Introduction
Our understanding of the evolution of the avian genome has recently been updated by the landmark avian phylogenomic paper by Jarvis et al. (Reference Jarvis, Mirarab, Aberer, Li, Houde, Li, Ho, Faircloth, Nabholz, Howard, Suh, Weber, da Fonseca, Li, Zhang, Li, Zhou, Narula, Liu, Ganapathy, Boussau, Bayzid, Zavidovych, Subramanian, Gabaldón, Capella-Gutiérrez, Huerta-Cepas, Rekepalli, Munch, Schierup, Lindow, Warren, Ray, Green, Bruford, Zhan, Dixon, Li, Li, Huang, Derryberry, Bertelsen, Sheldon, Brumfield, Mello, Lovell, Wirthlin, Schneider, Prosdocimi, Samaniego, Vargas Velazquez, Alfaro-Núñez, Campos, Petersen, Sicheritz-Ponten, Pas, Bailey, Scofield, Bunce, Lambert, Zhou, Perelman, Driskell, Shapiro, Xiong, Zeng, Liu, Li, Liu, Wu, Xiao, Yinqi, Zheng, Zhang, Yang, Wang, Smeds, Rheindt, Braun, Fjeldsa, Orlando, Barker, Jønsson, Johnson, Koepfli, O'Brien, Haussler, Ryder, Rahbek, Willerslev, Graves, Glenn, McCormack, Burt, Ellegren, Alström, Edwards, Stamatakis, Mindell, Cracraft, Braun, Warnow, Jun, Gilbert and Zhang2014). This phylogeny includes all extant orders from Neoaves (e.g. songbirds, parrots, pigeons) and species from several clades in the more basal orders including Anseriformes (waterfowl), Galliformes (landfowl), Tinamiformes (tinamous) and Struthioniformes (ostrich). The phylogeny supports the rapid radiation of the most derived order Passeriformes (i.e. perching birds) and their sister taxon, the parrots (Psittaciformes). This diversification occurred around 60–55 million years ago during the Late Paleocene in Gondwana (Gill, Reference Gill1995; Jarvis et al., Reference Jarvis, Mirarab, Aberer, Li, Houde, Li, Ho, Faircloth, Nabholz, Howard, Suh, Weber, da Fonseca, Li, Zhang, Li, Zhou, Narula, Liu, Ganapathy, Boussau, Bayzid, Zavidovych, Subramanian, Gabaldón, Capella-Gutiérrez, Huerta-Cepas, Rekepalli, Munch, Schierup, Lindow, Warren, Ray, Green, Bruford, Zhan, Dixon, Li, Li, Huang, Derryberry, Bertelsen, Sheldon, Brumfield, Mello, Lovell, Wirthlin, Schneider, Prosdocimi, Samaniego, Vargas Velazquez, Alfaro-Núñez, Campos, Petersen, Sicheritz-Ponten, Pas, Bailey, Scofield, Bunce, Lambert, Zhou, Perelman, Driskell, Shapiro, Xiong, Zeng, Liu, Li, Liu, Wu, Xiao, Yinqi, Zheng, Zhang, Yang, Wang, Smeds, Rheindt, Braun, Fjeldsa, Orlando, Barker, Jønsson, Johnson, Koepfli, O'Brien, Haussler, Ryder, Rahbek, Willerslev, Graves, Glenn, McCormack, Burt, Ellegren, Alström, Edwards, Stamatakis, Mindell, Cracraft, Braun, Warnow, Jun, Gilbert and Zhang2014). Passeriformes account for more than half of all avian species, are found on every continent except for Antarctica, and occupy a diverse range of habitats (Gill, Reference Gill1995). Although birds as a whole appear to have evolved at a conservative molecular evolutionary rate when contrasted with other large groups of organisms (notably mammals), passeriform songbirds have an average mutation rate that is close to twice that of other avian species (Jarvis et al., Reference Jarvis, Mirarab, Aberer, Li, Houde, Li, Ho, Faircloth, Nabholz, Howard, Suh, Weber, da Fonseca, Li, Zhang, Li, Zhou, Narula, Liu, Ganapathy, Boussau, Bayzid, Zavidovych, Subramanian, Gabaldón, Capella-Gutiérrez, Huerta-Cepas, Rekepalli, Munch, Schierup, Lindow, Warren, Ray, Green, Bruford, Zhan, Dixon, Li, Li, Huang, Derryberry, Bertelsen, Sheldon, Brumfield, Mello, Lovell, Wirthlin, Schneider, Prosdocimi, Samaniego, Vargas Velazquez, Alfaro-Núñez, Campos, Petersen, Sicheritz-Ponten, Pas, Bailey, Scofield, Bunce, Lambert, Zhou, Perelman, Driskell, Shapiro, Xiong, Zeng, Liu, Li, Liu, Wu, Xiao, Yinqi, Zheng, Zhang, Yang, Wang, Smeds, Rheindt, Braun, Fjeldsa, Orlando, Barker, Jønsson, Johnson, Koepfli, O'Brien, Haussler, Ryder, Rahbek, Willerslev, Graves, Glenn, McCormack, Burt, Ellegren, Alström, Edwards, Stamatakis, Mindell, Cracraft, Braun, Warnow, Jun, Gilbert and Zhang2014; Zhang et al., Reference Zhang, Li, Li, Li, Larkin, Lee, Storz, Antunes, Greenwold, Meredith, Ödeen, Cui, Zhou, Xu, Pan, Wang, Jin, Zhang, Hu, Yang, Hu, Xiao, Yang, Liu, Xie, Yu, Lian, Wen, Zhang, Li, Zeng, Xiong, Liu, Zhou, Huang, An, Wang, Zheng, Xiong, Wang, Wang, Wang, Fan, da Fonseca, Alfaro-Núñez, Schubert, Orlando, Mourier, Howard, Ganapathy, Pfenning, Whitney, Rivas, Hara, Smith, Farré, Narayan, Slavov, Romanov, Borges, Machado, Khan, Springer, Gatesy, Hoffmann, Opazo, Håstad, Sawyer, Kim, Kim, Kim, Cho, Li, Huang, Bruford, Zhan, Dixon, Bertelsen, Derryberry, Warren, Wilson, Li, Ray, Green, O'Brien, Griffin, Johnson, Haussler, Ryder, Willerslev, Graves, Alström, Fjeldså, Mindell, Edwards, Braun, Rahbek, Burt, Houde, Zhang, Yang, Wang, Avian Genome, Jarvis, Gilbert and Wang2014).
