Introduction
In December 2019, an acute respiratory disease caused by severe acute respiratory syndrome coronavirus SARS-CoV-2 [Reference Phelan, Katz and Gostin1], and officially named as Corona Virus Disease 2019 (COVID-19) occurred in Wuhan, which quickly caused a pandemic in the world [Reference Ahmad, Mueller and Tsamakis2]. By 23 April 2020, more than 2 million confirmed cases and 180 000 dead cases have been documented worldwide. Unfortunately, a simplified prognostic risk score, which could be easily used in primary care or general practice settings, has not been developed for this unprecedented disease [Reference Pan3].
In the assessment and management of pneumonia, disease severity assessment is crucial in guiding therapeutic options such as the need for hospital admission or intensive care (ICU) stay. Due to the high burden of COVID-19, we need a simple one to quickly triage severe patients. The pneumonia severity index (PSI) provides a means for stratifying groups of patients according to their risk of mortality and features [Reference Fine4]. Although it is complicated to use, requiring the computation of a score based on 20 variables, and hence may not be practical for routine application in busy hospital emergency departments or primary care settings. In addition, it is best validated for assessing patients with a low mortality risk who may be suitable for home management rather than those with severe pneumonia at the time of hospital admission [Reference Fine4].
It has been reported that a higher sequential organ failure assessment (SOFA) score was associated with increased odds of death in hospitalised COVID-19 patients [Reference Zhou5]. First developed in 1994, the SOFA score has been proved useful in predicting outcomes for patients in ICU. SOFA score measures organ dysfunction in six different systems (needs arterial blood gas result), using a 5-point scale, which also makes it difficult to use to triage patients.
It has recently been reported that older COVID-19 patients (>65 years) with comorbidities were at increased risk of death [Reference Yang6], which enlightens us to test the prognostic value of CURB-65 score in COVID-19 patients. CURB-65 score is a widely used score system to guide the treatment of community-acquired pneumonia (CAP), which consists of five easily acquired parameters (confusion, blood urea nitrogen (BUN), respiratory rate, blood pressure and age) [Reference Singanayagam and Chalmers7]. CURB-65 score has been verified to effectively predict clinical outcomes in CAP caused by viral infection [Reference Zhou8]. Our study aimed to analyse the prognostic value of CURB-65 at admission in patients with COVID-19 and to identify the CURB-65 cut-off value for in-hospital death.
Methods
Study design and participants
This is a retrospective, single-centre cohort study. All severe and critical adult patients with laboratory-confirmed COVID-19 in the West campus of Union Hospital, Tongji Medical College Huazhong University of Science and Technology (Wuhan, China), from 28 January 2020 to 29 February 2020, were included in this study. The confirmed diagnosis of COVID-19 was defined as a positive result by using real-time reverse-transcriptase polymerase chain reaction detection for routine nasal and pharyngeal swab specimens as described elsewhere [Reference Huang9]. Since this hospital was one of the major designated hospitals for the transfer of severe patients with COVID-19 in Wuhan, patients admitted were either diagnosed with severe or critical COVID-19. The diagnosis of severe or critical cases was in accordance with the 6th edition guideline issued by China's National Health Commission as previously reported [Reference Pan3]. Adult severe cases were diagnosed when any of the following criteria were met: (1) respiratory distress (⩾30 breaths/min (bpm)); (2) oxygen saturation ⩽93% at rest and (3) arterial partial pressure of oxygen (PaO2)/fraction of inspired oxygen (FiO2) ⩽300 mmHg (l mmHg = 0.133 kPa). Adult critical cases were diagnosed when any of the following criteria were met: (1) respiratory failure and requiring mechanical ventilation; (2) shock and (3) with other organ failures that require ICU care. Patients were treated in accordance with the 6th edition guideline issued by China's National Health Commission [Reference Pan3] with no bias. This study was conducted in accordance with the amended Declaration of Helsinki.
