Intellectual investment traits are characterized as “stable individual differences in the tendency to seek out, engage in, enjoy, and continuously pursue opportunities for effortful cognitive activity” (von Stumm, Chamorro-Premuzic, & Ackerman, Reference von Stumm, Chamorro-Premuzic and Ackerman2011, p. 225) and have been proposed as important factors influencing intelligence and academic achievement (e.g., von Stumm & Ackerman, Reference von Stumm and Ackerman2013). Previous studies found investment traits to be associated with academic performance in school (e.g., Luong et al., Reference Luong, Strobel, Wollschläger, Greiff, Vainikainen and Preckel2017), in college entry tests (e.g., Cacioppo & Petty, Reference Cacioppo and Petty1982), and in university (e.g., von Stumm & Ackerman, Reference von Stumm and Ackerman2013). Little, however, is known about the factors that affect individual differences in intellectual investment traits. In our view, such factors are likely to be both dispositional and environmental in nature. Hence, one must consider both, genes and environmental variables.
Such an approach can be seen as prototypical for personality neuroscience: an integrative attempt to bring together biological and environmental sources of variation in order to explain rather than merely describe individual differences. This can to some extent be viewed as a causal approach, as certain biological variables and associated mechanisms—here: gene-by-environment interactions—may readily qualify as causal antecedents. Dealing with environmental variables may, depending on the research design, be not as straightforward in establishing causal links than a purely genetic approach (that itself may not be able to provide direct causal relations as genetic variations under investigation may simply be in linkage disequilibrium with causal variants). Yet, in our view, a proper neuroscientific approach to identifying explanations for individual differences has to consider that the explanans may not only be a biopsychological variable—interactions with the environment will most likely occur, in one way or another (e.g., Reiss, Leve, & Neiderhiser, Reference Reiss, Leve and Neiderhiser2013).
1. Starting point of the present work
In the present contribution, we examine the role of both genetic variation and environmental influences in the modulation of intellectual investment traits. Indeed, a recent study provided first evidence of such effects. Building on extensive work on the biological foundations of personality (DeYoung, Reference DeYoung2010; DeYoung, Peterson, & Higgins, Reference DeYoung, Peterson and Higgins2005), DeYoung et al. (Reference DeYoung, Cicchetti, Rogosch, Gray, Eastman and Grigorenko2011) investigated the role of two genetic variants that presumably impact prefrontal dopamine (DA) function—COMT Val158Met and DRD4 exon III—in the modulation of the investment trait Openness/Intellect (DeYoung et al., Reference DeYoung, Cicchetti, Rogosch, Gray, Eastman and Grigorenko2011). They observed main effects for both polymorphisms in 608 children, with carriers of the COMT Met/Met genotype exhibiting higher scores in Openness/Intellect, and carriers of the DRD4 exon III 7-repeat allele exhibiting lower scores. In 214 adults, no main effects, but a gene–gene interaction emerged, with lower scores in Openness/Intellect in DRD4 exon III 7-repeat allele carriers being evident only for COMT heterozygotes.
To follow-up on these intriguing results, we (1) aimed at a conceptual replication of these findings and (2) extended the examination to Need for Cognition (NFC), an investment trait conceptually closely related to Openness/Intellect (e.g., Fleischhauer et al., Reference Fleischhauer, Enge, Brocke, Ullrich, Strobel and Strobel2010). To this end, we used an existing data set that had been collected from the general population of Dresden, Germany, during a research project on the neurogenetics of personality, results of which have in part already be reported (Anacker, Enge, Reif, Lesch, & Strobel, Reference Anacker, Enge, Reif, Lesch and Strobel2013; Strobel, Anacker, & Strobel, Reference Strobel, Anacker and Strobel2017). The study comprised several assessments that involved: (a) blood sampling for later genetic analyses and the assessment of personality traits; (b) self-reports on negative life events (NLE) in a smaller subsample; and (3) self-reports on positive life events (PLE) and NLE assessed several months later in an again smaller subsample. The questionnaires employed included the Revised NEO Personality Inventory (NEO-PI-R; Costa & McCrae, Reference Costa and McCrae1992). While different from the assessment methods used by DeYoung et al. (Reference DeYoung, Cicchetti, Rogosch, Gray, Eastman and Grigorenko2011), it enabled us to focus on Openness to Experience or more specifically on its facet Openness to Ideas that most strongly taps into the above definition of intellectual investment traits. The two genotyped DA-relevant polymorphisms were those that DeYoung et al. (Reference DeYoung, Cicchetti, Rogosch, Gray, Eastman and Grigorenko2011) had examined.
The also employed NFC scale measures an individual’s tendency to engage in and enjoy effortful cognitive endeavors (Cacioppo, Petty, Feinstein, & Jarvis, Reference Cacioppo, Petty, Feinstein and Jarvis1996). NFC is highly correlated with Openness to Ideas and, thus, they share a common core, as has also been pointed out in the comprehensive framework of intellectual investment traits proposed by Mussel (Reference Mussel2013). However, there are also differences in their predictive validity: Fleischhauer et al. (Reference Fleischhauer, Enge, Brocke, Ullrich, Strobel and Strobel2010) observed NFC to be a more valid predictor of activity- and goal-oriented traits, while Openness to Ideas was a more valid predictor of traits related to exploration and experience seeking.
