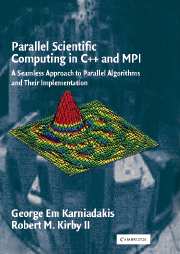
Book contents
- Frontmatter
- Contents
- Preface
- Acknowledgments
- 1 Scientific Computing and Simulation Science
- 2 Basic Concepts and Tools
- 3 Approximation
- 4 Roots and Integrals
- 5 Explicit Discretizations
- 6 Implicit Discretizations
- 7 Relaxation: Discretization and Solvers
- 8 Propagation: Numerical Diffusion and Dispersion
- 9 Fast Linear Solvers
- 10 Fast Eigensolvers
- A C++ Basics
- B MPI Basics
- Bibliography
- Index
10 - Fast Eigensolvers
Published online by Cambridge University Press: 05 October 2013
- Frontmatter
- Contents
- Preface
- Acknowledgments
- 1 Scientific Computing and Simulation Science
- 2 Basic Concepts and Tools
- 3 Approximation
- 4 Roots and Integrals
- 5 Explicit Discretizations
- 6 Implicit Discretizations
- 7 Relaxation: Discretization and Solvers
- 8 Propagation: Numerical Diffusion and Dispersion
- 9 Fast Linear Solvers
- 10 Fast Eigensolvers
- A C++ Basics
- B MPI Basics
- Bibliography
- Index
Summary
In this chapter we introduce methods for solutions of the standard eigenvalue problem
Ax = λx,
where A is a square n × n matrix, as well as for generalized eigenproblems. The main theory is based on the solvers of the previous chapter for linear systems. Unlike, however, the methods of the previous chapter where both direct and iterative approaches are effective, in eigenvalue problems only iterative solvers are efficient. We start with the simple power method and its variants, and we proceed with more sophisticated methods including a method for nonsymmetric eigenproblems using the Arnoldi iteration. We classify the different eigensolvers as local or global depending on whether they are typically used to compute one or two eigenvalues or the entire spectrum, respectively.
We introduce one new MPI function, MPI_Alltoall, and demonstrate its use through some of the algorithms presented in this chapter. In addition, we reiterate the use of MPI_Allgather and MPI_Allreduce through example implementations of algorithms.
LOCAL EIGENSOLVERS
We have already seen in Chapter 2 that computing the eigenvalues accurately from the determinant may not always be possible. The Newton-Raphson method of Chapter 4 is an accurate method of computing the roots of the characteristic polynomial, but it can be extremely inefficient for large systems. In the following, we present a simple method to compute iteratively the maximum and minimum eigenvalues and corresponding eigenvectors. We can also compute any other eigenvalue for which we have a good initial guess.
- Type
- Chapter
- Information
- Parallel Scientific Computing in C++ and MPIA Seamless Approach to Parallel Algorithms and their Implementation, pp. 538 - 574Publisher: Cambridge University PressPrint publication year: 2003