INTRODUCTION
Contact networks of individuals affect their exposure to infectious contacts and are therefore a crucial determinant of infection risk [Reference Adimora and Schoenbach1]. Thus, an individual's risk of infection not only depends on individual-level risk factors, such as gender, but on network-level risk factors. A classic example of this particularity of infectious diseases is herd protection: vaccinating a portion of the population reduces the chance of non-vaccinated individuals being exposed to the infectious agent [Reference Fine, Eames and Heymann2]. Hence, an individual's risk of infection following the introduction of vaccination depends on his/her vaccine status (individual-level risk factor), and the overall population-level vaccination coverage (network-level risk factor).
In observational studies that examine risk factors of sexually transmitted infections (STIs), control of confounding most often follows the traditional non-transmissible disease approach of controlling for individual-level risk factors (such as the subject's sexual activity), with little attention to control for network-level risk factors such as sexual activity of individuals in the subject's network. In doing so, authors usually acknowledge the possibility of misclassification of sexual activity (e.g. number of partners), due to misreporting by study subjects, which can cause residual confounding if sexual activity is associated with the risk factors [Reference Schlecht3, Reference Furber4]. What is less acknowledged in these studies is that, even if the sexual activity of the study subjects was perfectly measured and controlled for, the sexual activity of individuals in the subject's network can also confound the biological effect of a risk factor on acquisition of infection. We term one particular form of such confounding ‘assortativity bias’, because it stems from assortativity in sexual mixing (partner choice). That is, on average, people have partners with similar characteristics (e.g. age) and behaviour (e.g. smoking status) as themselves [Reference Doherty5–Reference Clark and Etile8].
In STI studies, assortativity bias can occur if two conditions are met: (C1) partner choice is assortative according to the risk factor of interest, and (C2) the association between this factor and infection is confounded by sexual activity. When these conditions are met, the risk factor of interest can be associated with the likelihood of having an infected partner, and confounding is likely to remain even after perfectly controlling the effect measure for individual-level confounders.
To illustrate assortativity bias, we consider a simplified example where the effect of smoking on the risk of a STI is examined. Thus, smoking is the exposure variable and the occurrence of a STI is the outcome. In this example, assortativity bias can occur because the two conditions above are met: sexual mixing is assortative according to smoking status (C1) [Reference Agrawal6, Reference Clark and Etile8], and smokers have a higher average level of sexual activity (C2) [Reference Vaccarella9, Reference Cavazos-Rehg10].
Figure 1 illustrates the essential components of assortativity bias, assuming, for simplicity, that smoking is the only factor for which partner selection is assortative and that smoking itself has no biological effect on STI acquisition/transmission or duration. In Figure 1, the study subject's smoking status is positively associated with the smoking status of his partner [assortative mixing by smoking status (C1)]. A subject who smokes will be at greater risk of infection not only because of his own sexual activity, but also because his/her partner is likely to be a smoker and thus more likely to be more sexually active and infected. The smoking–STI relationship is confounded by sexual activity of the subjects (Fig. 1, right panel, dotted arrows) and subjects’ partners (Fig. 1, left panel, dotted arrows). Therefore, even if sexual activity of the subjects is controlled for (individual level), residual confounding bias remains possible due to sexual activity of partners (network level). Of note, having a partner that smokes not only induces greater risk through the partner's greater chance of being highly sexually active, but also through the partner's own previous partners who were also more likely to be smokers. Hence, the assortativity bias ultimately reflects differences in sexual network of smokers compared to non-smokers, and not only differences in sexual activity of subjects’ current partners. Even if we assume a biological effect of smoking on STI, the assortativity bias would still occur resulting in an overestimation of this effect. Given that C1 and C2 are met for many common risk factors of STIs, including age, race and socioeconomic status (SES), and the assortativity bias affects measures of STI acquisition (e.g. incidence rate and prevalence ratios), the bias is likely present in many prospective and cross-sectional epidemiological STI studies.
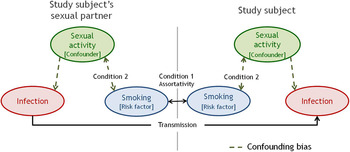
Fig. 1. Illustrated example of the assortativity bias in the association between smoking and a sexually transmitted infection. Direction arrows represent causal link and double-headed arrows represent statistical link. For simplicity, we assume that smoking status is the only factor determining partner selection and that smoking does not affect risk and duration of infection.