Immune genes are shaped by selection pressure from an onslaught of coevolving pathogens (Hendrick, Reference Hedrick1998; Acevedo-Whitehouse and Cunningham, Reference Acevedo-Whitehouse and Cunningham2006; Piertney and Oliver, Reference Piertney and Oliver2006). The genes of the major histocompatibility complex (MHC) have been the most useful for understanding the adaptive immune response in wildlife, particularly in birds (Acevedo-Whitehouse and Cunningham, Reference Acevedo-Whitehouse and Cunningham2006; Turner et al., Reference Turner, Begon, Jackson and Paterson2012), and many other systems (natural and model or experimental) have been used to explore gene-for-gene coevolutionary processes (reviewed in Brockhurst and Koskella, Reference Brockhurst and Koskella2013). However, the role of selection in shaping diversity in other aspects of the immune system, such as innate immunity, remains poorly understood (Acevedo-Whitehouse and Cunningham, Reference Acevedo-Whitehouse and Cunningham2006; Vinkler and Albrecht, Reference Vinkler and Albrecht2009; Grueber et al., Reference Grueber, Wallis, King and Jamieson2012, Reference Grueber, Wallis and Jamieson2014).
To date, the only non-MHC immune genes to be extensively studied in birds are Toll-like receptors (TLR). TLRs are membrane-bound sensors of the innate immune system that recognize invariant and distinctive molecular features of invading microbes and are essential for initiating adaptive and innate immune responses in vertebrates. These types of receptors are part of a large family of proteins called pattern-recognition receptors (PRRs). The genetic variation at TLR genes has been directly related to differential pathogen responses in birds, humans and livestock (Alcaide and Edwards, Reference Alcaide and Edwards2011; Grueber et al., Reference Grueber, Wallis, King and Jamieson2012; Grueber et al., Reference Grueber, Wallis and Jamieson2014; Bateson, et al., Reference Bateson, Hammerly, Johnson, Morrow, Whittingham and Dunn2016; Raven et al., Reference Raven, Lisovski, Klaassen, Lo, Madsen, Ho and Ujvari2017; Kannaki et al., Reference Kannaki, Reddy, Raja Ravindra and Chatterjee2018; Nelson-Flower et al., Reference Nelson-Flower, Germain, MacDougall-Shackleton, Taylor and Arcese2018). Prior research has shown that vertebrate TLR evolution is mostly characterized by purifying (negative) selection (Barreiro et al., Reference Barreiro, Ben-Ali, Quach, Laval, Patin, Pickrell, Bouchier, Tichit, Neyrolles, Gicquel, Kidd, Kidd, Alcaïs, Ragimbeau, Pellegrini, Abel, Casanova and Quintana-Murci2009; Alcaide and Edwards, Reference Alcaide and Edwards2011; Nelson-Flower et al., Reference Nelson-Flower, Germain, MacDougall-Shackleton, Taylor and Arcese2018) and, to a lesser extent, balancing selection (Ferrer-Admetlla et al Reference Ferrer-Admetlla, Bosch, Sikora, Marquès-Bonet, Ramírez-Soriano, Muntasell, Navarro, Lazarus, Calafell, Bertranpetit and Casals2008). However, Grueber et al. (Reference Grueber, Wallis and Jamieson2014) also found evidence of episodic positive selection in avian TLRs. This discovery was aided by the doubling of avian transcriptomic and genomic data made available at the time of their research (Grueber et al., Reference Grueber, Wallis and Jamieson2014). These new data allowed Grueber et al. (Reference Grueber, Wallis and Jamieson2014) to evaluate up to 23 bird species per gene and gave added resolution in discerning patterns of selection.
More generally, PRRs are highly conserved and found in a diverse range of animal species. They provide the foundation upon which the hosts' immune response to pathogens has evolved (e.g. Bagheri and Zahmatkesh, Reference Bagheri and Zahmatkesh2018). PRRs account for many other families of sensors beside TLRs (Acevedo-Whitehouse and Cunningham, Reference Acevedo-Whitehouse and Cunningham2006). These include soluble components (e.g. lipopolysaccharide-binding protein, collectins and pentraxins) and cell-associated components (e.g. TLRs, C-type lectins, NOD-like receptors, RIG-I like receptors, scavenger receptors, formyl-peptide receptors and various intracellular receptors; Chen et al., Reference Chen, Cheng and Wang2013). Pattern recognition is considered to occur at three different levels. These are interactions involving soluble extracellular PRRs, membrane-bound PRRs and PRRs found in the cytoplasm (see also Zhang et al., Reference Zhang, Gao, Yu, Zhang, Wang and Zhou2018). Most major pathogen-response groups are included in one or more of these three levels. The redundancy in recognition of particular pathogen-associated molecular patterns and coordination of different PRRs to sense any single invading class of microorganism is an important feature of the PRR network. The outcome of PRR-mediated responses is to initiate downstream immune cascades that represent the first stage in establishing the immune response. These cascades are in turn mediated by a large group of regulatory proteins called cytokines. Cytokines include interleukins, interferons, tumour necrosis factors and chemokines (Chen et al., Reference Chen, Cheng and Wang2013). Variation in cytokine genes has been associated with certain pathogens in humans, but relatively little research is available for birds, and what is available is primarily limited to fowl (e.g. Chhabra et al., Reference Chhabra, Chantrey and Ganapathy2015; Kannaki et al., Reference Kannaki, Reddy, Raja Ravindra and Chatterjee2018).
