Article contents
Patients prefer artificial intelligence to a human provider, provided the AI is better than the human: A commentary on Longoni, Bonezzi and Morewedge (2019)
Published online by Cambridge University Press: 01 January 2023
Abstract
We call attention to an important, but overlooked finding in research reported by Longoni, Bonezzi and Morewedge (2019). Longoni et al. claim that people always prefer a human to an artificially intelligent (AI) medical provider. We show that this was only the case when the historical performance of the human and AI providers was equal. When the AI is known to outperform the human, their data showed a clear preference for the automated provider. We provide additional statistical analyses of their data to support this claim.
Keywords
- Type
- Article Commentary
- Information
- Creative Commons
- The authors license this article under the terms of the Creative Commons Attribution 3.0 License.
- Copyright
- Copyright © The Authors [2020] This is an Open Access article, distributed under the terms of the Creative Commons Attribution license (http://creativecommons.org/licenses/by/3.0/), which permits unrestricted re-use, distribution, and reproduction in any medium, provided the original work is properly cited.
Footnotes
1 Study 3C provided complication rates instead of accuracy rates such that a lower value suggested better performance. For simplicity, we refer only to accuracy throughout this paper.
References
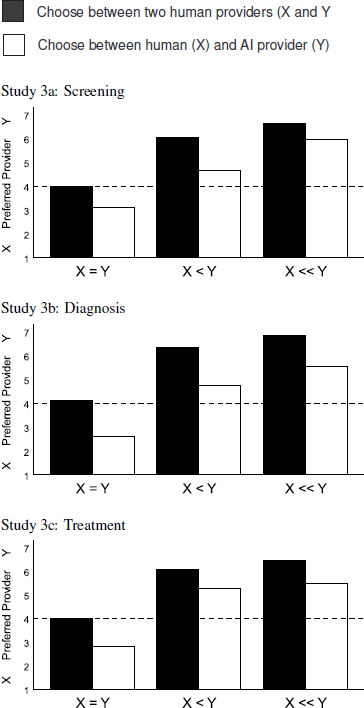
Figure 1: Preference for human vs. AI providers as a function of relative accuracy (adapted from Longoni et al. 2019, Figure 2). A response of 4 indicates indifference.
- 9
- Cited by
1 Introduction
Reference Longoni, Bonezzi and MorewedgeLongoni, Bonezzi and Morewedge (2019) recently reported a series of ten clever experiments demonstrating that people prefer to receive medical care (i.e., diagnosis, screening, and treatment) from a human provider rather than from an equally competent artificially intelligent computer (AI provider). Particularly interesting is their finding that perceived “uniqueness neglect” on the part of an AI provider can explain this preference.
There is an important message, however, that we believe became lost among the other interesting findings: People actually did prefer the AI provider so long as it outperformed the human provider. The effects of accuracy could not be tested in seven of their ten studies, either because participants received incomplete accuracy information that did not allow for a direct comparison between human and computer (Studies 1 and 4) or were explicitly told that the human and computer had equal accuracy rates (Studies 2, 5, 6, 7 and 8). We believe, however, that the findings of Studies 3A to 3C provide strong support that people do sometimes embrace AI.
Studies 3A to 3C were identical in design, differing only in the type of service provided (screening for skin cancer, triaging a potential emergency, surgical implant of pacemaker). Participants rated provider X (human) and Y (human or comupter) on a 7-point scale such that scores above the midpoint of the scale indicated a preference for Y, below the midpoint indicated a preference for X, and scores exactly at 4 indicated indifference. One variable that they manipulated was the relative accuracyFootnote 1 rate of the X and Y providers. Accuracy rates were either equal (X = Y), slightly favored provider Y (X < Y), or strongly favored provider Y (X << Y). They also manipulated choice set: Provider X was always human, but provider Y was either another human or a computer (AI provider). Longoni et al. report significant main effects of both relative accuracy and choice set, but no interaction (see Figure 1). Before discussing the results, here is their stated goal for these three studies:
Figure 1: Preference for human vs. AI providers as a function of relative accuracy (adapted from Reference Longoni, Bonezzi and MorewedgeLongoni et al. 2019, Figure 2). A response of 4 indicates indifference.
