2076 results in Statistical Physics
5 - Polymers near a surface
-
- Book:
- Lattice Models of Polymers
- Published online:
- 08 January 2010
- Print publication:
- 30 April 1998, pp 74-88
-
- Chapter
- Export citation
7 - Dense polymers
-
- Book:
- Lattice Models of Polymers
- Published online:
- 08 January 2010
- Print publication:
- 30 April 1998, pp 104-118
-
- Chapter
- Export citation
Frontmatter
-
- Book:
- Lattice Models of Polymers
- Published online:
- 08 January 2010
- Print publication:
- 30 April 1998, pp i-viii
-
- Chapter
- Export citation
6 - Percolation, spanning trees and the Potts model
-
- Book:
- Lattice Models of Polymers
- Published online:
- 08 January 2010
- Print publication:
- 30 April 1998, pp 89-103
-
- Chapter
- Export citation
References
-
- Book:
- Lattice Models of Polymers
- Published online:
- 08 January 2010
- Print publication:
- 30 April 1998, pp 210-219
-
- Chapter
- Export citation
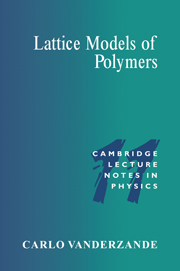
Lattice Models of Polymers
-
- Published online:
- 08 January 2010
- Print publication:
- 30 April 1998
2 - Excluded volume and the self avoiding walk
-
- Book:
- Lattice Models of Polymers
- Published online:
- 08 January 2010
- Print publication:
- 30 April 1998, pp 19-37
-
- Chapter
- Export citation
3 - The SAW in d = 2
-
- Book:
- Lattice Models of Polymers
- Published online:
- 08 January 2010
- Print publication:
- 30 April 1998, pp 38-61
-
- Chapter
- Export citation
11 - Self avoiding surfaces
-
- Book:
- Lattice Models of Polymers
- Published online:
- 08 January 2010
- Print publication:
- 30 April 1998, pp 194-209
-
- Chapter
- Export citation
1 - From polymers to random walks
-
- Book:
- Lattice Models of Polymers
- Published online:
- 08 January 2010
- Print publication:
- 30 April 1998, pp 1-18
-
- Chapter
- Export citation
8 - Self interacting polymers
-
- Book:
- Lattice Models of Polymers
- Published online:
- 08 January 2010
- Print publication:
- 30 April 1998, pp 119-148
-
- Chapter
- Export citation
4 - The SAW in d = 3
-
- Book:
- Lattice Models of Polymers
- Published online:
- 08 January 2010
- Print publication:
- 30 April 1998, pp 62-73
-
- Chapter
- Export citation
Contents
-
- Book:
- Lattice Models of Polymers
- Published online:
- 08 January 2010
- Print publication:
- 30 April 1998, pp ix-x
-
- Chapter
- Export citation
10 - Polymer topology
-
- Book:
- Lattice Models of Polymers
- Published online:
- 08 January 2010
- Print publication:
- 30 April 1998, pp 176-193
-
- Chapter
- Export citation
Index
-
- Book:
- Lattice Models of Polymers
- Published online:
- 08 January 2010
- Print publication:
- 30 April 1998, pp 220-222
-
- Chapter
- Export citation
11 - Immiscible lattice gases in three dimensions
-
- Book:
- Lattice-Gas Cellular Automata
- Published online:
- 23 September 2009
- Print publication:
- 28 August 1997, pp 128-140
-
- Chapter
- Export citation
9 - Immiscible lattice gases
-
- Book:
- Lattice-Gas Cellular Automata
- Published online:
- 23 September 2009
- Print publication:
- 28 August 1997, pp 106-118
-
- Chapter
- Export citation
Appendix F - Hydrodynamic response to forces at fluid interfaces
-
- Book:
- Lattice-Gas Cellular Automata
- Published online:
- 23 September 2009
- Print publication:
- 28 August 1997, pp 284-287
-
- Chapter
- Export citation
Appendix E - Derivation of the Gibbs distribution
-
- Book:
- Lattice-Gas Cellular Automata
- Published online:
- 23 September 2009
- Print publication:
- 28 August 1997, pp 281-283
-
- Chapter
- Export citation
Contents
-
- Book:
- Lattice-Gas Cellular Automata
- Published online:
- 23 September 2009
- Print publication:
- 28 August 1997, pp ix-xiv
-
- Chapter
- Export citation