Personal protective equipment (PPE) is used by health-care personnel to protect themselves and others from infectious diseases and other workplace hazards. During the coronavirus disease 2019 (COVID-19) pandemic, an increase in PPE demand was evident as early as March of 2020 when the World Health Organization (WHO) called on manufacturers to increase PPE production by 40%. 1 Shortages of PPE posed a tremendous challenge to the US health-care system. This shortage revealed a significant vulnerability in U.S. healthcare emergency preparedness and prompted the Centers for Disease Control and Prevention (CDC) to issue “Optimizing Supply of PPE and Other Equipment during Storages” to identify a continuum of approaches to conserve supplies of PPE while maximizing protection to healthcare personnel when there is limited supply. 2 Shortages during the COVID-19 pandemic also forced health-care personnel to wear particulate respirators that they were unfamiliar with, placing them at an increased risk of exposure due to having poorer fit. Reference Coffey, Miller and Szalajda3 When hospitals cannot find PPE models that they typically use, they may begin to purchase similar models from the same or other manufacturers. Consequently, decreasing numbers of different PPE models could be used as an indicator of supply chain stabilization. Unlike exact counts of PPE inventory, the number of PPE models may be more easily ascertained and tracked by health systems, health-care coalitions, and state or local health departments.
In the United States, all respirators used in occupational settings are approved by the National Institute for Occupational Safety and Health (NIOSH) and used in accordance with Occupational Safety and Health Administration (OSHA) standards. An N95 filtering facepiece respirator (FFR) is a type of disposable respirator that forms a tight seal to the face and filters out particles from the air. 4 To ensure a proper seal, N95 FFRs must be fit tested before being worn in an occupational setting. Fit testing is a time-intensive effort as users must be fit tested for each new model to determine size and whether the respirator achieves the required fit factor to ensure adequate respiratory protection. Changing respirator models is burdensome as staff are required by the OSHA respiratory protection standard 1910.134 5 to fit test for each different model.
Although the need to fit test each new or different model may seem excessive, the sizes of respirators are not the same across models or brands. 6 Therefore, anytime there is a change to a respirator type, brand, model, or size, a new fit test must occur to ensure adequate protection. 7 Furthermore, a mixed-methods demonstration study that involved 43 health delivery settings that deployed reusable elastomeric half mask respirators (EHMRs) during the COVID-19 pandemic Reference Haas, Greenawald and Furek8 revealed several challenges and consequences related to an unstable supply of N95 FFRs. Specifically, participating organizations discussed the burden of constant fit testing for new or different N95 FFRs. In 1 presentation discussing results of the project, Reference Haas, Greenawald and Villalon9 1 emergency response unit stated: “One of the issues we had with N95 FFRs is the switch up in what models manufacturers were going to give us. So, we may be fit tested for the 1870+ and then suddenly, we get the 8205 and we have to re-fit test everyone and that is horrible. Our respiratory protection program (RPP) specifies that people cannot wear something they are not fit tested for which is great but also can be a challenge with these model change-ups.”
In this same presentation, Reference Haas, Greenawald and Villalon9 another hospital indicated that “Throughout the (COVID-19) pandemic, we fit them (employees) to multiple (FFR) models for different sizes so they could pull from whatever. Especially if they were floating, they needed to grab what was there. But that was a huge labor impact.” Although this information is not generalizable, the health-care organizations in this study did comply with OSHA requirements to fit test any new FFR brand, model, or size with their employees and noted the burden this had during the COVID-19 pandemic. Researchers also learned that, anecdotally at least, common practice and preference among hospitals was to have 1 or 2 different models on hand for employees rather than half a dozen or more. Reference Haas10
OSHA’s fit testing resources indicate that it takes approximately 15-20 min to complete an annual fit test. 11 A more recent study showed that the time to complete qualitative fit testing improved with the number of attempts, with the average time to fit test being 6 min and 29 s. Reference Pompeii, Kraft and Brownsword12 Regardless, time and effort go into each fit test, making supply of FFR inventory important from both a time management and resource perspective. Consequently, the authors explored PPE inventory reporting in the health-care industry to advance a reliable mechanism for completing and deploying a national PPE surveillance and monitoring system. Reference Haas, Casey and Furek13 In partnership with the Center for Medical Interoperability (C4MI) 14 and several US hospitals, PPE supply data were tracked, recorded, and shared with NIOSH for 15 wk in 2020. Data showed that most PPE models were accurately, if not consistently, reported but that a universal PPE data standard would be necessary to correctly count PPE inventories. Reference Haas, Casey and Furek13 In the absence of such a standard, this study sought to explore an intermediate solution to track the number of N95 FFR models, rather than N95 FFR quantities, as a potential indicator of supply chain stability in US health-care systems.