Mathematical modelling has been used to understand potential biases in epidemiological studies of STIs [Reference Boily and Anderson11–Reference Koopman15]. With modelling, an artificial world is created where transmission and natural history of disease can be simulated based on model inputs which are either assumed or fitted to empirically observed data. Epidemiological studies can be nested within the model to examine potential biases under different assumptions regarding behaviour, transmission, and natural history.
In this paper, we examine the assortativity bias focusing on the smoking–STI association. Smoking is a suspected risk factor for STIs through increased transmission and/or duration of infection [Reference Wolf and Freedman16]. As smoking is a modifiable behaviour, there is a high interest in understanding its role on STI incidence/prevalence. Smoking has been independently associated with the prevalence of STIs such as human immunodeficiency virus (HIV), herpes simplex virus 2 (HSV-2), genital and oral infections by human papillomavirus (HPV) in many studies [Reference Furber4, Reference Vaccarella9, Reference Gillison17–Reference Sellors19], and these associations have been shown to follow monotone dose-response relationships [Reference Vaccarella9]. However, the possibility of assortativity bias was not addressed in these studies.
The specific objectives of this paper are to use mathematical modelling: (1) to illustrate and describe the assortativity bias, using as an example the association between smoking and HPV infection, and (2) to examine the sensitivity of the assortativity bias to biological and behavioural parameters, for generalization of results.
METHODS
Mathematical model
We developed a deterministic transmission-dynamic model of HPV infection (see Supplementary material for a list of the model's equations). The modelled population is heterosexual, open and stable. For the base-case scenario, we modelled HPV16 infection, which is the most prevalent and oncogenic type. The simulated population is stratified for the two behavioural aspects from which the assortativity bias stems: (1) smoking status (smoker/non-smoker), and (2) sexual activity (low/high). For simplicity, we did not stratify the model by age. On average, individuals spend 30 years in the modelled population, representing the years of higher sexually active life (ages 15–44 years). Sexual mixing depends on an individual's smoking status and sexual activity. For each of these behavioural factors, we allowed mixing to vary from random to completely assortative (smokers only form partnerships with smokers). We included assortativity according to sexual activity as it is a key feature of sexual networks [Reference Granath20, Reference Aral21]. Based on empirical evidence, we assume that the two conditions for the assortativity bias are met: (C1) sexual mixing is assortative for smoking [Reference Agrawal6, Reference Clark and Etile8], and (C2) smokers are more sexually active than non-smokers [Reference Vaccarella9, Reference Cavazos-Rehg10].
Importantly, in our base case, we assumed that smoking has no biological effect (smoking does not increase HPV transmission probabilities or duration), to test whether observed associations between smoking and HPV infection can be explained by the assortativity bias.
Parameterization
Model parameter values and references are presented in Table 1. We used biological parameter values estimated in prior modelling work [Reference Van de Velde22–Reference Bogaards24], and estimated the proportion of smokers in each sexual activity group from an epidemiological study [Reference Drolet25]. Although studies suggest that sexual mixing by level of sexual activity and smoking status is assortative [Reference Agrawal6, Reference Clark and Etile8, Reference Manhart26, Reference Liljeros, Edling and Nunes Amaral27], no empirical estimates of assortativity are available in the literature. In our base case, we assumed assortativity parameter values for smoking status and for sexual activity to be 0·8 and 0·4, respectively (0·0 = random, 1·0 = complete assortativity), using equations presented in the Supplementary material. We performed extensive sensitivity analysis on mixing parameters given their uncertainty.
