According to the latest adult psychiatry morbidity survey, approximately one in six adults in England meet the criteria for a mental disorder.Reference McManus, Bebbington, Jenkins and Brugha1 Yet in many cases we are still relying on these individuals to advocate for their own diagnosis and treatment, despite continuing stigma and overstretched resources. Evidently, the problems are multifaceted, and artificial intelligence (AI) is not a magic bullet. However, in this analysis I will argue that AI is a tool that can be leveraged to alleviate some of the ever-increasing burden on mental health services in the future.
Although there is no single accepted definition, Ida Arlene Joiner describes AI as ‘the theory and development of computer systems that are able to perform tasks normally requiring human intelligence, such as visual perception, speech recognition, decision-making, and translation between languages’.Reference Joiner2 As ‘human intelligence’ is subjective, the resulting field of AI is dynamic and diverse. Machine learning is a more specific term, referring to a subset of AI techniques that allow machines to learn from data automatically from past data without explicit programming. Deep learning is a subset of machine learning that uses a particular modelling technique called neural networks to learn from data. This will be discussed in more detail in the deep learning section.
In this paper I explore the role of AI in psychiatry by following the patient journey though diagnosis, monitoring and treatment. I finish by drawing attention to ethical issues and the current limitations of the technology that may act as barriers to their adoption.
What are the strengths of AI?
Human intelligence relies on heuristics to decipher causal relationships in our environment. However, we are not so good at spotting complex patterns in large data-sets, because our heuristics can lead us to oversimplified conclusions. This is where deep learning is at its most powerful. I can summarise the key strengths of AI as the following.
(a) Scalability to large data-sets: once a workflow is established, the incremental cost of adding more data is small, making the system cheaply scalable and easy to keep up to date as new data becomes available.
(b) Capacity to supersede human performance on specialised tasks: AI is well suited to solving highly specific tasks with quantifiable performance metrics. It exploits reliable patterns in patient data without getting fatigued or bored.
(c) Automation: AI can have a particular impact in situations where there is significant stress on hospital resources. It has the potential to increase the capacity of a service by easing the workload demands from highly trained specialists, translating to real differences in patient outcomes. For example, this could be translating speech into written text for documenting in medical notes after a consultation.
Deep learning
Deep learning in particular has shown incredible promise over the past decade; for example, in identifying objects in images, transcribing speech into text, matching news items or products with users’ interests, and selecting relevant search results.Reference LeCun, Bengio and Hinton3 A recent review of AI in health also found that deep learning tools obtained more positive results than traditional machine learning.Reference Zhou, Chen, Cao and Peng4 The original inspiration for the technology came from neuroscience.Reference Hassabis, Kumaran, Summerfield and Botvinick5 In the 1980s, early AI researchers knew that the brain comprised networks of neurons propagating signals encoded as action potentials. As these biological neural networks were known to underpin human intelligence, they hypothesised that they could induce intelligent reasoning if they replicated the basic structure of these networks.
Therefore, the discovery of biological neural networks laid the foundations for deep learning. The core concept that neurons are connected together in layers is preserved (Fig. 1) with some modifications to improve computational efficiency or performance. Just like in the brain, the architecture and connectivity of the neurons can be specifically designed to extract particular information from a given input. A good example of this in the brain is the simple cells in the primary visual cortex that are specialised for extracting lines and edges from images.
