs when countries’ levels of public social spending differ in ways that are not explained by the independent variables included in the estimation. For example, it could be that a specific country has a political tradition of high public social spending levels. If this tradition is not accounted for, higher levels of social spending would be (falsely) attributed to other included factors. Unobserved heterogeneity thus results in biased estimators (Rabe-Hesketh and Skrondal Reference Rabe-Hesketh and Skrondal2008; Raudenbush and Bryk Reference Raudenbush and Bryk2002).
The use of country FE offers no one-size-fits-all solution and has the problems previously discussed. Huber, Mustillo, and Stephens (Reference Huber, Mustillo and Stephens2008) prefer to use pooled data precisely to explore country differences. Unfortunately, their solution does not properly deal with unobserved heterogeneity and may result in biased estimators. These problems are illustrated by Martín-Mayoral and Fernández Sastre (Reference Martín-Mayoral and Fernández Sastre2017), who provide estimates from a variety of techniques, among them pooled and FE OLS. Their results show marked differences between estimated coefficients and corresponding standard errors.
Nonstationarity
Many of the macro variables used in the studies we review likely exhibit nonstationarity (see, e.g., Phillips and Moon Reference Phillips2000), and some variables may be cointegrated. When time series exhibit nonstationarity in levels—and this is clearly the case with public social spending in Latin America or GDP per capita—spurious regressions can be the result (Granger and Newbold Reference Granger and Newbold1974; Entorf Reference Entorf1997). In the presence of nonstationarity and/or cointegration, OLS estimators are not appropriate, and alternatives such as fully modified estimators or dynamic OLS estimators should be considered (e.g., Kao and Chiang Reference Kao and Chiang2000; Baltagi Reference Baltagi2008). Where cointegration is present, approaches in the spirit of Engle and Granger (Reference Engle and Granger1987) may provide a viable solution.
In our sample of studies, only three articles address issues of nonstationarity and cointegration, at least partly or indirectly. Kaufman and Segura-Ubiergo (Reference Kaufman and Segura-Ubiergo2001) and Zarate Tenorio (Reference Tenorio2014) use error-correction models even though this choice is not motivated through nonstationarity concerns. Martín-Mayoral and Fernández Sastre (Reference Martín-Mayoral and Fernández Sastre2017) are the only ones to actually test for the presence of structural long-run relationships in their time series, employing unit root and then cointegration tests. In the two other cases, it is not made clear whether the choice of an error-correction model was appropriate, given the time series characteristics of the data. Overall, econometric requirements of the specific data employed are hardly ever discussed in the articles we review.
Serial correlation, heteroscedasticity, and contemporaneous correlation of the error terms
TSCS data typically exhibit a number of cross-sectional and temporal dependencies. Serial correlation of the error terms occurs when temporal dependencies exist in observations over time. In the case of heteroscedasticity, error terms have a constant variance within, but not across countries. Furthermore, there may be contemporaneous correlation of errors across countries, for example, through a common shock. Different types of dependencies may easily occur together but do not necessarily do so. Ignoring these dependencies can lead to biased inferences (e.g., Wooldridge Reference Wooldridge2010).
Diverse methods have been proposed to adjust standard errors. It is important to note that different solutions are appropriate depending on the case of dependencies. For example, heteroscedasticity-robust standard errors can be used in the presence of heteroscedastic residuals. Clustered standard errors adjust for cross-sectional dependence of observations within clusters such as countries. The latter also accounts for temporal dependency, while the first does not (Hoechle Reference Hoechle2007). Moreover, different robust estimators have different requirements in terms of panel size or structures.
Beck and Katz (Reference Beck and Katz1995) proposed panel-corrected standard errors (PCSE) that address heteroscedasticity, contemporaneous correlation, and serial correlation of order 1. While many authors seem to have taken this procedure as a universal remedy for all sorts of situations, PCSE have their own problems. For example, they may be problematic when the panel data set consists of a rather small number of years, or in the presence of serial correlation (Reed and Webb Reference Robert and Webb2010).
Our point is that the choice of appropriate standard errors is complicated, and there is hardly a one-size-fits-all solution. In practice, however, most articles in our sample employed PCSE without discussing whether PCSE was appropriate. They did not provide information on whether they tested for serial correlation, heteroscedasticity, and contemporaneous correlation in their data to choose appropriate standard errors. This is not a problem of the studies on social policy alone. Wilson and Butler (Reference Wilson and Butler2007, 102) analyze the intellectual aftermath of Beck and Katz (Reference Beck and Katz1995) in the political science literature and conclude that “a nontrivial number of studies appear to be nothing more than a blind application of the method”; others speak of a “de facto Beck-Katz standard” (Plümper, Troeger, and Manow Reference Plümper, Troeger and Manow2005, 327).
When reading the various articles, it is hard to know how exactly the problems were resolved, and the implication that this may have for the estimated coefficients and standard errors. The same technical solution may not always be the best for different studies, which means that the results are not necessarily comparable just because the same standard errors have been used.
