Introduction
Weeds are adaptable to all environments, and in the absence of any control methods, severe infestations might result in complete yield losses for important crops (Chauhan Reference Chauhan2020). Globally, weeds have been demonstrated as the most important pest group in wheat (Triticum aestivum L.), rice (Oryza sativa L.), maize (Zea mays L.), and soybean [Glycine max (L.) Merr.] production systems (Oerke Reference Oerke2006). Globally, herbicide application is preferred over other weed management options in most agricultural regions to achieve optimum weed control and higher yields with the lowest economic cost. Moreover, herbicide use is associated with reduced levels of soil erosion and greenhouse gas emissions and increased levels of water conservation (Gianessi Reference Gianessi2013). However, overreliance on herbicides is often associated with poor performance in the field and increased herbicide resistance (Peterson et al. Reference Peterson, Collavo, Ovejero, Shivrain and Walsh2018).
Optimizing current herbicides’ efficacy is now a priority, given the absence of new herbicide mechanisms of action along with the phaseout of several active ingredients (Rueegg et al. Reference Rueegg, Quadranti and Zoschke2007). To deal with this situation, research has been carried out to develop methods to evaluate herbicide efficacy against targeted weed species as quickly as possible. For instance, the rapid detection of herbicide resistance has been the major objective of several studies (Boutsalis Reference Boutsalis2001; Kaiser et al. Reference Kaiser, Menegat and Gerhards2013; Kaundun et al. Reference Kaundun, Hutchings, Dale, Bailly and Glanfield2011; Wang et al. Reference Wang, Zhang, Dong and Lou2016; Zhang et al. Reference Zhang, Lim, Kim, Nah, Fischer and Kim2016). Although such techniques have provided important results, most focused only on the detection of herbicide resistance in a small number of weed species without evaluating herbicide efficacy at the field level. In any case, the role of sensors and precision agriculture is crucial in developing more effective weed management strategies (Gée and Denimal Reference Gée and Denimal2020).
The Normalized Difference Vegetation Index (NDVI) is the most widely used vegetation index in agricultural settings (Xue and Su Reference Xue and Su2017). NDVI is estimated as the normalized differences between near-infrared (NIR) and red reflectance [NDVI = (RNIR – Rred)/(RNIR + Rred)], where RNIR and Rred are the reflectance values measured in the NIR (770 nm) and red regions (660 nm), respectively (Tremblay et al. Reference Tremblay, Wang, Ma, Belec and Vigneault2009). NDVI is a nondestructive tool for estimating various parameters regarding vegetation growth and health status. For instance, several studies have highlighted the importance of using NDVI values to make estimations regarding crop phenology, canopy development, vegetation biomass, and final yield performance in major crops (Berger et al. Reference Berger, Ettlin, Quincke and Rodríguez-Bocca2019; Ravier et al. Reference Ravier, Meynard, Cohan, Gate and Jeuffroy2017; Vannoppen et al. Reference Vannoppen, Gobin, Kotova, Top, De Cruz, Vīksna, Aniskevich, Bobylev, Buntemeyer, Caluwaerts, De Troch, Gnatiuk, Hamdi, Reca Remedio, Sakalli, Van De Vyver, Van Schaeybroeck and Termonia2020; Wang et al. Reference Wang, Christensen, Svensgaard, Jensen and Liu2020; Yang et al. Reference Yang, He, Shen, Zhou, Zhu, Gao, Chen and Li2020). Regarding its practical implementation in the field of weed management, NDVI is mainly used for weed detection and mapping in agricultural fields. For example, NDVI has been used for the detection of late-emerging weed patches in soybean and wheat (Koger et al. Reference Koger, Shaw, Watson and Reddy2003; López-Granados et al. Reference López-Granados, Jurado-Expósito, Peña-Barragán and García-Torres2006). Savin et al. (Reference Savin, Dokukin, Verniuk and Zhogolev2017) noted that weeds’ contribution to the NDVI values obtained in spring barley (Hordeum vulgare L.) fields is significant, especially if a measurement is taken in the middle of the growing season.
There is evidence that weed responses to stress factors can be estimated via sensor-based techniques (Peteinatos et al. Reference Peteinatos, Korsaeth, Berge and Gerhards2016). Abiotic factors such as drought periods along with insect and disease infestations are important parameters inducing plant stress. In these cases, lower NDVI values are recorded, indicating the role of this index in evaluating vegetation health status (Durgun et al. Reference Durgun, Gobin, Duveiller and Tychon2020; Franke and Menz Reference Franke and Menz2007; Nansen et al. Reference Nansen, Macedo, Swanson and Weaver2009; Naser et al. Reference Naser, Khosla, Longchamps and Dahal2020; Vannoppen et al. Reference Vannoppen, Gobin, Kotova, Top, De Cruz, Vīksna, Aniskevich, Bobylev, Buntemeyer, Caluwaerts, De Troch, Gnatiuk, Hamdi, Reca Remedio, Sakalli, Van De Vyver, Van Schaeybroeck and Termonia2020). Herbicide application is also a factor inducing stress in treated plants. Carotenoid inhibition, leaf bleaching, and lower chlorophyll content in the meristematic tissues are the typical outcomes of herbicide application affecting plant health (Peñuelas and Filella Reference Peñuelas and Filella1998). Although NDVI is not targeted to detect herbicide injury symptoms exclusively, it has been reported to detect changes in vegetation growth caused by herbicide application (Streibig et al. Reference Streibig, Rasmussen, Andújar, Andreasen, Berge, Chachalis, Dittmann, Gerhards, Giselsson, Hamouz, Jaeger-Hansen, Jensen, Jørgensen, Keller and Laursen2014). Henry et al. (Reference Henry, Shaw, Reddy, Bruce and Tamhankar2004) stated that the process of red light’s absorption followed by energy reflectance in the NIR region is an attribute of healthy vegetation. Given the way NDVI is computed (Tremblay et al. Reference Tremblay, Wang, Ma, Belec and Vigneault2009), a decrease in the health status of vegetation can be detected from reduced NDVI values. In a recent study, Lewis et al. (Reference Lewis, Jeffries, Gannon, Richardson and Yelverton2014) used NDVI as part of their herbicide evaluation processes. The use of NDVI has been suggested for the evaluation of wheat’s tolerance to selective herbicides and the detection of herbicide injury symptoms in maize (Zea mays L.) and soybean (Henry et al. Reference Henry, Shaw, Reddy, Bruce and Tamhankar2004; Kong et al. Reference Kong, Si, Feng, Li, Wang and Sayre2009; Thelen et al. Reference Thelen, Kravchenko and Lee2004). It must be noted that the majority of these studies focused on cultivated species and not on weeds treated with herbicides. Given the limited evidence available, research is required to investigate whether NDVI is a reliable index for providing information regarding the efficacy of herbicide application on weeds. If this vegetation index is proven to be a reliable tool for this purpose, it can serve as the basis for developing novel strategies to evaluate herbicide efficacy shortly after treatment. However, any novel sensor-based technique in agriculture should be compared with the classic destructive methods for calibration purposes (Xue and Su Reference Xue and Su2017).
The main objective of the current study was to implement a method for the in situ evaluation of herbicide efficacy in several durum wheat (Triticum durum Desf.) fields in Greece. The herbicide efficacy evaluation was based on the NDVI values recorded at 1 and 2 wk after treatment (WAT). Destructive measurements were carried out to validate the reliability of the method, and the effect of herbicide application on wheat yield performance was also evaluated. Another research target was to validate in pot experiments whether the high NDVI values were linked with the presence of herbicide-resistant weeds.
Materials and Methods
Description of the Experimental Fields
Two-year field trials were conducted in the 2018 to 2019 and 2019 to 2020 growing seasons in several durum wheat fields in Greece. Fields in Aliartos (Aliartos 1, Aliartos 2), Thiva, Domokos, Larissa, and Orestiada were selected for field experiments based on local farmers’ concerns regarding increased weed infestation levels and low efficacies of herbicide applications.
For all the studied sites and fields, experiments were set up during the initial growth stages of durum wheat. In each field, sowing had already been carried out by the local farmers approximately 2 mo before trial establishment. After communicating with the landowner of each field, we noted that the soil of each field had been plowed to approximately 25-cm depth for seedbed preparation before durum wheat was sown in both the experimental years. Durum wheat sowing was carried out during November, for both the growing seasons, in Aliartos, Thiva, and Domokos. Crop sowing was conducted later, during December, for both growing seasons in the fields of Larissa and Orestiada (Table 1). In all fields, wheat was sown in rows with 20-cm row spacing and 5-cm spacing within the rows. In 2018 to 2019 and before experimental setup, our research group noticed a wheat density of around 300 plants m−2 in each field. The same wheat density was observed during the second growing season. After wheat was sown in 2018, we walked through each field on the two diagonals to identify the dominant weed species present. In each field, a position with representative weed flora was selected for field experimentation in 2018 and GPS coordinates were noted (Gerhards et al. Reference Gerhards, Wyse-Pester and Johnson1997); these same locations were used for the second series of experimental runs carried out during 2019 to 2020 (Table 1). Before experimental setup, soil samples were taken from each field to perform soil analyses for both experimental years (Table 2). Typical climatic conditions for Greece prevailed during both the growing seasons in the experimental fields of the regions surveyed (Table 3).
Table 1. Geographic position of each experimental field and wheat sowing dates for both growing seasons (2018–2019 and 2019–2020).