Data collection
The clinical characteristics, including symptoms, signs, laboratory results, treatments and outcomes, were obtained from the hospital's electronic medical records according to previously designed standardised data collection forms. Routine blood examinations were complete blood count, coagulation profile, serum biochemical tests (including renal and liver function, creatine kinase (CK), lactate dehydrogenase (LDH) and electrolytes), myocardial injury markers, C-reactive protein (CRP) and procalcitonin. The frequency of examinations was determined by the treating physician. The results at admission were analysed in our research. CURB-65 score was calculated in all patients as previously described [Reference Alyacoubi10]. Briefly, data for confusion, BUN, respiratory rate, systolic blood pressure (SBP) or diastolic blood pressure (DBP), age were collected and scored.
Two researchers independently reviewed the data collection forms, and a third researcher adjudicated any difference in interpretation between the two primary reviewers.
Statistical analysis
We first compared different characteristics between survivors and non-survivors. Continuous and categorical variables were reported as median (interquartile range (IQR)) and N (%). They were tested by Wilcoxon rank-sum test, χ2 test or Fisher's exact test where appropriate. Then univariate analysis for risk factors associated with death were conducted with logistic regressions and plotted by forest plots. We then applied the receiver operating characteristic (ROC) curve analysis to evaluate the performance of CURB-65 on death. An area under curve (AUC) over 0.8 was considered a good discrimination ability. To identify a better cut-off value for CURB-65 and death, diagnostic statistics (sensitivity, specificity and F1 score) were compared, and associated approaches for optimal threshold identification (Younden's index, minimum distance estimation and cross-over point for sensitivity-specificity curve) were applied. In addition, univariate Cox models for different CURB-65 cut-off values and death were conducted. The model with a smaller Akaike information criterion (AIC) was considered as a better model. A Kaplan–Meir curve was then plotted, with a log-rank test conducted for the comparison between the two groups divided by our identified cut-off value. Finally, a multi-variate Cox proportional-hazard model was used to evaluate the contribution of potential risk factors for death. To avoid overfitting in the model with a small sample size (number of deaths = 31), three variables were finally chosen for multivariate analysis based on the results of univariate analysis and clinical knowledge. In all Cox models, time was measured as the time since the admission day. A two-sided α of less than 0.05 was considered statistically significant. The statistical analyses were performed using SAS 9.4 (SAS Institute, Cary, NC, USA).
Results
From 28 January 2020 to 29 February 2020, 113 adult patients were hospitalised, after excluding 35 patients that were still hospitalised or not confirmed by COVID-19 RNA detection, and four patients without available key information in their medical records, a total of 74 patients were included in this study. Among all 74 patients, 31 patients died and 43 patients survived (Fig. 1).

Fig. 1. Patient flowchart.
Baseline characteristics between survivors and non-survivors
As shown in Table 1, the median age of all patients was 64 years (IQR 46–67), ranging from 21 to 91 years, among whom 58% were male. Of all patients, 89% lived in Wuhan and 98% showed bilateral patchy shadowing in chest computed tomography (CT). The median length of hospital stay was 15.5 days. Hypertension (31%) and diabetes (18%) were common comorbidities. The most common symptoms on admission were fever (77%) and cough (70%), followed by dyspnoea (54%) and fatigue (53%). On admission, fever was observed in only 28% of all patients, 24% had percutaneous oxygen saturation (SpO2) below 90%, 26% had respiratory rate higher than 24 bpm. Patients illustrated low blood lymphocyte count with a median of 0.89 (0.58, 1.37) × 109/l, low serum albumin with a median of 29.4 (25.4, 33.8) g/l, high neutrophil-to-lymphocyte ratio (NLR) with a median of 6.51 (3.43, 10.28), high CRP with a median of 40.95 (10.63, 92.29) mg/l and high D-dimer with a median of 0.86 (0.3, 6) μg/ml. Of all patients, 61% had a CURB-65 score 0 or 1, 28% had a CURB-65 score 2, 11% had a CURB-65 score 3 or above.
