Introduction
The gender wage gap, which indicates that a man’s wage is higher than a comparable woman’s, is a significant worldwide issue (Manning and Robinson Reference Manning and Robinson2004; Blau and Kahn Reference Blau and Kahn2007). In 2020, the World Bank released a report entitled, How large is the gender dividend? Measuring selected impacts and costs of gender inequality. The report indicates that women’s human capital wealth could be more than doubled if they were paid as much as men. For an extended period, many governments and international organisations have committed to reducing the enormous costs caused by gender inequality, and the Chinese government is no exception.
During the planned economy period, the highly centralised wage distribution system helped to narrow the gender wage gap in China. The ratio of urban women’s labour income to men’s was 82.5%, much higher than in other countries (Meng and Kidd Reference Meng and Kidd1997; Gustafsson and Li Reference Gustafsson and Li2000).
After 1990, however, the gender wage gap in China widened significantly (Maurer-Fazio and Hughes, Reference Maurer-Fazio and Hughes2002; Zhang Reference Zhang2004; Qi and Liang Reference Qi and Liang2016). First, this phenomenon arises because of the conversion of the wage determination mechanism. China transitioned from a planned economy to a market economy in 2001, and the employment system also changed from a government-led designed employment mechanism to a market-oriented one. The second reason is the change in the employment structure. The restructuring and reorganisation of state-owned and collective enterprises have led to abundant unemployment and layoffs, and the female layoff rate is twice that of men. Women also have a higher early retirement rate, resulting in decreased labour participation and income (Gustafsson et al Reference Gustafsson, Shi and Sicular2008). We can see the trend clearly in Figure 1. The third reason is the impact of rural migrant labour. The Chinese government has implemented an active policy of encouraging, guiding, and supporting rural labour mobility since 2000. The interaction of these three factors has led to a widening gender wage gap.
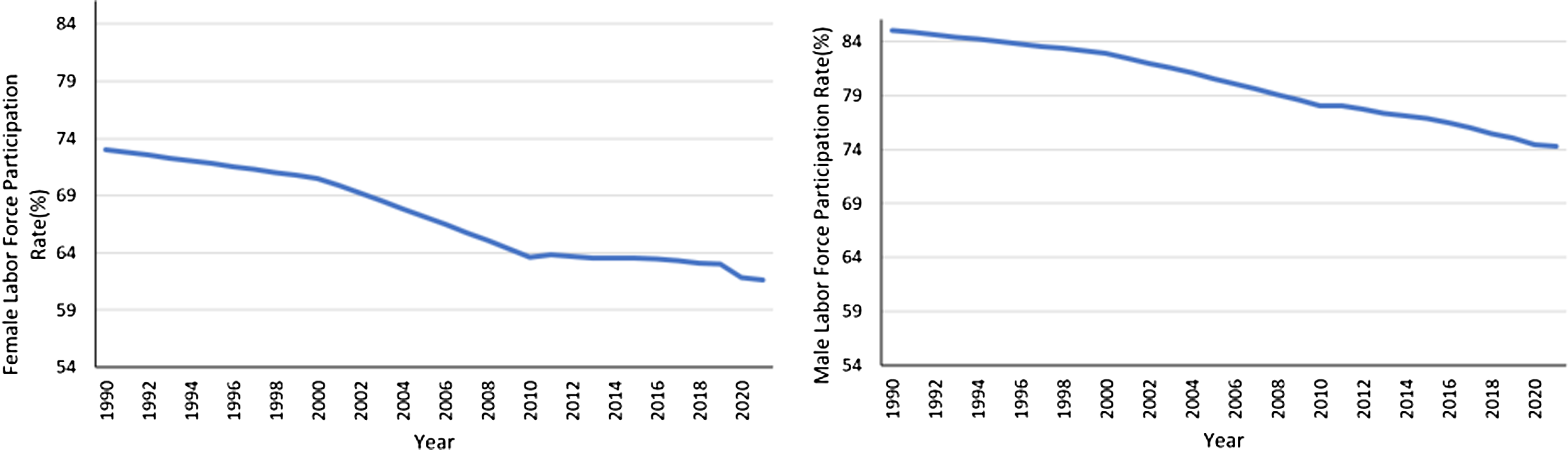
Figure 1. Labour force participation rate in urban China (1990–2021). Data source: The World Bank.
The World Economic Forum has published the Global Gender Gap Report annually since 2006. According to this report, women’s labour income compared to men’s in China was 66% in 2006, dropping to 61% in 2020. The smaller the value, the wider the gender wage gap, which has increased by 7.58% in 15 years. Figure 2 shows this trend.
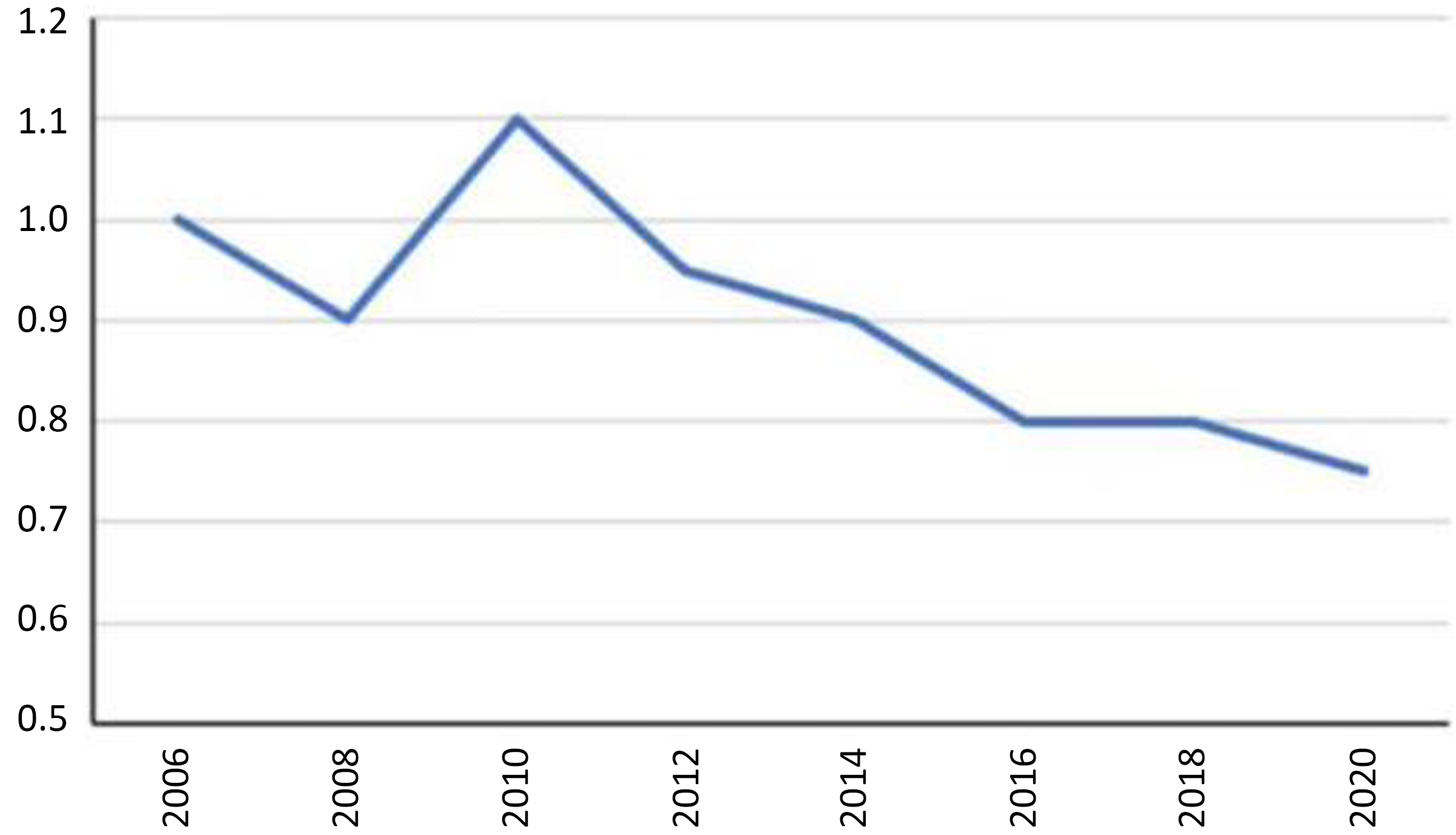
Figure 2. Ratio of women’s labour income to men’s in urban China (2006–2020). Data source: Global Gender Gap Report from the World Economic Forum.