Table 1. Base-case model parameter values

Experimental design and outcome measure
To examine the association between smoking and HPV, we nested a prevalence study in the simulated population. Study subjects are a cross-section of the simulated population. HPV prevalence was estimated at endemic equilibrium without HPV vaccination with perfect sensitivity and specificity. We used odds ratios (ORs) of HPV infection (positivity) in smokers compared to non-smokers as the measure of association. The overall adjusted ORs were calculated as the weighted average of the stratum-specific ORs of the two sexual activity groups using, as weights, the proportion of the population in each sexual activity group (see Supplementary material for the equations used to compute ORs). In the simulated study, adjusted or stratum-specific ORs different from 1·00 can only be due to assortativity bias, because there is no biological effect of smoking in our model, sexual activity of subjects is perfectly adjusted for. The magnitude of the assortativity bias is the magnitude of the deviation of the adjusted and stratum-specific ORs from 1·00. Hence, the simulation reproduces the conduct of a perfect study with no other biases, be it misclassification or confounding, other than the assortativity bias. Because the simulated population is at equilibrium and the duration of infection is assumed to be unaffected by smoking, the incidence rate ratios from nested longitudinal studies in our model have the same numerical value to the ORs from cross-sectional studies: a result given by the formula:

Sensitivity analyses
We varied the key biological/behavioural parameters, one at a time, keeping the value of all other parameters fixed at their base-case values. We used the stratum-specific ORs to isolate the bias in each sexual activity group. Finally, we estimated the potential impact of the assortativity bias on adjusted ORs assuming different magnitudes of a true biological effect of smoking on infection.
RESULTS
Base case
Table 2 shows the base-case model predictions of the ORs of HPV infection in smokers compared to non-smokers. We estimated crude and adjusted ORs of 1·64 and 1·51, respectively (Table 2). Given that, in our model, smoking has no causal effect on HPV and we can perfectly control for sexual activity of subjects (no residual confounding), assortativity bias is the only possible cause of adjusted OR > 1·00.
Table 2. Odds ratios of HPV infection between smokers and non-smokers in modelled study subjects
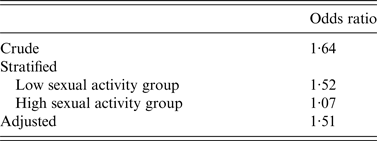
The magnitude of assortativity bias is generally lower for those with greater sexual activity (Table 2, Figs. 2, 3). This is because highly sexually active subjects will likely have highly sexually active partners (assortativity by sexual activity), irrespective of smoking status.

Fig. 2. Impact of behavioural parameters on the assortativity bias. Univariate sensitivity analysis of the odds ratios of prevalence between smokers and non-smokers with one parameter varying: (a) proportion of smokers that are highly sexually active, (b) assortativity by smoking status, and (c) assortativity by sexual activity. For panel (a), the proportion of non-smokers that are highly sexually active is fixed at its base-case value. Hence, increasing the parameter in (a) increases the strength of the association between smoking and sexual activity.

Fig. 3. Impact of biological parameters on the assortativity bias. Univariate sensitivity analysis of the odds ratios of infection between smokers and non-smokers varying: (a) probability of transmission per partnership, (b) duration of infection, and (c) probability of developing natural immunity after clearance of infection.
Impact of behavioural factors
Association between sexual activity and smoking
The ORs of HPV infection (assortativity bias) increase as the strength of the association between smoking and sexual activity in study subjects increases (Fig. 2a ). This is because a stronger association causes greater confounding by sexual activity (increased impact of C2, Fig. 1).
Assortativity by smoking status
Greater assortativity according to smoking status results in a steep increase in the ORs of HPV infection comparing smokers with non-smokers (Fig. 2b ). When smoking assortativity is stronger, the imbalance in sexual activity between smokers and non-smokers will be replicated between smokers’ partners and non-smokers’ partners to a greater extent (increased impact of C1, Fig. 1).
Assortativity by sexual activity
The ORs of HPV infection decrease with greater assortativity between individuals of the same sexual activity group (Fig. 2c ). As assortativity by sexual activity increases, the sexual activity of the study subject becomes a better proxy of his/her partners’ sexual activity. When mixing by sexual activity is completely assortative, subjects will have partners belonging to their own sexual activity group, irrespective of smoking status. Therefore, there will be no bias after adjustment for sexual activity.
Impact of biological factors
Transmission probability and duration of infectiousness
The model shows that the magnitude of the assortativity bias is highly sensitive to the transmission probability or duration of infection (Fig. 3a , b ). The ORs of HPV infection in smokers compared to non-smokers, stratified by sexual activity of study subjects, decreases steeply with increased transmission probability or duration of infectiousness. In general, if the reproductive number (R 0) is low (i.e. low transmission probability, short duration or low partner acquisition rate), the difference in sexual activity between smokers and non-smokers can lead to large differences in HPV prevalence between the two groups.