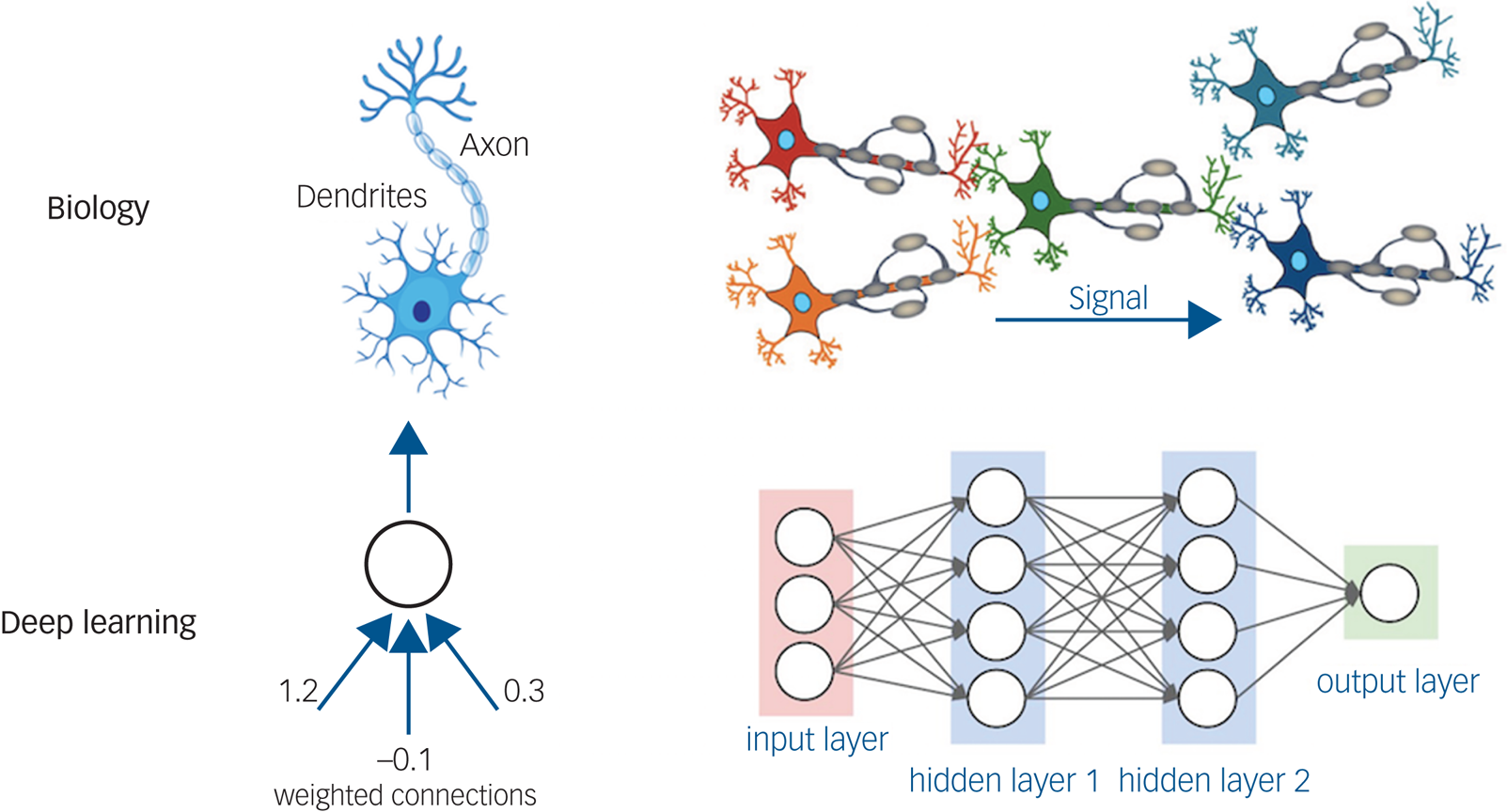
Fig. 1 A comparison of biological and artificial neural networks. The basic structure consists of layers of neurons (shown as circles) that are connected together by axons (represented by arrows with associated ‘weights’ which indicate the strength of the connection between those neurons). Reproduced with permission from Laura Dubreuil Vall.
Just like learning in the brain, deep learning works by trial and error. If we imagine that we want to classify patients who have psychosis based on their eye movements then our training data will need examples from both patients with psychosis and healthy controls. Before training, the AI will not know how to distinguish the patients. However, each time the AI gets the classification wrong, the ‘weights’ between the artificial neurons will be updated such that the network is less likely to return the same mistake again. The training continues until the algorithm converges.
Applications in psychiatry
In this section, I highlight some recent and upcoming AI developments in three major categories of psychiatry: diagnosis, monitoring and treatment.
Diagnostic tools
Computers have been suggested for use as medical diagnostic aids as early as the 1950s.Reference Ledley and Lusted6 Seventy years later, we are starting to see increasing numbers of applications in general medicine making it to clinic, especially in medical imaging.Reference Nagendran, Chen, Lovejoy, Gorden, Komorowski and Harvey7 However, developments in psychiatry are at an earlier stage, in large part because the data is more subjective and difficult to use, relying heavily on mental state examination findings and retrospective accounts of symptoms. This data can be complicated by the lack of insight and cognitive and memory deficits that make accurate recall of symptoms challenging.