Table 1. Baseline characteristics of study population

Compared with survivors, non-survivors were older in age (68 vs. 60, P = 0.001) and presented more with dyspnoea (81% vs. 35%, P < 0.0001) and confusion (26% vs. 2%, P = 0.002). At admission, higher proportion of non-survivors suffered from fever (43% vs. 19%, P = 0.02), SpO2 < 90% (37% vs. 3%, P = 0.0004) and respiratory rate over 24 bpm (52% vs. 7%, P < 0.0001) (Table 1). Non-survivors exhibited different profiles of laboratory tests compared with survivors. In general, they had higher white blood cells (WBC) (8.2 vs. 6.3, P = 0.002), higher neutrophil (7.2 vs. 4.4, P < 0.0001), lower lymphocyte (0.7 vs. 1.1, P < 0.0001) and thus resulted in a much higher NLR (10.3 vs. 4.1, P < 0.0001). Higher levels of inflammatory or tissue injury markers were observed in non-survivors compared with survivors, including CRP (85 vs. 25, P < 0.0001), LDH (541 vs. 252, P < 0.0001) and α-hydroxybutyrate dehydrogenase (449 vs. 201, P < 0.0001). Markers for liver function, especially syntheses function, were lower in non-survivors than survivors: albumin (27 vs. 33, P < 0.0001), pre-albumin (171 vs. 86, P < 0.0001). Elevated BUN (8.9 vs. 5.4, P = 0.001) was observed in non-survivors than survivors, with no significant difference in estimated glomerular filtration rate (eGFR). The serum levels of CK (150 vs. 55, P = 0.001) and CK-MB (150 vs. 55, P = 0.001) were elevated in non-survivors compared with survivors, with no significantly difference in troponin T or NT-proBNP (data not shown). Remarkable elevated D-dimer (8 vs. 0.5, P = 0.0002) and prolonged prothrombin time (14.3 vs. 13.1, P = 0.002) were observed in non-survivors. As shown in Figure 2 and Table 1, non-survivors had higher proportion of patients with CURB-65 score 2 (42% vs. 19%), 3 or above (26% vs. 0%) (P < 0.0001).

Fig. 2. CURB-65 distribution for survivors and non-survivors. The distribution of patients with different CURB-65 score ranges was compared in survivors and non-survivors. In survivors, 81% had a CURB-65 score 0 or 1, 19% had a CURB-65 score 2. In non-survivors, 32% had CURB-65 score 0 or 1, 42% had CURB-65 score 2, while 26% had CURB-65 score 3.
Univariate analysis of risk factors for death
Significant variables in univariate analysis are plotted in Figure 3. Among all the factors associated with in-hospital death, CURB-65 (odds ratio (OR) 4.11, 95% confidence interval (CI) 2.10–8.02) had the largest effect size, followed by neutrophil (OR 1.73, 95% CI 1.28–2.34), NLR (OR 1.37, 95% CI 1.16–1.62), WBC (OR 1.45, 95% CI 1.14–1.84) and D-dimer (OR 1.54, 95% CI 1.24–1.91). For discrimination ability, LDH demonstrated the highest AUC of 0.93 and 1 unit increment of LDH increased the odds for death by 1% (OR 1.01, 95% CI 1.01–1.02). Among all risk factors, CURB-65 still showed good performance with an AUC of 0.81 (95% CI 0.71–0.91) (Supplementary Figure 2).