PRR immune genes have shown signatures of selection that can be associated with specific bird species or evolutionary families throughout the avian phylogenetic tree (Grueber et al., Reference Grueber, Wallis and Jamieson2014). For example, chickens, unlike ducks, geese and finches, lack RIG-I, the PRR sensor used to detect highly pathogenic avian influenza. Instead, they express a gene called the melanoma differentiation-associated gene 5 (MDA5), which compensates for the missing RIG-I (Chen et al., Reference Chen, Cheng and Wang2013; Magor et al., Reference Magor, Navarro, Barber, Petkau, Fleming-Canepa, Blyth and Blaine2013). Some PRR-induced interferon genes are missing from birds completely. These include interferon genes ISG15, ISG54 and ISG56 (see Velova et al., Reference Velová, Gutowska-Ding, Burt and Vinkler2018). Birds as a whole have a reduced repertoire of immune genes when compared to mammals (Magor et al., Reference Magor, Navarro, Barber, Petkau, Fleming-Canepa, Blyth and Blaine2013), and because of this, we could predict that avian PRRs are evolutionarily constrained (Chen et al., Reference Chen, Cheng and Wang2013; Magor et al., Reference Magor, Navarro, Barber, Petkau, Fleming-Canepa, Blyth and Blaine2013; Grueber et al., Reference Grueber, Wallis and Jamieson2014).
To explore PRR evolution in birds, we conducted an in silico analysis of bird gene sequences in the GenBank. Additionally, we examined gene expression differences in Northern cardinals uninfected by haemosporidians, infected by Plasmodium haemosporidians, or infected by Haemoproteus haemosporidians. The computational analyses suggest that some avian PRRs are under positive, diversifying selection, while the differential gene expression analysis highlighted the differences in response among naturally-occurring ‘treatment’ groups.
Methods
Sequence mining and alignment
Using annotations of immune genes from the GenBank, we used BLAST against the known genomes and transcriptomes of birds and aligned 47 individual gene datasets (i.e. fasta files). We included most PRRs that we could align with at least 250 base pairs (bp), some non-PRR genes and some from the adaptive immune system with at least 250 bp; we did not include all the TLRs because they had been analysed in another study (Grueber et al., Reference Grueber, Wallis and Jamieson2014). We then trimmed each down to contiguous alignments for each taxon that were aligned at a first codon position and ended at a third codon position using webPrank (https://www.ebi.ac.uk/goldman-srv/webprank/). There were no ambiguities, stop codons or missing data in any alignment, and all genes were checked for recombination using RDP4 (Martin et al., Reference Martin, Murrell, Golden, Khoosal and Muhire2015). Numbers of taxa per dataset ranged from 18 to 57, and gene regions ranged in length from 279 to 2346 bp. Only coding regions were used in these analyses. All gene alignments and MEME results are freely available in the Dryad Depository (doi:10.5061/dryad.r5594f0).
Characterization of gene evolution
We analysed each dataset to determine patterns of selection using several methods within the HyPhy platform (via the Adaptive Evolution server at DataMonkey.org; Kosakovsky Pond and Frost, Reference Kosakovsky Pond and Frost2005; Delport et al., Reference Delport, Poon, Frost and Kosakovsky Pond2010). The single-likelihood ancestor counting (SLAC; Kosakovsky Pond and Frost, Reference Kosakovsky Pond and Frost2005) method was used to determine the overall d N/d S per gene dataset. The fixed-effects likelihood (FEL; Kosakovsky Pond and Frost, Reference Kosakovsky Pond and Frost2005) method was used to determine the number of sites (codons) under negative selection; FEL fits a site-specific likelihood model on a per-codon basis and then determines whether d N is significantly greater than d S. FEL reconstructs the phylogeny using maximum likelihood while optimizing parameters. The mixed effects model of evolution (MEME; Murrell et al., Reference Murrell, Wertheim, Moola, Weighill, Scheffler and Kosakovsky Pond2012) was then used to determine the number of positively-selected sites; MEME essentially fits a likelihood model assuming a background d N/d S and determines, on a site-by-site basis, whether d N is greater than the background rate. Like FEL, MEME reconstructs the phylogeny using maximum likelihood while optimizing parameters. Finally, even with the limitations recently pointed out by Venkat et al. (Reference Venkat, Hahn and Thornton2018), we used adaptive branch-site REL (aBSREL; Kosakovsky Pond et al., Reference Kosakovsky Pond, Murrell, Fourment, Frost, Delport and Scheffler2011; Smith et al., Reference Smith, Wertheim, Weaver, Murrell, Scheffler and Kosakovsky Pond2011), a branch-based rather than site-based model, to determine if and where episodic diversifying selection has occurred in branches within trees reconstructed with distance methods (per the programme).