Endogeneity
Endogeneity refers to situations in which the dependent variable is correlated with the error term. Endogeneity can be attributed to three types of causes: omitted variables, measurement error, and simultaneity (Wooldridge Reference Wooldridge2010). These constitute violations to the fundamental assumptions made in OLS estimations.
While many authors discuss omitted variable bias and measurement error in some form (recall the discussion about how to measure trade openness), simultaneity is less often addressed. For example, most studies in our sample measure the dependent variable as percentage of GDP, while also incorporating GDP as an independent variable on the right-hand side. As a consequence, the estimated coefficient for the effect of GDP is likely to be biased and may even change signs. Such an effect is possibly at work in Kaufman and Segura-Ubiergo’s (Reference Kaufman and Segura-Ubiergo2001) comparison of the results obtained using social spending as percentage of GDP and in per capita figures. When using the latter, they find a positive and statistically significant relationship between GDP per capita and public social spending per capita, in accordance to their theoretical expectations. Yet when using social spending as a percentage of GDP, most results are not statistically significant. If researchers want to incorporate GDP per capita on the right-hand side to test the modernization hypothesis, they should either use social spending figures per capita to measure the dependent variable or implement an estimation technique that takes care of this kind of endogeneity.
The assumption made about the absence of reverse or simultaneous causality in most studies is also problematic. For example, including the share of old-age population on the right-hand side of the equation can lead to biased estimators: a larger share of elderly people increases public social spending, but, at the same time, increased social spending could also increase life expectancy and thus the share of elderly people in the population. Martín-Mayoral and Fernández Sastre (Reference Martín-Mayoral and Fernández Sastre2017) raise a potential simultaneity relation between social spending and economic growth: while a favorable economic situation could increase public budgets, social spending could also lead to higher growth, for instance because public spending in education and health increases human capital.
In our sample, only Martín-Mayoral and Fernández Sastre (Reference Martín-Mayoral and Fernández Sastre2017) deal explicitly with endogeneity. They use system and differenced generalized methods-of-moments (GMM) estimators to control for potential endogeneity.Footnote 9 Yet even if the other articles considered endogeneity issues more explicitly, they could still resolve them in different ways. Furthermore, some simultaneous relationships are not immediately clear but are discovered in new research. Different considerations and expectations about potential simultaneity relationships can thus change results and render them incomparable.
In summary
The articles in our sample address technical issues in different ways, which has consequences for their results. Not all choices are equally satisfactory from a technical point of view. In some instances, authors may have introduced biases in their estimators in their attempt to resolve some other problems. In other cases, the choice of econometric tools is inconsistent with the research question to be addressed. In these cases, it is the use of the tool rather than the tool itself that causes problems.
This is our central concern: even if all choices researchers make were technically and theoretically satisfactory, there is still some scope for incommensurability. We think that an important first step to deal with this incommensurability is that authors provide more transparent reflections of choices made. Such discussions could not only help readers to understand the extent to which results are comparable but also ensure that choices made correspond to the authors’ specific analytical goals. Further, results and findings should be presented more clearly: for example, rather than stating that trade openness benefits public social spending, authors should explain that public social spending increases in countries as these countries open up for trade, or that in cross-country comparison, countries with higher trade openness have higher spending levels.
Mixed methods and conversations across methodologies as a useful response
Some of the problems we have identified in previous sections can be tackled by improving the methods (e.g., dealing with the standard errors appropriately) and the data researchers use. Yet, as this article shows at several points, many econometric challenges do not have an easy cure and contribute to the diversity of results. How can we deal with this situation? How can we advance more quickly in our understanding of the determinants of social policy? Although there is no single answer to these questions, we believe that more active conversations between qualitative and quantitative researchers would be particularly useful.
This is already done by researchers using mixed methods in a single research project. For example, in their recent review of mixed methods in the study of welfare regimes, Niedzwiecki and Nunnally (Reference Niedzwiecki and Nunnally2017, 1028) argue that “incorporating multiple methodologies in a single research design has the potential to significantly advance knowledge.” Let us illustrate how this interaction between methods has already taken place with a few examples that use econometrics to identify correlations and case studies to determine how the causal chain unfolds within and between countries. For example, Huber and Stephens’s (Reference Huber and Stephens2012) econometric analysis focuses on the correlation between political regimes and left-wing parties in governments and social spending in various areas. They then select five cases within the region to evaluate how democracy and the left operate in practice, and to identify omitted variables. Segura-Ubiergo (Reference Segura-Ubiergo2007) follows a similar methodology, although he focuses on three countries with similar levels of development but different degrees of openness and political institutions.
Case studies may also precede and inform subsequent econometric research. Niedzwiecki’s Uneven Social Policies (Reference Niedzwiecki2018) helps us explain why the relationship between politics and social policy at the national level can vary depending on the type of policy and a country’s level of decentralization. Focusing on the behavior of subnational governments in Argentina and Brazil, she shows that subnational politicians will act differently depending on the characteristics of the policies. When the attribution of responsibility is clear—that is, when voters have no doubt that the central government is responsible for the new program—subnational governments ruled by the opposition will be reluctant to implement it. This is exactly what happened with Asignación Familiar por Hijo and Bolsa Família, Argentina’s and Brazil’s conditional cash transfers, in the first decade of the 2000s. Niedzwiecki’s insights could inform future econometric research in at least two ways. First, they highlight the need to consider the relationship between level of decentralization and subnational government ideology through interaction effects. Second, researchers can use her work to determine when using central government statistics is appropriate and when it is not.