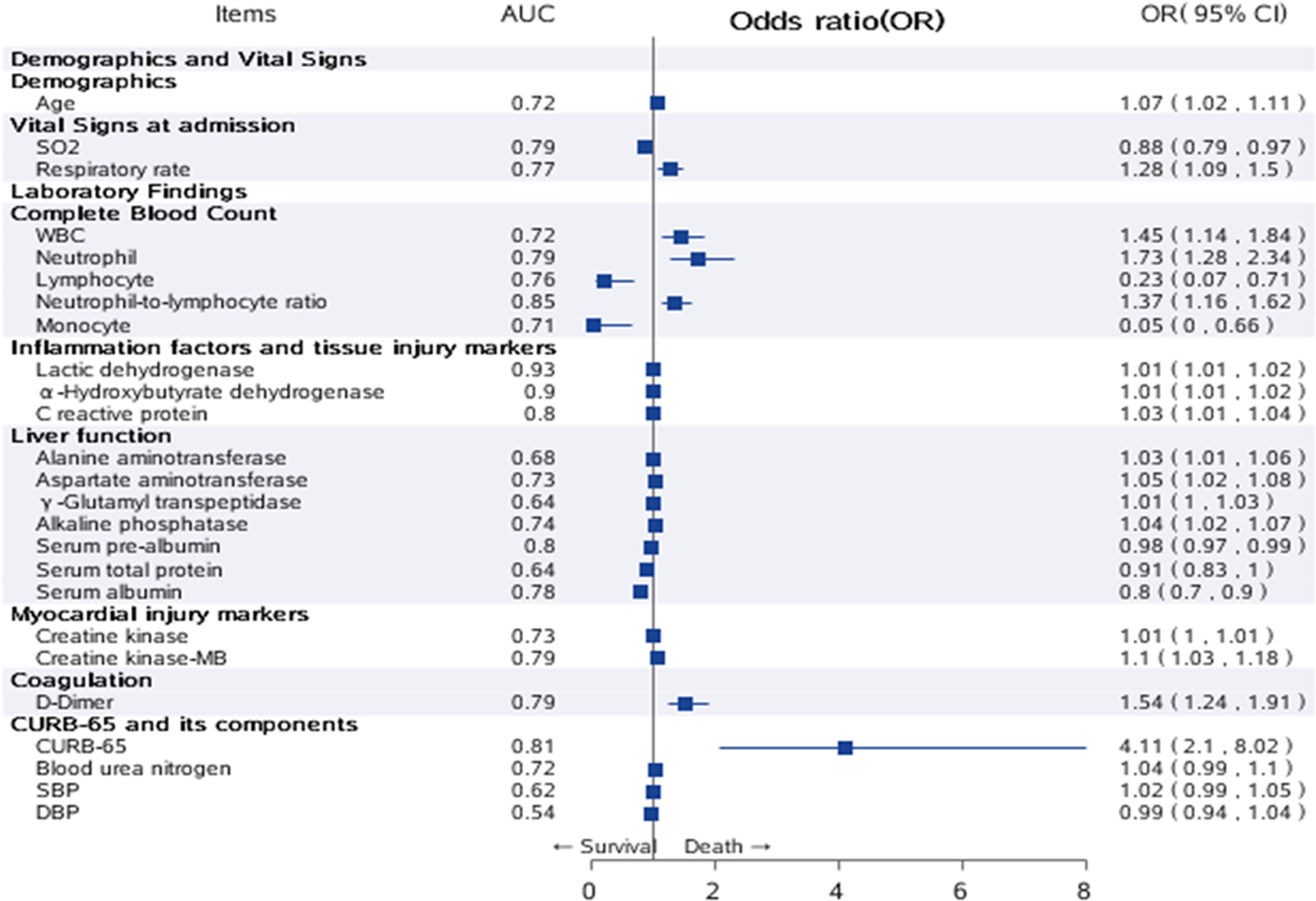
Fig. 3. Univariate analysis of risk factors for in-hospital death. Significant variables in univariate analysis were plotted as a forest plot. The second column listed the AUC of all risk factors, the third column listed OR and 95% CI for all risk factors. Note: Those factors with extremely wide CI were ignored in the plot.
Identification of cut-off value for CURB-65
We further explored the potential cut-off value for CURB-65 in predicting in-hospital death. As shown in Supplementary Figure 3, all survivors had CURB-65 <3, while only 26% of non-survivors had CURB-65 ⩾3, which indicates 3 may not be a proper cut-off in predicting death. As shown in Supplementary Figure 4, Youden's index for the ROC curve of CURB-65 was 2, with a sensitivity of 68% and specificity of 81%, suggesting 2 seems a better cut-off value. When the CURB-65 cut-off value was set to 2, the highest F1 score (0.70) and lowest AIC (222) for univariate Cox models were observed (Supplementary Table 1).