Table 2. Soil conditions of each experimental field. a

a Data were averaged over both the growing seasons (2018–2019 and 2019–2020).
Table 3. Mean monthly temperature and total monthly precipitation for the experimental fields during both the growing seasons (2018–2019 and 2019–2020).

Experimental Setup
For every field trial, the area selected for experimentation was separated into blocks (replications), and each block was separated into seven plots. All the separate field trials were conducted as a randomized complete block design with seven different experimental treatments in four blocks. Each plot was 2.5-m wide and 4-m long. Block size was 70 m2, and as a result, the whole experimental area in each studied field was 280 m2.
At experimental setup, durum wheat plants were at the middle of their tillering stage. In most cases, weeds were between the 4- and 6-leaf growth stages. The weeds and the durum wheat plants were treated with five POST herbicides, resulting in a total of six different herbicide treatments, because 2,4-D was applied at two rates. A weedy check (control) treatment was also included. The POST herbicides were 2,4-D, a prepackaged mixture of pyroxsulam + florasulam, a prepackaged mixture of florasulam + clopyralid, a prepackaged mixture of mesosulfuron-methyl-methyl + iodosulfuron-methyl-sodium-methyl, and fenoxaprop-p-ethyl. The application rates for the herbicides used and the abbreviations for the seven experimental treatments applied during the current study are presented in Table 4. Herbicides were applied with a pressurized backpack Gloria® 405 T sprayer (Gloria Haus- & Gartengeraete GMBH, Daermannsbusch 7, 58456 Witten, Germany) equipped with five flat spray nozzles (spray angle: 80°) delivering 300 L ha−1 of spray solution at 300 kPa. An exception was made for 2,4-D, which was applied with 200 kPa pressure according to the recommendations provided on the product label.
Table 4. Descriptions of the experimental treatments.
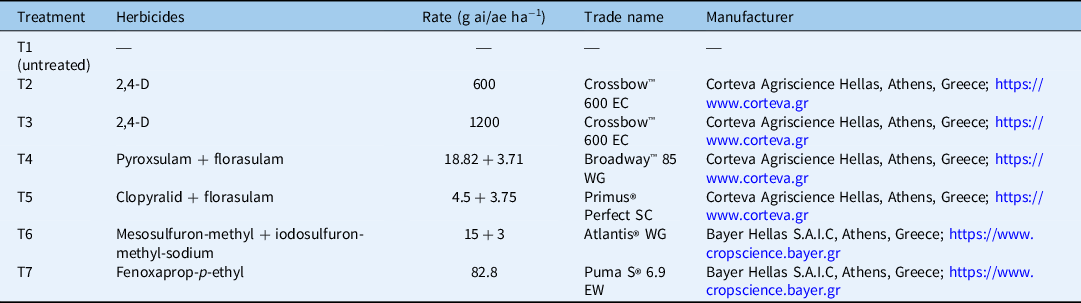
Data Collection
In each field, four metallic 0.25-m2 quadrats were placed permanently around the middle of each plot in areas with uniform weed flora and away from the plot margins. Each quadrat contained two rows of durum wheat along with weeds. Herbicides were applied after the major weed species present in the quadrats were identified visually (data not shown). NDVI values were used as an estimate of herbicide efficacy, given the evidence derived from previous studies (Lewis et al. Reference Lewis, Jeffries, Gannon, Richardson and Yelverton2014). To make the evaluations possible, auxinic herbicides (2,4-D, clopyralid), acetolactate synthase (ALS) inhibitors (pyroxsulam, florasulam, mesosulfuron-methyl-methyl, iodosulfuron-methyl-sodium-methyl), and an acetyl-CoA carboxylase (ACCase) inhibitor (fenoxaprop-p-ethyl) were selected for this study. These herbicides cause chlorotic symptoms in the tissues of the treated vegetation, and the symptoms are usually obvious a short period after treatment. There is evidence indicating that broadleaf weeds enter a decay phase approximately 72 h after treatment with auxinic herbicides, with the weeds displaying chlorosis, necrosis, senescence, and plant death (Grossman Reference Grossmann2010). Although photosynthesis is not regarded to be a primary target of ALS-inhibiting herbicides, changes in chlorophyll fluorescence responses are observed in the treated plants (Riethmuller-Haage et al. Reference Riethmuller-Haage, Bastiaans, Kropff, Harbinson and Kempenaar2006). Injury symptoms due to application of fenoxaprop-p-ethyl, a lipid synthesis inhibitor belonging to the aryloxyphenoxypropionate family, are expressed in the leaf tissues of weeds between 7 to 14 d after treatment (Gunsolus and Curran Reference Gunsolus and Curran1991).
At 1 WAT, a very short time for field test results, we returned to each field for the first NDVI measurements in the areas marked by the quadrats. At approximately 2 WAT, NDVI values were measured for a second time. Hereafter, the timing of the first NDVI measurements is referred to as “1 WAT,” and the timing of the second NDVI measurements is referred to as “2 WAT.” We tried and almost managed to adhere to the timings of measuring NDVI at 1 WAT and 2 WAT; but this was dependent on weather conditions and predicted forecasts. The measurements were performed using a Trimble® GreenSeeker® handheld crop sensor (Trimble Agriculture Division, Westminster, CO, USA). The sensor unit has self-contained illumination in both red and NIR bands and measures reflectance in the red and NIR regions of the electromagnetic spectrum (Tremblay et al. Reference Tremblay, Wang, Ma, Belec and Vigneault2009) according to Equation 1:

The direction of the device was parallel to the direction of the crop rows. The sensor was held stable at approximately 20 to 25 cm above the weed leaf area for 5 s, in the area delimited from the rows of the crop, and focused only on the weeds. The 5-s time period was based on the recommendations of Kong et al. (Reference Kong, Si, Feng, Li, Wang and Sayre2009). To measure the NDVI values of almost all the weeds present in the area between the crop rows, this action was repeated twice in each quadrat. The recorded values from each quadrat were then averaged. The same course of action was carried out for the other three quadrats. The NDVI values recorded from the four quadrats were averaged to obtain a final NDVI value for each plot. We noticed that almost 2 h are required to accomplish a full measurement in a single field. To validate the method and ascertain whether the low NDVI values corresponded to the most efficient herbicide applications, destructive measurements were conducted before durum wheat harvest. In particular, weed samples were selected from the quadrats of each plot at the crop’s maturity stage (Alba et al. Reference Alba, Syrovy, Duddu and Shirtliffe2020). The aboveground biomass was harvested by clipping the vegetation at 3- to 5-cm height as in previous studies (Kanatas et al. Reference Kanatas, Travlos, Gazoulis, Antonopoulos, Tsekoura, Tataridas and Zannopoulos2020) and was stored carefully in large numbered plastic bags. Afterward, wheat spikes were harvested by hand from each quadrat and were also stored carefully in separate plastic bags. The weed samples and wheat spikes were transferred to the Laboratory of Agronomy of Agricultural University of Athens. The seeds from the harvested weeds were sampled and stored at room temperature for future pot experiments (Travlos et al. Reference Travlos, Kanatas, Tsekoura, Gazoulis, Papastylianou, Kakabouki and Antonopoulos2020); the harvested weed vegetation was then oven-dried for 48 h at 60 C, and total weed aboveground biomass per unit area was measured. In addition, to estimate wheat grain yield, the number of spikes per unit area (no. m−2), the number of grains per spike, and the weight of 1,000 grains (g) were measured for each plot (Kanatas et al. Reference Kanatas, Travlos, Gazoulis, Antonopoulos, Tsekoura, Tataridas and Zannopoulos2020). The same series of actions, from the experimental setup until the wheat harvest, were conducted in exactly the same way for both experimental periods. Details regarding the dates of all the experimental activities carried out during both years are presented in Table 5.
Table 5. Dates of herbicide applications, Normalized Difference Vegetation Index (NDVI) measurements, wheat harvest, and weed biomass harvest during both growing seasons (2018–2019 and 2019–2020).

a The wind speed values (km h−1) were recorded at the time of spraying, and they are presented in parentheses.
b WAT, weeks after treatment.
c Wheat harvest and weed biomass harvest were performed on the same dates.
Pot Experiments
After screening the NDVI along with the weed biomass values recorded, the weed accessions that were suspected of being resistant to specific herbicides were recognized and tested for their resistance status in pot experiments. The current study presents the results of whole-plant bioassays evaluating the response of two accessions of catchweed bedstraw (Galium aparine L.), one of corn poppy (Papaver rhoeas L.), and one of sterile oat [Avena sterilis L. ssp. ludoviciana (Durieu) Gillett & Mange] to herbicides that were applied in our field trials.
The seeds for two accessions of G. aparine presented here were derived from the fields in Aliartos. Accession GA (ART-1) was collected from the Aliartos 1 field; accession GA (ART-2) was collected from the Aliartos 2 field. After their initial storage, the collected seeds were buried in the soil at approximately 3- to 4-cm depth in large rectangular pots (28 by 30 by 70 cm3) and kept outdoors under natural conditions for 3 mo to break their dormancy, as suggested in the recent study by Wang et al. (Reference Wang, Zhang, Dong and Lou2016). Afterward, seeds were removed from the soil, air-dried, placed in paper bags, and stored temporarily at room temperature until use. The P. rhoeas accession PR (THV) was collected from Thiva. The pretreatment of these P. rhoeas seeds was carried out with 0.5 g L−1 KNO3 for 24 h as suggested by Golmohammadzadeh et al. (Reference Golmohammadzadeh, Zaefarian and Rezvani2020). To release dormancy in the seeds of the A. sterilis ssp. ludoviciana oat accession AS (ORD), collected from Orestiada, the seeds were individually nicked with two dental tweezers and placed in petri dishes on two sheets of Whatman No.1 paper filter disk (Whatman, Maidstone, UK) saturated with 6 ml of distilled water. The petri dishes were kept at 2 to 4 C in a refrigerator for a period of 7 d, as in previous studies (Travlos Reference Travlos2013). After all the appropriate pretreatment processes, the nondormant weed seeds could be used for sowing. Twenty seeds of G. aparine, 100 seeds of P. rhoeas, and 10 seeds of A. sterilis ssp. ludoviciana were sown in separate plastic pots (12 by 13 by 15 cm3) and placed outdoors (under natural sunlight conditions with air temperatures ranging from 11 to 24 C). The seeds were sown at 1-cm depth to achieve maximum seedling emergence. Pots had been filled with a mix of herbicide-free soil from the experimental field of the Agricultural University of Athens and peat at a ratio of 1:1 (v/v). The soil of the experimental field is clay loam with a pH value of 7.29, with CaCO3 and organic matter content of 15.99% and 2.37%, respectively. The pots were uniformly watered as needed and rerandomized each week to achieve uniform growth conditions for all plants. After the weeds passed the cotyledon stage, they were thinned to five individuals per pot.
The G. aparine accessions, GA (ART-1) and GA (ART-2), were evaluated regarding their response to the mixture of mesosulfuron-methyl + iodosulfuron-methyl-sodium (Atlantis® WG, Bayer Hellas S.A.I.C, Athens, Greece) and also to the mixture of pyroxsulam + florasulam (Broadway™ 85 WG, Corteva Agriscience Hellas, Athens, Greece). The response of the P. rhoeas accession PR (THV) to mesosulfuron-methyl + iodosulfuron-methyl-sodium was also studied, as was the response of the A. sterilis ssp. ludoviciana accession AS (ORD) to different fenoxaprop-p-ethyl (Puma S® 6.9 EW, Bayer Hellas S.A.I.C, Athens, Greece) application rates. For each dose–response experiment presented, the herbicides were applied at 0.25X, 0.5X, X, 2X, and 4X the recommended field dose suggested from the label of each commercial product. The recommended field dose for the sulfonylurea prepackage mixture is 500 g ha−1, which is equivalent to 15 g mesosulfuron-methyl + 3 g iodosulfuron ha−1. The recommended field dose for the triazolopyrimidine mixture is 265 g ha−1, which is equivalent to 18.8 g pyroxsulam + 3.7 g florasulam ha−1. The recommended field dose for fenoxaprop is 82.8 g ai ha−1. Two runs of each experiment were conducted, established in a completely randomized design with four replicates (pots). Herbicides were applied with a pressurized backpack Gloria® 405 T sprayer equipped with five flat spray nozzles (spray angle: 80°) delivering 300 L ha−1 of spray solution at 300 kPa pressure. For G. aparine, herbicide applications were performed when the weeds were at the 2- to 3-whorl (a circular pattern of leaves occurring in Galium spp.) growth stage, as suggested by Papapanagiotou et al. (Reference Papapanagiotou, Damalas, Bosmali, Madesis, Menexes and Eleftherohorinos2019a). For P. rhoeas, the herbicides were applied at the 6-leaf growth stage (Rey-Caballero et al. Reference Rey-Caballero, Menéndez, Osuna, Salas and Torra2017); for A. sterilis ssp. ludoviciana, the treatments were applied at the 13 to 15 Zadoks stage (Travlos et al. Reference Travlos, Giannopolitis and Economou2011). An untreated control was kept for each experiment. At 4 WAT, the broadleaf weeds were harvested, and their fresh weight was measured. For A. sterilis ssp. ludoviciana, the fresh weight measurements were carried out at 21 DAT. Accessions of each species susceptible to the corresponding herbicide were also included in each dose–response experiment, treated with the same herbicide doses as the putative resistant accessions.
Statistical Analysis
According to our research protocol, we implemented the same experimental treatments in every durum wheat field studied. However, each trial initiated in each field during 2018 to 2019 was a separate experiment that was repeated during 2019 to 2020. There was no repetition in space, as our method targets providing in situ herbicide efficacy evaluation at the field level and giving specific recommendations to each farmer for maximum weed control. Thus, data from each field were analyzed separately across the two growing seasons.
Normal distribution of all data was checked with a Shapiro-Wilk test (Shapiro and Wilk Reference Shapiro and Wilk1965), while homoscedasticity was tested by performing Levene’s test (Levene Reference Levene1960). Because the variances were homogenous in all cases, arcsine square-root transformations of the data were not required. Such transformations are much more useful in studies in which herbicide efficacy is expressed as a percentage of the untreated control (Ahrens et al. Reference Ahrens, Cox and Budhwar1990). For each separate field trial, the NDVI values recorded from the first measurements and the second measurements as well as the total weed biomass and wheat grain yield values were subjected to ANOVA using Statgraphics Centurion XVI (Statgraphics Technologies, Inc., P.O. Box 134, The Plains, VA 20198, USA). Analyses were performed at the α = 0.05 significance level. The factors of herbicide treatment and year and the interactions between these factors were considered as fixed effects, while the factor of block (replication) was considered as a random effect for analyzing data derived from each trial. For each field trial, the effects of year and year by herbicide treatment interactions on the studied parameters were not significant (P ≥ 0.05). Therefore, data were pooled across the two experimental years. Multiple comparisons were performed to separate means among treatments using Fischer’s LSD test at an α = 0.05 significance level. A linear regression was also performed according to the linear model:

where y represents the values of total aboveground biomass, and x represents the NDVI values recorded at 2 WAT for each field trial. The slope is represented by b. The selection of NDVI values at 2 WAT was made according to the hypothesis that all the treated weeds would have exhibited more obvious chlorotic symptoms in their leaf tissues, compared with 1 WAT, if they were affected by the herbicide application.
ANOVA was used to analyze the data obtained from the pot experiments. Because the ANOVAs indicated no significant treatment by experimental run interactions, means were averaged over the repeated experiments. The weed fresh weight data were expressed as a percentage of the untreated control. The GR50 values were obtained by nonlinear regression using the following log-logistic equation (Seefeldt et al. Reference Seefeldt, Jensen and Fuerst1995):

where y represents dry weight at herbicide dose (x); c and d denote the lower and upper limits, respectively; GR50 is the herbicide dose centered between the asymptotic values; and b is the slope of the response curve. The level of resistance was expressed by means of the resistance index (RI), which was calculated as the ratio of the GR50 of each potentially resistant (R) accession by the GR50 of the corresponding susceptible (S) biotype (R/S).
Results and Discussion
Herbicide Efficacy
The overall effect of herbicide application on the NDVI values recorded both at 1 and 2 WAT in the first field in Aliartos (Aliartos 1) was significant (P ≤ 0.001). The species that occurred at the highest density in this field was G. aparine for both years (data not presented). The lowest NDVI values corresponded to the applications of 2,4-D at either 600 or 1,200 g ae ha−1 (T2, T3) and the mixture of clopyralid + florasulam (T5) as observed at 1 WAT (Table 6). The rapid effects of 2,4-D application on G. aparine have been well documented by Grossmann (Reference Grossmann2010). The results of the second NDVI measurements were similar, and the values recorded for the remaining herbicide treatments (T4, T6, T7) remained high (Table 7). The aboveground weed biomass measurements were in accordance (R2 = 86.94%; Figure 1) with the NDVI values recorded at 2 WAT, indicating that the auxinic-herbicide treatments (T2, T3, T5) provided the highest levels of weed control. Clopyralid + florasulam has been reported as an effective mixture against broadleaves, especially when combined with cultural practices (Kanatas et al. Reference Kanatas, Travlos, Gazoulis, Antonopoulos, Tsekoura, Tataridas and Zannopoulos2020). High NDVI and weed biomass values were observed for the mixture of mesosulfuron-methyl + iodosulfuron-methyl-sodium (T6) (Table 8). The same result was obtained regarding the mixture of pyroxsulam + florasulam (T4). This finding is in line with another study in which the low efficacy of this mixture against broadleaves was noted (Hada et al. Reference Hada, Khammassi, Jenfaoui, Menchari, Torra and Souissi2020). The observations were quite similar for the second field in Aliartos (Aliartos 2), a field where the dominant species was again G. aparine. High NDVI and weed biomass reductions were attributed to the application of clopyralid + florasulam (T5) as well as to the application of 2,4-D at both application rates (T2, T3). The results of this field trial are in line with the findings of Baghestani et al. (Reference Baghestani, Zand, Soufizadeh, Bagherani and Deihimfard2007). The opposite results were obtained for T4 and T6 treatments (Tables 6–8). The regression between NDVI and weed biomass was again noticeable (Figure 1; R2 = 82.34 %). Such results validate that NDVI values at 2 WAT pose a reliable estimate of herbicide efficacy and agree with another study in which lower NDVI values were attributed to aminocyclopyrachlor application as compared with clopyralid (Lewis et al. Reference Lewis, Jeffries, Gannon, Richardson and Yelverton2014). In both these field trials, very few grasses were present in the experimental fields, and they did not contribute significantly to the values for NDVI and weed biomass. In any case, the small populations of grasses observed in some plots did not survive the T4, T6, and T7 treatments (visual observation). However, the low efficacy of the T4 and T6 treatments against the broadleaf weeds created concerns about the presence of herbicide-resistant populations.
Table 6. Normalized Difference Vegetation Index (NDVI) values recorded at 1 wk after treatment. a

a Data were averaged across the two growing seasons (2018–2019 and 2019–2020). The means are separated according to Fischer’s LSD test at α = 0.05 significance level. Different letters in the same row indicate significant differences among treatments.
Table 7. Normalized Difference Vegetation Index (NDVI) values recorded at 2 wk after treatment. a

a Data were averaged across the two growing seasons (2018–2019 and 2019–2020). The means are separated according to Fischer’s LSD test at α = 0.05 significance level. Different letters in the same row indicate significant differences among treatments.