Due to China’s sluggish fertility rate, shrinking working-age population, and severe ageing problems, the strict population control policy seemed no longer appropriate. In response to these trends, the universal two-child policy was officially implemented nationwide on 1 January 2016, allowing all Chinese couples to have two children. On the other hand, motherhood is vital in bringing women to a disadvantage in the labour market. Indeed, employers treat women with children unequally (e.g. Budig and England Reference Budig and England2001; Doren Reference Doren2018). Considerable empirical evidence indicates a negative relationship between a woman’s number of children and her wage, commonly known as the ‘motherhood wage penalty’ (Torzewska and Matysiak Reference Torzewska and Matysiak2020; Wu Reference Wu2022; Huang and Jin Reference Huang and Jin2022; Casarico and Lattanzio Reference Casarico and Lattanzio2023). Our study is fuelled by our concern about whether this policy adversely affected the gender wage gap and led to a more severe motherhood penalty and gender discrimination in China’s labour market.
With the China Family Panel Studies (CFPS) data, we employ the difference-in-differences-in-differences (DDD) model to assess the effect of China’s universal two-child policy on the gender wage gap. Our empirical study shows that China’s universal two-child policy has significantly expanded the urban gender wage gap by 12.86% in the low-policy-fertility-rate (PFR) provinces versus high-PFR provinces. The policy expands the gender wage gap by 18.53% for younger employees while having no significant effect on those above 40 years old. It also widens the gender wage gap by 36.48% for low-educated people, while the outcome is only 11.40% for high-educated employees. Furthermore, the gender wage gap was not noticeably affected by the universal two-child policy in the implementation year but significantly expanded 2 years later. Finally, there has been more severe gender discrimination in China’s labour market since the introduction of the universal two-child policy.
Compared with the existing literature, our possible contributions are as follows:
First, we empirically analyse the effect of China’s universal two-child policy on the gender wage gap using the DDD model and objectively assess some policy consequences, which are rarely involved in the existing literature. Since China’s family planning policy in the 1960s, and especially since the introduction of a more stringent fertility policy in 1979, many worldwide scholars have studied the possible effects of fertility restrictions in a comprehensive manner and accumulated a wealth of research results. However, research on the effects of fertility policy relaxation is rare. By analysing the impact of the universal two-child policy on the gender wage gap, this paper could have significant implications for understanding women’s fertility intention, female labour rights, and social policymaking in China.
Second, most empirical studies on the ‘motherhood penalty’ have focused on how fertility behaviour affects a mother’s labour market performance. Nevertheless, China has converted the mandatory, stable, and long-term one-child policy to a two-child policy, affecting mothers and influencing those who have not given birth yet. It provides a unique opportunity to investigate the systemic influence of fertility policy on the entire women’s workforce, which is a beneficial and illuminating way to address the ‘motherhood penalty’.
Third, we explore the heterogeneity and underlying mechanisms of the policy effect. We divide women into specific groups based on age and education. Younger or lower-educated women are more adversely affected by this policy than other women, which can practically guide the feasible measures to realise gender equality for more disadvantaged groups. Meanwhile, we empirically conclude that because women are allowed to give birth more than once, employers strengthen their preference for males, leading to further severe gender discrimination.
Background and literature review
This section discusses the evolution of China’s family planning policy, which has profoundly influenced the whole country and has dynamically evolved for more than half a century. Meanwhile, it is essential to review the literature on this important topic.
Evolution of family planning policy in China
Chinese society experienced sizeable population growth during the following decade after 1949. The total fertility rate (TFR) reached more than six births per woman in the early 1960s (Banister Reference Banister1987). The rapid population expansion directly pushed China’s central government to be concerned about birth control in 1962, which was the beginning of China’s family planning policies (Liang Reference Liang2014).
The government set birth quotas to decrease the number of children; each couple was allowed to have from three to two during the 1970s. Despite this downward fertility trajectory, fears of overpopulation have persisted since the baby boomers born after the great famine entered their reproductive ages. The one-child policy, the world’s strictest family planning policy, was implemented in 1979. It requested that each Han Chinese couple have no more than one child, and a second child was permitted only under particular circumstances.
Under the so-called one-and-a-half-child policy, the one-child policy was gradually liberalised by allowing a couple in rural areas, where around 70% of the total population lived, to have a second child if the first child was a girl. After 1982, more provinces relaxed the conditions that qualified people to have a second child. Until 2007, all provinces in China (except Henan, which followed in 2011) had permitted couples who were both the only child to have a second child.
Since 1990, the fertility policy has become relatively stable, and the TFR has been lower than the replacement level in China. The Chinese government has commenced liberalising the population control policy gradually. Couples with only one partner who was an only child were generally allowed to have a second child around 2014. On 15 October 2015, the Chinese government announced it would implement a universal two-child policy on 1 January 2016. Figure 3 shows the trend of the total fertility rate in China (1960–2019).