Methods
Researchers leveraged existing PPE inventory data from June 1 to September 10, 2020, that was obtained in partnership with C4MI. 14 Because a standardized nomenclature and data format for collecting PPE inventory data did not exist, a PPE supply management app was used to collect counts of PPE quantities from participating hospitals. Hospitals established daily automated reports that queried their relevant information systems, converted the daily reports to a common data model, and transferred results to the app. Aggregated hospital data were made accessible through data use agreements facilitated by C4MI, allowing for third-party usage. This activity was reviewed by CDC, deemed not research, and was conducted consistent with applicable federal law and CDC policy.Footnote 1
NIOSH’s Certified Equipment List (CEL) was consulted to clean and sort the N95 FFR data by model number and manufacturer. The CEL is a database of NIOSH Approved® respirators organized by model number and manufacturer. 15 For additional PPE inventory details about the original hospitals that participated, see Haas and colleagues. Reference Haas, Casey and Furek13
Sample
This retrospective study used the data received from a health-care system in Illinois, comprised of 11 hospitals, because this system shared N95 FFR inventory data over the entire 15-wk demonstration. One hospital did not report respirator model information for 9 wk and was excluded from the analysis. The daily number of respirator models (defined by manufacturer and model number) was averaged each week to calculate the mean number of respirator models on hand for each of the 15 wk.
Statistical Analysis
All analyses were performed in IBM SPSS Statistics, version 26. 16 A linear mixed effect model was run to examine whether there was a significant difference in the weekly average number of respirator models on hand over the time considered. According to the Get Us PPE Shortage Index, in September 2020, hospitals were benefiting from the recovering PPE supply chain; from June 2020 to September 2020, PPE requests made to the Get Us PPE website from hospitals decreased from 36% to 10%. 17 Our research question assumed that the supply chain would be more stable at the end of the surveillance period rather than at the beginning; therefore, the last week, Week 15, was used as the reference week for analysis. It was expected that the weekly average of models on hand would be more similar within the same hospital than the weekly average across different hospitals.
Results
Descriptive Characteristics
Descriptive statistics include central tendency and spread of the mean number of respirator models on hand, by week, during the 15-week demonstration. The 10 hospitals reported a total of 14 unique respirator models and 5 unique manufacturers during the 15-week study period. The number of unique respirator models for each hospital ranged from 5 to 11 (see Table 1).
Table 1. Number of unique respirator models and manufacturers represented by hospital during 15-week demonstration period

On average, Hospital J reported the highest weekly average of respirator models on hand (mean = 10.76; SD = 0.40) as shown in Table 2. Hospital G saw the largest range in their weekly average number of models, decreasing from an average of 7.29 to 5.00 models during the study period.
