The Centers for Disease Control and Prevention (CDC) has published national- and state-level comparisons of prepandemic and pandemic-era standardized infection ratios (SIRs) and incidence rates. Reference Weiner-Lastinger, Pattabiraman and Konnor1 A limitation of this analysis is that it does not account for long-term trends in incidence. This is a serious limitation because the incidence of these HAIs had been falling for several years prior to the pandemic and likely would have continued to fall absent major disruption. 2 Thus, to measure the true effect of the pandemic on HAI, we should compare pandemic era incidence to the incidence we would have expected if long-term trends continued, rather than the incidence at an earlier date. Accordingly, we assessed the impact of the coronavirus disease 2019 (COVID-19) pandemic on Clostridioides difficile infection (CDI), methicillin-resistant Staphyolococcus aureus bloodstream infection (MRSA BSI), and central-line–associated bloodstream infection (CLABSI) incidence in California acute-care hospitals (ACHs) using multiple analytic approaches, including interrupted time series (ITS) regression. which are regression models with special variables related to time trends before and after the pandemic that enable evaluation of differences in the presence of long-term trends. We also conducted an exploratory analysis of the association between individual hospital COVID-19 burden and HAI incidence and an examination of prepandemic and pandemic-era measures of antibacterial drug utilization.
Methods
Under normal circumstances hospitals are required to report data on CLABSI, MRSA BSI, and CDI to the California Department of Public Health (CDPH) via the NHSN. 3 We performed all analyses for this report with NHSN data sets generated for CDPH annual HAI reports. Data-set generation dates were February 27, 2019, for 2018 data, February 24, 2020, for 2019 data, and April 1, 2021, for 2020 data. All analyses were performed using Statistical Analysis Software (SAS) version 9.4 software (SAS Institute, Cary, NC).
We performed 3 analyses to examine the impact of the pandemic on HAI incidence in ACHs. ACHs comprise a subset of hospitals that excludes critical-access hospitals, rehabilitation hospitals, and long-term acute-care hospitals. For the first analysis, we compared statewide incidence in the second half of 2020 (2020-H2, pandemic era) to the second half of 2019 (2019-H2, before the pandemic). We compared adjusted incidence using standardized infection ratios (SIRs) with a risk adjustment model based on the 2015 national baseline. SIRs are ratios of the observed number of infections in each facility or set of facilities and the number of infections that are predicted using measures of person time (patient days or central-line days) and a number of risk adjustment variables. Risk adjustment variables are facility characteristics and may be collected monthly, quarterly, or annually. Some risk adjustment variables are collected in the annual hospital survey, which is completed early in the following year. Thus, data for 2020, 2019, and 2018 are entered in 2021, 2020, and 2019, respectively. For facilities where the Annual Hospital Survey has not been completed by the data-set generation date, data from the previous annual survey are used. Complete risk adjustment models and the source for each variable used in these models have been published by the NHSN. 4 Statewide values are created by aggregating observed and expected values across facilities. Significance tests for difference were performed using the exact binomial test using scripts developed by the CDC for use on NHSN data. 5 We used only the second half of the year because reporting requirements were suspended for the first half of 2020 and were reinstated in July 2020. 6
To account for long-term trends in HAI incidence, we used ITS to evaluate differences in outcomes following pandemic onset. ITS regression models use a “pre-trend” (time) covariate to model the preinterruption trend; a “level change” covariate that is equal to zero in the preinterruption period and one in the postinterruption period and a “slope change” covariate that measures time since the interruption. Reference Kontopantelis, Doran, Springate, Buchan and Reeves7 Parameters for the slope-change and level-change variables give the immediate and long-term changes in HAI incidence, respectively.