2. Rationale of the study
While for Openness/Intellect, DeYoung and colleagues have provided evidence for a role of prefrontal DA function in the modulation of this intellectual investment trait (e.g., DeYoung, Reference DeYoung2010; DeYoung, Reference DeYoung2013), evidence on NFC’s underlying mechanisms is rather scarce. With regard to biological factors, it has been shown that NFC is moderately heritable (20–40%; Ksiazkiewicz, Ludeke, & Krueger, Reference Ksiazkiewicz, Ludeke and Krueger2016; Tucker-Drob, Briley, Engelhardt, Mann, & Harden, Reference Tucker-Drob, Briley, Engelhardt, Mann and Harden2016). With regard to cognitive mechanisms, NFC has been associated with behavioral and electrocortical indices of higher (especially early) attention allocation (Enge, Fleischhauer, Brocke, & Strobel, Reference Enge, Fleischhauer, Brocke and Strobel2008; Enge, Fleischhauer, Lesch, & Strobel, Reference Enge, Fleischhauer, Lesch and Strobel2011), lower effort discounting (Westbrook, Kester, & Braver, Reference Westbrook, Kester and Braver2013), and an electrocortical index of higher mental effort investment (Mussel, Ulrich, Allen, Osinsky, & Hewig, Reference Mussel, Ulrich, Allen, Osinsky and Hewig2016).
The associations of NFC with interest-, action-, and goal-oriented personality traits on the one hand (Fleischhauer et al., Reference Fleischhauer, Enge, Brocke, Ullrich, Strobel and Strobel2010) and with attention allocation and effort investment on the other also point to a role of DA-induced neuromodulation in individual differences in NFC. DA plays a key role in cognition and motivation, as midbrain DA-specific neurons project to both prefrontal and cingulate cortical areas critically involved in attention and executive control processes and to nucleus accumbens as a brain structure that integrates learned contingencies, contextual information, and the organisms’ current goal status in order to maximize reward (Depue & Collins, Reference Depue and Collins1999; Grace, Reference Grace2000). At the same time, DA also plays an activational role and is crucially involved in invigorating behavior and exerting effort (Cools, Nakamura, & Daw, Reference Cools, Nakamura and Daw2011; Niv, Daw, Joel, & Dayan, Reference Niv, Daw, Joel and Dayan2007) and, moreover, is likely to play a role in curiosity (Ikemoto & Panksepp, Reference Ikemoto and Panksepp1999). Taken together, the evidence on the functions of DA fits well with the assumption that it has a major modulatory role in resource investment such as the allocation of attentional resources subserving goal-directed action.
One approach to investigate the influence of neuromodulatory pathways on behavior is to examine the behavioral impact of genetic variation in these pathways. In the present context, the two genetic polymorphisms examined by DeYoung et al. (Reference DeYoung, Cicchetti, Rogosch, Gray, Eastman and Grigorenko2011) lie in especially promising candidate genes: the gene encoding the DA-degrading enzyme catechol-O-methyltransferase (COMT) and the gene encoding the DA receptor subtype D4 (DRD4), both of which playing a prominent role in prefrontal DA functioning (Bilder, Volavka, Lachman, & Grace, Reference Bilder, Volavka, Lachman and Grace2004; Frank & Fossella, Reference Frank and Fossella2011; Oak, Oldenhof, & Van Tol, Reference Oak, Oldenhof and Van Tol2000).
The COMT gene contains a well-studied single nucleotide polymorphism consisting of a guanine-to-adenine transition (rs4680) that results in a substitution of the amino acid valine (Val) to methionine (Met) at position 158 of the COMT enzyme (COMT Val158Met), with the two alleles being nearly equally frequent in European populations. The presence of the Met allele reduces enzyme activity, which results in lower DA degradation and, thus, in a higher level of extrasynaptic DA in carriers of the Met allele (Bilder et al., Reference Bilder, Volavka, Lachman and Grace2004). As COMT is the major terminating factor for DA action in prefrontal cortex due to the paucity of DA transporters in this brain region, the presence of the Met allele has been shown to result in better performance in working memory tasks and other executive control paradigms (Mier, Kirsch, & Meyer-Lindenberg, Reference Mier, Kirsch and Meyer-Lindenberg2010). Enhanced prefrontal cortex-dependent executive control may have some influence on the inclination to engage in controlled processing. Indeed, evidence suggests that individuals high in NFC are less likely to discount the effort required to engage in tasks that place higher demands on working memory capacity (Westbrook, Kester, & Braver, Reference Westbrook, Kester and Braver2013). Thus, the conclusion seems warranted that the presence of the Met allele may be associated with higher scores in intellectual investment traits.
The DRD4 gene features a variable number of tandem repeats polymorphism in its coding region (DRD4 exon III), where a sequence of 48 base pairs is repeated 2–11 times. The most frequent alleles in European populations are the 4-repeat allele (about 65%) and the 7-repeat allele (about 20%), with the latter resulting in lower receptor function (Oak, Oldenhof, & Van Tol, Reference Oak, Oldenhof and Van Tol2000). While meta-analytic evidence has cast some doubt on initial findings of the role of the DRD4 exon III 7-repeat allele in the modulation of impulsive personality traits (Kluger, Siegfried, & Ebstein, Reference Kluger, Siegfried and Ebstein2002; Munafo, Yalcin, Willis-Owen, & Flint, Reference Munafo, Yalcin, Willis-Owen and Flint2008), the 7-repeat allele has rather consistently been observed to be a risk factor for another impulsivity-related phenotype, attention-deficit hyperactivity disorder; (e.g., Nikolaidis & Gray, Reference Nikolaidis and Gray2010; Wu, Xiao, Sun, Zou, & Zhu, Reference Wu, Xiao, Sun, Zou and Zhu2012), although the results for adult attention-deficit hyperactivity disorder are less conclusive (e.g., Bonvicini, Faraone, & Scassellati, Reference Bonvicini, Faraone and Scassellati2016; Sanchez-Mora et al., Reference Sanchez-Mora, Ribases, Casas, Bayes, Bosch, Fernandez-Castillo and Cormand2011). Together, the available evidence on DRD4 exon III main effects suggests, albeit with some reservations, 7-repeat allele carriers to be less likely to engage in controlled processing.