Figure 1. Linear regression between Normalized Difference Vegetation Index (NDVI) (at 2 wk after treatment) and weed biomass (kg ha−1) for the fields of Aliartos (Aliartos 1, Aliartos 2) and Thiva. R2 values are presented for each field trial (vertical and horizontal bars represent the standard errors of the measurements).
Table 8. Total weed aboveground biomass per unit area (kg ha−1). a

a Data were averaged across the two growing seasons (2018–2019 and 2019–2020). The means are separated according to Fischer’s LSD test at a = 0.05 significance level. Different letters in the same row indicate significant differences among treatments.
Four herbicide treatments (T2, T3, T4, T5) were effective in Thiva given the low NDVI values recorded after their application, as noted at 1 and especially 2 WAT (Tables 6 and 7). The NDVI values recorded at 2 WAT were positively correlated with the total weed biomass values measured at the end of the two growing seasons (R2 = 0.8652; Figure 1). These findings align with earlier studies in which this vegetation index was used to detect herbicide injury symptoms on cultivated species by nondestructive means (Kong et al. Reference Kong, Si, Feng, Li, Wang and Sayre2009; Thelen et al. Reference Thelen, Kravchenko and Lee2004). Combined over 2018 to 2019 and 2019 to 2020, the applications of 2,4-D (T2, T3), pyroxsulam + florasulam (T4), and clopyralid + florasulam mixture (T5) resulted in significantly lower aboveground weed biomass as compared with the T1, T6, and T7 treatments (Table 8). In a field where P. rhoeas was present in almost all quadrats for both the growing seasons (data not presented), high NDVI and weed biomass values were recorded for the mesosulfuron-methyl + iodosulfuron-methyl-sodium mixture (T6). To examine the response of this population to the sulfonylurea mixture in pot experiments, P. rhoeas seeds were collected from this field. This species is notorious for developing resistance to herbicides commonly applied in wheat fields. Recent studies reported that populations of P. rhoeas have emerged in the Mediterranean area with increased ability to exhibit resistance to 2,4-D via translocation, and other populations exhibit multiple resistance patterns to 2,4-D and ALS-inhibiting herbicides (Kati et al. Reference Kati, Scarabel, Thiery-Lanfranchi, Kioleoglou, Liberopoulou and Délye2019; Torra et al. Reference Torra, Rojano-Delgado, Rey-Caballero, Royo-Esnal, Salas and De Prado2017). Resistance to 2,4-D is quite an interesting topic, as novel resistance mechanisms have been reported in noxious broadleaf weed species (de Queiroz et al. Reference de Queiroz, Delatorre, Lucio, Rossi, Zobiole and Merotto2020). Thus, the sufficient efficacy of 2,4-D–based treatments and the mixture containing clopyralid (T5) reported in our study is encouraging. In the field trial carried out in Domokos, ivy-leaved speedwell (Veronica hederifolia L.) was the most frequently emerged species for both the years (data not shown). The chlorotic symptoms in the leaf tissues of weeds treated with auxinic herbicides are visible a very short time after application (Gunsolus and Curran Reference Gunsolus and Curran1991). In the present study, the sensor detected those symptoms at 1 WAT, whereas at 2 WAT, lower NDVI values corresponded to T4 and T6 treatments as compared with the control (T1) (Tables 6 and 7). The correlation between NDVI and total weed biomass values was noticeable (R2 = 0.8595; Figure 1). The lower efficacy of the sulfonylurea mixture and the low dose of 2,4-D were at some point expected, given the recommendations on the product labels. However, the V. hederifolia populations were very sensitive to the triazolopyrimidine mixture (T4), clopyralid + florasulam (T5), and the increased dose of 2,4-D (T3). The results from this trial are agreement with Mayerová et al. (Reference Mayerová, Mikulka and Soukup2018), who obtained higher than 90% levels of weed control after 2,4-D applications in wheat fields where Veronica spp. individuals were present at a density of approximately 20 plants m−2.
In Larissa, the dominant species was blessed milk thistle [Silybum marianum (L.) Gaertn.], a native broadleaf weed species of the Mediterranean basin (Tamouridou et al. Reference Tamouridou, Alexandridis, Pantazi, Lagopodi, Kashefi and Moshou2016). The treatments containing auxinic active ingredients (T2, T3, T5) reduced NDVI at 1 and 2 WAT in comparison to the control (T1) and the mixtures based on ALS inhibitors (T4, T6) (Tables 6 and 7). Noticeable R2 values (0.8149) were obtained between NDVI (2 WAT) and weed biomass (Figure 2). The measurements of weed biomass validated that the T4 and T6 treatments did not affect the S. marianum populations. A population of this species has been reported to be reduced by only 8% after treatment with chlorsulfuron at 15 g ai ha−1 (Zand et al. Reference Zand, Baghestani, Soufizadeh, Eskandari, PourAzar, Veysi, Mousavi and Barjasteh2007). In a study by Shimi et al. (Reference Shimi, Poorazar, Jamali and Bagherani-Torshiz2006), these mixtures were effective when applied at the seedling growth stage of S. marianum. However, in the present study, the weeds were in their rosette stage, and it was expected that only the auxinic herbicides could provide adequate levels of control. The reduced weed biomass values in the T5 plots are in line with the suggestions of Khan et al. (Reference Khan, Blackshaw and Marwat2009) regarding the efficacy of clopyralid and clopyralid mixtures at all stages of growth. 2,4-D reduced weed biomass only at the high concentration (T2), as shown in Table 8. Although NDVI was 20% lower in the plots treated with 2,4-D at 600 g ae ha−1 (T2) than in the untreated plots (T1) at 2 WAT (Table 7), the recorded weed biomass values were high at the end of both the growing seasons. A possible explanation might be that the weeds exhibited some regrowth, and many individuals survived the treatment in contrast to the initial chlorosis detected in their leaf tissues. Regrowth is a common phenomenon observed after application of 2,4-D against noxious weeds belonging to the thistle (Asteraceae) family (Lym and Deibert Reference Lym and Deibert2005). Small patches of grass weeds were also present in some plots but were effectively controlled by mesosulfuron-methyl + iodosulfuron-methyl-sodium (T6) and fenoxaprop-p-ethyl (T7). This explains the slight differences observed in NDVI (2 WAT) and weed biomass between these treatments and the control (T1).

