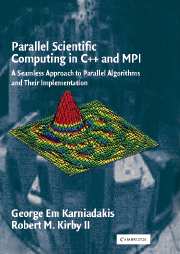
Book contents
- Frontmatter
- Contents
- Preface
- Acknowledgments
- 1 Scientific Computing and Simulation Science
- 2 Basic Concepts and Tools
- 3 Approximation
- 4 Roots and Integrals
- 5 Explicit Discretizations
- 6 Implicit Discretizations
- 7 Relaxation: Discretization and Solvers
- 8 Propagation: Numerical Diffusion and Dispersion
- 9 Fast Linear Solvers
- 10 Fast Eigensolvers
- A C++ Basics
- B MPI Basics
- Bibliography
- Index
6 - Implicit Discretizations
Published online by Cambridge University Press: 05 October 2013
- Frontmatter
- Contents
- Preface
- Acknowledgments
- 1 Scientific Computing and Simulation Science
- 2 Basic Concepts and Tools
- 3 Approximation
- 4 Roots and Integrals
- 5 Explicit Discretizations
- 6 Implicit Discretizations
- 7 Relaxation: Discretization and Solvers
- 8 Propagation: Numerical Diffusion and Dispersion
- 9 Fast Linear Solvers
- 10 Fast Eigensolvers
- A C++ Basics
- B MPI Basics
- Bibliography
- Index
Summary
In this chapter we consider implicit discretizations of space and time derivatives. Unlike the explicit discretizations presented in the previous chapter, here we express a derivative at one grid point in terms of function values as well as derivative values at adjacent grid points (spatial discretization) or in terms of previous and current time levels (temporal discretization). This, in turn, implies that there is implicit coupling, and thus matrix inversion is required to obtain the solution.
The material of this chapter serves to introduce solutions of tridiagonal systems and correspondingly parallel computing of sparse linear systems using MPI. We also introduce two new MPI functions: MPI_Barrier, used for synchronizing processes, and MPI_Wtime, used for obtaining the wall-clock timing information.
IMPLICIT SPACE DISCRETIZATIONS
The discretizations we present here are appropriate for any order spatial derivative involved in a partial differential equation, but they are particularly useful when high-order accuracy and locality of data is sought. The explicit finite differences could also lead to high accuracy but at the expense of long stencils, and this, in turn, implies coupling involving many grid points and consequently a substantial communications overhead. In contrast, the implicit finite differences employ very compact stencils and guarantee locality, which is the key to success of any parallel implementation. We only consider discretizations on uniform (i.e., equidistant) grids, as we assume that a mapping of the form presented in the previous chapter is always available to transform a nonuniform grid to a uniform one. We also present discretizations only for one-dimensional grids since multidimensional discretizations are accomplished using directional splitting, as before.
- Type
- Chapter
- Information
- Parallel Scientific Computing in C++ and MPIA Seamless Approach to Parallel Algorithms and their Implementation, pp. 309 - 346Publisher: Cambridge University PressPrint publication year: 2003