Comparative transcriptome analyses
In a previous study (Walstrom and Outlaw, Reference Walstrom and Outlaw2016), we collected blood samples from Northern cardinals (Cardinalis cardinalis). All samples were adult (after hatch-year) females. We determined whether each animal was (1) uninfected with haemosporidians, (2) infected with Plasmodium or (3) infected with Haemoproteus (Parahaemoproteus) parasites. As only a small amount of blood was required to determine the haemosporidian infection status, excess blood for each cardinal was stored at −80°C. In the current study, we extracted RNA from blood samples of three birds uninfected with haemosporidians, three birds infected with Plasmodium (two with OZ03OZ01 and one with SIAMEX01) and three birds infected with Haemoproteus (two with OZ45_MEX19 and one with TUTI233) for a total of nine samples. RNA was extracted using a chloroform/ethanol protocol followed by a DNase digestion, and then cleaned using a Qiagen RNeasy Mini kit (Qiagen.com). cDNA libraries were prepared using a NEBNext® Ultra™ II RNA Library Prep Kit for Illumina and run on a HiSeq (Illumina.com). Salmon (v0.9.1, Patro et al., Reference Patro, Duggal, Love, Irizarry and Kingsford2017) generated transcript expression estimates for each library by mapping the raw Illumina reads to the zebra finch (Taeniopygia guttata) transcriptome (v3.2.4, Warren et al., Reference Warren, Clayton, Ellegren, Arnold, Hillier, Künstner, Searle, White, Vilella, Fairley, Heger, Kong, Ponting, Jarvis, Mello, Minx, Lovell, Velho, Ferris, Balakrishnan, Sinha, Blatti, London, Li, Lin, George, Sweedler, Southey, Gunaratne, Watson, Nam, Backström, Smeds, Nabholz, Itoh, Whitney, Pfenning, Howard, Völker, Skinner, Griffin, Ye, McLaren, Flicek, Quesada, Velasco, Lopez-Otin, Puente, Olender, Lancet, Smit, Hubley, Konkel, Walker, Batzer, Gu, Pollock, Chen, Cheng, Eichler, Stapley, Slate, Ekblom, Birkhead, Burke, Burt, Scharff, Adam, Richard, Sultan, Soldatov, Lehrach, Edwards, Yang, Li, Graves, Fulton, Nelson, Chinwalla, Hou, Mardis and Wilson2010). We recovered ~11 200 genes from each ‘treatment’. Gene expression estimates were produced by combining the transcript expression estimates from the same gene for each sample (Sonenson et al., Reference Soneson, Love and Robinson2015). Genes expressed in fewer than three of the nine samples were removed from further analysis (Bourgon et al., Reference Bourgon, Gentleman and Huber2010). The gene expression estimates were normalized based on sequencing depth of each library. EdgeR (v3.12.1; Robinson et al., Reference Robinson, McCarthy and Smyth2009) was used to find differentially expressed genes. Generalized linear models were used to test for differential expression (DE) based on contrasting each treatment against each other, i.e. Plasmodium-infected vs uninfected, Haemoproteus-infected vs uninfected and Plasmodium-infected vs Haemoproteus-infected. Genes with an FDR adjusted P value ≤ 0.05 were considered differentially expressed. DE results are freely available in the Dryad Depository (doi:10.5061/dryad.r5594f0).
Results
Gene evolution
The number of taxa in each aligned immune-associated gene dataset ranged from 18 to 57 with an average number of 39 species. The number of bps included in each dataset ranged from 279 to 2346 with an average of 940 bps. The number of positively selected sites ranged from 0 to 29 (detected using MEME) and the number of negatively selected sites (detected using FEL) ranged from 0 to 398. Across all datasets d N/d S ranged from 0.040 to 0.789. Eight phylogenetic trees generated for specific gene datasets showed statistically significant evidence of positive, diversifying selection and 39 showed no evidence of positive, diversifying selection (detected using aBSREL). These results are listed in Table 1. Two trees with evidence of positive selection – one with high taxon and high bp values and one with low taxon and low bp values, and two trees with no evidence of positive selection – one with high taxon and high bp values and one with low taxon and low bp values are shown in Fig. 1 (from aBSREL analyses). Note that MEME and aBSREL analyses returned similar topologies.