Figure 2. Linear regression between Normalized Difference Vegetation Index (NDVI) (at 2 wk after treatment) and weed biomass (kg ha−1) for the fields of Domokos, Larissa, and Orestiada. R2 values are presented for each field trial (vertical and horizontal bars represent the standard errors of the measurements).
The NDVI values recorded at 1 WAT were significantly (P ≤ 0.001) affected by herbicide application in Orestiada. Low values corresponded to the mixtures of pyroxsulam + florasulam (T4) and mesosulfuron-methyl + iodosulfuron-methyl-sodium (T6), whereas higher values were observed for the remaining herbicide treatments (T2, T3, T5, T7) and the control treatment (T1). In this field, the most dominant species was Avena sterilis ssp. ludoviciana (data not presented), the most widespread and harmful weed in cereals in Greece (Travlos et al. Reference Travlos, Giannopolitis and Paspatis2008). Although the differences were not significant, lower NDVI values were observed at 1 WAT for the treatments based on 2,4-D (T2, T3) and the mixture of clopyralid + florasulam (T5), as shown in Table 6. This can be explained by the presence of small patches of broadleaf weeds that were effectively controlled, as shown by the measurements carried out a very short time after application. In the majority of our field trials, the satisfactory performance of 2,4-D against the broadleaves is encouraging, given the cases where noxious species develop resistance via translocation of the active ingredient out of the treated leaf tissues (Goggin et al. Reference Goggin, Cawthray and Powles2016). The same has to be noted for the mixture of clopyralid + florasulam. The use of herbicides with different modes of action in mixtures is inevitable to deal with the aggravated situation regarding the resistance patterns evolved widely to ALS-inhibiting herbicides (Ntoanidou et al. Reference Ntoanidou, Madesis, Diamantidis and Eleftherohorinos2017). Low NDVI values were also observed for the two herbicide mixtures targeting both grasses and broadleaves (T4, T6). The second NDVI measurements validated the high efficacy of these two treatments (Table 7). Lower values corresponded to the triazolopyrimidine mixture (T4); the difference was noticed but was not significant. The high NDVI values for the T2, T3, and T5 treatments did not indicate herbicide inefficacy, as this was a durum wheat field infested mainly with A. sterilis ssp. ludoviciana. The same cannot be noted for the treatment based on the ACCase herbicide fenoxaprop-p-ethyl (T7). The NDVI at 2 WAT was very close to the values recorded for the control, suggesting herbicide inefficacy. The measurements of aboveground biomass validated this trend, being in full accordance with the NDVI values (Figure 2; R2 = 0.8295). The R2 value observed in this field trial was almost 90%, suggesting that NDVI was an accurate index of herbicide efficacy. The low weed biomass values recorded for the sulfonylurea prepackage mixture agree with the findings of Travlos et al. (Reference Travlos, Giannopolitis and Economou2011), who suggested it as an efficient alternative for the control of diclofop-resistant A. sterilis ssp. ludoviciana accessions that were also cross-resistant to fenoxaprop-p-ethyl. However, recent research from a study of wheat fields of Greece detected noticeable levels of resistance in A. sterilis ssp. ludoviciana populations to this herbicide mixture (Papapanagiotou et al. Reference Papapanagiotou, Damalas, Menexes and Eleftherohorinos2019b). In any case, this trend was not observed during the current study, where it was also noticed that the other mixture based on ALS inhibitors (T4; pyroxsulam + florasulam) provided excellent control of the A. sterilis ssp. ludoviciana populations along with the elimination of the small patches of broadleaves.
Wheat Grain Yield
Wheat grain yield was significantly affected by herbicide application in the first field trial in Aliartos (Aliartos 1) as indicated by ANOVA (P ≤ 0.001). Combined across the 2 yr, the applications of clopyralid + florasulam (T5) and 2,4-D at both application rates (T2, T3) resulted in the highest grain yield values. For instance, yield increases obtained for T3 treatment ranged between 26% and 30% as compared with the plots of control (T1) and mesosulfuron-methyl + iodosulfuron-methyl-sodium (T6) (Table 9). Wheat yield has been reported to be favored by the increased weed control levels provided by 2,4-D applications either as a single treatment or in mixtures with MCPA (Baghestani et al. Reference Baghestani, Zand, Soufizadeh, Bagherani and Deihimfard2007; Walsh et al. Reference Walsh, Maguire and Powles2009). In the second field trial carried out in Aliartos (Aliartos 2), it was also noticed that the higher grain yield values corresponded with treatments containing auxinic herbicides (T2, T3, T5), whereas significantly lower yields were obtained in the plots treated with pyroxsulam+florasulam, mesosulfuron-methyl + iodosulfuron-methyl-sodium and fenoxaprop-p-ethyl (T4, T6 and T7, respectively) (Table 9). For example, the application of 2,4-D at 600 g ae ha−1 (T2) increased wheat yield by 25% to 28% in comparison to the two herbicide mixtures that are based on ALS inhibitors (T4, T6). The low values for grain yield obtained after the application of pyroxsulam + florasulam align with the findings of another recent study in which this particular mixture did not positively impact wheat yield (Mahmoud and Safina Reference Mahmoud and Safina2017). In contrast, Zargar et al. (Reference Zargar, Bayat, Romanova and Izadi-Darbandi2020) noticed that tribenuron was the most effective herbicide against G. aparine and its application was beneficial for wheat’s productivity. In Thiva, wheat yield was affected at a significant point from herbicide application (P ≤ 0.001). Averaged over the two growing seasons, the low weed biomass reductions after the application of the sulfonylurea mixture (T6) resulted in approximately 25% lower yield than the values recorded after the T2, T3, T4 and T5 treatments, which were beneficial for wheat yield performance (Table 9). The yield losses attributed mainly to P. rhoeas competition are in accordance with Wilson et al. (Reference Wilson, Wright, Brain, Clements and Stephens1995), who highlighted the negative impact of this weed on wheat’s productivity. The higher yields in the plots treated with clopyralid + florasulam (T5) agree with recent studies in which this mixture was part of integrated weed management strategies against P. rhoeas (Kanatas et al. Reference Kanatas, Travlos, Gazoulis, Antonopoulos, Tsekoura, Tataridas and Zannopoulos2020). Similarly, the T2, T4, and T5 treatments provided sufficient weed biomass reductions in Domokos, and the final grain yield was increased by up to 30% in comparison to the control (T1). The overall effects of herbicide treatments on wheat grain yield were significant (P ≤ 0.001). The yield losses due to weed competition in the control plots (T1) were important in a field dominated by V. hederifolia. This was expected, because competition from this noxious species affects wheat tillering and causes nitrogen deficiency during the stem elongation and flowering growth stages (Angonin et al. Reference Angonin, Caussanel and Meynard1996).
Table 9. Wheat grain yield (kg ha-1).