We examined adjusted incidence during March–December 2020 compared with January 2018 through February 2020 using negative binomial ITS models with repeated measures at the facility level and first-order autoregression. Time was measured in 1-month periods, with the interruption beginning in March of 2020, which we considered the onset of the COVID-19 pandemic in California. Because predicted CLABSI infections for the SIR models are calculated for each month, we used the natural log of the number of predicted cases as an offset, adjusting for both the number of central-line days and facility-level factors contributing to patient risk of infection. The risk adjustment model used to calculate the predicted cases can be found in the NHSN “Guide to the SIR.” 4 Because MRSA BSI and CDI predicted cases are not estimated at the monthly level, we used the natural log of the number of inpatient days as the offset and included the variables used in the NHSN risk adjustment model as covariates. Although the NHSN SIR calculation uses annual measures for length of stay and quarterly measures of community onset prevalence, we calculated these variables at the monthly level for inclusion in regression models for MRSA BSI and CDI. Because some HAI outcomes are correlated with seasons or weather, Reference Richet8,Reference Leekha, Diekema and Perencevich9 we used data from the National Oceanic and Atmospheric Administration to add the mean monthly temperature, measured at the closest weather observation station, as a covariate in all models. 10
We sought to determine whether the ratio of the mean daily census of COVID-19 patients to licensed facility beds in May and June of 2020 was associated with HAI incidence in individual hospitals, using log-link models. Facilities enrolled in the NHSN COVID-19 tracking modules at different times, but almost all were enrolled by May 1, 2020. Hospital reporting to the NHSN COVID-19 module ended in mid-July. Therefore, May and June have nearly complete COVID-19 reporting to the NHSN. As with the ITS analysis, the model included offsets for the number of predicted cases for CLABSI and for covariates used in the NHSN risk adjustment model as well as an offset for patient days for CDI and MRSA BSI. Because the model for MRSA BSI incidence would not converge using the full set of risk-adjustment covariates used in the ITS analysis, we used a forward addition stepwise procedure to select these models. Risk adjustment covariates were added to the model based on the largest change in the value of the COVID-19 occupancy parameter until the model would not converge.
For the ITS and COVID-19 occupancy analyses, we created nonresponse weights to correct for the impact of missing data during the pandemic (see the Appendix online).
Because antibiotic use is a risk factor for MRSA BSI and CDI, we also compared standardized antimicrobial administration ratios (SAARs) for the second halves of 2019 and 2020 for all adult antimicrobial agents and a subset of drugs that pose a high risk for CDI. These data are only available for a subset of facilities that voluntarily report data to the NHSN Antimicrobial Use and Resistance (AUR) module and elect to share these data with the CDPH. The SAAR is a risk-adjusted measure of antimicrobial use days per inpatient day. 11 Mid P values were calculated using exact binomial tests.
Results
Figure 1 shows the number of total inpatient days and the number of COVID-19 inpatient days per month from all California hospitals, along with incidence rates of CLABSI, MRSA BSI, and CDI in ACHs, for April through December 2020 using publicly available data on COVID-19 patient burden. 12 The number of COVID-19 cases in ACHs is not available for this entire period, but ACHs account for most facilities and patient days in California. The statewide number of inpatient days in ACHs decreased from 7,469,067 in the second half of 2019 to 7,225,102 in the second half of 2020.

Figure 1. Monthly statewide inpatient confirmed COVID-19 and total patient-days (bars) and crude incidence rates per 10,000 central line-days (CLABSI), 10,000 patient-days (CDI), and 100,000 patient-days (MRSA BSI), April to December 2020.
The results of the comparison of statewide SIRs are shown in Table 1. We detected substantial and significant increases in the SIRs for CLABSI and MRSA BSI. Changes in the SIR for CDI were insignificant and unsubstantial.
Table 1. Comparison of Incidence of Healthcare-Associates Infection (HAI) Incidence in California Acute-Care Hospitals in the Second Half of 2019 Versus the Second Half of 2020

Note. CLABSI, central-line–associated bloodstream infection CDI, Clostridioides difficile; MRSA BSI, methicillin-resistant Staphylococcus aureus bloodstream infection; SIR, standardized infection ratio.
a Exact binomial test mid P value.