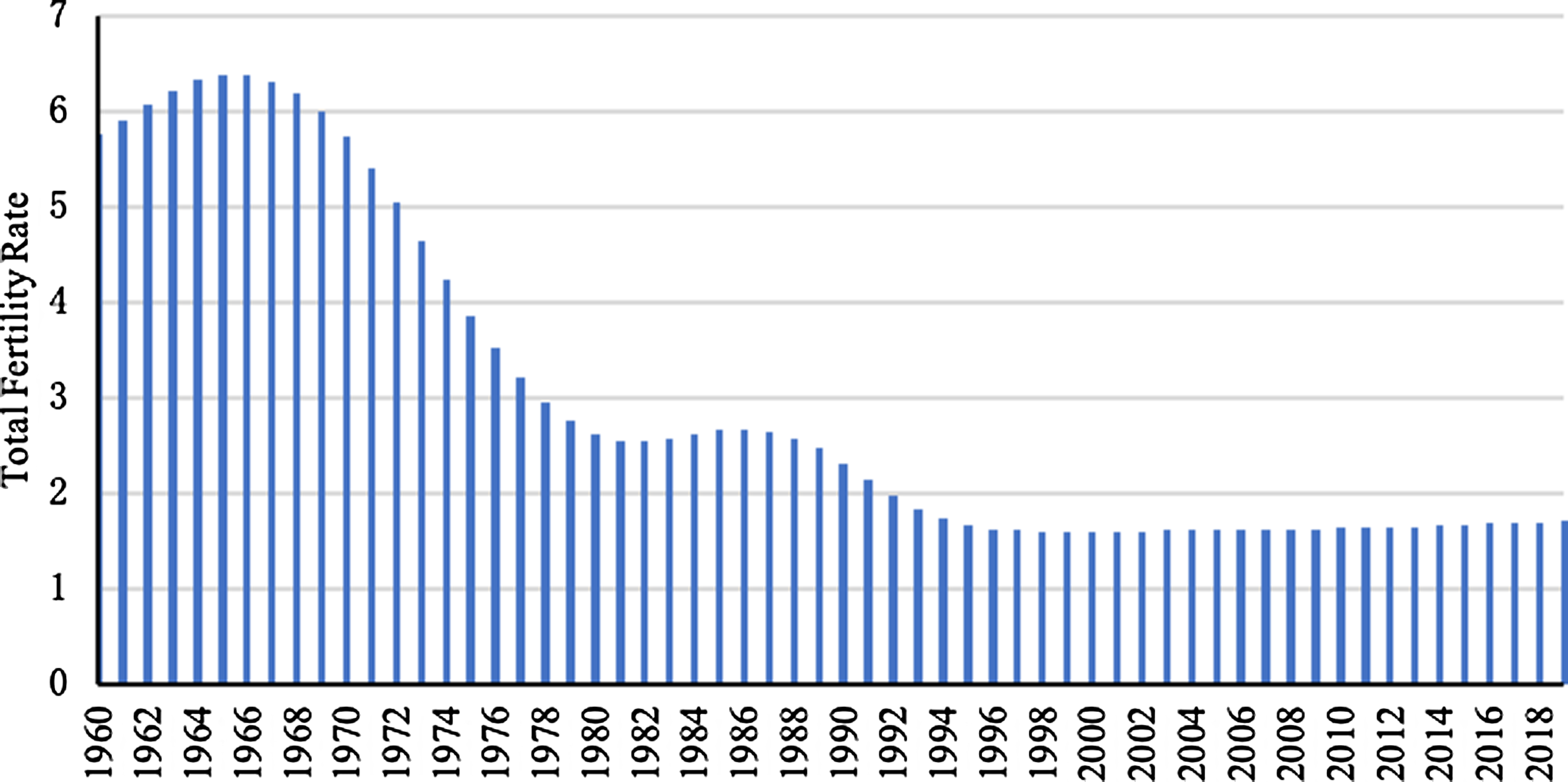
Figure 3. The trend of the total fertility rate in China (1960–2019). Data source: The World Bank.
Literature review
Extensive research has been stimulated by an increasing awareness of the importance of the gender wage gap in the labour market (e.g. Miller Reference Miller1987; Farrell Reference Farrell2005; Blau and Kahn Reference Blau and Kahn2017), which is often decomposed into explained and unexplained contributing factors. Reasons offered and evaluated often refer to observable personal characteristics, including labour participation (e.g. Goldin Reference Goldin2006), employment segregation (e.g. Groshen Reference Groshen1991; Bayard et al Reference Bayard, Hellerstein, Neumark and Troske2003; Goldin Reference Goldin2014), and human capital characteristics (e.g. Acemoglu and Pischke Reference Acemoglu and Pischke1999; Goldin Reference Goldin2014; Cebrián and Moreno Reference Cebrián and Moreno2015), including education attainment (Goldin et al Reference Goldin, Katz and Kuziemko2006; Becker et al Reference Becker, Hubbard and Murphy2010), work experience, and on-the-job training (Mincer and Polachek Reference Mincer and Polachek1974). The unexplained causes are often identified as discrimination since they are difficult to measure, even if not all of them are due to discrimination.
Recent studies have found that the explained causes of the gender wage gap are decreasing because of higher female labour participation, higher female human capital, and less gender segregation. Simultaneously, the unexplained elements of the gender wage gap have been increasing (Razzu Reference Razzu2014; Boll et al Reference Boll, Leppin, Rossen and Wolf2016), including but not limited to noncognitive skills, gender norms, and institutional factors. Among these unexplained causes, women’s fertility behaviour is quite significant.
First, extensive studies show that women’s labour supply and incomes decline significantly with increasing numbers of children (e.g. Moffitt Reference Moffitt1984; Waldfogel Reference Waldfogel1997; Angelov et al Reference Angelov, Johansson and Lindahl2016). Waldfogel (Reference Waldfogel1997) finds that hourly wages are reduced by 6% for women with one child and 13% for those with two children compared to unmarried women using data from 1968 to 1988 in the USA. Jia et al (Reference Jia, Gan and Zhang2013), using China Health and Nutrition Survey (CHNS) data from 1991 to 2009, find that having a child induces an 18% decrease in female earnings, adversely affecting women’s fertility demand. Yu and Xie (Reference Yu and Xie2014), using data from 1993 to 2020, demonstrate that having one child reduces 7.0% of women’s wages, and having two children results in a 16.8% reduction compared to those with no children. Motherhood brings about income loss throughout women’s entire careers (International Labour Organization 2018), a pattern that affects women’s marriage, fertility, and labour participation decisions.