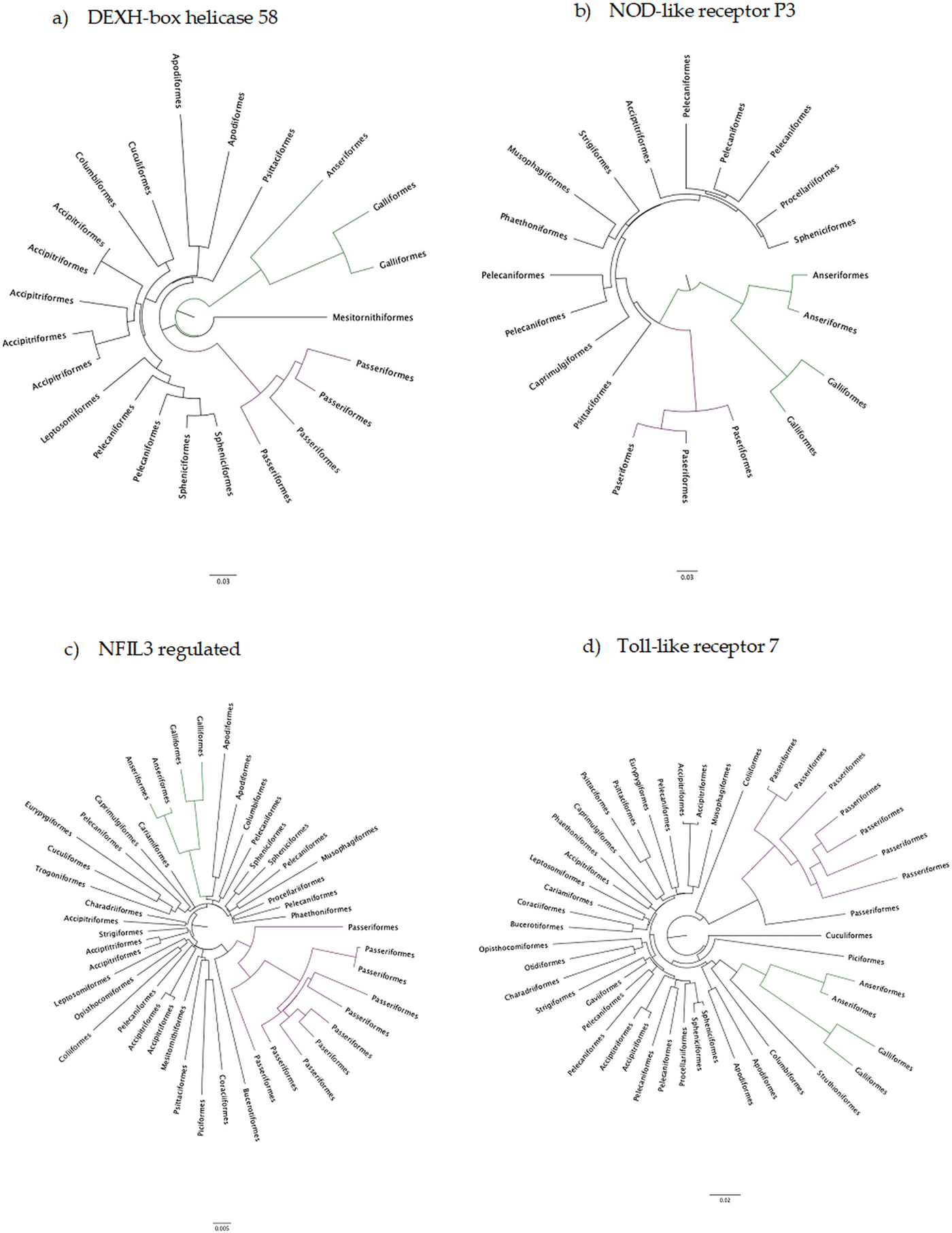
Fig. 1. Gene trees reconstructed with aBSREL. Branch lengths are proportional to the number of codon substitutions. Songbirds (Passeriformes) are the purple clade and game birds (Anseriformes and Galliformes) are the green clade.
Table 1. Summary of results for each gene from MEME (# + sites), FEL (# − sites), SLAC (d N/d S) and aBSREL (diversifying selection) analyses in HyPhy
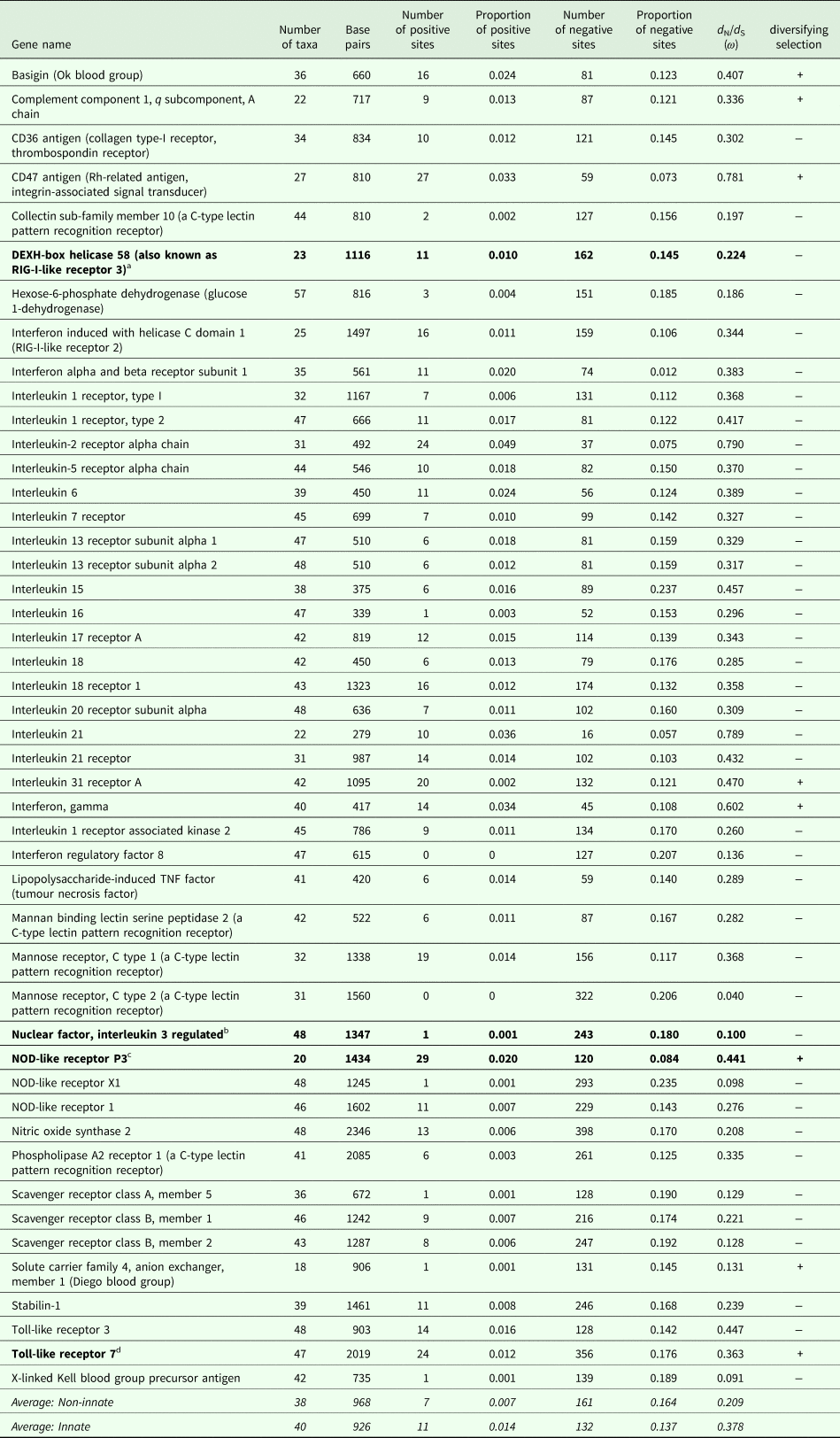
a DEXH-box helicase 58 is involved in nucleic acid binding and helicase activity (https://www.genecards.org/cgi-bin/carddisp.pl?gene=DHX58&keywords=DEXH-box).