Natural immunity
The probability of developing natural immunity has little impact on the magnitude of the assortativity bias (Fig. 3c ). Lower natural immunity has the same relative impact on HPV prevalence in both smokers and non-smokers.
Assortativity bias assumes a true biological effect of smoking
Figure 4 shows the OR when varying the effect of smoking on the duration of infection with and without assortativity by smoking status. The assortativity bias produces an overestimation of the OR when smokers have a longer duration of infection than non-smokers. This overestimation rises steeply as the biological effect of smoking increases. The OR is also overestimated when smoking affects the transmission probability, or the probability of developing natural immunity (results not shown).

Fig. 4. Impact of a biological effect of smoking on the duration of infection. Univariate sensitivity analysis of the odds ratio of prevalence between smokers and non-smokers varying: ratio of smokers’ vs. non-smokers’ duration of infection. Two scenarios are shown both with base-case parameters except for the assortativity by smoking status: the blue curve is a scenario with assortativity parameter of 0·8 as in the base case and the dashed curve is a scenario without assortativity (parameter of 0). Hence, the difference in height between the two curves measures the magnitude of the overestimation due to the assortativity bias.
DISCUSSION
In this paper we present the assortativity bias, a frequently unrecognized confounding bias specific to studies examining risk factors of infectious diseases. To illustrate this bias, we considered the example of smoking as a possible biological cause of HPV infection. Using mathematical modelling, we showed that adjustment for the subjects’ individual-level sexual activity is insufficient to attribute the association between smoking and HPV to a biological effect when mixing is assortative by smoking status (C1) and smoking status is associated with sexual activity (C2). There is empirical evidence that these two conditions hold for smoking [Reference Agrawal6, Reference Clark and Etile8–Reference Cavazos-Rehg10], and many other risk factors of STIs such as age, race/ethnicity and SES. Hence, the assortativity bias is likely present in many epidemiological studies examining risk factors of STIs.
Our modelling analysis suggests that the assortativity bias could produce ORs of the magnitude seen in empirical studies on HPV if assortativity by smoking status is high. In a recent large-scale study, the adjusted ORs of HPV infection in smokers compared to non-smokers was 1·4 (95% confidence interval 1·2–1·7) [Reference Vaccarella9], and most other studies have found ORs higher than 1·0 [Reference Sellors19, Reference Ley28–Reference Burk30]. The association between smoking and HPV infection is supported by traditional criteria of causality such as dose-response. However, the assortativity bias can produce a dose-response relationship if: (C1) mixing is assortative by smoking intensity and (C2) there is a dose-response relationship between sexual activity and smoking intensity. Significant associations between infection and smoking have also been observed in empirically based studies for many other STIs [Reference Furber4, Reference Gillison17, Reference Beachler18]. We also showed that the size of the assortativity bias should be more important for STIs such as HIV, which have low R 0.
Our results should not be interpreted as evidence that smoking is not a cause of HPV infection. Smoking may have a direct biological influence on HPV risk by negatively affecting mucosal immunity and/or by consuming micronutrients that mediate resistance to or clearance of HPV infection [Reference Franco and Spence31]. When we assume, in our model, that smoking is a biological cause of HPV infection, the assortativity bias greatly increases the adjusted ORs beyond the true biological effect. In addition, it is important to note that the magnitude of the assortativity bias may vary substantially between studies due to differences in the behaviour of participants (differences in the magnitude of C1–C2).
The assortativity bias could affect many risk factors other than smoking, such as age and race. For example, young adults are generally the most at risk of STIs, even after adjustment for sexual activity of subjects [Reference Manhart26, Reference Wheeler32]. It is suggested that this is due to a biological cause (e.g. cervical ectopy makes young women vulnerable to STIs) [Reference Chinsembu33]. Yet, sexual mixing is highly assortative with respect to age [Reference Aral21, Reference Johnson34] (C1), and younger adults are more sexually active [Reference Johnson34, Reference Chandra35] (C2), and hence an age–STI association can be partly due to the assortativity bias. For other risk factors, complete assortativity between individuals with the risk factor can hold automatically and cause assortativity bias in prevalence studies. For example, in a cross-sectional study examining HPV as a risk factor of another STI, a subject infected with HPV will have a previous/current partner also infected with HPV. However, subjects’ partners infected with HPV will have greater sexual activity on average and thus higher risk of other STIs. Hence, HPV can be identified in prevalence studies as a risk factor of other STIs, due to the assortativity bias.