Second, childbirth and parenting responsibilities are evidently unequal for women and men, so they play a crucial role in the gender wage gap. However, empirically analysing these responsibilities takes work (Kahn and Fabian Reference Kahn and Fabian2014). Among new employers, studies show that men and women with the same education tend to enter at similar wage levels. The wages begin to diverge during their early career (Fitzenberger and Kunze Reference Fitzenberger and Kunze2005; Manning and Swaffield Reference Manning and Swaffield2008), and the divergence substantially increases after women have children (Mincer and Polachek Reference Mincer and Polachek1974; Bertrand et al Reference Bertrand, Goldin and Katz2010). Women’s anticipation of family responsibilities can influence their choice of occupation at an early age. During maternity leave, women will experience occupational downward, long-term wage decline, and a low re-employment probability, even after only a few months (Angrist and Evans Reference Angrist and Evans1996; Stone Reference Stone2007; Qi and Dong Reference Qi and Dong2016). After giving birth, the wage gap is even more prominent because mothers are more inclined to seek part-time instead of full-time jobs or switch their work to more flexible, less labour-intensive, less committed, or lower-skilled employment (Becker Reference Becker1985; Juhn and Mccue Reference Juhn and Mccue2017). Such translations often disadvantage their wages (Adair et al Reference Adair, Guilkey, Bisgrove and Gultiano2002). Women undertake long-term caregiver duties after giving birth to a child; thus, childrearing and parenting behaviours are detrimental to their paid employment (Lokshin Reference Lokshin2004).
Third, there is limited literature on the impact of fertility policy relaxation on urban women’s labour market performance. He and Zhu (Reference He and Zhu2016) argue that the labour supply of urban women in China is less negatively affected by the fertility policy and conclude that the relaxation of the one-child policy did not significantly affect women’s labour force participation in 1990 and 2000. Lai (Reference Lai2017) shows that the universal two-child policy harms urban women’s labour force participation. Wang and Qiao (Reference Wang and Qiao2018) assert that the relaxation of the one-child policy will lead to early retirement and lower labour force participation. Agarwal et al (Reference Agarwal, Li, Qin and Wu2019) use a proprietary employer–employee matched dataset from an anonymous major Chinese city and find a 22% increase in the gender wage gap due to the selective two-child policy (if one parent is from an only-child family, the couple is allowed to have a second child). Liu and Liu (Reference Liu and Liu2020) think a significant U-shaped relationship exists between female income and the two-child policy fertility choice. Huang and Jin (Reference Huang and Jin2022) show that the universal two-child policy has significantly reduced women’s employment by 4.06% and decreased their labour income by 10.43%. Leng and Kang (Reference Leng and Kang2022) reveal that expanding the scope of the two-child policy exhibits no reduction in the proportion of female performance in Chinese listed companies and the economic benefits of female employment for firms result from wage discrimination. Zhao et al (Reference Zhao, Zhang, Lu and Wen2023) focus on the migrant workers and find that China’s pilot two-child policy reduced female labour force participation and increased the gender wage gap.
Empirical strategy and data description
This section will briefly introduce the database and elaborate on our empirical strategy. Based on the DDD estimator, the coefficient of the triple interaction term, we can identify the changes in the gender wage gap in low-PFR provinces versus high-PFR provinces attributed to the universal two-child policy by the triple interaction.
Data description
The CFPS database, collected by the Institute of Social Science Survey (ISSS) Centre of Peking University, is utilised in our research. The database was launched in 2010 and has been the subject of further investigation every 2 years. These surveys cover 25 provinces in China, and these nationally representative panel data support our research.
Empirical research first requires the identification of the sample. We restrict participants to those who are between 16 and 55 years old. We set 16 as the lower bound because it is the age threshold for China’s labour force, and employed workers under 16 years old are rare. We use 55 years old as the upper bound, the statutory female retirement age Footnote 1 in China. Meanwhile, we exclude full-time students and incapacitated or retired people. Since engaging in agriculture differs from formal employment in urban cities, we also exclude those with rural hukou (household registration) from the sample. After deleting the missing values, we obtained 2255, 2237, 953, and 2179 observations in 2012, 2014, 2016, and 2018, respectively. Summary statistics are given in Table A1 in the appendix.
Specifically, we consider the following variables:
Our dependent variable is Log wage (lnY), which represents the logarithm of individual i’s average monthly wage from the main job during the past year in the renminbi (CNY). We make the price adjustments using 2010 as a benchmark in order to compare wages in different years. Also, the data are two-sided 1% winsorised to eliminate outliers.
The control variables include age, age squared, work experience, work experience squared, the number of young children, a set of education level dummies, occupation dummies, and industry dummies Footnote 2 . Table A2 in the appendix shows a more detailed description of above variables.
Policy fertility rate
Because of the endogenously determined local regulations and the one-child policy’s discrepant implementation in each province (McElroy and Yang Reference McElroy and Yang2000), the universal two-child policy’s influence differs throughout provinces. Instead of TFR, we choose the ‘PFR’ in each province as the indicator to divide the sample since TFR is often deemed to be inaccurate in China (e.g. Guo Reference Guo2011; Wang and Ge Reference Wang and Ge2013; Chen and Yang Reference Chen and Yang2014). According to the local fertility policy, the PFR is defined as the average number of children born if a woman gives birth according to the local policy during her lifetime (Guo et al Reference Guo, Zhang, Gu and Wang2003). Since the local fertility policy in every province is definite, the PFR in 30 provinces would be accurate, so we have sufficient confidence in the data quality. Also, the PFRs in each province are different because the local authority has considered their regional economic growth, social development, population situation, and widely various implementation variables across the provinces, such as local-specific situations, fines, or subsidies. We chose the PFR of each province in 2010, which is more relevant and exogenous for the universal two-child policy in 2016, and show them in Table 1.
Table 1. PFR of China’s 30 provinces in 2010 (population-weighted corrected)

Note. Yin, Yao and Li (Reference Yin, Yao and Li2013).
The provinces with a lower PFR under the one-child policy are more significantly affected by the universal two-child policy because of stricter birth control before. The coefficient is –0.0035 and significant at the 10% level when we roughly use PFR to explain the birth rate difference in these provinces between 2010 and 2018. The assumption is verified. Therefore, in the DDD design, we divide the treated provinces and control provinces according to PFR. We regard the provinces with lower PFR as treated provinces, which are expected to be more significantly affected by the universal two-child policy. We use the population-weighted national PFR of 1.416 calculated by Yin et al (Reference Yin, Yao and Li2013) as the cut-off rate. After eliminating Tibet, Macao, Hong Kong, and Taiwan, we put 18 provinces as the treatment group (including Tianjin, Beijing, Inner Mongolia, Heilongjiang, Jiangsu, Shanghai, Jiangxi, Shandong, Guangdong, Henan, Chongqing, Sichuan, Guangxi, Hainan, Yunnan, Shanxi, Qinghai, and Ningxia) and 12 provinces as the control group (including Liaoning, Jilin, Hubei, Zhejiang, Hunan, Anhui, Fujian, Shanxi, Gansu, Hebei, Guizhou, and Xinjiang).