Mobile technologies offer the opportunity for the patient to capture numerical and mood data at home on a regular basis. However, as of yet, none of the most common clinical questionnaires have been adapted and validated for home use on a large scale.Reference Roberts, Chan and Torous8 Furthermore, early evidence suggests that simple translations of scores such as the nine-item Patient Health Questionnaire onto smartphones do not correlate well with results obtained in the clinic.Reference Torous, Staples, Shanahan, Lin, Peck and Keshavan9 We need specialised and large-scale research efforts before we can realise the potential of smartphone data.
Early research has also highlighted use-cases of AI in hospital settings, for example anxiety and depressionReference Priya, Garg and Tigga10 and suicide prediction.Reference Jiang, Rosellini, Horváth-Puhó, Shiner, Street and Lash11 A comprehensive review of diagnosis tools in mental health can be found in Graham et al.Reference Graham, Depp, Lee, Nebeker, Tu and Kim12 However, it is important to note that many of these models never make it to clinic because they are hampered by limitations in the research – especially validation against the performance of existing tools and also commercial licensing issues.Reference Carroll13 Of the models that do make it, a recent systematic review by Zhou et alReference Zhou, Chen, Cao and Peng4 found that most have limited impact despite good performance. In order to add value in the short term we need to invest in other areas as well; I focus on these opportunities next.
Monitoring
AI has exciting potential in the monitoring of patients with known diagnoses who are already engaged with mental health services. For example, physiological data (such as heart rate variability, skin conductance, sleep quality) can be used in patients with schizophrenia to stratify cardio-arrhythmic risk. Using Holter electrocardiogram (ECG) recordings, Bär et alReference Bär, Boettger, Koschke, Schulz, Chokka and Yeragani14 showed that there is reduced heart rate variability in patients with schizophrenia. These data might enable the early detection of elevated cardiovascular risk, leading to a more proactive approach to reducing mortality. Until recently, this has not been feasible because of the practical constraints of recording this type of data at home. However, with the widespread adoption of fitness trackers it may soon become reality. In fact, early research has shown that people with serious mental health problems are adherent to, and even enjoy using fitness trackers to monitor their health.Reference Naslund, Aschbrenner, Barre and Bartels15
In addition, we may be able to use behavioural data to identify patients with mental health crises before they present to the hospital. This can either be from electronic health records,Reference Garriga, Mas, Abraha, Nolan, Harrison and Tadros16 or from smartphones that are already tracking our location and social activities. For example, we can imagine that a patient with bipolar disorder who enters a manic phase may demonstrate atypical GPS location data or increased social activity at night.
The use of voice data is another area of high interest. We know that pitch, tempo and volume of voice are biomarkers of many psychiatric illnesses such as depression and anxiety.Reference Cummins, Scherer, Krajewski, Schnieder, Epps and Quatieri17 In addition, automatic transcription and analysis of patient consultations could alleviate some of the administrative burden on services.
Treatment
For many years, psychiatrists have attempted to understand individualised patient responses to medications and psychotherapy when personalising treatment choices. The role of scientific research was to confirm or refute specific hypotheses for groups of patients who share symptoms, and personalisation was left to the clinician. With the advent of deep learning, we have the opportunity to shift this paradigm towards predictive modelling for the individual.
Predicting treatment outcomes for psychiatric medications is the most active area of research, primarily because there are large volumes of data with clearly labelled outcomes. The most studied application is the use of antidepressants in the acute phase of depression. For example, Chekroud et alReference Chekroud, Zotti, Shehzad, Gueorguieva, Johnson and Trivedi18 identified 25 patient-reportable variables that were most predictive of treatment outcome and used these to train the model. The model was able to predict the response to two similar antidepressant regimens (escitalopram plus placebo and escitalopram plus bupropion, each with an accuracy of around 60%). This is encouraging, but there is more potential.
Finally, any discussion of AI intervention in psychiatry would be incomplete without a mention of online psychotherapy. Internet-based cognitive–behavioural therapy (CBT) may be particularly amenable to machine learning contributions because of the high numbers of patients. Initially this will take the form of guided treatments, with alerts and feedback to both patients and therapists. Whether it will be possible to deliver fully autonomous and effective treatment via AI agents remains to be seen. However, small-scale studies using conversational chatbots have already been shown to reduce anxiety in college students.Reference Fulmer, Joerin, Gentile, Lakerink and Rauws19 Meanwhile outside of medicine, models such as OpenAI's InstructGPT,Reference Ouyang, Mishkin, Wu, Hilton, Askell and Christiano20 can generate human-like text and Tacotron 2Reference Shen, Pang, Weiss, Schuster, Jaitly and Yang21 is capable of producing speech from text that is indistinguishable from humans.