Interestingly, the behavioral impact of the DRD4 exon III 7-repeat allele appears to be moderated by environmental influences: Multiple studies have reported that 7-repeat allele carriers particularly benefit from high parenting quality or secure attachment with respect to lower impulsivity and higher effortful control (e.g., Bakermans-Kranenburg & van Ijzendoorn, Reference Bakermans-Kranenburg and van Ijzendoorn2011; Sheese, Rothbart, Voelker, & Posner, Reference Sheese, Rothbart, Voelker and Posner2012; Sheese, Voelker, Rothbart, & Posner, Reference Sheese, Voelker, Rothbart and Posner2007). This underscores the notion that the 7-repeat allele is a plasticity factor that depending on environmental support impacts on behavioral tendencies with adaptive or maladaptive consequences (Belsky et al., Reference Belsky, Jonassaint, Pluess, Stanton, Brummett and Williams2009). It may also explain the inconclusive results regarding the DRD4 exon III so far and advocate the inclusion of environmental variables in association studies on the role of the DRD4 exon III in personality differences. In the current context, one would assume carriers of the 7-repeat allele to exhibit lower levels of intellectual investment, as both NFC and Openness to Ideas are only loosely if at all associated with impulsivity (e.g., Fleischhauer et al., Reference Fleischhauer, Enge, Brocke, Ullrich, Strobel and Strobel2010), and NFC is associated with enhanced goal-directed attention allocation (Enge et al., Reference Enge, Fleischhauer, Brocke and Strobel2008). Indeed, in a sample of N=117 composed of carriers of the DRD4 exon III 4/4 and 4/7 genotype, the latter showed significantly lower NFC scores (Fleischhauer, Enge, & Donsbach, Reference Fleischhauer, Enge and Donsbach2008), which to some extent mirrors the findings by DeYoung et al. (Reference DeYoung, Cicchetti, Rogosch, Gray, Eastman and Grigorenko2011). However, based on the reported interactive effects with environmental variables, carriers of the 7-repeat allele with positive or at least less adverse environmental experiences should rather show higher levels of intellectual investment.
3. General outline
Taken together, here we aimed at elucidating the role of genetic variation of DA system function in modulating individual differences in intellectual investment traits. Our study can be regarded as a conceptual replication of the study of DeYoung et al. (Reference DeYoung, Cicchetti, Rogosch, Gray, Eastman and Grigorenko2011) and an extension to NFC as a related intellectual investment trait. As outlined above and justified by the findings of study 1 in DeYoung et al. (Reference DeYoung, Cicchetti, Rogosch, Gray, Eastman and Grigorenko2011), we predicted the COMT Met Allele to be positively associated with NFC and Openness to Ideas, while for the DRD4 exon III 7-repeat allele, a negative association was assumed with regard to a possible main effect. With regard to possible gene–gene interactions, the results of study 2 of DeYoung et al. (Reference DeYoung, Cicchetti, Rogosch, Gray, Eastman and Grigorenko2011) argue for lower intellectual investment in carriers of the DRD4 exon III 7-repeat allele who are also COMT Val158Met heterozygotes. With regard to possible gene–environment interactions, we assumed that, based on the above-outlined literature, life events would interact especially with DRD4 exon III, with carriers of the 7-repeat allele exhibiting higher scores in NFC and Openness to Ideas in case of less adverse or even PLE.
However, given that candidate gene research has been the target of much criticism (see, e.g., Johnson et al., Reference Johnson, Border, Melroy-Greif, de Leeuw, Ehringer and Keller2017, for an overview and a recent example), we also aimed at comparing a standard approach to data analysis to a more straightforward approach: the determination of Bayes factors. Originally proposed by Jeffreys (Reference Jeffreys1935), Bayes factors provide a measure of the evidence for one model as compared with another, typically the alternative hypothesis of the presence of an effect as compared to the null hypothesis of no effect. On the basis of certain agreed-upon thresholds (see, e.g., Jeffreys, Reference Jeffreys1961; Wetzels et al., Reference Wetzels, Matzke, Lee, Rouder, Iverson and Wagenmakers2011), this evidence can then be classified regarding its strength. Importantly, in contrast to classical significance testing that only allows to reject the null hypothesis, the Bayesian approach allows to determine the strength of evidence in favor of the null hypothesis.
In order to structure analyses for the sake of readability, we will subdivide the present report into three parts: in Investigation 1, we report on the analyses regarding genetic influences on intellectual investment traits in a sample of N=524; in Investigation 2, the results on interactions of genetic variation with NLE in a subsample of n=455 will be reported; and finally, Investigation 3 is devoted to the results of the reexamination of part of that sample comprising n=252 of the original participants for whom we analyzed interactions of the genetic variables with PLE and NLE.