According to the Statistical Yearbooks in each province, the birth rate growths for the treatment group are much higher than that for the control group after the universal two-child policy. Taking 2014 as a reference, the birth rate growths are 7.38%, 10.45%, and 0.35% for the treatment group and 1.37%, 5.16%, and −6.44% for the control group in 2016, 2017, and 2018, respectively, as shown in Figure 4. The growths in birth rate differ significantly between the control and treatment groups, and the latter is much more sensitive to the universal two-child policy. The assumption is affirmed to be valid.
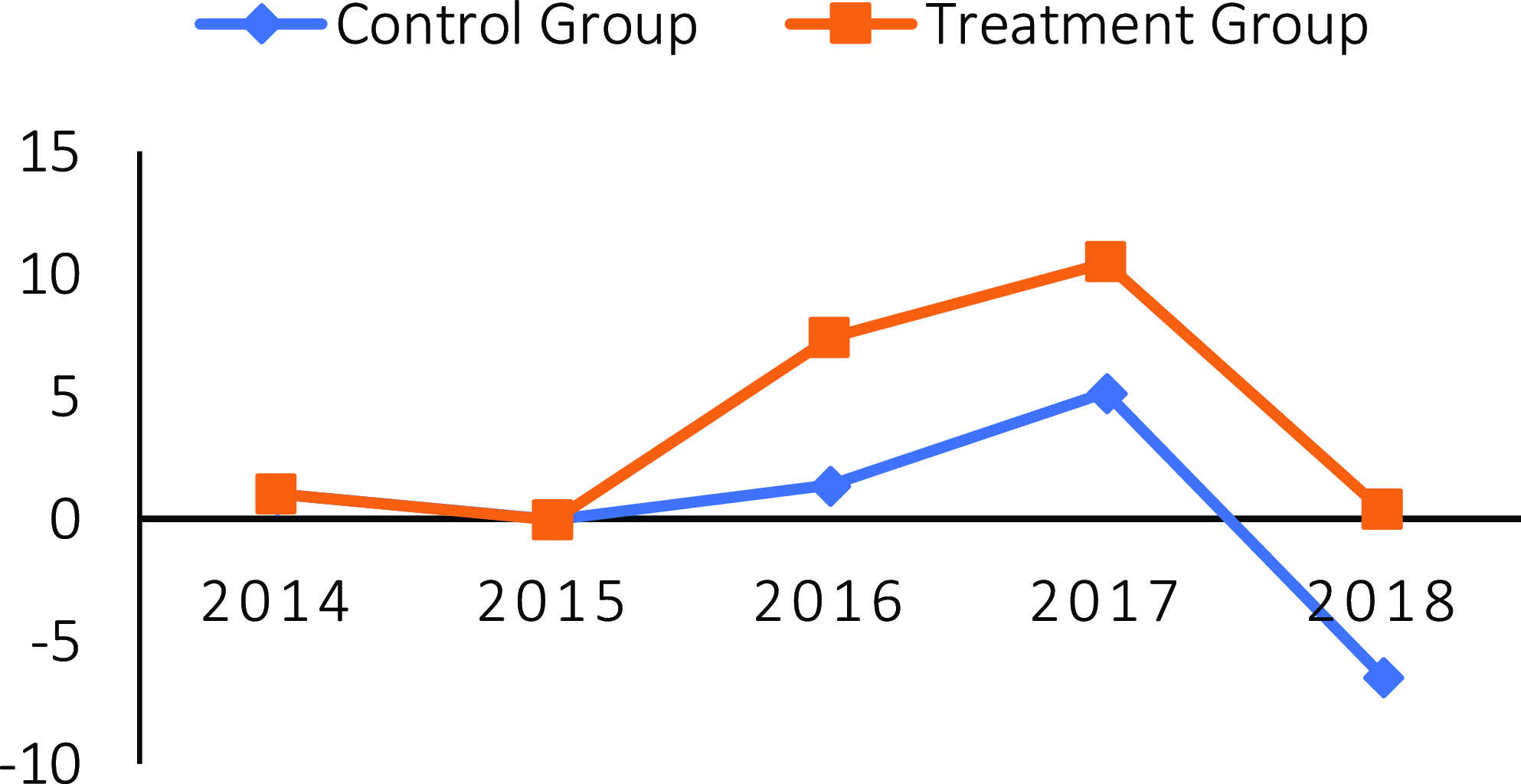
Figure 4. Annual birth rate growth in China, 2015–2018.
Also, when measuring the trend of the gender wage gap between 2012 and 2018, the PFR used in 2010 can be regarded as an exogenous variable.
Model specification
To avoid potential endogenous problems, such as omitted variables or reverse causality, and to improve the estimation accuracy, this paper employs the DDD model to assess the effect of China’s universal two-child policy on the gender wage gap. Specifically, the first level of the difference stems from the provinces’ (high or low PFRs), the second level relates to time (before or after the policy) and the third level is about gender (male or female). On the one hand, we have illustrated that the treated provinces with lower PFRs tend to be more sensitive to the universal two-child policy. On the other hand, females bear more responsibility for childbirth and care in the family, so they are more likely to suffer from the motherhood penalty after the two-child policy relative to males, which leads to poor performance in the labour market and the widening gender wage gap caused by the universal two-child policy. Therefore, we essentially consider women as the treated population whose wage will be more negatively affected by the policy, while males will be regarded as the non-treated population. We could control the observables by adding demographic characteristics to the equation and eliminate the unobservables by the triple difference. In this way, we can estimate the average change in the outcome variable for females relative to males in low-PFR provinces versus high-PFR provinces after the policy, which could be interpreted as the average treatment effect on the gender wage gap in the treated provinces.
The universal two-child policy has been implemented since 1 January 2016; therefore, we use the sample in 2012 and 2014 as the pretreatment group while 2016 and 2018 as the post-treatment group.
In practice, we use the DDD model to examine the policy’s effect. Consider

where
$\;i,\;t,\;{{and}}\;p\;$
denote the individual, time, and province, respectively; ln
${Y_{it}}$
denotes the logarithm of the individual’s after-tax wage in the year
$t$
from the main job each month. treat is a binary variable equal to 1 for the treated provinces (where PFR is lower than 1.416) and 0 for the control provinces (where PFR is higher than 1.416). It captures region-specific time-invariant differences between low-PFR and high-PFR provinces. female is a binary variable equal to 1 for the female population group and 0 for the male group. It captures gender-specific time-invariant differences between females and males.
