Book contents
- Frontmatter
- Contents
- Preface
- 1 Introduction
- 2 Foundations of Smooth Optimization
- 3 Descent Methods
- 4 Gradient Methods Using Momentum
- 5 Stochastic Gradient
- 6 Coordinate Descent
- 7 First-Order Methods for Constrained Optimization
- 8 Nonsmooth Functions and Subgradients
- 9 Nonsmooth Optimization Methods
- 10 Duality and Algorithms
- 11 Differentiation and Adjoints
- Appendix
- Bibliography
- Index
- References
Bibliography
Published online by Cambridge University Press: 31 March 2022
- Frontmatter
- Contents
- Preface
- 1 Introduction
- 2 Foundations of Smooth Optimization
- 3 Descent Methods
- 4 Gradient Methods Using Momentum
- 5 Stochastic Gradient
- 6 Coordinate Descent
- 7 First-Order Methods for Constrained Optimization
- 8 Nonsmooth Functions and Subgradients
- 9 Nonsmooth Optimization Methods
- 10 Duality and Algorithms
- 11 Differentiation and Adjoints
- Appendix
- Bibliography
- Index
- References
Summary
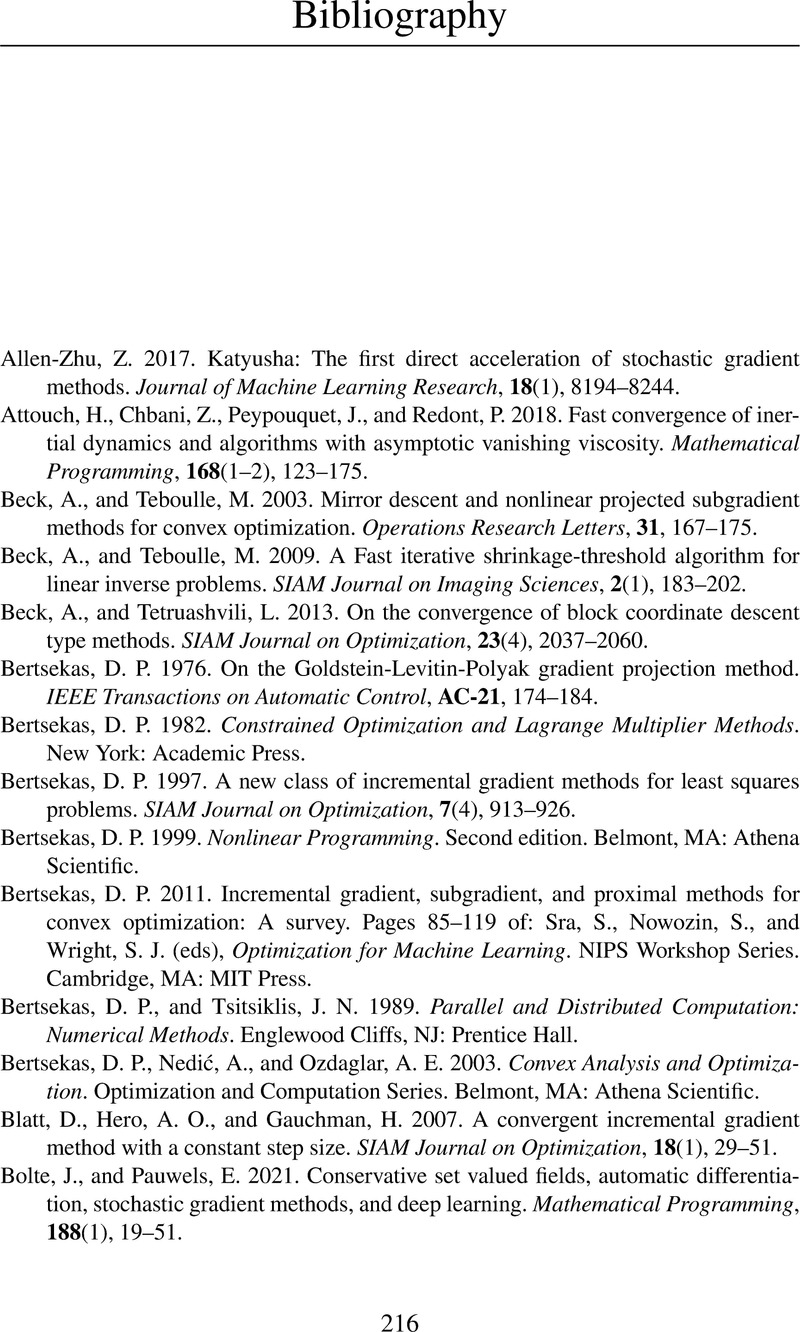
- Type
- Chapter
- Information
- Optimization for Data Analysis , pp. 216 - 222Publisher: Cambridge University PressPrint publication year: 2022