4. Investigation 1: Genetic influences on investment traits
4.1. Method
4.1.1. Sample
The sample consisted of N=524 participants of middle European ancestry (48% men, age mean±SD: 36.4±11.8 years, age range: 18–61 years) who had complete data for DRD4 exon III and COMT Val158Met genotypes. Fifty-nine percent of the participants held a university entrance diploma. Among a subsample of 422 participants, who had been assessed using the MINI International Neuropsychiatric Interview (Ackenheil, Stotz-Ingenlath, Dietz-Bauer, & Vossen, Reference Ackenheil, Stotz-Ingenlath, Dietz-Bauer and Vossen1999; Sheehan et al., Reference Sheehan, Lecrubier, Sheehan, Amorim, Janavs, Weiller and Dunbar1998), 5% of the participants reported past diagnoses of psychiatric disorders. They were not excluded, but the potential impact of past psychiatric diagnoses on the results outlined below was examinedFootnote 1 .
4.1.2. Procedure
A random sample of residents of the city of Dresden in the age of 18–60 years was drawn from the local residents’ registration office and was invited to take part in our study. Interested individuals were screened via telephone interviews for the relevant criteria including an age range of 18–60 years, middle European ancestry, not taking medication likely to impact on the central nervous system, modest consumption of legal or illegal drugs, and not having received psychotherapy or having been diagnosed for relevant neuropsychiatric conditions within the past three years. After inclusion, they were scheduled for two sessions of about 2 hr each. At the start of the first session, participants were once more introduced into the aims and procedures of the study and gave written informed consent. Afterwards, they filled in several personality questionnaires, of which those related to broad personality traits and to investment traits were used in the present study (see the Measures section), while other questionnaires related specifically to anxiety and depression were not used here and will be described elsewhere. Finally, 9 ml blood samples were taken by a nurse, and the second session was scheduled (see the Investigation 2: The role of NLE section). All procedures reported here and in the following complied with the ethical standards of the relevant national and institutional committees on human experimentation and with the Helsinki Declaration of 1975, as revised in 2008, and were approved by the ethics committee of the Deutsche Gesellschaft für Psychologie (reference number: ASKPLBB21092007DGPS).
4.1.3. Measures
NFC was assessed with the German 16-item short version of the NFC scale (Bless, Wänke, Bohner, Fellhauer, & Schwarz, Reference Bless, Wänke, Bohner, Fellhauer and Schwarz1994). Responses to each item were recorded on a 7-point Likert scale. The scale shows comparably high internal consistency (Cronbach’s α>.80; Bless et al., Reference Bless, Wänke, Bohner, Fellhauer and Schwarz1994; Fleischhauer et al., Reference Fleischhauer, Enge, Brocke, Ullrich, Strobel and Strobel2010).
A German adaptation of the NEO-PI-R (Ostendorf & Angleitner, Reference Ostendorf and Angleitner2004) was used to assess Neuroticism, Extraversion, Openness to Experiences, Agreeableness, and Conscientiousness of the Five-Factor-Model of Personality (Costa & McCrae, Reference Costa and McCrae1992). Each factor comprises six facets, each consisting of eight 5-point Likert scale items resulting in 240 items. Internal consistencies for the German NEO-PI-R are high (Cronbach’s α from .87 to .92; Ostendorf & Angleitner, Reference Ostendorf and Angleitner2004).
4.1.4. Genotyping
DNA was extracted from whole blood and genotypes were determined as described earlier for COMT Val158Met (Armbruster et al., Reference Armbruster, Mueller, Strobel, Lesch, Kirschbaum and Brocke2011) and DRD4 exon III (Ebstein et al., Reference Ebstein, Novick, Umansky, Priel, Osher, Blaine and Belmaker1996). DRD4 exon III genotypes slightly deviated from Hardy–Weinberg equilibrium (HWE), χ 2=16.51, df=10, p=.086, mainly due to one individual with the rare 3/6 genotype, exclusion of whom resulting in HWE, χ 2=11.18, df=9, p=.264. As in DeYoung et al. (Reference DeYoung, Cicchetti, Rogosch, Gray, Eastman and Grigorenko2011), we compared the absence versus presence of the 7-repeat allele. COMT Val158Met genotypes were in HWE in the total sample as well as in the DRD4 exon III genotype subgroups (see Table 1).
Table 1 COMT Val158Met genotypes stratified by DRD4 exon III genotype groups
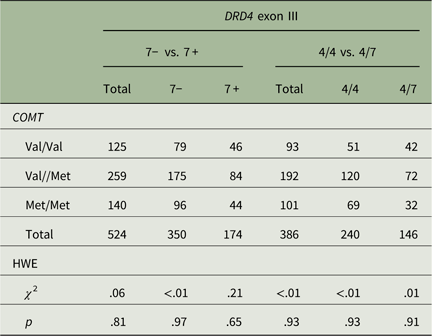
Notes: 7− versus 7+ =7-repeat allele absent versus present; 4/4 versus 4/7=4/4 versus 4/7 genotype; Val=valine; Met=methionine; HWE=Hardy–Weinberg equilibrium; χ 2=χ 2 test statistic at 1 df; p=level of significance.
4.1.5. Statistical analyses
Analyses employed RStudio (RStudio Team, 2015) with R 3.3.1 (R Core Team, 2016) and the packages psych (Revelle, Reference Revelle2016), car (Fox & Weisberg, Reference Fox and Weisberg2011), phia (De Rosario-Martinez, Reference De Rosario-Martinez2015), and BayesFactor (Morey & Rouder, Reference Morey and Rouder2015).