Survival analysis according to modified CURB-65 cut-off value
We compared the clinical characteristics of different CURB-65 score categories. As shown in Supplementary Table 2, patient with CURB-65 score 2 or above illustrated similar clinical characteristics to non-survivors, such older age (71 vs. 60, P < 0.0001), higher respiratory rate (24 vs. 20, P = 0.0006), lower SpO2 (92 vs. 97, P = 0.0003), higher NLR (10.8 vs. 4.4, P < 0.0001) and higher D-dimer (5.4 vs. 0.5, P = 0.004). Kaplan–Meier curve demonstrated significantly higher survival rates in patients with CURB-65 <2 than patients with CURB-65 ⩾2 (log-rank P-value <0.0001) (Fig. 4, Supplementary Table 3). We also performed univariate analysis to identify the risk factors for CURB-65 ⩾2 and found that apart from the composing factors of CURB-65 (age, BUN and respiratory rate), the risk factors for CURB-65 ⩾2 were high WBC, neutrophil, NLR, D-dimer and low lymphocyte (Supplementary Fig. 5), which were very similar to risk factors for death.

Fig. 4. Kaplan–Meier curve for death and CURB-65 categories. Survival analysis for patients categorised by the CURB-65 score. Patients with CURB-65 ⩾2 had significantly lower survival probability than those with CURB-65 <2 (P < 0.05).
Multivariate analysis
We found that CURB-65, LDH and serum albumin were associated with increased hazards of death in the multivariable Cox model (Table 2). We excluded variables from the univariable analysis if their between-group differences were not significant, if the number of events was too small to calculate hazard ratios (HRs), or if they had collinearity with CURB-65. One unit increase of CURB-65 increased the hazards of death by 61% (HR 1.61; 95% CI 1.05–2.46), while one unit decrease of serum albumin increased the hazards of death by 10% (HR 0.90; 95% CI 0.81–1.00).
Table 2. Multivariate model for death

Discussion
This retrospective cohort study identified several risk factors for in-hospital death in adult patients in Wuhan who were hospitalised for COVID-19. In particular, higher serum LDH, lower serum albumin and higher CURB-65 score on admission were associated with higher hazards of in-hospital death. Previous reports showed that older age, D-dimer levels >1 μg/l and higher SOFA score on admission were associated with higher odds of in-hospital death. Our findings share similarities with previous reports in that older age is included in the CURB-65 score. In addition, our findings make useful supplementation to current knowledge by shedding light on the prognostic value of serum LDH and albumin.
Previous publications showed that non-survivors of COVID-19 tend to be older in age [Reference Yang6], and have higher plasma concentrations of alanine aminotransferase, aspartate aminotransferase, creatinine, CK, LDH, cardiac troponin I, NT-proBNP and D-dimer [Reference Chen11]. In hospitalised COVID-19 patients, common symptoms included fever, fatigue and dry cough. Lymphopoenia occurred in 70.3% of patients, prolonged prothrombin time (PT) was noticed in 58% of patients, elevated serum LDH presented in 39.9% patients, while critical patients were more likely to have dyspnoea and anorexia [Reference Wang12]. Similarly, we found that non-survivors were older and presented more with dyspnoea, confusion, fever and hypoxia. Non-survivors exhibited higher laboratory parameters such as WBC, neutrophil, NLR, CRP, LDH, α-hydroxybutyrate dehydrogenase, BUN, CK, CK-MB, D-dimer and PT, but lower laboratory parameters such as lymphocyte, albumin and pre-albumin.