b NFIL3 is involved in DNA-binding transcription factor activity and transcription corepressor activity (https://www.genecards.org/cgi-bin/carddisp.pl?gene=NFIL3&keywords=NFIL3).
c NOD-like receptor P3 is involved in peptidoglycan binding (https://www.genecards.org/cgi-bin/carddisp.pl?gene=NLRP3&keywords=NOD-like,P3).
d TLR7 is involved in transmembrane signalling receptor activity and double-stranded RNA binding (https://www.genecards.org/cgi-bin/carddisp.pl?gene=TLR7&keywords=TLR7).
Trees from genes in bold are shown in Fig. 1.
Differential gene expression
All samples in this study were collected from free-living birds, and although our polymerase chain reaction-based tests were repeated several times and have been shown in our lab and in others to be highly reliable, we did not control or specifically account for infection with other pathogens. Relevant values for significantly differentially expressed genes are listed in Table 2. Six genes were uniquely downregulated and four were uniquely upregulated in samples classified as being infected solely with Haemoproteus parasites. Nine genes were uniquely downregulated and seven genes were uniquely upregulated in samples putatively infected solely with Plasmodium parasites. Three genes were upregulated in both Haemoproteus-infected samples and Plasmodium-infected samples. The first of these, SIX Homeobox 4 is found to be involved in eye development in Drosophila and is a transcription factor in mouse development (NCBI gene). The second, immunoglobulin superfamily2C member 11, is involved in cell adhesion (NCBI gene), and the third, tetratricopeptide repeat domain 37, is involved in protein–protein interactions (NCBI gene). The small sample sizes almost certainly bias our results and affect the substantiation of our interpretation, but regardless of these limitations, DE is evident between groups (see also Supplementary Table 1). Surprisingly, none of the genes included in the phylogenetic analyses was found in the DE analyses. Neither did our DE analyses include overlap with the results from Videvall et al. (Reference Videvall, Cornwallis, Palinauskas, Valkiūnas and Hellgren2015), in which the authors examined patterns of DE in response to experimental infection of birds with Plasmodium.
Table 2. Comparative patterns of gene expression between uninfected birds and those infected with Plasmodium or Parahaemoproteus parasites
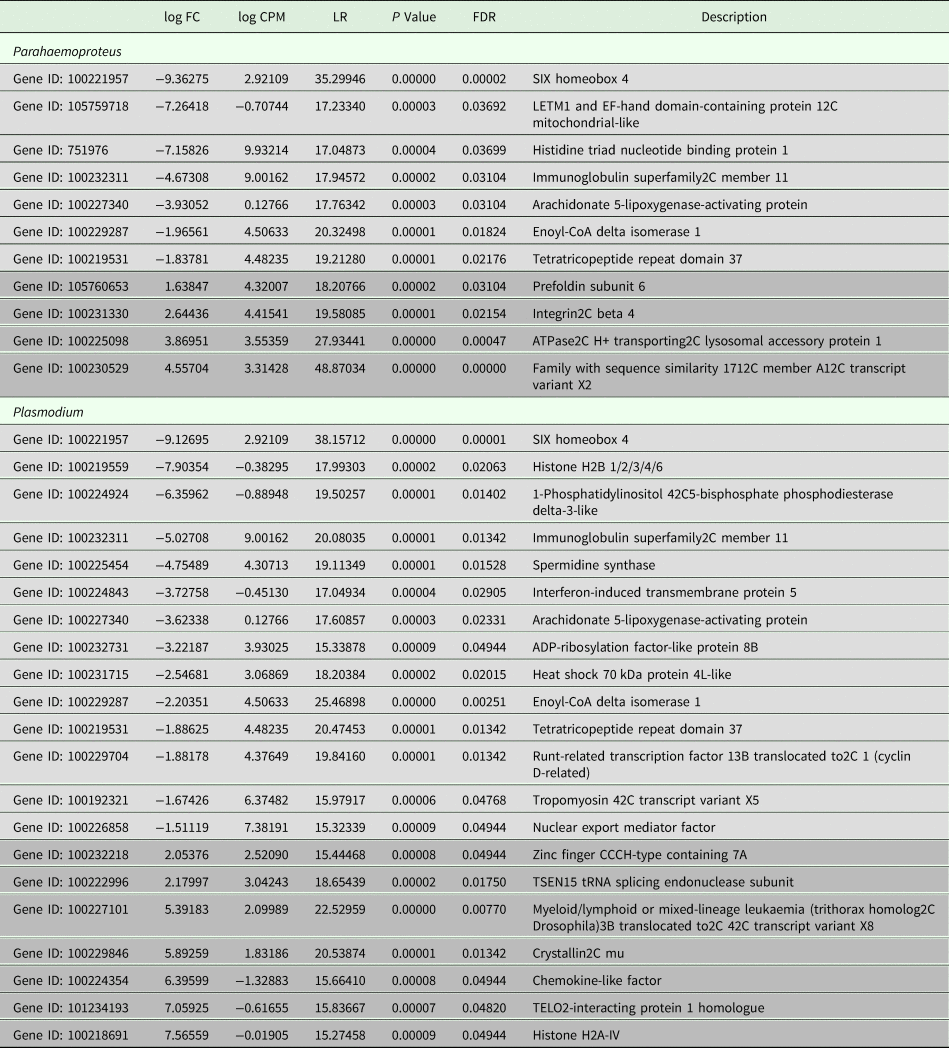
Upregulated genes are in light grey and downregulated genes are in darker grey. See also Supplementary Table 1.