The number of facilities and facility months included in the regression analyses is given in Table 2. Incidence rate ratio (IRR) estimates and confidence intervals (CIs) from the ITS analysis are shown in Table 3. We present unweighted estimates because weighting had minimal effect on the value and variance of the parameters of interest. For all infection types the “pre-trend” IRR was significantly < 1 at α = 0.05, indicative of decreasing incidence in the prepandemic period. For CLABSI, both the level-change IRR (1.27; 95% CI, 1.11–1.46) and the slope change IRR (1.04; 95% CI, 1.02–1.07) were significantly > 1. This corresponds an immediate increase of 27% in incidence of CLABSI at the start of the pandemic followed by a change in the magnitude of the time trend by 4% relative to what would have been expected if long-term trends in incidence continued. For MRSA BSI, the level-change IRR was positive but not significantly > 1 (1.06; 95% CI, 0.84–1.34), indicating uncertainty about immediate effects on incidence. The slope-change IRR (1.04; 95% CI, 1.01–1.08) was significantly > 1. The sum of the pre-trend and slope-change parameters was > 0 for both MRSA BSI and CLABSI, suggesting a reversal of the previous long-term trend to a long-term increase in incidence over time. For CDI, the level-change IRR (1.04; 95% CI, 0.94–1.14) and the slope-change IRR (1.01; 95% CI, 1.00–1.03) were both >1 but were not significantly different from 1. Figure 2 shows the model-predicted values of SIR (CLABSI) and incidence rates (MRSA BSI and CDI).
Table 2. Facilities and Facility Months of Data for the Interrupted Time Series (ITS) and COVID-19 Occupancy Regression Analyses by Infection Type

Note. HAI, healthcare-associated infection; CLABSI, central-line–associated bloodstream infection; CDI, Clostridioides difficile; MRSA BSI, methicillin-resistant Staphylococcus aureus bloodstream infection.
Table 3. Results of Interrupted Time Series Analysis of the Impact of the COVID-19 Pandemic on Incidence of Healthcare-Associated Infections (HAIs)

Note. CI, confidence interval; IRR, incidence rate ratio; CLABSI, central-line–associated bloodstream infection; CDI, Clostridioides difficile infection; MRSA BSI, methicillin-resistant Staphylococcus aureus bloodstream infection.
a For definitions of risk adjustment variables, see the NHSN guide to the SIR at https://www.cdc.gov/nhsn/pdfs/ps-analysis-resources/nhsn-sir-guide.pdf.
b Prepandemic time covariate to model the preinterruption trend.
c Start of pandemic parameter which is equal to zero in the preinterruption period and one in the postinterruption period.
d Slope change, product of time and pandemic parameter to evaluate the change in temporal trends after interruption.
* Variables from the NHSN Annual Hospital Survey.

Figure 2. Interrupted time series model predicted statewide values of a) standardized infection ratios for central line-associated bloodstream infection and incidence rate per 10,000 person days for b) Clostridioides difficile infection, and c) methicillin-resistant Staphylococcus aureus bloodstream infection by month, 2018 to 2020.
Results of the analysis of COVID-19 occupancy on HAI incidence are shown in Table 4. Increased COVID-19 occupancy was positively and significantly associated with CLABSI and MRSA BSI incidence, with IRR estimates of 1.04 (95% CI, 1.02–1.06) and 1.05 (95% CI, 1.02–1.08), respectively. These parameter estimates indicate an estimated increase of 4% (95% CI, 2%–6%) in CLABSI incidence and a 5% (95% CI, 2%–8.0%) increase in MRSA BSI incidence for every 1% increase in the proportion of licensed beds occupied by COVID-19 patients. The IRR estimate for CDI was 0.99 (95% CI, 0.97–1.01) and was not significantly different from 1.
