2076 results in Statistical Physics
Preface
-
- Book:
- Self-Organised Criticality
- Published online:
- 05 September 2012
- Print publication:
- 30 August 2012, pp xiii-xvi
-
- Chapter
- Export citation
10 - Summary and discussion
- from Part III - Theory
-
- Book:
- Self-Organised Criticality
- Published online:
- 05 September 2012
- Print publication:
- 30 August 2012, pp 346-356
-
- Chapter
- Export citation
9 - Mechanisms
- from Part III - Theory
-
- Book:
- Self-Organised Criticality
- Published online:
- 05 September 2012
- Print publication:
- 30 August 2012, pp 317-345
-
- Chapter
- Export citation
Part I - Introduction
-
- Book:
- Self-Organised Criticality
- Published online:
- 05 September 2012
- Print publication:
- 30 August 2012, pp 1-2
-
- Chapter
- Export citation
Part III - Theory
-
- Book:
- Self-Organised Criticality
- Published online:
- 05 September 2012
- Print publication:
- 30 August 2012, pp 241-242
-
- Chapter
- Export citation
Appendix: The OLAMI–FEDER–CHRISTENSEN Model in C
-
- Book:
- Self-Organised Criticality
- Published online:
- 05 September 2012
- Print publication:
- 30 August 2012, pp 357-390
-
- Chapter
- Export citation
Author index
-
- Book:
- Self-Organised Criticality
- Published online:
- 05 September 2012
- Print publication:
- 30 August 2012, pp 459-473
-
- Chapter
- Export citation
Frontmatter
-
- Book:
- Self-Organised Criticality
- Published online:
- 05 September 2012
- Print publication:
- 30 August 2012, pp i-vi
-
- Chapter
- Export citation
2 - Scaling
- from Part I - Introduction
-
- Book:
- Self-Organised Criticality
- Published online:
- 05 September 2012
- Print publication:
- 30 August 2012, pp 25-51
-
- Chapter
- Export citation
7 - Numerical methods and data analysis
- from Part II - Models and numerics
-
- Book:
- Self-Organised Criticality
- Published online:
- 05 September 2012
- Print publication:
- 30 August 2012, pp 210-240
-
- Chapter
- Export citation
Part II - Models and numerics
-
- Book:
- Self-Organised Criticality
- Published online:
- 05 September 2012
- Print publication:
- 30 August 2012, pp 79-84
-
- Chapter
- Export citation
List of symbols
-
- Book:
- Self-Organised Criticality
- Published online:
- 05 September 2012
- Print publication:
- 30 August 2012, pp xvii-xxii
-
- Chapter
- Export citation
6 - Stochastic sandpiles
- from Part II - Models and numerics
-
- Book:
- Self-Organised Criticality
- Published online:
- 05 September 2012
- Print publication:
- 30 August 2012, pp 162-209
-
- Chapter
- Export citation
Subject index
-
- Book:
- Self-Organised Criticality
- Published online:
- 05 September 2012
- Print publication:
- 30 August 2012, pp 474-494
-
- Chapter
- Export citation
Notes
-
- Book:
- Self-Organised Criticality
- Published online:
- 05 September 2012
- Print publication:
- 30 August 2012, pp 391-397
-
- Chapter
- Export citation
4 - Deterministic sandpiles
- from Part II - Models and numerics
-
- Book:
- Self-Organised Criticality
- Published online:
- 05 September 2012
- Print publication:
- 30 August 2012, pp 85-110
-
- Chapter
- Export citation
References
-
- Book:
- Self-Organised Criticality
- Published online:
- 05 September 2012
- Print publication:
- 30 August 2012, pp 398-458
-
- Chapter
- Export citation
Foreword by Henrik J. Jensen
-
-
- Book:
- Self-Organised Criticality
- Published online:
- 05 September 2012
- Print publication:
- 30 August 2012, pp xi-xii
-
- Chapter
- Export citation
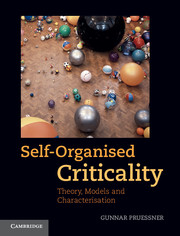
Self-Organised Criticality
- Theory, Models and Characterisation
-
- Published online:
- 05 September 2012
- Print publication:
- 30 August 2012
Contents
-
- Book:
- Self-Organised Criticality
- Published online:
- 05 September 2012
- Print publication:
- 30 August 2012, pp vii-ix
-
- Chapter
- Export citation