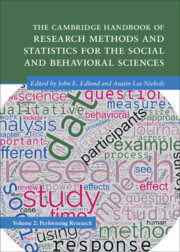
Book contents
- The Cambridge Handbook of Research Methods and Statistics for the Social and Behavioral Sciences
- Cambridge Handbooks in Psychology
- The Cambridge Handbook of Research Methods and Statistics for the Social and Behavioral Sciences
- Copyright page
- Dedication
- Contents
- Figures
- Tables
- Contributors
- Preface
- Part I Quantitative Data Collection Sources
- 1 Student Samples in Research
- 2 Mechanical Turk: A Versatile Tool in the Behavioral Scientist’s Toolkit
- 3 Social Media Research
- 4 Prolific: Crowdsourcing Academic Online Research
- 5 Field Research
- 6 Organizational Research
- 7 Integrating Culture in Research
- 8 Mixed Methods and Multimethod Research
- Part II Important Methodological Considerations
- Part III Self-Report Measures
- Part IV Behavioral Measures
- Part V Physiological Measures
- Part VI Qualitative Data Collection Sources
- Index
- References
4 - Prolific: Crowdsourcing Academic Online Research
from Part I - Quantitative Data Collection Sources
Published online by Cambridge University Press: 12 December 2024
- The Cambridge Handbook of Research Methods and Statistics for the Social and Behavioral Sciences
- Cambridge Handbooks in Psychology
- The Cambridge Handbook of Research Methods and Statistics for the Social and Behavioral Sciences
- Copyright page
- Dedication
- Contents
- Figures
- Tables
- Contributors
- Preface
- Part I Quantitative Data Collection Sources
- 1 Student Samples in Research
- 2 Mechanical Turk: A Versatile Tool in the Behavioral Scientist’s Toolkit
- 3 Social Media Research
- 4 Prolific: Crowdsourcing Academic Online Research
- 5 Field Research
- 6 Organizational Research
- 7 Integrating Culture in Research
- 8 Mixed Methods and Multimethod Research
- Part II Important Methodological Considerations
- Part III Self-Report Measures
- Part IV Behavioral Measures
- Part V Physiological Measures
- Part VI Qualitative Data Collection Sources
- Index
- References
Summary
Prolific is a website that offers researchers the ability to recruit and sample participants for online research. In contrast to earlier crowdsourcing platforms, such as Amazon Mechanical Turk (MTurk), it focuses primarily on academic and marketing research – typically done through online surveys and experiments. In this chapter, I aim to introduce this platform to researchers conducting online studies and to provide knowledge and practical advice on how to best use the platform for online research. The review includes explanations of how the site works, the composition of its pool of participants, the options available to researchers for sampling and recruiting participants online, how to achieve advanced abilities by connecting Prolific to research software (e.g., Qualtrics, Gorilla), and how to ensure high data quality when using Prolific. I then review the evidence on the current state of data quality on Prolific, suggesting that it can provide higher data quality than MTurk and also better than some commercial panels. I conclude with a summary of the advantages and disadvantages of using Prolific for online research and potential future developments in the platform that could promote more credible online research.
- Type
- Chapter
- Information
- The Cambridge Handbook of Research Methods and Statistics for the Social and Behavioral SciencesVolume 2: Performing Research, pp. 72 - 92Publisher: Cambridge University PressPrint publication year: 2024