Our main hypothesis was that participants would be more reluctant to choose an automated provider if a human provider was available, even when the automated provider performed better than the human provider (p. 7).
First, their reported main effect shows that, overall, increases in accuracy for provider Y did lead to a preference for this provider. On the far left of each graph in Figure 1, we see that when accuracy rates are identical (X = Y), ratings are at or below the midpoint, indicating either indifference between humans (black bars) or a preference for the human over AI (white bars). This replicates the findings of studies 2, and 5–8. However, when accuracy rates are better for provider Y (X < Y or X << Y), mean responses increased above the midpoint of the scale indicating a preference for provider Y. The lack of an interaction showed this to be the case regardless of whether provider Y was another human (black bars) or a computer AI (white bars). Clearly, participants did not always resist AI technology. In fact, they arguably embraced AI when its historical performance was described as being better than that of the human (Reference Carlson, Desai, Drury, Kwak and YancoCarlson et al., 2011). Note that the effect size for accuracy is quite large, with partial eta squared values of η2p = .445 to .479. These effects are 2 to 3 times larger than effects for choice set (η2p = .131 to .222).
Using the means and standard deviations provided in their Table 2, and assuming that the sample size was equal across conditions, we calculated the one-sided lower 95% confidence limit on each reported mean. In all six conditions involving a superior automated provider, the lower bound for the mean response was above the null value of 4.0 (i.e., the value indicating no preference) suggesting that participants preferred the more accurate automated provider to the less accurate human one.
Study 3A:
X < Y: M = 4.64, lower limit = 4.07; X << Y: M = 5.97, lower limit = 5.60.
Study 3B:
X < Y: M = 4.75, lower limit = 4.21; X << Y: M = 5.54, lower limit = 5.07.
Study 3C:
X < Y: M = 5.25, lower limit = 4.81; X << Y: M = 5.48, lower limit = 5.11.
Given these lower bounds, we were surprised that Longoni et al. made the following claim:
Together, studies 3A-3C provided evidence that consumer resistance to medical AI emerges across a variety of medical domains. Resistance to medical AI was robust across … providers’ performance rates (i.e., accuracy/success vs. complications/failure)…. Participants were resistant to medical AI even when the performance of AI providers was explicitly specified to be superior to that of human providers. (p. 8)
We respectfully disagree with this conclusion. The data reported in studies 3A-3C show that accuracy rates did matter, and that when they favor the AI provider, consumers did not resist medical AI. We do not dispute the findings of the pairwise comparisons, but argue that these comparisons don’t ask the right question. Remember, the black bars represent a choice between two human providers; only the white bars represent a choice between a human and AI provider. Thus, the pairwise comparisons merely show that participants didn’t prefer superior AI over an inferior human as much as they preferred a superior human over an inferior human. The fact remains, however, that, when given the choice, participants did prefer the more accurate AI provider to the less accurate human.
We should be clear that we are not arguing that algorithm aversion (Reference Dietvorst, Simmons and MasseyDietvorst, Simmons & Massey, 2015) doesn’t exist. Rather, we are merely noting that its effects did not overwhelm the effects of accuracy. This is important, because a long history of research comparing clinical and actuarial judgment has shown that in most cases algorithms are more accurate than humans (Reference Dawes, Faust and MeehlDawes, Faust & Meehl, 1989; Reference KleinmuntzKleinmuntz, 1990; Reference Grove, Zald, Lebow, Snitz and NelsonGrove, Zald, Lebow, Snitz & Nelson, 2000). Longoni et al. acknowledge this fact, and further suggest that “given the superior accuracy of statistical models over human intuition, people should prefer to follow the advice of statistical models….” (p. 2). We take an optimistic view of their data and suggest that this is exactly what they found.