The main analyses were analyses of variance (ANOVA) with the two factors COMT (Val/Val vs. Val/Met vs. Met/Met) and DRD4 exon III (7-repeat absent vs. present), and either the Openness to Ideas or the NFC score as dependent variable. As these scores were not normally distributed, Shapiro–Wilk (S–W) test, both p<.003, we opted for normalizing the Openness to Ideas and NFC scores using Blom’s (Reference Blom1958) formula. The normalized scores were normally distributed, all p≥.24, and were used for all further analyses. Moreover, we mean-centered the variables for better interpretation and visualization. To exclude that possible genetic effects were simply due to the action of covariates likely to be implicated in intellectual investment, the results were further scrutinized via a model with a priori covariates. These were age, sex, and educational level, operationalized as holding or not holding a university entrance diploma. Regression analyses with these predictors suggested an influence of age on NFC, B=0.20, p=.001, lower scores for female participants for both NFC, B=−6.01, and Openness to Ideas, B=−2.17, both p<.001, and higher scores for educational level in both NFC, B=8.93, and Openness to Ideas, B=2.68, both p<.001. For no covariate, genotype effects were observed, χ 2 tests, all p≥.16.
As we had only one general assumption that the two dopaminergic gene variants would impact on intellectual investment traits, but performed six tests, that is, two main effects and one interaction for both NFC and Openness to Ideas, the level of significance was Bonferroni-adjusted to α′=.05/6=.008 for all ANOVAs. Our sample size, together with an α of .008 and a power of .80, enabled us to detect effects explaining about 2% of the variance in the dependent variable, an effect size well within the scope of standard candidate gene association studies (Lesch et al., Reference Lesch, Bengel, Heils, Sabol, Greenberg, Petri and Murphy1996).
Furthermore, we scrutinized possible results via the determination of Bayes factors using the BayesFactor package. Specifically, we used the function generalTestBF(), a multipurpose function that can handle interactions of both categorical and continuous variables (relevant for Investigations 2 and 3) and used the default Jeffreys–Zellner–Siow prior (Rouder, Speckman, Sun, Morey, & Iverson, Reference Rouder, Speckman, Sun, Morey and Iverson2009) and 50,000 random samples to compute Bayes factors. Bayes factors indicate the relative evidence for either one of the null or the alternative hypothesis, with the commonly used BF10 providing information of the probability of the data under the specified model as compared to the null model. BF10 of less than 1 indicate evidence in favor of the null hypothesis, and values greater than 1 indicate evidence in favor of the alternative hypothesis. According to, for example, Wetzels et al. (Reference Wetzels, Matzke, Lee, Rouder, Iverson and Wagenmakers2011), a BF10 of less than 1/3 or greater than 3 is considered as moderate evidence, of less than 1/10 or greater than 10 as strong evidence, of less than 1/30 or greater than 30 as very strong evidence and of less than 1/100 or greater than 100 as decisive evidence for either the null or the alternative hypothesis. As an example, a BF10 of 5 would indicate that the data are five times more probable under the specified model than under the null model, which would according to the above categorization be interpreted as moderate evidence for the specified model. Conversely, a BF10 of 0.05 would indicate that the data are 20 times more probable under the null than under the specified model, which would be interpreted as strong evidence for the null model.
4.2. Results
4.2.1. Influence of COMT Val158Met and DRD4 exon III on Openness to Ideas
There were no significant gene main effects and no interaction, all p≥.188, all
$$\eta _{p}^{2} \,\lt\,.01$$
(Figure 1B). This was also the case when entering age, sex, and university entrance diploma as covariates into the model (all p≥.160, all
$$\eta _{p}^{2} \,\lt\,.01$$
). We also examined whether there would be different results when we used the broader construct of Openness/Intellect as examined by DeYoung et al. (Reference DeYoung, Cicchetti, Rogosch, Gray, Eastman and Grigorenko2011). This was not the case, all p≥.123,
$$\eta _{p}^{2} \leq .01$$
. Thus, we could not obtain evidence in favor of the results obtained by DeYoung et al. (Reference DeYoung, Cicchetti, Rogosch, Gray, Eastman and Grigorenko2011).