$post$
is a binary variable equal to 0 before the policy and 1 afterwards. The coefficient of
$trea{t_p} \times fema{e_i} \times pos{t_t}$
represents the percentage difference change of on female wages relative to male wages between low-PFR provinces and high-PFR provinces after the policy, indicating the average treatment effect on the gender wage gap in treated provinces.
In addition,
${{\;}}{\mu _i},{\theta _t},$
and
$\;{\delta _p}$
indicate the individual, year, and province fixed effects, respectively. We put
$trea{t_p},femal{e_i},{{pos}}{{{t}}_{{t}}}$
and their pairwise interaction terms in the equation, where
$trea{t_p},femal{e_i},pos{t_t}\;$
is absorbed by province, individual, and year fixed effect separately.
${\varepsilon _{it}}$
is the robust standard error clustering at the individual and provincial level to deal with heteroscedasticity and autocorrelation.
Using the DDD model, the unbiased estimation of
$\beta $
requires more variables to provide counterfactuals to reflect the state if the policy was not implemented. As mentioned before, we put a vector of variables
$\boldsymbol{X_{it}}$
in the equation to control some demographic characteristics contributing to the income.
A vital precondition for identification using the DDD model is exogenous treatment, including the exogeneity of policy implementation time and province division in the treatment and control group. On the one hand, China’s main internal reason for implementing the universal two-child policy is the increasingly prominent demographic structural issues, such as deepening ageing and shrinking labour force. Such macro-population policy transformation is not influenced by micro-individual performance. The change in the gender wage gap, in which we are interested, is only one potential consequence of the universal two-child policy, so the implementation time of the policy can be considered exogenous. In addition, the selected two-child policy, which is close to the universal two-child policy, may also have an impact on the results. We run a placebo test in robustness check and rule out this possibility.
On the other hand, our sample starts in 2012 but is grouped using the 2010 provincial PFRs. Therefore, the division between treated and control provinces can be considered ‘predetermined’ and will not be affected by individual characteristics after 2012. It should also be noted that the calculation of the PFR depends on some demographic characteristics, such as the ratio of one-child families, the ratio of the population with urban and rural Hukou, and the ratio of minorities in each province. Therefore, our grouping is not random, and there may be significant differences in the above characteristics between provinces with high and low PFR. These differences may persist over time. In the robustness check, we include the interactions of the population structure characteristics in 2010 and mentioned above, as well as the time trend in the model to control their time-varying trends, so we can more rigorously verify the conditional exogeneity.
Effect of the universal two-child policy on the gender wage gap
This section examines the validity of the DDD model’s parallel hypothesis and observes the policy’s effect dynamics. Then, we assess whether China’s universal two-child policy has affected the gender wage gap and estimate the magnitude.
Parallel trend hypothesis
The DDD estimation must satisfy the parallel trend hypothesis, which requires parallel and consistent trends in the treatment and control group before the fertility policy. Specific to our model, this means the trend of change in the gender wage gap should be slightly later than the universal two-child policy.
We use the event study methodFootnote
3
to confirm this assumption. We take the data from 2012 as a reference and use
$year_{}^\tau $
as dummy variables to represent the year, so we have the expanded DDD model:

where
$year_{}^\tau $
(
$\tau $
= 2014, 2016, and 2018) is the dummy variables and equal to 1 if the year is
$\tau $
; otherwise, 0.
The regression results are shown in column 1 of Table 2. The coefficient of
$trea{t_p} \times femal{e_i} \times yea{r^{2014}}\;$
is not statistically significant, while the coefficients of
$trea{t_p} \times femal{e_i} \times yea{r^{2016}},$
$trea{t_p} \times femal{e_i} \times yea{r^{2018}}$
are significant at the 5% level. These results demonstrate that the gender wage gap is not shocked by the universal two-child policy before it came into effect.
Table 2. The regression of the DDD model

Note . Due to space limitations, we omitted the regression results for all control variables. The complete results are kept for request. t-statistics are in parentheses. FE includes the individual, year, and province fixed effects.
* Represent significance levels of 10%.
** Represent significance levels of 5%.
Figure 5 shows the result of the parallel-trend test more apparently. We can see that before 2016, the interaction coefficient is not significantly different from 0 (the 95% confidence interval contains 0) but is significantly negative after the policy. Thus, the gender wage gap is not affected by the universal two-child policy’s effect before 2016.

Figure 5. The result of the parallel-trend test.
Baseline DDD model
We estimate the universal two-child policy’s effect on the gender wage gap using the multidimensional fixed-effect model with panel data. After controlling the individual characteristic variables discussed above, the coefficient on female×post indicates the average percentage wage change for females versus males in the control provinces after the policy, which could be interpreted as the change of gender wage gap in the control provinces; similarly, the coefficient of treat×female×post could be interpreted as the change of gender wage gap in the treated provinces versus control provinces (i.e. the average treatment effect of the universal two-child policy on gender wage gap in treated provinces), which is our primary concern. Column 3 of Table 2 shows that the coefficient of female×post is –0.352 but is not statistically significant; the coefficient of treat×female×post is –0.121 and is significant at the 5% level, which indicates that the gender wage gap expands by 12.86% (
${e^{0.121}} - 1$
) in the treated provinces versus control provinces. The minus sign indicates a negative correlation between the DDD estimator and the explained variable, which implies that in provinces with a higher PFR in 2010, the effect of the universal two-child policy on the gender wage gap is less significant than that in provinces with a lower PFR. It is consistent with our previous assumption.
In addition, on the one hand, women may turn to industries and jobs with lower wages but more flexibility; on the other hand, they may suffer more gender discrimination when searching for a new job. Both of these could be reasons to explain the widening gender wage gap after the universal two-child policy. We exclude the industry and occupation dummies and re-estimate the baseline model to check this possibility. Column 2 of Table 2 shows that the coefficient on treat×female×post is –0.129, and the absolute value is seemingly larger than the result in column 3. There is some evidence indicating that female job switching during fertility could lead to widening gender gap.
Robustness check
Our model’s robustness may be influenced by sample selection, model specification, and within-sample heterogeneity; therefore, we conducted a series of checks to verify our results. First, we provided a placebo test by constructing the false policy time. Second, more variables were put into the baseline model. Third, we employed other DDD specifications to examine the policy effect.
Placebo test
We also ran a placebo test by constructing a false policy time. If the DDD coefficient was not zero in statistics based on the false policy time; the expansion of the gender wage gap could be arguably seen to stem from other factors such as the selective two-child policy. Otherwise, we can confirm that the universal two-child policy indeed changed the gender wage gap.