Numerous scoring systems have been used to assess the severity of illness in patients with CAP (e.g. PSI [Reference Fine4, Reference Fine13] and CURB-65 [Reference Lim14]) or in ICU (e.g. APACHE-II and SOFA [Reference Hillas15]). However, they either require complicated input variables (e.g. APACHE-II) or require repeated measures (e.g. SOFA). In contrast, CURB-65, consisting of only five easily obtained parameters (mainly derived from vital signs), has been validated in different populations [Reference Lim14, Reference Capelastegui16, Reference Aujesky17] and regarded as a strong predictor for mortality in CAP patients [Reference Zhou8]. Celal Satici et al. also found an excellent discrimination ability of CURB-65 for prediction of 30-day mortality in hospitalised patients, although it was outperformed by the more complex score system PSI [Reference Satici18]. In our study, CURB-65 demonstrated a good performance (AUC 0.81) and had a relatively large effect size (OR 4.11, 95% CI 2.10–8.02) for in-hospital death in univariate analysis. Previous CAP guideline suggested that patients with CURB-65 score 0 and 1 should be treated as outpatients, those with CURB-65 score 2 should be hospitalised, while patients with CURB-65 score 3 or above should be assessed for ICU admission [Reference Wunderink and Waterer19]. Here we found the cut-off value of 2 had better performance on diagnostic statistics and univariate COX model to predict in-hospital death. This lower cut-off might also imply a faster progression of COVID-19 compared with other CAP, which may need earlier intervention.
Our study has some limitations. First, due to the retrospective study design, laboratory tests or vital signs were incomplete in some patients. Second, patients were sometimes transferred late in their illness to the included hospital, which may interfere with observation of the disease process. Third, by excluding patients still in hospital as of 29 February 2020, and thus relatively more severe disease at an earlier stage, the case fatality ratio in our study cannot reflect the true mortality of COVID-19. Last but not least, the interpretation of our findings might be limited by the sample size, which needs to be confirmed in large-scale multicentre research.
Conclusion
CURB-65 may serve as a useful prognostic marker in COVID-19 patients, which could be used to quickly triage severe patients in primary care or general practice settings. CURB-65 ⩾2 may serve as a cut-off value for the prediction of in-hospital death. Low serum albumin, elevated LDH levels and high CURB-65 score could help clinicians to identify at an early stage those patients with COVID-19 who have a poor prognosis.
Supplementary material
The supplementary material for this article can be found at https://doi.org/10.1017/S0950268820002368
Acknowledgements
We acknowledge all health-care workers involved in the diagnosis and treatment of patients in the Union Hospital, Tongji Medical College Huazhong University of Science and Technology. We thank Dr Yong Xue for suggestions on writing.
Author contributions
J.G., B.Z., M.Z. and Y.Y. contributed equally to this paper and share joint first authorship. P.H. and P.Z. contributed equally to this paper and share joint corresponding authorship. J.G., M.Z., H.Z., X.W., Q.W. and P.H. treated patients and collected data. B.Z., Yifang Yuan, T.L., S.L., P.L., Ying Yang, P.Z. designed research and performed statistics, J.G., B.Z., M.Z., Yifang Yuan, P.H. and P.Z. wrote the paper. All authors reviewed and approved the final version. P.Z. is the guarantor of the paper, taking responsibility for the integrity of the work as a whole.
Financial support
This study is supported by funding from Grant 81970299 from the National Natural Science Foundation of China, Beijing Hospitals Authority Ascent Plan (No. DFL20190902), Beijing Hospitals Authority Clinical medicine development of special funding support (No. ZYLX201831) and The capital health research and development of special (No. 2020-4-2243).
Role of the funders/sponsors
The funders had no role in study design, data collection and analysis, decision to publish or preparation of the manuscript.
Conflict of interest
All authors declare no competing interests.
Ethical standards
This study received approval from the Research Ethics Committee of the Union Hospital, Tongji Medical College Huazhong University of Science and Technology, Wuhan, China (2020-0213). The Research Ethics Committee waived the requirement of informed consent before the study started because of the urgent need to collect clinical data. We analysed all the data anonymously.
Data availability statement
The data that support the findings of this study are available from the corresponding author. Restrictions apply to the availability of these data, which were used under licence for this study. Data are available upon request with the permission of corresponding author.