The main strength of this study was the use of mathematical modelling to perfectly control a fictive population, allowing us to explore the theoretical basis for the bias and the relationship between the bias and behavioural and biological parameters. However, the main limitation of our model is that many sources of heterogeneity (sexual activity, smoking intensity) were not included and we assumed independence between mixing by sexual activity and by smoking status. Greater heterogeneity in sexual activity would require specifying in C2 that the association between sexual activity and smoking is monotonic, which seems to be the case [Reference Drolet25]. Furthermore, we did not include in the model other factors that could cause assortativity by smoking status. For example, SES is a risk factor for smoking [Reference Hiscock36, Reference Laumann, Michael and Michaels37], and sexual mixing is assortative by SES [Reference Laumann, Michael and Michaels37], which indirectly produces assortativity with respect to smoking. In this case, the bias would be partly corrected by adjustment for the SES of study subjects. These model simplifications do not affect the robustness of our overall qualitative conclusions. However, one should not use the precise model OR estimates as being representative of reality.
To correct for the assortativity bias in studies examining risk factors of STIs, one must control for systematic differences in exposure to infection that can occur between individuals with and without a given risk factor (e.g. smoking). To control for differences in exposure to infection, studies have restricted their population to individuals known to have been exposed to infection. For instance, studies examining risk factors of HIV transmission have used populations of serodiscordant couples [Reference Cohen38, Reference Zhang39], where the uninfected partner is known to be exposed. However, such studies are rarely performed for other STIs, as they are costly and methodologically challenging (difficult to adequately condition on exposure to infection). Randomized trials on the other hand would suffer from the assortativity bias if the treatment is a cause of assortativity and if subjects can acquire sexual partnership after the randomization. If sexual partnerships are stable from the randomization until the end of follow-up, there remains the difficulty of interpreting the measure of effect because of the absence of conditioning on exposure to infection. Furthermore, not all causal factors can be investigated in randomized trials (e.g. smoking, age) and only one factor can be examined per trial. Hence, most studies examining STI risk factors are based on cross-sectional or prospective data, where infection status and risk factors are assessed without specific data on exposure to infection. For such studies, the characteristics of the study subjects’ sexual partners should be used to reduce the assortativity bias. Taking the example of smoking, the smoking status of new sexual partners of study subjects should be assessed in prospective studies to control for the higher chance of smokers having partners who are smokers. In addition, information on past partners would also be needed, with a recall window depending on duration of infection. Given that many risk factors are investigated at once in empirical studies, it is also necessary to have simultaneous adjustments for the key risk factors being investigated (e.g. age, race/ethnicity, SES) at the subject- and partner-level.
In conclusion, assortative sexual mixing by smoking status can cause bias in studies assessing the biological effect of smoking on HPV acquisition. For a thorough adjustment of measures of association, data on risk factors of sexual partners of study subjects is required to mitigate the impact of the bias.
SUPPLEMENTARY MATERIAL
For supplementary material accompanying this paper visit http://dx.doi.org/10.1017/S0950268815002915.
ACKNOWLEDGEMENTS
This work was supported by the Canadian Institutes of Health Research (GSD- 130 809 to P.L.M.), and the Canada Research Chair programme (to M.B.). The funding sources had no involvement in the study design and conduct of the study; and preparation, review, or approval of the manuscript.
DECLARATION OF INTEREST
Over the past 3 years, M.B. has received an unrestricted grant from Merck (herpes zoster - none are ongoing). Although unrelated to the content and message of the manuscript, M.D. has consulted for GlaxoSmithKline (herpes zoster vaccine); E.L.F. receives occasional consultancy fees from companies involved with HPV diagnostics (Roche, Qiagen, Gen-Probe) and has also served as an occasional paid consultant to companies involved with HPV vaccines (Merck, GSK).