We artificially set 2014 as the policy year. So, 2012 is the year before the policy, while 2014, 2016, and 2018 are post-policy years. Column 1 of Table 3 shows that the DDD coefficient is insignificant. Therefore, we conclude that the effect of the university two-child policy is substantial. What is more, our placebo test could also exclude the impact of the selected two-child policy which was implemented in 2014.
Table 3. Robustness check
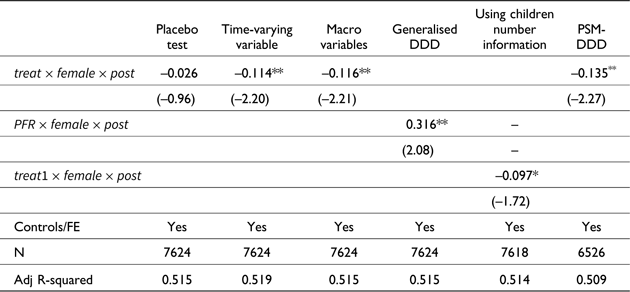
Note. Due to space limitations, only the interaction’s coefficients are reported, and the control variables are omitted but kept for request. t-statistics are in parentheses. FE includes the individual, year, and province fixed effects.
* Represent significance levels of 10%.
** Represent significance levels of 5%.
Controlling more variables
Controlling interactive fixed effects
To alleviate the time-varying influence of occupation and industry on the gender wage gap, we added the occupation-year fixed effect and industry-year fixed effect to the regression. The DDD coefficient in column 2 of Table 3 is still significantly negative.
The model demonstrates that after controlling the occupation and industry trend in different years, we get similar results as the baseline model. Our main conclusion is, therefore, robust and reliable.
Controlling macro variables
The control variables in the benchmark model, such as age, work experience, and education, are all microdata at the individual level. However, the macroeconomic environment also significantly impacts wages and the gender wage gap. We added the gross domestic product growth rate, the contribution rate of the tertiary industry, and the urbanisation rate of each province to eliminate the interference of macroeconomic factors. Specifically, as mentioned above, we controlled the interactions of the ratio of one-child families, the ratio of the population with urban and rural hukou, the ratio of minorities in each province in 2010, and time trend, in order to control their time-varying trends, so that we can more rigorously verify the conditional exogeneity. Table 3 shows that the supplemental macroeconomic environment does not significantly change the magnitude or significance of the coefficient of
$treat \times female \times post$
. From this perspective, the benchmark model is reliable.
Alternative DDD specifications
Generalised DDD
Following the identification strategy by Duflo (Reference Duflo2001), we use the numerical PFR as a continuous variable instead of dichotomy classification to measure the differences in policy sensitivity among provinces. The provinces with a lower PFR are more sensitive to the universal two-child policy.

where PFR is the policy fertility rate of each province in 2010.
Column 4 of Table 3 shows that the DDD estimator possesses a plus sign. The coefficient is 0.316 and significant at the 5% level, which indicates that the gender wage gap expands by 37.16% (e 0.316 − 1) after the universal two-child policy if the PFR increases by 1.
With this coefficient, we can calculate the change in the gender wage gap by province or for the whole country. For example, the universal two-child policy expands the gender wage gap in Heilongjiang province (which has the lowest PFR) by 33.99%, while the outcome for Guizhou province (which has the highest PFR) is only 15.29%. For the whole country, the policy has expanded the gender wage gap by 18.45%.Footnote 4
DDD specification using the information of children number
Besides dividing treated and control provinces by PFR, we also base the policy-treatment definition on certain demographics. Akin to Yin et al (Reference Yin, Yao and Li2013), families that have already had two or more than two children before the policy would not be eligible to have more children, so we define the individuals with less than two children as a treatment group, otherwise as a control group:

where treat1 is a dummy variable of whether individual i has less than two children (if i has less than two children, treat1=1, otherwise treat1=0). Column 5 of Table 4 shows that the DDD estimator possesses a minus sign at the 10% significance level, which indicates the universal two-child policy expands the gender wage gap by 10.19% (e 0.097 − 1) in the population who are eligible to have more children.
Table 4. Heterogeneity analysis
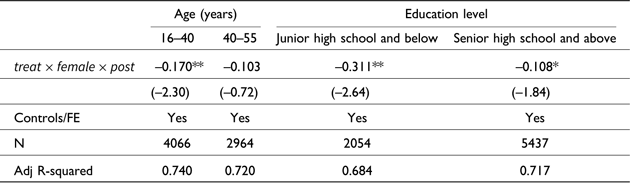
Note. Due to space limitation, only the interaction terms’ coefficients are reported, and the control variables are omitted but kept for request.t-statistics are in parentheses. FE includes the individual, year, and province fixed effects.
* Represent significance levels of 10% level.
** Represent significance levels of 5% level.
PSM-DDD test
To reduce the bias caused by group division, the individual characteristic variables, including age, work experience, the number of young children, education level, occupation, and industry, are used for propensity score matching (PSM). We employ Logit estimation and 1:4 nearest neighbour matching. There is no significant difference for most variables at the 10% level after PSM.Footnote 5
The last column in Table 3 is the quantitative outcome of the PSM-DDD estimation. It is consistent with our previous conclusion: the universal two-child policy expands the gender wage gap among people living in cities and towns. Such an outcome reaffirms the robustness of our empirical study.
How does the effect of the policy differ?
The previous analysis affirms the universal two-child policy’s influence on the gender wage gap, but we wondered whether the effect was different in each group of people. Therefore, further explorations were conducted based on age and education.
Based on age
Childbirth is closely related to age, so the fertility policy’s effect probably varies widely among different age groups. We divided the urban people into two groups based on age: young people whose age is not far from the fertility peak and the comparatively older ones above 40 years. Columns 1 and 2 of Table 4 show the regression results. The coefficient is −0.170 in the young group, which indicates that the gender wage gap expands by 18.53% (
${e^{0.170}} - 1$
) in the treated provinces versus control provinces among the population under 40 years. The outcome among the old population is not significant. Therefore, the young population is more affected by the universal two-child policy.
Based on education
Education level is of great importance to people’s employment and income. According to the highest academic qualifications, this section divides people into two groups: the low education group who graduated from junior high school or below and the high education group who graduated from senior high school or above. The results are reported in columns 3 and 4 of Table 4. The coefficient is –0.311 in the low-educated group, which indicates that the gender wage gap expands by 36.48% (
${e^{0.311}} - 1$
) in the treated provinces versus control provinces among the low-educated population. The outcome among the high-educated population is just 11.40% (
${e^{0.108}} - 1$
), which is much smaller. Therefore, the low-educated population are more affected by the universal two-child policy.
