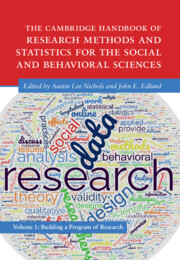
Book contents
- The Cambridge Handbook of Research Methods and Statistics for the Social and Behavioral Sciences
- Cambridge Handbooks in Psychology
- The Cambridge Handbook of Research Methods and Statistics for the Social and Behavioral Sciences
- Copyright page
- Dedication
- Contents
- Figures
- Tables
- Contributors
- Preface
- Part I From Idea to Reality: The Basics of Research
- 1 Promises and Pitfalls of Theory
- 2 Research Ethics for the Social and Behavioral Sciences
- 3 Getting Good Ideas and Making the Most of Them
- 4 Literature Review
- 5 Choosing a Research Design
- 6 Building the Study
- 7 Analyzing Data
- 8 Writing the Paper
- Part II The Building Blocks of a Study
- Part III Data Collection
- Part IV Statistical Approaches
- Part V Tips for a Successful Research Career
- Index
- References
6 - Building the Study
from Part I - From Idea to Reality: The Basics of Research
Published online by Cambridge University Press: 25 May 2023
- The Cambridge Handbook of Research Methods and Statistics for the Social and Behavioral Sciences
- Cambridge Handbooks in Psychology
- The Cambridge Handbook of Research Methods and Statistics for the Social and Behavioral Sciences
- Copyright page
- Dedication
- Contents
- Figures
- Tables
- Contributors
- Preface
- Part I From Idea to Reality: The Basics of Research
- 1 Promises and Pitfalls of Theory
- 2 Research Ethics for the Social and Behavioral Sciences
- 3 Getting Good Ideas and Making the Most of Them
- 4 Literature Review
- 5 Choosing a Research Design
- 6 Building the Study
- 7 Analyzing Data
- 8 Writing the Paper
- Part II The Building Blocks of a Study
- Part III Data Collection
- Part IV Statistical Approaches
- Part V Tips for a Successful Research Career
- Index
- References
Summary
This chapter discusses the key elements involved when building a study. Planning empirical studies presupposes a decision about whether the major goal of the study is confirmatory (i.e., tests of hypotheses) or exploratory in nature (i.e., development of hypotheses or estimation of effects). Focusing on confirmatory studies, we discuss problems involved in obtaining an appropriate sample, controlling internal and external validity when designing the study, and selecting statistical hypotheses that mirror the substantive hypotheses of interest. Building a study additionally involves decisions about the to-be-employed statistical test strategy, the sample size required by this strategy to render the study informative, and the most efficient way to achieve this so that study costs are minimized without compromising the validity of inferences. Finally, we point to the many advantages of study preregistration before data collection begins.
Keywords
- Type
- Chapter
- Information
- The Cambridge Handbook of Research Methods and Statistics for the Social and Behavioral SciencesVolume 1: Building a Program of Research, pp. 103 - 124Publisher: Cambridge University PressPrint publication year: 2023