Discussion
Recently, the genomic sequences of 45 species have been made available from across the avian tree of life (Zhang, Reference Zhang, Li, Li, Li, Larkin, Lee, Storz, Antunes, Greenwold, Meredith, Ödeen, Cui, Zhou, Xu, Pan, Wang, Jin, Zhang, Hu, Yang, Hu, Xiao, Yang, Liu, Xie, Yu, Lian, Wen, Zhang, Li, Zeng, Xiong, Liu, Zhou, Huang, An, Wang, Zheng, Xiong, Wang, Wang, Wang, Fan, da Fonseca, Alfaro-Núñez, Schubert, Orlando, Mourier, Howard, Ganapathy, Pfenning, Whitney, Rivas, Hara, Smith, Farré, Narayan, Slavov, Romanov, Borges, Machado, Khan, Springer, Gatesy, Hoffmann, Opazo, Håstad, Sawyer, Kim, Kim, Kim, Cho, Li, Huang, Bruford, Zhan, Dixon, Bertelsen, Derryberry, Warren, Wilson, Li, Ray, Green, O'Brien, Griffin, Johnson, Haussler, Ryder, Willerslev, Graves, Alström, Fjeldså, Mindell, Edwards, Braun, Rahbek, Burt, Houde, Zhang, Yang, Wang, Avian Genome, Jarvis, Gilbert and Wang2014). These data should provide a more complete picture of immune gene evolution in birds, including both TLRs and other non-MHC-related immune genes. To date, the only examination of molecular evolution in avian immune genes has been researched by making pairwise comparisons of orthologous genes between bird species (see Ekblom and Galindo, Reference Ekblom and Galindo2010; Ekblom et al., Reference Ekblom, French, Slate and Burke2011, but see Minias et al., Reference Minias, Pikus, Whittingham and Dunn2018). Here we evaluated signatures of natural selection in avian PRR gene families using a phylogenetic framework.
Like humans, birds are highly susceptible to malaria (haemosporidian) parasite infection and are confronted with no fewer than four genera of malaria parasites (Valkiunas, Reference Valkiunas2004; see also Bichet et al., Reference Bichet, Moodley, Penn, Sorci and Garnier2015). Because malaria has had such a profound effect on the human genome (see Kwiatkowski, Reference Kwiatkowski2005) it would seem necessary that we look for the effects of molecular evolution in avian genomes. However, given that it is relatively unknown which genes are turned on or off in response to infection with malaria parasites, studies – like this one – must first compare birds with different types of parasite infections. Many genetic mechanisms of malaria resistance in humans were shaped by evolution in such a way that they have even become harmful to the constituent populations. The most well-known example is heterozygote advantage in the HBB (haemoglobin beta) gene, which confers such resistance to human Plasmodium falciparum malaria that the deleterious allele remains at 10% frequency or higher even though the homozygous recessive is lethal (Kwiatkowski, Reference Kwiatkowski2005). Such costly mechanisms have arisen independently many times and in response to different species of human Plasmodium (summarized in Kwiatkowski, Reference Kwiatkowski2005).
General evolutionary trends
Innate immune genes of birds appear to evolve in a highly conserved manner under strong purifying selection (see Table 1) which is entirely consistent with their evolutionary role. In the eight phylogenetic trees with at least one branch under positive selection, selection occurred mostly on terminal branches and there was no identifiable pattern with which taxa were under selection.
Haemosporidian malaria parasites elicited different transcriptomic responses from our wild-caught Northern cardinal samples. None of the significantly differentially expressed genes overlap with the immune genes in the first part of the study. Of note, a few of the differentially expressed genes in the birds are known to be involved in immune responses and signal transduction. With further sampling of other bird species, differentially expressed genes may be candidates for further studies of avian–haemosporidian interactions. However, considerable caution is warranted as the birds were caught in the wild and not tested for infection with other parasites, and the sample size is very small. Thus, the observed DE may reflect the effects of many other environmental factors.
