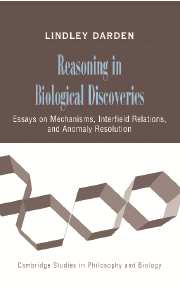
Book contents
- Frontmatter
- Contents
- Long Contents
- List of Figures
- List of Tables
- Acknowledgments
- Introduction
- PART I BIOLOGICAL MECHANISMS
- PART II REASONING STRATEGIES: RELATING FIELDS, RESOLVING ANOMALIES
- 5 Interfield Theories with Nancy Maull
- 6 Theory Construction in Genetics
- 7 Relations Among Fields in the Evolutionary Synthesis
- 8 Selection Type Theories with Joseph A. Cain
- 9 Strategies for Anomaly Resolution: Diagnosis and Redesign
- 10 Exemplars, Abstractions, and Anomalies: Representations and Theory Change in Mendelian and Molecular Genetics
- 11 Strategies for Anomaly Resolution in the Case of Adaptive Mutation
- PART III DISCOVERING MECHANISMS: CONSTRUCTION, EVALUATION, REVISION
- Bibliography
- Index
- References
9 - Strategies for Anomaly Resolution: Diagnosis and Redesign
Published online by Cambridge University Press: 31 August 2009
- Frontmatter
- Contents
- Long Contents
- List of Figures
- List of Tables
- Acknowledgments
- Introduction
- PART I BIOLOGICAL MECHANISMS
- PART II REASONING STRATEGIES: RELATING FIELDS, RESOLVING ANOMALIES
- 5 Interfield Theories with Nancy Maull
- 6 Theory Construction in Genetics
- 7 Relations Among Fields in the Evolutionary Synthesis
- 8 Selection Type Theories with Joseph A. Cain
- 9 Strategies for Anomaly Resolution: Diagnosis and Redesign
- 10 Exemplars, Abstractions, and Anomalies: Representations and Theory Change in Mendelian and Molecular Genetics
- 11 Strategies for Anomaly Resolution in the Case of Adaptive Mutation
- PART III DISCOVERING MECHANISMS: CONSTRUCTION, EVALUATION, REVISION
- Bibliography
- Index
- References
Summary
INTRODUCTION
Understanding the growth of scientific knowledge has been a major task in philosophy of science. No successful general model of scientific change has been found; attempts were made by, for example, Kuhn (1970), Toulmin (1972), Lakatos (1970), and Laudan (1977). A different approach is to view science as a problem-solving enterprise. The goal is to find both general and domain-specific heuristics (i.e., reasoning strategies) for problem solving. Such heuristics produce plausible but not infallible results (Nickles 1987; Thagard 1988).
Viewing science as a problem-solving enterprise and scientific reasoning as a special form of problem solving owes much to cognitive science and artificial intelligence (AI) (e.g., Langley et al. 1987). Key issues in AI are representation and reasoning. More specifically, AI studies methods for representing knowledge and methods for manipulating computationally represented knowledge. From the perspective of philosophy of science, the general issues of representation and reasoning become how to represent scientific theories and how to find strategies for reasoning in theory change. Reasoning in theory change is viewed as problem solving, and implementations in AI computer programs provide tools for investigating methods of problem solving. This approach is called “computational philosophy of science” (Thagard 1988).
Huge amounts of data are now available in online databases. The time is now ripe for automating scientific reasoning, first, to form empirical generalizations about patterns in the data (Langley et al. 1987); then, to construct new explanatory theories; and, finally, to improve them over time in the light of anomalies.
- Type
- Chapter
- Information
- Reasoning in Biological DiscoveriesEssays on Mechanisms, Interfield Relations, and Anomaly Resolution, pp. 207 - 228Publisher: Cambridge University PressPrint publication year: 2006