Table 2. Summary statistics of mean respirator models on hand, reported by hospital
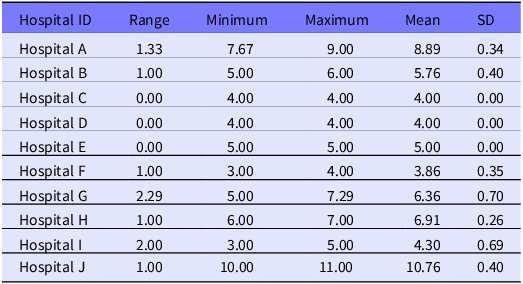
The highest weekly average of mean respirator models on hand across all hospitals (mean = 6.13) were reported in the third week of July (ie, week 7) and the third week of August (ie, wk 12). The lowest average number of respirator models on hand was reported in first and second week of September (ie, weeks 14 and 15) (mean = 5.50; SD = 2.42). Refer to the Supplementary Data section for more detailed results.
Linear Mixed Effect Model
The intraclass correlation coefficient was calculated to see if the hospitals were heterogeneous and to determine if the longitudinal nature of the data violated the assumption of independence. Researchers accounted for the heterogeneity among hospitals and the individual effect of serial correlation by completing a mixed effect model. This approach allowed researchers to investigate how the weekly average of respirator models on hand varied over the study period. The intraclass correlation coefficient was 0.984. Thus, the proportion of the total variance in the average number of models on hand that was accounted for by hospitals was 98.4%.
Results showed that a significant difference across week was indicated (F (14, 140) = 4.71; P < 0.001). There was a small, albeit statistically significant difference in the number of respirator models in wk 2-13 relative to the last week, after accounting for hospital effects. As an example, compared with the last week of the study, the average number of models on hand was 0.56 more in the second week, (P < 0.001; 95% CI [0.27, 0.85]) with a standard error of 0.14 (see Table 3 for more supplementary data results).
Table 3. Parameter estimates for fixed effects

* Note: Significantly different week with reference to the last week of the study.
Discussion
Even though hospitals may try to limit the number of N95 FFR models on hand, during the COVID-19 pandemic, both the shortages of N95 FFRs and temporary emergency use authorizations facilitated additional orders from different respirator manufacturers and the ability to accept donations, to name just a few procurement strategies. Reference Livingston, Desai and Berkwits18,19 Each week, the average number of mean N95 FFR model numbers was greater than for the last week, Week 15. Results identified trends among the hospital system showing that, with respect to the final 2 wk, the weekly number of mean N95 FFR models was greater than that of each week, indicating a significant decline at the end of study period. Even though the effect size is very small, the statistically significant decrease in the weekly average of respirator models on hand over the 15 wk supports an impetus to further explore this metric as a possible indicator to supply chain stability.
Regarding overall health-care system surveillance efforts, researchers have argued that surveillance tools and methods are needed to track respirator inventories to improve accessibility during an emergency. Reference Wizner, Radonovich and Bell20 In 1 case example, Vanderbilt University developed a respiratory protective device surveillance tool that proved useful for benchmarking respirator supplies across 4 participating hospitals. Reference Yarbrough, Ficken and Lehmann21 This tool showed commonalities among respirator brands and models as well as key differences in the respirator quantities available on-site and strategies for stockpiling. In other words, it is not uncommon for hospitals to already monitor this type of information but the way in which they primarily use these data has not effectively or proactively identified shortages. The results of the current study show that including and tracking the number of different model types across health-care systems can serve as a leading indicator to benchmark averages across different-sized hospitals to alert of potential shortages, share resources, and inform decision-making in real time.
While many states have memorandums of understanding that specify resource sharing procedures among health-care entities during public health emergencies, empirical triggers to activate such resource sharing remain challenging. Unlike exact respirator inventory counts, the number of models may be more easily ascertained and tracked by health systems, health-care coalitions, and state, local, or territorial health departments to share resources—whether in the form of respirators, fit testing equipment, or training. Furthermore, a baseline awareness of respirator model types used in local hospitals would allow health departments and health-care coalitions to identify alternative suppliers before they are needed.