Adaptive strategy
The adaptive strategy of avian innate immune genes is their conservation: a d N/d S less than 1. Overwhelmingly, genes of the innate immune system have been under intense selection to stay the same, although it is likewise clear than many sites are under positive selection even against the background of constraint. Therefore, why then, have other studies found immune genes to be rapidly evolving? The most comprehensive study was conducted between a galliform (chicken) and a passerine (zebra finch), two branches of the avian tree of life separated by ~40% genomic sequence divergence (Ekblom et al., Reference Ekblom, French, Slate and Burke2011). In a follow-up study, Ekblom et al. (Reference Ekblom, French, Slate and Burke2011) summarized across gene ontology groups within the zebra finch genome and found d N/d S values about half of what we see here. On the other hand, analyses of selection in the MHC genes show rampant evidence of positive selection, a d N/d S greater than 1 within passerines (Minias et al., Reference Minias, Pikus, Whittingham and Dunn2018), which is much higher than we found. More recently, big data from across the avian tree of life have shown that songbirds radiated in a big bang – even with low overall values of d N/d S (Zhang et al., Reference Zhang, Li, Li, Li, Larkin, Lee, Storz, Antunes, Greenwold, Meredith, Ödeen, Cui, Zhou, Xu, Pan, Wang, Jin, Zhang, Hu, Yang, Hu, Xiao, Yang, Liu, Xie, Yu, Lian, Wen, Zhang, Li, Zeng, Xiong, Liu, Zhou, Huang, An, Wang, Zheng, Xiong, Wang, Wang, Wang, Fan, da Fonseca, Alfaro-Núñez, Schubert, Orlando, Mourier, Howard, Ganapathy, Pfenning, Whitney, Rivas, Hara, Smith, Farré, Narayan, Slavov, Romanov, Borges, Machado, Khan, Springer, Gatesy, Hoffmann, Opazo, Håstad, Sawyer, Kim, Kim, Kim, Cho, Li, Huang, Bruford, Zhan, Dixon, Bertelsen, Derryberry, Warren, Wilson, Li, Ray, Green, O'Brien, Griffin, Johnson, Haussler, Ryder, Willerslev, Graves, Alström, Fjeldså, Mindell, Edwards, Braun, Rahbek, Burt, Houde, Zhang, Yang, Wang, Avian Genome, Jarvis, Gilbert and Wang2014) – as we have seen in our gene trees (Fig. 1a–d). This big bang would at least partially explain the elevated rates that Ekblom et al. (Reference Ekblom, French, Slate and Burke2011) found between these very divergent lineages, except that their overall estimate of selection was closer to neutral, with positive selection showing up at specific residues rather than across whole genes.
Our analyses paint a more complex picture given that we could leverage more data than in previous studies. Immune genes are a blend of both positively and negatively selected sites in a constrained background, and their complex patterns of selection reflect their complex roles. The complexity of evolutionary interactions between hosts and pathogens is becoming increasingly apparent, particularly within the plant literature (e.g. Thrall et al., Reference Thrall, Barrett, Dodds and Burdon2016 and references therein), and mathematical models to understand these interactions increasingly refer to them as ‘tangled’. Detailed analyses of sites under selection and the interpretation thereof are beyond our expertise, but the myriad parasites that differentially affect avian clades suggest that this pursuit would unlock many secrets about the evolution of avian immune genes.
Although studies of parasites in wild birds are extremely patchy in geographic location and host taxonomy, there is ample evidence to suggest that some parasites infect all birds but that different birds are affected by different parasites in many cases. Plasmodium (Atkinson, Reference Atkinson, Atkinson, Thomas and Hunter2008), Toxoplasma (Dubey, Reference Dubey, Atkinson, Thomas and Hunter2008) and capillarid nematodes (Yabsley, Reference Yabsley, Atkinson, Thomas and Hunter2009) are cosmopolitan and seemingly ubiquitous across birds (and other vertebrate taxa). On the other hand, Trichomonas primarily infects doves, raptors and parrots (Forrester and Foster, Reference Forrester, Foster, Atkinson, Thomas and Hunter2008), and the Dispharynx/Echinuria/Streptocara complex and tracheal worms (Fernando and Barta, Reference Fernando, Barta, Atkinson, Thomas and Hunter2008) are most commonly found in songbirds and galliformes. There are many other examples of host-specificity (at the order and family level) in bird parasites (see Wobeser, Reference Wobeser, Atkinson, Thomas and Hunter2008; see also Kumar et al., Reference Kumar, Vijayakumar, Gandhale, Ranaware, Kumar, Kulkarni, Raut and Mishra2017) and coevolutionary relationships between these hosts and parasites presumably over millions of years have shaped the adaptive responses within and between avian clades. Differences between the innate and adaptive immune systems are profound, and therefore, our expectations of patterns of selection between these groups of genes are likewise very different. Here, we focused on (primarily) genes of the innate immune system, and found an overall signature of purifying selection. However, where there are clear distinctions between groups of birds that are infected by different parasite groups, there is evidence of molecular evolution that may correspond with these differences.
Author ORCIDs
Diana C. Outlaw, 0000-0002-9072-4090
Supplementary material
The supplementary material for this article can be found at https://doi.org/10.1017/pao.2019.3.
Financial support
Funding for the project was provided by the National Institutes of Health R03AI117223-01A1 to DCO and U.S. Department of Agriculture ARS 58-6066-6-046 to DGP.
Conflict of interest
None.
Ethical standards
The authors assert that all procedures contributing to this work comply with the ethical standards of the relevant national and institutional guides on the care and use of laboratory animals. Birds were mist-netted and released under both United States Federal (MB6214) and Mississippi State collecting permits to DCO, and under MSU's Institutional Animal Care and Use Committee (IACUC).