a Data were averaged across the two growing seasons (2018-2019 and 2019-2020). The means are separated according to the Fischer’s Least Significant Difference (LSD) test at a = 0.05 significance level. Different letters in the same row indicate significant differences among treatments.
In Larissa, poor wheat productivity was attributed to the T1, T6, and T7 treatments. Two years of experimentation revealed the superiority of the mixture containing clopyralid (T5) and the high application rates of 2,4-D (T3) over the rest of the herbicide treatments for controlling S. marianum. It is well established that the management of this species in cereal fields is followed by important yield increases (Turk et al. Reference Turk, Rahman and Tawaha2002). The wheat yields obtained under the highest 2,4-D application rate are contradictory to the findings of Soltani et al. (Reference Soltani, Shropshire and Sikkema2006), who attributed a 9% reduction to crop injury after 2,4-D applications. Although we had concerns for phytotoxicity regarding the application of this treatment, we did not obtain yield losses in our field trials after applying the herbicide carefully and in full accordance with the product label recommendations. The T3 and T5 treatments increased grain yield up to 30% as compared with the control (T1) or T6 treatment. Intermediate yield values corresponded with the triazolopyrimidine mixture (T4) (Table 9). Yield reductions attributable to low herbicide efficacy were anticipated in this field, because S. marianum competition can decrease the number of the grains per spike, weight of 1,000 grains, and final grain yield of wheat by 23% to 26%, 28%, and 29% to 30%, respectively (Rehman et al. Reference Rehman, Qamar, Safdar, Javeed, Shehzad, Ali, Asif, Takar, Ahmed and Jamil2020). The weed control levels induced by the different herbicide treatments did significantly impact wheat grain yield for Orestiada as indicated by ANOVA (P ≤ 0.001). In particular, the application of the triazolopyrimidine mixture (T4) developed for the simultaneous control of grasses and broadleaves increased wheat yield by 28% as compared with the treatments devoted only to the control of broadleaves (T2, T3, T5). In comparison with the control plots (T1) and the plots treated with fenoxaprop-p-ethyl (T7), the differences were even greater and ranged between 34% and 37%. Similarly, the sulfonylurea mixture was also beneficial for wheat yield performance. The very low yields in the T1 and T7 treatment plots were expected, given that a very strong competitor of wheat (e.g., A. sterilis ssp. ludoviciana) was left uncontrolled along with the presence of some broadleaves. Avena sterilis ssp. ludoviciana competition is a major threat for the productivity of wheat, as its presence in the field can lead to up to 62% yield reductions (Balyan et al. Reference Balyan, Malik, Panwar and Singh1991). This annual grass weed has been reported to be very harmful also in barley, which is generally considered to exhibit increased competitiveness against grass weeds in comparison to wheat (Dhima et al. Reference Dhima, Eleftherohorinos and Vasilakoglou2000).
Pot Experiments
The G. aparine GA (ART-1) accession derived from the first field in Aliartos (Aliartos 1) exhibited noticeable levels of resistance to the mixture of mesosulfuron-methyl + iodosulfuron-methyl-sodium. In particular, when treated with half the field dose, the plants were slightly affected, whereas after the recommended field dose, fresh weight reduction was recorded at 14% of control. The application of double the recommended dose resulted in only 30% fresh weight reduction compared with the control (Figure 3). The GR50 value (56.83 g mesosulfuron-methyl + 11.37 g iodosulfuron-methyl-sodium ha−1) of this accession was 13.2 times higher than the corresponding susceptible accession (Table 10). These results are in full accordance with the findings of Nosratti and Muhammadyari (Reference Nosratti and Muhammadyari2019), who recorded 85% survival for a G. aparine accession after treatment with the recommended field dose of mesosulfuron-methyl + iodosulfuron-methyl-sodium. The outcome was similar for the dose–response experiments carried out with the second G. aparine accession, GA (ART-2), derived from the second Aliartos field (Aliartos 2). The GR50 (41.81 g mesosulfuron-methyl + 8.36 g iodosulfuron-methyl-sodium ha−1) and RI (9.7) values were noticeable, suggesting the development of another resistant accession. A similar case has been reported during the last decade in Turkey, where the application of this sulfonylurea mixture did not affect two populations of this species when applied at the recommended field dose, and only the double dose caused significant biomass reduction (Mennan et al. Reference Mennan, Streibig, Ngouajio and Cankaya2011).

Figure 3. The responses of two Galium aparine accessions, GA (ART-1) and GA (ART-2), and one Papaver rhoeas accession, PR (THV), to six doses (0, 0.25X, 0.5X, X, 2X, 4X) of the prepackaged mesosulfuron-methyl + iodosulfuron-methyl-sodium mixture (Atlantis® WG; field dose X is 500 g ha−1 = 15 g mesosulfuron-methyl + 3 g iodosulfuron-methyl-sodium ha−1). GA (S1) and PR (S) are susceptible accessions of G. aparine and P. rhoeas, respectively (vertical bars represent the standard errors of the measurements).
Table 10. Accessions of each weed species tested for herbicide resistance. a

a The response of each accession to each herbicide is represented by the GR50 (g ai ha−1) and resistance index (RI), e.g., GR50 (R/S) ratio values derived from the dose–response experiments.
b Field: the field from which the seeds of each accession were collected.
c Accession: the abbreviated name of each accession. GA (S1) is an accession of Galium aparine susceptible to mesosulfuron-methyl + iodosulfuron-methyl-sodium. GA (S2) is an accession of G. aparine susceptible to pyroxsulam + florasulam. PR (S) is an accession of Papaver rhoeas susceptible to mesosulfuron-methyl + iodosulfuron-methyl-sodium. AS (S) is an accession of Avena sterilis ssp. ludoviciana susceptible to fenoxaprop-p-ethyl.
In the present study, the accessions GA (ART-1) and GA (ART-2) were also found to be resistant to the mixture of pyroxsulam + florasulam. Fresh weight reductions were recorded at 25% and 46% in comparison to the control for GA (ART-1) after treatment with 1X and 2X doses, respectively, while the corresponding biomass reductions for the second accession from Aliartos, GA (ART-2), were 37% and 55% (Figure 4). Galium aparine resistance to ALS inhibitors has risen, and new cases have recently been reported (Papapanagiotou et al. Reference Papapanagiotou, Damalas, Bosmali, Madesis, Menexes and Eleftherohorinos2019a). After calculating the ratios of the GR50 values of these accessions to the corresponding of the susceptible accession GA (S2), RIs were 8.3 and 6.1 for GA (ART-1) and GA (ART-2), respectively, suggesting the presence of herbicide-resistant weeds (Table 10). These results align with the recent study by Deng et al. (Reference Deng, Di, Cai, Chen and Yuan2019), in which G. aparine accessions resistant to sulfonylureas (tribenuron) were also resistant to a triazolopyrimidine herbicide (flumetsulam).