Table 4. Results of Multivariate Regression of COVID-19 Patient Occupancy on Incidence of Healthcare-Associated Infections (HAIs)
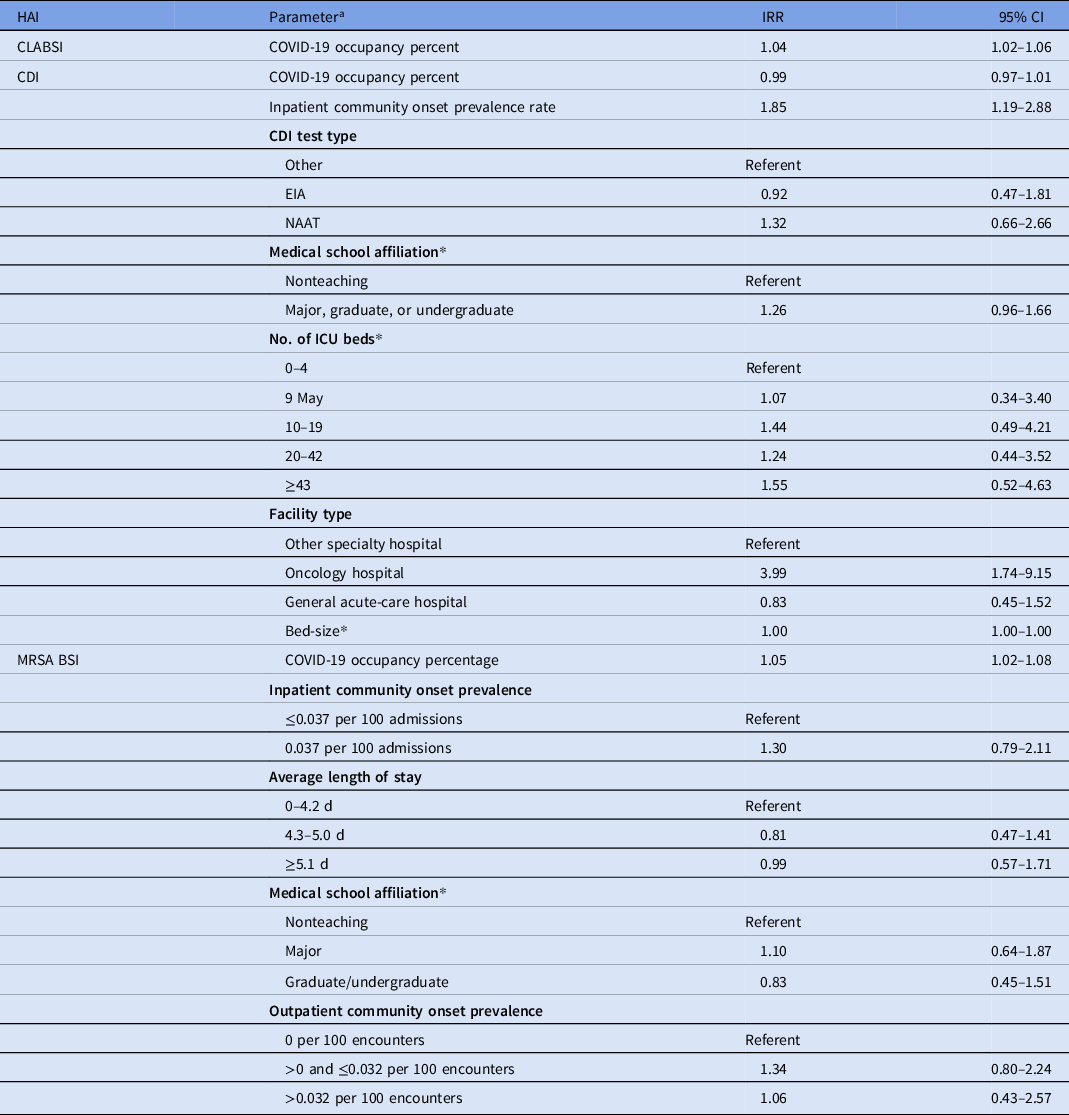
Note. CI, confidence interval; IRR, incidence rate ratio; CLABSI, central-line–associated bloodstream infection; CDI, Clostridioides difficile infection; EIA, enzyme-linked immunosorbent assay; NAAT, nucleic acid amplification test; MRSA BSI, methicillin-resistant Staphylococcus aureus bloodstream infection.