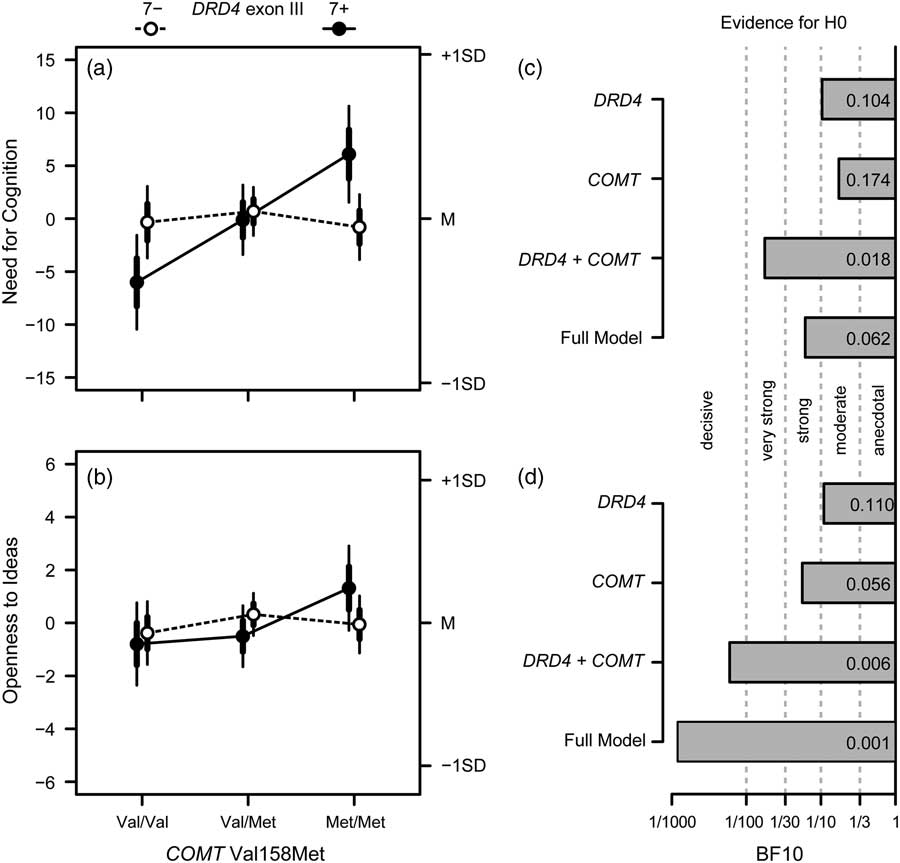
Figure 1 Overview on the results of Investigation 1. (a) Effects of COMT Val158Met and DRD4 exon III on Need for Cognition: depicted are the means for non-carriers (7−; open circles, dashed lines) and carriers (7+; filled circles, solid lines) of the DRD4 exon III 7-repeat allele together with their standard errors (thicker error bars) and 95% confidence intervals (CI; thinner error bars); cell sizes for COMT genotypes (Val/Val, Val/Met, and Met/Met) are 79, 175, and 96 for 7- carriers; and 46, 84, and 44 for 7+carriers. (c) Bayes factors for the alternative hypothesis of the validity of the respective effects drawn at the y-axis versus the null model (i.e., no effect); verbal labels denote the magnitude of evidence in favor of the H0; (b and d). Same as (a) and (c) for Openness to Ideas.
4.2.2. Influence of COMT Val158Met and DRD4 exon III on NFC
The DRD4 exon III polymorphism had no significant impact on NFC scores, F(1,5 18)=0.01, p=.927,
$$\eta _{p}^{2} \,\lt\,.01$$
, but there was a significant COMT Val158Met main effect, F(2, 518)=4.30, p=.014,
$$\eta _{p}^{2} {\equals}.02$$
, that was obviously due to a significant DRD4 exon III×COMT Val158Met interaction effect, F(2, 518)=5.08, p=.007,
$$\eta _{p}^{2} {\equals}.02$$
: while in the absence of the DRD4 exon III 7-repeat allele, the NFC scores of the different COMT genotype groups did not differ much from each other, carriers of the DRD4 exon III 7-repeat allele showed a pronounced effect, with COMT Val/Val genotype carriers exhibiting the lowest NFC scores and Met/Met genotype carriers exhibiting the highest NFC scores (Figure 1A). Considering the Bonferroni-adjusted level of significance of .008, the DRD4×COMT interaction was significant. This also held when considering age, sex, and educational level as covariates, p<.007, all
$$\eta _{p}^{2} {\equals}.02$$
. Thus, the COMT×DRD4 interaction was rather specific for NFC. However, their direction was different from expectations based on DeYoung et al. (Reference DeYoung, Cicchetti, Rogosch, Gray, Eastman and Grigorenko2011).
4.2.3. Bayes factors
For both Openness to Ideas and NFC, the Bayes factors of all model terms were smaller than 1 (see Figures 1C and D). Specifically, there was moderate to strong evidence for a model without gene main effects, all BF10≤0.17. With regard to the interaction, there was strong evidence for the null model with regard to NFC, BF10=0.06, and decisive evidence for the null model for Openness to Ideas, BF10 COMT =0.001. Repeating the analyses for the broader construct Openness to Experience, the results remained essentially unchanged, all BF10≤0.12. Thus, the Bayesian approach lends support for the assumption of no role of DRD4 exon III and COMT Val158Met in the modulation of cognitive investment traits—with one caveat: if we used the sample of the DRD4 7-repeat allele carriers only, the Bayes factor pointed to strong evidence of the COMT main effect on NFC in this subgroup, BF10 COMT =21.88.
4.3. Discussion
Taken together, with regard to the role of genetic variation in dopaminergic neurotransmission in the modulation of investment traits, a standard approach suggested increasing NFC scores with increasing number of COMT Met alleles in carriers of the DRD4 exon III 7-repeat allele. As such, while not exactly corroborating the findings of DeYoung et al. (Reference DeYoung, Cicchetti, Rogosch, Gray, Eastman and Grigorenko2011), the ANOVA results coincide with this earlier report in suggesting that prefrontal dopaminergic function has a modulatory role in individual variation in intellectual investment traits. However, using Bayesian analysis, there was moderate to strong evidence for the hypothesis of no gene effects on NFC and moderate to decisive evidence for the null hypothesis for Openness to Ideas or the broader construct. Thus, based on this more straightforward approach of hypothesis testing, the results do not support the assumption of a role of these two genetic variants in the modulation of investment traits. Moreover—and this makes the Bayesian approach appealing from an inferential viewpoint—they provide support for the null hypothesis of no interaction. However, when analyzing the COMT effect separately for DRD4 7-repeat allele carriers, we found strong evidence for a COMT main effect, which at first glance may lead to a more positive evaluation of the gene effects. Yet, as this analysis was not planned at the outset, we refrain from venturing an interpretation of this effect at this stage and will come back to this issue in the General Discussion section. Despite this sobering conclusion regarding genetic main effects, we next examined potential gene–environment interactions.
