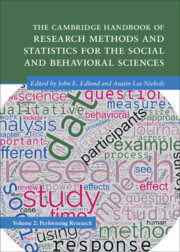
Book contents
- The Cambridge Handbook of Research Methods and Statistics for the Social and Behavioral Sciences
- Cambridge Handbooks in Psychology
- The Cambridge Handbook of Research Methods and Statistics for the Social and Behavioral Sciences
- Copyright page
- Dedication
- Contents
- Figures
- Tables
- Contributors
- Preface
- Part I Quantitative Data Collection Sources
- 1 Student Samples in Research
- 2 Mechanical Turk: A Versatile Tool in the Behavioral Scientist’s Toolkit
- 3 Social Media Research
- 4 Prolific: Crowdsourcing Academic Online Research
- 5 Field Research
- 6 Organizational Research
- 7 Integrating Culture in Research
- 8 Mixed Methods and Multimethod Research
- Part II Important Methodological Considerations
- Part III Self-Report Measures
- Part IV Behavioral Measures
- Part V Physiological Measures
- Part VI Qualitative Data Collection Sources
- Index
- References
1 - Student Samples in Research
from Part I - Quantitative Data Collection Sources
Published online by Cambridge University Press: 12 December 2024
- The Cambridge Handbook of Research Methods and Statistics for the Social and Behavioral Sciences
- Cambridge Handbooks in Psychology
- The Cambridge Handbook of Research Methods and Statistics for the Social and Behavioral Sciences
- Copyright page
- Dedication
- Contents
- Figures
- Tables
- Contributors
- Preface
- Part I Quantitative Data Collection Sources
- 1 Student Samples in Research
- 2 Mechanical Turk: A Versatile Tool in the Behavioral Scientist’s Toolkit
- 3 Social Media Research
- 4 Prolific: Crowdsourcing Academic Online Research
- 5 Field Research
- 6 Organizational Research
- 7 Integrating Culture in Research
- 8 Mixed Methods and Multimethod Research
- Part II Important Methodological Considerations
- Part III Self-Report Measures
- Part IV Behavioral Measures
- Part V Physiological Measures
- Part VI Qualitative Data Collection Sources
- Index
- References
Summary
This chapter provides an overview on the use and validity of student samples in the behavioral and social sciences. In some instances, data collected from students can be of limited value or even inappropriate; however, in other cases, this approach provides useful data. I offer three general ways to evaluate the use of student samples. First, consider the research design. Descriptive studies that rely on students to draw inferences about the overall population are likely problematic. Second, statistical controls such as multivariate analyses that adjust for other factors may reduce some of the biases that may be introduced through sampling. Third, consider the theorized mechanism – a clear theoretical mechanism that does not vary based on the demographics of the sample allows us to put more faith in constrained samples. Despite these approaches, and regardless of our methods, statistics, and theoretical mechanism, we should be cautious with generalizability claims.
- Type
- Chapter
- Information
- The Cambridge Handbook of Research Methods and Statistics for the Social and Behavioral SciencesVolume 2: Performing Research, pp. 3 - 23Publisher: Cambridge University PressPrint publication year: 2024