a For definitions of risk adjustment variables, see the NHSN guide to the SIR at https://www.cdc.gov/nhsn/pdfs/ps-analysis-resources/nhsn-sir-guide.pdf.
* Variables from the NHSN Annual Hospital Survey.
The number of facilities with available data in the AUR for the second half of 2019 and the second half of 2020 were 98 and 86, respectively. The SAAR for all adult antimicrobials decreased from 1.08 to 1.05 (P < .001), but the SAAR for antibacterial agents posing the highest risk for CDI increased from 1.05 to 1.13 (P < .001).
Discussion
Long-term decreases in CLABSI and MRSA BSI incidence were reversed in California hospitals during the COVID-19 pandemic. Furthermore, increased incidence of these infections in May and June of 2020 was directly correlated with the proportion of an individual hospital’s licensed beds occupied by COVID-19 patients. This finding supports the hypothesis that the increases in HAI incidence were due to COVID-19 case-burden–related challenges at the individual hospital level. Possible causes of these increases cited in the literature include resource diversion from infection prevention activities, personal protective equipment (PPE) shortages and misuse, and hospital overcrowding. Reference McMullen, Smith and Rebmann13–Reference Palmore and Henderson15 Also, the patient case mix during the pandemic, including persons admitted for non–COVID-19 reasons, was sicker overall and thus was possibly at higher risk of HAIs than before the pandemic. Reference Kazakova, Baggs and Parra16,Reference LeBrun, Konnaris and Ghahramani17 Therefore, some of the increases seen in CLABSI and MRSA BSI were likely due to shifts in the underlying patient population risk. Notably, CLABSI and MRSA BSI are not mutually exclusive diagnoses, 9% of CLABSI in the prepandemic era and 4% in the pandemic era were MRSA infections and would also count as MRSA BSI.
CDI was the only HAI for which the SIR decreased between the second half of 2019 and the second half of 2020, although the decrease was very small and not statistically significant. CDI is the most common HAI examined, so the power to detect substantial true changes should be large. Nonetheless, we did not find any significant association with pandemic onset or COVID-19 occupancy. Thus, CDI incidence may have been less affected by the pandemic than MRSA BSI and CLABSI incidence, although we cannot rule out small associations.
Our analysis of the SAAR showed that overall risk-adjusted antibacterial drug use in a subset of facilities declined slightly, but the use of drugs posing the highest risk for CDI increased by a very modest amount (8%). This finding, along with the lack of detected changes in CDI incidence trends, may be an indicator that antibiotic stewardship efforts were robust during the pandemic.
Our findings are consistent with multiple other studies that have described increases in CLABSI or methicillin-resistant Staphylococcus aureus (MRSA) bacteremia incidence in other places in the United States, using various data sources and statistical methods. Reference Weiner-Lastinger, Pattabiraman and Konnor1,Reference McMullen, Smith and Rebmann13,Reference Fakih, Bufalino and Sturm18,Reference Patel, Weiner-Lastinger and Dudeck19 They are also consistent with a study from a large hospital system that reported an association between the proportion of facility beds occupied by COVID-19 patients and CLABSI and MRSA bacteremia. Reference Baker, Sands and Huang20 At least 2 other study groups also found no associations with pandemic onset or facility COVID-19 burden and CDI. Reference Weiner-Lastinger, Pattabiraman and Konnor1,Reference Baker, Sands and Huang20 Our analysis adds to the literature on the effect of the COVID-19 pandemic by examining changes in incidence in the context of long-term trends and by directly examining the relationship between a facility’s COVID-19 patient burden and HAI incidence.