Garay’s Social Policy Expansion in Latin America (Reference Garay2016) is another recent book that could inform quantitative research and explain some of the confusing results we observe.Footnote 10 She uses the experience of Argentina, Brazil, Chile, and Mexico to discuss the reasons behind different levels of expansion in pensions, income support, and health care programs. Her work emphasizes the central role of outsiders (i.e., workers in informal jobs without social security benefits) in contemporary Latin America. In her view, it is not democracy per se but the level of electoral competition and the presence of social mobilization that determine social policy expansion. Her work invites quantitative researchers to consider electoral results and the relative share of insiders and outsiders when considering the growth of social spending. Her research also highlights the importance of coverage as an alternative measure of social policy expansion.
Yet we are not just calling for more mixed-methods research but for more and better interactions between quantitative and qualitative researchers as well. In particular, even when not conducting a mixed-methods study themselves, quantitative researchers may benefit from actively drawing on qualitative studies. In Multi-method Social Science, Seawright (Reference Seawright2016, 10) argues that “integrating designs are a wonderful tool for evaluation and critiquing others’ research, as well as for strengthening one’s own causal inferences.” In his view, qualitative research can support regression analysis in at least three ways. First, it can contribute to better measurement (and theoretical understanding) of the dependent and independent variables. The above-mentioned study by Niedzwiecki (Reference Niedzwiecki2018) is a straightforward example of a study that may help reconcile contradictory results from studies that use different government levels of spending. Referring to the data and measurement problems discussed in this article, qualitative knowledge could illuminate the extent data from different government levels are comparable in different countries, or which political processes are at play in specific countries, indicating whether a level or change specification is more appropriate. Likewise, case knowledge may help to identify breaks in political processes over time, which could help to compare results obtained from different study periods.
Second, qualitative studies can test causal paths, thus illuminating the processes behind certain statistical correlations. This strength has been highlighted by authors like Lieberman (Reference Lieberman2005), who propose a “nested” approach where case studies illuminate some of the findings and assumptions of the econometric exercise. Given the high number of contradictory findings in the literature we review, insights from country case studies may be used to discriminate between different findings and suggest avenues for future model specifications.
Third, qualitative studies can also illuminate the role of certain omitted variables (Seawright Reference Seawright2016, chapter 3). The appropriate specification of econometric models, in particular when it comes to control variables that should be included or not, requires theoretical and case knowledge. Mistaken ideas about variables to be left out or included have critical impacts on estimation results. Qualitative knowledge is crucial when setting up a specification and theorizing about relationships between relevant variables.
If the accumulation of knowledge is going to increase in this field (as in other fields of political economy), both qualitative and quantitative researchers should make an effort to design their work in a way that enhances communication. For quantitative researchers, this would require stating analytical goals and key decisions more clearly. Besides making their work more accessible for qualitative researchers, explicitly spelling out analytical goals could also improve the alignment of goals and technical decisions. Qualitative researchers could try to present their work (including its key dependent and independent variables) in such a way as to allow their findings to be assessed and expanded by quantitative researchers. Advancing in this direction may be easier for researchers of Latin America’s social policy than in other fields because they are part of a small but vibrant community where quantitative, qualitative, and mixed-method research is growing rapidly.
Conclusion
This article has studied the literature on the determinants of public social policy in Latin America to illustrate the difficulties that applied econometric research has in accumulating knowledge. We showed that the key studies do not offer any clear conclusion regarding the role of modernization, globalization, and politics. Why is this the case?
One potential answer is that the underlying social reality is contradictory: for example, some variables may affect the level of social spending in some periods and not others, depending on how they interact with other processes. This is implicitly Doyle’s (Reference Doyle2018) view in a recent review of the literature on social spending and taxation in the region. From this perspective the goal of future research should be to elaborate new theories to account for differences in results and to add new studies.
Instead, we have argued that applied econometric research of this kind may have some inherent problems. We have focused on three factors to explain the diversity of results: different technical decisions that lead to differences in the analytical questions asked; differences in the data sources; and diverse estimation problems that can affect results. All these problems demonstrate that the craft of applied econometric work is messier and more ambiguous than often expected.
To be clear, this should not lead us to conclude that econometric research is unhelpful, but that it both can be done better and should not occupy a monopolistic position in political economy research. We need to build more effective communication between qualitative and quantitative research, exploring with more detail how one can inform the other. We should also acknowledge the problems of quantitative research (as we more often do with qualitative studies) and spend more time building better databases, comparing econometric choices, and explaining the implications of our research. These are all challenging tasks, but they are particularly important when dealing with a topic of such intellectual but also political relevance as the expansion of social policy in the most unequal region of the world.