Figure 4. The responses of two Galium aparine accessions, GA (ART-1) and GA (ART-2), to six doses (0, 0.25X, 0.5X, X, 2X, 4X) of the prepackaged pyroxsulam + florasulam mixture (Broadway™ 85 WG; field dose X is 2.65 g ha−1 = 18.82 g pyroxsulam + 3.71 g florasulam ha−1). GA (S2) is a susceptible accession of G. aparine (vertical bars represent the standard errors of the measurements).
It must be noted that high NDVI values were recorded for the resistant accessions after treatment with the corresponding herbicides in our field trials carried out in Aliartos. The same pattern was observed for Thiva. A P. rhoeas accession, PR (THV), was harvested from plots treated with mesosulfuron-methyl + iodosulfuron-methyl-sodium where high NDVI values were recorded at 2 WAT. The individuals from this accession were tested for herbicide resistance, as suspected herbicide inefficacy was validated by the biomass measurements in our field trial. In fact, the development of P. rhoeas resistance to ALS inhibitors is a widespread phenomenon in the Mediterranean region and has been well documented in recent studies (Rey-Caballero et al. Reference Rey-Caballero, Menéndez, Osuna, Salas and Torra2017; Scarabel et al. Reference Scarabel, Pernin and Délye2015). After treatment with 1X, 2X, and 4X the recommended field doses, the fresh weight of the weeds was recorded at 69%, 48%, and 21% of control (Figure 3). The GR50 values were 28.43 and 5.69 g ai ha−1 for mesosulfuron-methyl and iodosulfuron-methyl-sodium, respectively, resulting in a noticeable RI value (6.2) after calculating the GR50 (R/S) ratio with the GR50 value of a susceptible accession. Resistance to mesosulfuron-methyl + iodosulfuron-methyl-sodium was also reported in the study by Kaloumenos et al. (Reference Kaloumenos, Adamouli, Dordas and Eleftherohorinos2011), in which the applications of 4X the recommended rate did not cause significant fresh weight reductions in populations of P. rhoeas. The situation regarding the resistance status of this weed to mesosulfuron-methyl + iodosulfuron-methyl-sodium has been aggravated in the Greek cereal fields (Kati et al., Reference Kati, Scarabel, Thiery-Lanfranchi, Kioleoglou, Liberopoulou and Délye2019).
Similar concerns arose regarding A. sterilis ssp. ludoviciana resistance to fenoxaprop-p-ethyl in Orestiada after the NDVI measurements and weed biomass measurements. The responses of the individuals of this accession validated the hypothesis that the high NDVI values were observed due to the presence of herbicide-resistant weeds that were not affected by the ACCase herbicide. In particular, the applications of fenoxaprop-p-ethyl at 0.5X, 1X, and 2X the recommended field doses resulted in only 10%, 26%, and 39% fresh weight reductions compared with the control. Applying the herbicide at 4X the recommended rate reduced A. sterilis ssp. ludoviciana biomass by 56% (Figure 5). The high GR50 value obtained (272.4) indicated that the accession was 8.8 times more resistant than a susceptible accession after calculating the GR50 (R/S) ratio (Table 10). These results are in line with those of previous study in which the resistance indices of A. sterilis ssp. ludoviciana to fenoxaprop-p-ethyl ranged between 4.1 and 9.4 (Travlos et al. Reference Travlos, Giannopolitis and Economou2011). Accessions of A. sterilis ssp. ludoviciana from northern Greece were reported to be four times more resistant to fenoxaprop-p-ethyl and a wide range of ACCase-inhibiting herbicides in a very recent study (Papapanagiotou et al. Reference Papapanagiotou, Damalas, Menexes and Eleftherohorinos2019b). Selective grass weed control in the wheat fields of Greece was mainly dependent on the repeated use of ACCase-inhibiting herbicides during the previous years. The situation regarding herbicide resistance has been aggravated, and alternative strategies are required for the management of this harmful species, whose resistant populations tend to be more competitive than the susceptible ones (Travlos Reference Travlos2013).
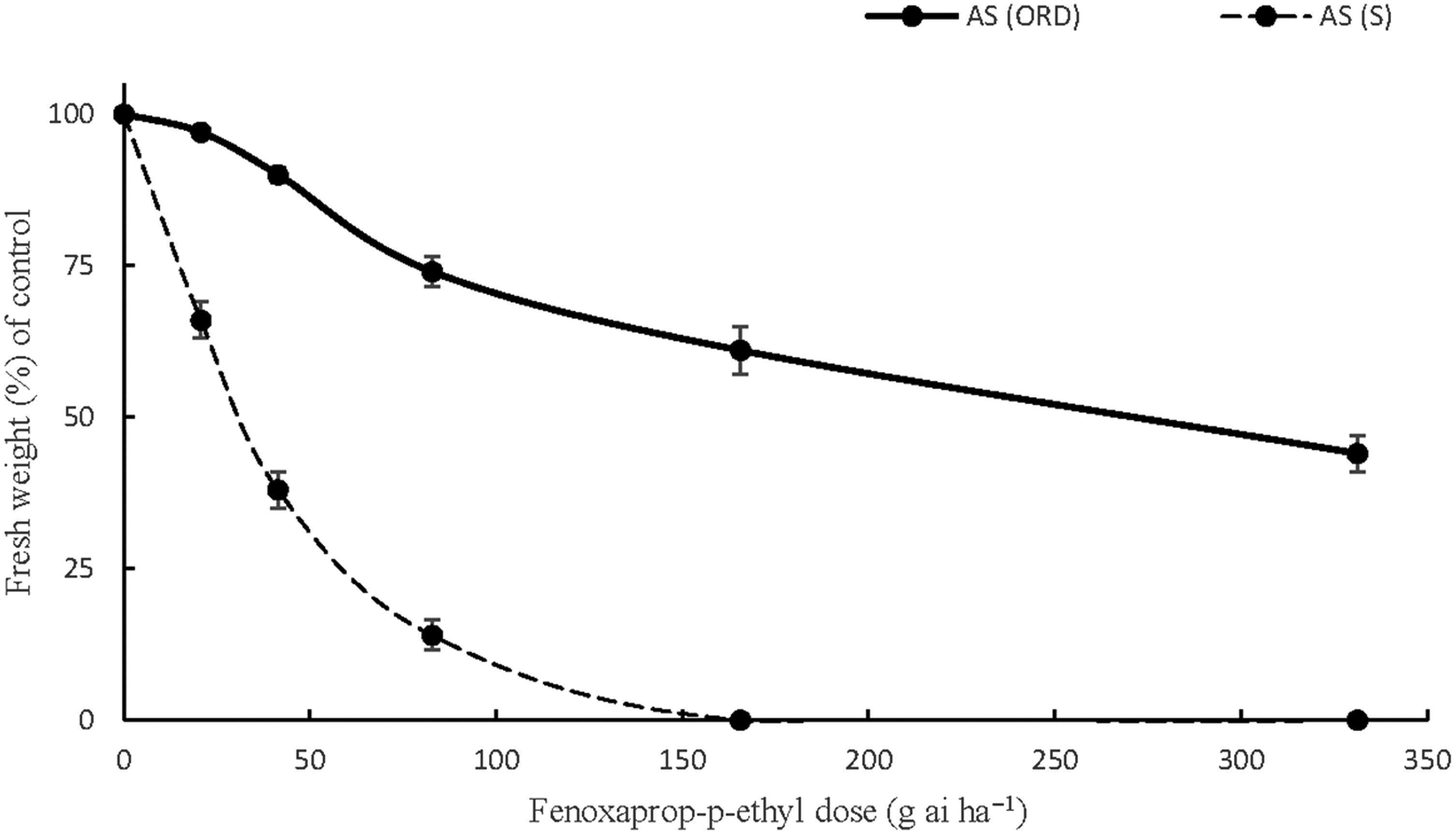
Figure 5. The responses of one A. sterilis ssp. ludoviciana accession, AS (ORD), to six doses (0, 0.25X, 0.5X, X, 2X, 4X) of fenoxaprop-p-ethyl (field dose X = 82.8 g ai ha−1). AS (S) is a susceptible accession of Avena sterilis ssp. ludoviciana (the vertical bars represent the standard errors of the measurements).
To summarize, the pot experiments results support our hypothesis suggesting that high NDVI values recorded after herbicide application might be linked with the presence of herbicide-resistant weeds in a cultivated area. A critical issue is the optimum timing for capturing NDVI values to determine which herbicide is efficient according to each field’s special needs for weed control. We intend to pursue this goal with further experimentation. In any case, the present study is targeted at making a significant contribution toward establishing cause–effect relationships and presenting a useful tool for developing more effective weed management practices in more arable crops and under different soil and climatic conditions.
Acknowledgments
This research received support from CortevaTM (“Quick-Test for the Optimization of Herbicide Use and In-Season Recommendations to the Farmers” project, ELKE code: 340456). No conflicts of interest have been declared.