Ethical issues
From the development of machine learning tools to their deployment, we can identify a number of ethical challenges that could be critical to the success of AI applications in psychiatry. Some of these are ubiquitous within healthcare, whereas others are specific to mental health. I start with the general challenges.
(a) AI systems have no sense of intrinsic morality; therefore, they require specific instructions on how to behave in difficult scenarios. For example, when we program self-driving cars, we need to explicitly instruct the car not to hit pedestrians on the way to its destination. Even with basic safety principles we will still encounter challenging dilemmas akin to the trolley problem.Reference Thomson22 Although these issues may be surmountable for self-driving cars, the complexity and legal issues presented in medicine preclude us from running these algorithms autonomously in all but the simplest clinical scenarios in the foreseeable future.
(b) Questions of responsibility: when AI makes a decision, whose faults are the consequences? Is it the authorising psychiatrist, the patient, the AI algorithm, the developers, the National Health Service trust or nobody? This remains an open question.
(c) Interpretability: we are often given very little information about why the algorithm has come to a certain decision.Reference Linardatos, Papastefanopoulos and Kotsiantis23 Therefore, how can we trust that the algorithm is reliable without explanation, and even if we could, can it be used if the psychiatrist does not agree with the decision? Is it preferable for the psychiatrist to trust AI which is known to be more accurate, or whether they should choose a treatment for which that they can defend the rationale?
Further to these, there are ethical dilemmas that are more specific to psychiatry. For example, should AI have a role in Mental Health Act assessment decisions? I cannot hope to be exhaustive in this discussion, but I present some general themes.
(a) Capacity and consent: mental illness can impair capacity and thus the ability to consent to both the use of AI in their treatment and the sharing of their data with third parties.
(b) Privacy: mental health data can be intimate and personal by nature, making it harder to anonymise. The sensitivity of patient data might even increase as a result of developments in AI technology if GPS or social media data were to be used. The consequences of any data breeches are likely to be severe.
(c) Bias and structural injustices: this is already a pervasive issue in medicine, but it can be especially difficult to systematically detect in text-based data, which is often key in mental health records. There is an excellent discussion written by Straw & Callison-Burch on this issue,Reference Straw and Callison-Burch24 where they found several questionable associations by analysing vector similarity, such as, ‘White is to “depression”, as black is to “undergone_electroshock_therapy”’.
Limitations
As well as ethical issues we also have limitations in the technology. In psychiatry, data is arguably the most obvious constraint. This is because we do not have the luxury of rich numerical data-sets such as those available in intensive care units. Currently, the main types of data are demographics, diagnoses, medications, procedures, self-reported questionnaires and text from clinical encounters. There is significant potential for this to expand in the future.
The next problem relates to the generalisability of the models. Empirically we know that a model trained on one set of training data can make catastrophic errors on a different data-set, even if it is very similar to the first.Reference Nagarajan, Andreassen and Neyshabur25 As it is not practical to train a new model for every scenario, we need further work in this area.
The performance of deep learning models is also influenced by several stochastic processes during training, for example the order in which the training data is presented. This means that it is impossible to get solid guarantees for performance. In cases where the problems are heavily deterministic, for example, if they rely on basic physical laws, it is usually more reliable to simulate the system rather than use deep learning.
Finally, we need to consider tasks where the outcome cannot be mathematically defined, for example, ‘deliver CBT’. It is more difficult to objectively measure to what extent the agent has performed correctly. For these tasks, we need a human in the loop to reinforce good behaviour and penalise unsuccessful behaviour. This slows down the learning process because it can no longer operate automatically.
Conclusion
There is great potential for AI-assisted technologies to enhance diagnosis, monitoring and treatment in psychiatry. I have reviewed several early technologies that have demonstrated that it is possible to predict outcomes and personalise treatment using deep learning. The vast potential has only just begun to be explored and realised. However, we must approach these technologies from a systematic research perspective and create a framework to assess the risk of any unintended negative consequences of their implementation. Now is the time to invest in AI research so that we can uncover new cost-effective and ethical strategies to reduce the burdens associated with mental health conditions around the world.
Funding
This study received no specific grant from any funding agency, commercial or not-for-profit sectors.
Declaration of interest
No conflicts of interest to disclose.
eLetters
No eLetters have been published for this article.