5. Investigation 2: The role of NLE
5.1. Method
5.1.1. Sample
The sample comprised a subset of participants of Investigation 1 who had complete data on self-reported NLE and consisted of n=455 participants (47% men, age mean±SD: 36.7±11.8 years, age range: 18–60 years). The composition of the sample was nearly identical to that of the total sample of Investigation 1 (61% university entrance diploma, 4% past psychiatric diagnoses). Again, the COMT Val158Met genotypes were in HWE, χ 2=0.37, df=1, p=.545, as were the DRD4 exon III genotypes when excluding the individual with the rare 3/6 genotype, χ 2=13.63, df=9, p=.136.
5.1.2. Procedure
NLE were assessed using a 28-item self-report questionnaire (Canli et al., Reference Canli, Qiu, Omura, Congdon, Haas, Amin and Lesch2006) adapted from the life history calendar (Caspi et al., Reference Caspi, Moffitt, Thornton, Freedman, Amell, Harrington and Silva1996). Participants indicated whether and how often they had experienced adverse life events related to, among others, family and relationships, work, health, financial, and legal problems. For further analyses, we calculated the number of different NLE categories (range in the present sample: 0–24, median=7) and the total number of NLE across all categories (range in the present sample: 0–75, median=11).
5.1.3. Statistical analyses
All analyses were performed with the same software as in Investigation 1. In order to analyze gene–environment interactions, we used analysis of covariance (ANCOVA) with normalized NFC and Openness to Ideas scores as dependent variables, DRD4 and COMT as categorical predictors and the NLE measures as continuous covariates. As both measures were highly correlated, r s=.78, p<.001, separate analyses were run. Both variables were not normally distributed, S–W tests, both p<.001, and, thus, were normalized which for the number of NLE resulted in normally distributed scores, p=.522, while the number of different NLE still deviated from normality, p=.014.
With regard to the covariates, sex, and educational level were not considered further given that they had not impacted the results in Investigation 1 and in order to reduce the complexity especially of the Bayesian analysis. However, a relation of age on the two NLE measures variables is theoretically plausible and was empirically supported, both p<.001. Therefore, we partialled out age from the NLE scores. Again, all continuous variables were mean-centered for better interpretation and visualization. Simple slope analysis was based on regressions of the intellectual investment traits on the NLE variables, separately for the different genotype groups. As we ran four ANCOVAs with seven predictors (three main effects, three two-way interactions, and one three-way interaction), the Bonferroni-adjusted level of significance was set to .05/28~.002. At this level, our sample size enabled us to detect effects accounting for about 4% variance at 1−β=.80.
With regard to Bayesian analyses, we tested models with the NLE main effects as well as all gene–environment interactions up to the NLE×DRD4×COMT interaction. In addition, for persuasive models that included interactions, but also the NLE main effect, the former models were tested against the latter to examine whether the interaction models provided substantially more evidence than the simpler NLE main effect model.
5.2. Results
Figure 2 illustrates the results of the analyses on the impact of NLE on NFC and Openness to Ideas and possible interaction effects with genetic variation by providing the slopes for the two DRD4 genotypes as well as their mean, that is, the NLE main effects. Interaction effects with COMT genotypes are not displayed due to a lack of evidence in favor of such effects. Moreover, the figure gives the results of the Bayesian analyses.
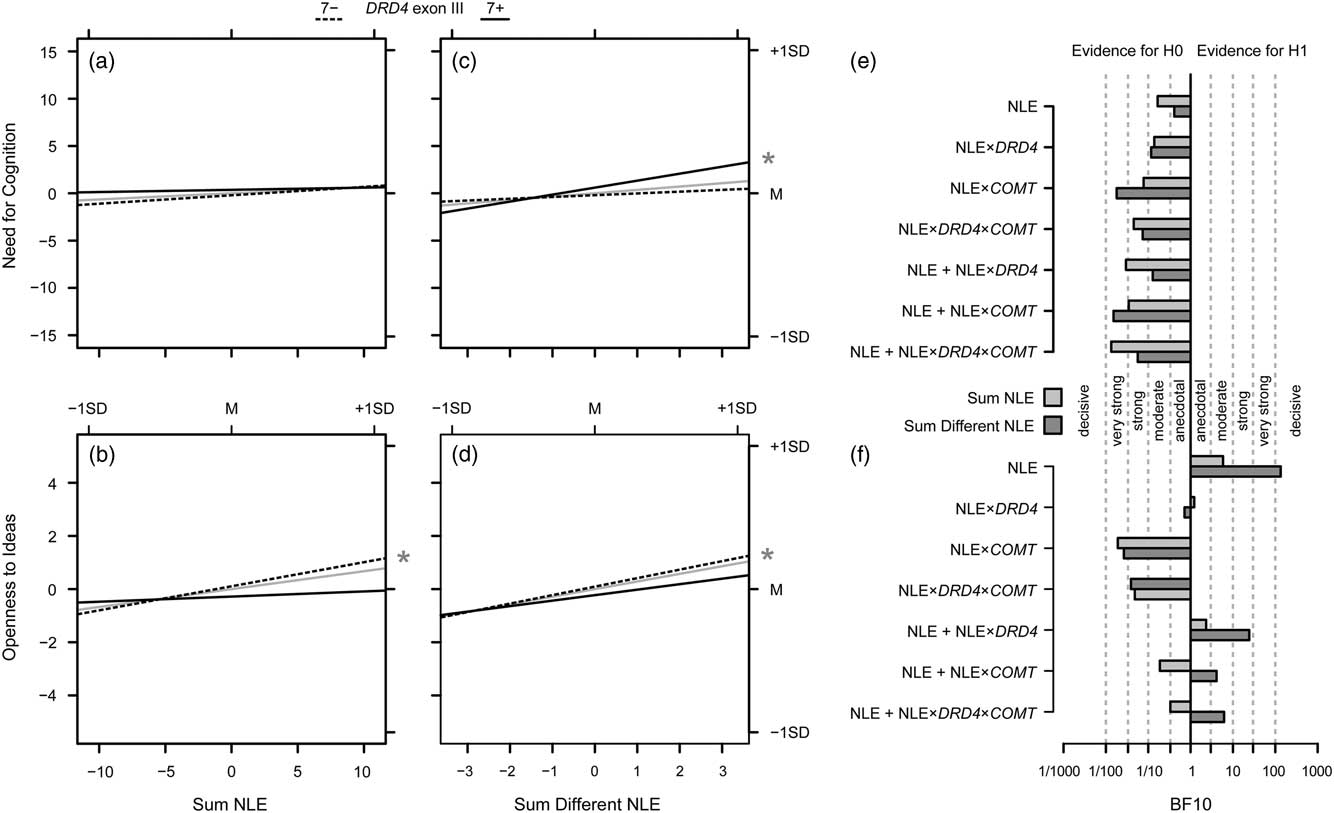