Looking to the future and real-world applications of these data, ideas include suggestions for autonomous warehouses equipped with AI-powered robotics to alert organizations when supplies change and even predict future supply shortages. 22 It is possible that these autonomous functions can include respirator model types into its surveillance activities for applicable health-care systems and other industries. Generally, real-time analytics are being discussed as a method to improve decision-making for procurement, assess demands, and improve visibility of the supply chains—much of which is being integrated into current surveillance systems for real-time monitoring. Reference Singh23,Reference Owczarek24 The results of this study provide additional metrics to support such advancements.
Limitations
This study has limitations to consider with its implications. First, the results are not generalizable as they were collected from 1 hospital system in Illinois with no access to the hospital characteristics. While data entry by hospitals occurred by mean of a PPE application developed by C4MI, the participating hospitals still had to manually import their daily inventory into this application, which comes with potential data entry errors and other limitations. Even though the authors underwent a rigorous data cleaning process to ensure accuracy of N95 respirator model numbers and manufacturers, it is still possible that there were some errors based on how the data were entered by hospitals. Finally, the implications of the results have not been empirically studied with health-care entities, so the utility of the results still needs to be explored in future research with public health partners.
Another limitation is that it was never verified with the hospitals whether the supply chain was stable during Week 15 for them and how that week compared with other weeks. Furthermore, any decreases in the average number of model numbers could also be a result of alternative procurement strategies becoming less generative, as opposed to an indicator of the general supply chain stability. For example, the number of models could potentially increase if other procurement sources are used, but then those other sources might run out or stop supplying, and the number of models could decrease.
Conclusions
In the event of a future pandemic, organizations are likely to again receive different N95 FFR models due to supply chain instability. During public health emergencies, state, local, tribal, and territorial (SLTT) health departments and emergency response agencies serve as a vital resource for health-care and first responder organizations. During the COVID-19 pandemic, the Federal Emergency Management Agency (FEMA) and the Administration for Strategic Preparedness and Response (ASPR) sent stockpiled PPE to SLTT governments to help address PPE needs 25 While these local stockpiles of PPE can serve as important resources for health-care and first responder organizations, knowing when and how to deploy these local stockpiles is critical. Using the number of respirator models as an early warning of supply chain disruption warrants further explanation as a relatively simple metric for SLTT governments to track and assess respirator needs during a public health emergency. Additional research is also needed to determine if this association holds true for other PPE types. Consequently, a system to capture this data quickly and efficiently to provide to those who can act on it is important moving forward.
Because previous research has shown inconsistency in model number reporting, Reference Haas, Casey and Furek13 it is suggested that health-care systems or entities use NIOSH’s CEL, 15 which is routinely updated, to serve as a more user-friendly tool to help respirator users, managers, and industrial hygienists determine and select NIOSH-approved respirators to protect against workplace hazards. The CEL allows for quick searches by approval number, manufacturer, or brand, and can be used to help hospitals routinely cross-check their respirator model numbers on hand.
Supplementary material
To view supplementary material for this article, please visit https://doi.org/10.1017/dmp.2024.9
Author contributions
Alexa Furek: Conceptualization, Data analysis, Interpretation of data, Writing – original draft and editing. Mihili Edirisooriya: Data analysis, Interpretation of data, Writing – review and editing. Megan Casey: Conceptualization, Acquisition of data, Interpretation of data, Writing – review and editing. Emily J. Haas: Conceptualization, Data analysis, Acquisition of data, Interpretation of data, Writing – review and editing.
Competing interests
None.
Disclaimer
The findings and conclusions in this report are those of the authors and do not necessarily represent the official position of the National Institute for Occupational Safety and Health, Centers for Disease Control and Prevention. Mention of any company or product does not constitute endorsement by the National Institute for Occupational Safety and Health, Centers for Disease Control and Prevention.
Attribution Statement
N95 and NIOSH Approved are certification marks of the US Department of Health and Human Services (HHS) registered in the United States and several international jurisdictions.