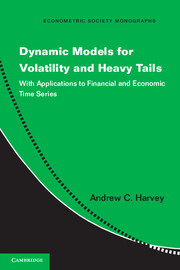
Book contents
- Frontmatter
- Contents
- Preface
- Acronyms and Abbreviations
- 1 Introduction
- 2 Statistical Distributions and Asymptotic Theory
- 3 Location
- 4 Scale
- 5 Location/Scale Models for Non-negative Variables
- 6 Dynamic Kernel Density Estimation and Time-Varying Quantiles
- 7 Multivariate Models, Correlation and Association
- 8 Conclusions and Further Directions
- A Derivation of Formulae in the Information Matrix
- B Autocorrelation Functions
- C GED Information Matrix
- D The Order of GARCH Models
- E Computer Programs
- Bibliography
- Author Index
- Subject Index
- Other titles in the series
8 - Conclusions and Further Directions
Published online by Cambridge University Press: 05 May 2013
- Frontmatter
- Contents
- Preface
- Acronyms and Abbreviations
- 1 Introduction
- 2 Statistical Distributions and Asymptotic Theory
- 3 Location
- 4 Scale
- 5 Location/Scale Models for Non-negative Variables
- 6 Dynamic Kernel Density Estimation and Time-Varying Quantiles
- 7 Multivariate Models, Correlation and Association
- 8 Conclusions and Further Directions
- A Derivation of Formulae in the Information Matrix
- B Autocorrelation Functions
- C GED Information Matrix
- D The Order of GARCH Models
- E Computer Programs
- Bibliography
- Author Index
- Subject Index
- Other titles in the series
Summary
Many nonlinear unobserved components models can be approximated by analogous observation-driven models in which the dynamics are driven by the score of the conditional distribution. Given a judicious choice of link function, everything falls into place. When the dynamic conditional score model is taken to be the true model, the asymptotic theory for maximum likelihood estimation can be developed, and in many cases, an analytic expression can be derived for the information matrix. Furthermore, maximum likelihood estimation seems to work well in practice. In the applications reported here, convergence of the likelihood function for the models estimated was fast and reliable.
In much of the literature, the way in which observation-driven models are constructed is essentially arbitrarily. The use of the conditional score in models associated with unobserved components parameter-driven models provides guidance and discipline, as well as a unified approach to nonlinear time series modelling. Even when the asymptotic theory cannot be developed along the lines set out here, as is the case with any parameter associated with the distributions typically employed for count data and qualitative observations, the evidence suggests that the conditional score is still the best way forward.
A theory of testing, both before and after model estimation, is also developed, and the evidence suggests that it is appropriate and effective. For example, the Lagrange multiplier tests for serial correlation are robust to heavy tails, and the tests based on the probability integral transform are useful for assessing the validity of distributional assumptions.
- Type
- Chapter
- Information
- Dynamic Models for Volatility and Heavy TailsWith Applications to Financial and Economic Time Series, pp. 231 - 232Publisher: Cambridge University PressPrint publication year: 2013