A strength of our analysis is our ability at the CDPH to access and include NHSN HAI data from all nonfederal California hospitals for the 3 HAIs examined for the entire period under study, with the exception of the first half of 2020. 6 Unlike some previous analyses, this analysis was not limited to a single healthcare system nor was it hampered by the limitations and possible biases that accompany voluntary reporting or data rights conferral. Another strength of this study was the inclusion of the ITS analysis, which allowed examination of changes in adjusted incidence within the context of long-term trends.
This study also had several limitations. The analysis lacked individual-level comorbidity and severity data provided by the NHSN. Risk adjustment for the infections examined here was based on hospital characteristics, some of which are summary measures reported only once yearly in an annual survey (conducted early in the following calendar year). Under normal circumstances, hospital characteristics collected annually may be a reasonable proxy for the underlying risk in the patient population, but they may not be sufficient to adjust for rapid changes in patient risk profile within the same institution, as occurred during the COVID-19 pandemic. The variables of most concern are length of stay (for MRSA BSI SIR and SAAR calculations), facility bed size (used in all SIRs, SAARs, and regression models), and ICU size (used in all risk adjustment models except for CLABSI). These variables may have changed in response to the pandemic but had the same values for the pandemic months and the prepandemic months of January and February 2020. Bed size and ICU size variables are of particular concern because the values collected are the maximum number of staffed beds. If facilities added beds in response to the pandemic, it is likely in some cases that the pandemic-era values were used for the prepandemic months of January and February 2020. This issue has affected not only our analysis but also other analyses using NHSN data and risk adjustment methodology during the COVID-19 pandemic. In addition, some facilities did not complete their annual survey by the generation date for the public report data files, in which case data from the previous year’s survey were substituted. The proportion of facilities that had not completed the annual survey was 4.3% for 2020 data, 19.2% for 2019 data, and 11.6% for 2018 data. Another major limitation is the possibility of incomplete reporting. We are most concerned with incomplete reporting during the first half of 2020, during the suspension of reporting requirements, but we also have concerns about data completeness and quality for the remainder of 2020. Nonresponse weights to designed to minimize possible biases had little effect on parameter estimates, but residual bias is possible if the nonresponse model was mis-specified. Also, the CDPH was unable to conduct any validation of NHSN patient-care location mapping during 2020, which might have affected risk adjustment models for CLABSI SIRs that include location type. Also, our analysis of antimicrobial use was limited by the facts that reporting of this data was voluntary and that data were obtained from a nonrandom subset of facilities.
Plans for future research include further investigating the relationship between HAI COVID-19 burden and HAI incidence during later phases of the pandemic, including surges in the winters of 2020–2021 and 2021–2022, and exploring the effect measure modification of pandemic related changes in incidence by hospital characteristics, such as ownership, teaching status, or state region. Another avenue for future research is the use of supplementary data sources, such as hospital discharge data, to add information on COVID-19–related occupancy, nurse-to-patient ratios, COVID-19 status of patients with HAIs, and changing characteristics of the patient population. Our findings underscore the urgent need for renewed focus on HAI reduction, particularly for CLABSI and MRSA BSI, and for the identification of ways to increase the resilience of hospital infection control programs to major disturbances in the healthcare system during the ongoing COVID-19 pandemic and in anticipation of future pandemics.
Supplementary material
To view supplementary material for this article, please visit https://doi.org/10.1017/ice.2022.269
Acknowledgments
We thank Monise Magro for her assistance in analyzing pathogens for CLABSI. The findings and conclusions in this article are those of the author(s) and do not necessarily represent the views or opinions of the California Department of Public Health or the California Health and Human Services Agency.
Financial support
No financial support was provided relevant to this article.
Conflicts of interest
All authors report no conflicts of interest relevant to this article.