Further analysis: mechanism
Since the increases in the gender wage gap are clearly attributed to the universal two-child policy, is it possible that the policy has led to more severe gender discrimination against women? The Oaxaca–Blinder decomposition is the most commonly used method for decomposing the gender wage gap (Oaxaca Reference Oaxaca1973; Blinder Reference Blinder1973). Nevertheless, Cotton (Reference Cotton1988), Neumark (Reference Neumark1988), and Oaxaca and Ransom (Reference Oaxaca and Ransom1994) point out that the decomposition results are widely divergent due to different adoptions of the benchmark group in the Oaxaca–Blinder decomposition. We use the Oaxaca–Ransom model to avoid the ‘index benchmark problem’.
Table 5 shows the Oaxaca–Ransom results. The decomposition found that the unexplainable portion of the gender wage gap accounted for 79.37% (0.2675/0.3369) in 2014, while it climbed to 79.90% (0.2439/0.3053) in 2018. Therefore, after implementing the universal two-child policy, the evident rise of the unexplainable part of the gap indicates that the universal two-child policy probably induces more severe gender discrimination in China’s labour market.
Table 5. Oaxaca–Ransom decomposition

Conclusion
This paper examines the universal two-child policy’s effect on the gender wage gap and its internal mechanism in the urban labour force in China. The main findings are summarised below.
First, the universal two-child policy has significantly expanded the gender wage gap. Using the CFPS 2014 and 2018 survey, we find the policy leading to a significant 12.86% increase in China’s urban gender wage gap in the low-PFR provinces.
Second, the universal two-child policy’s effect on the gender wage gap in different groups varies. The policy has significantly expanded the gender wage gap among younger or poorly educated people. However, it does not significantly influence people above 40 and only slightly influences high-educated people.
Third, we provide empirical evidence to show that gender discrimination in the labour market was more severe after the implementation of the policy. Employers generally expect higher costs if they hire women; such costs can include maternity insurance fees, allowances, pregnancy-test leave, maternity leave, breastfeeding leave, finding a replacement during occupational interruption, and work decrement due to childbearing and childcare. Employers thus strengthen their preference for male workers, which further intensifies gender discrimination and expands the gender wage gap.
In the labour market, employers seeking profit maximisation can see they must endure higher costs if they hire women than comparable men. In households, the responsibilities of raising children and domestic labour are mainly shouldered by women, which incurs great conflict between the family and women’s career development. The universal two-child policy has simultaneously intensified both contradictions and burdened women with the majority cost of rearing a second child. The policy falls far short of expectations. Therefore, the government should take adequate measures to share the responsibility and cost of raising children with all of society, such as encouraging fathers to participate in parenting duty and housework, providing more affordable infant and childcare services, supplying employers with tax subsidies or financial incentives for hiring females, protecting women’s employment opportunities and wage equality, establishing a childbirth-friendly institutional and cultural environment, and paying particular attention to the disadvantaged females.
From a global perspective, birth rates in many countries have fallen below the replacement rate of 2.1. Many governments have successfully introduced family, economic, and social policies to cope with the sluggish fertility and ageing society. According to the conclusions of this article, a more relaxed fertility policy or onefold fertility incentives would expand the gender wage gap and induce more severe gender discrimination in the labour market. Therefore, governments should explore lowering gender discrimination and effectively guaranteeing women’s equal rights in the labour market. These measures would greatly promote women’s fertility willingness and behaviours.
Funding statement
Our work was supposed by the National Natural Science Foundation of China [Grant number: 72074128].
Competing interests
The authors have no relevant financial or non-financial interests to disclose.
Qian Huang is a distinguished professor of economics at the Institute of Population and Development in Nankai University in China. His research interests and publications are in the areas of labour economics, population economics, and health economics.
Xiaofei Jin is a Postdoctoral Researcher of Higher Education Press in China, holding a PhD of economics. Her research focuses on labour economics and education publishing.
Ziang Fan is a PhD candidate of economics at the Institute of Population and Development in Nankai University in China. His research focus on labour force and employment, health economics and public policy.
Appendix
Table A1. Summary statistics for Chinese adults in the 2012–2018 CFPS samples
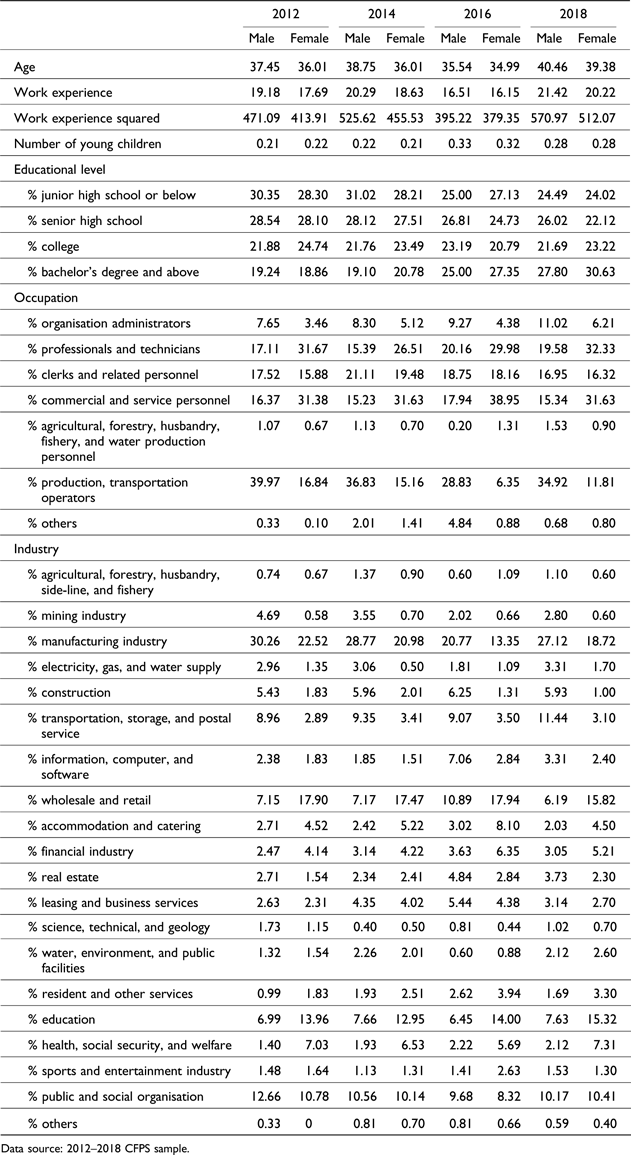
Data source: 2012–2018 CFPS sample.
Table A2. Variable descriptions