Figure 2 Overview on the results of Investigation 2. (a) Effects of the absolute sum of negative life events (NLE), both for the total effect on Need for Cognition (gray solid lines), and separately for non-carriers (7−; dashed lines) and carriers (7+; solid lines) of the DRD4 exon III 7-repeat allele. (c) Same for the effects of the sum of different NLE; the asterisk in the margin indicates a significant slope for 7-repeat carriers. (e) Bayes factors for models of interest (i.e., the NLE main effects, the NLE×gene interactions and the additive effects of the NLE variables plus the respective interactions) drawn at the y-axis versus the null model (i.e., no effect); verbal labels denote the magnitude of evidence in favor of the H0; light gray bars give the Bayes factors against the null model for models including the absolute number of NLE, dark gray bars provide the information for models including the number of different NLE; b, d, f). Same as (a, c, e) for Openness to Ideas.
5.2.1. Genetic and environmental influences on Openness to Ideas
For the models with the absolute number of NLE (Figure 2B), no significant gene×interactions emerged, neither at the adjusted level nor nominally, all p≥.222, all
$$\eta _{p}^{2} \leq .01$$
, except for weak trends toward an NLE main effect, F(1, 443)=3.39, p=.066,
$$\eta _{p}^{2} \,\lt\,.01$$
, and a DRD4×NLE interaction, F(1, 443)=3.43, p=.065,
$$\eta _{p}^{2} \,\lt\,.01$$
. A similar picture emerged for the analyses with the number of different NLE (Figure 2D): none of the effects reached the adjusted level of significance, all p≥.221, all
$$\eta _{p}^{2} \leq .01$$
, and only the main effect of the sum of different NLE was at least nominally significant, F(1, 443)=6.59, p=.011,
$$\eta _{p}^{2} {\equals}.02$$
(Figure 2E).
5.2.2. Genetic and environmental influences on NFC
For the models with the sum of all NLE (Figure 2A), the analyses with NFC as dependent variable showed that apart from the again significant DRD4×COMT interaction, F(2, 443)=7.28, p<.001,
$$\eta _{p}^{2} {\equals}.03$$
, none of the effects reached the adjusted level of significance, all p≥.016 (the COMT main effect was indeed nominally significant, all other p≥.264), all
$$\eta _{p}^{2} \leq .01$$
(except COMT,
$$\eta _{p}^{2} {\equals}.02$$
). A similar picture emerged for the analyses with the sum of different NLE (Figure 2C): apart from the DRD4×COMT interaction, F(2, 443)=6.99, p=.001,
$$\eta _{p}^{2} {\equals}.03$$
, none of the effects reached the adjusted level of significance, all p≥.018 (the COMT main effect was again nominally significant, all other p≥.221), all
$$\eta _{p}^{2} \leq .01$$
(except COMT,
$$\eta _{p}^{2} {\equals}.02$$
; Figure 2C).
5.2.3. Bayes factors
For Openness to Ideas, both the analyses with regard to the absolute number of NLE and the number of different NLE provided evidence in favor of the alternative hypothesis of a modulatory role of NLE: there was moderate evidence for the alternative hypothesis of a main effect of the absolute number of NLE on Openness to Ideas, BF10=5.84, and decisive evidence in favor of the hypothesis that the number of different life events modulates Openness to Ideas, BF10=133.93 (Figure 2F).
Moreover, the models with gene–environment interactions in addition to the main effect of the number of different NLE had Bayes factors in favor of the alternative hypothesis, DRD4: BF10=24.25, COMT: BF10=4.11, DRD4×COMT: BF10=6.18. However, when comparing these models against the model with only the environmental effect—by dividing the Bayes factors obtained for the interaction models by the Bayes factor of the model with only the main effect of the number of different NLE—the obtained Bayes factors were as follows: adding DRD4: BF10=0.18, adding COMT: BF10=0.03, adding DRD4×COMT: BF10=0.05. That is, the data were about 6–30 times less probable under the hypothesis of an increment in predictive power by adding the gene–environment interaction effects than assuming environmental effects only. Hence, the main result of the Bayesian analysis is that there is moderate (for the absolute number of NLE) and decisive evidence (for the number of different NLE) for a role of these variables in the modulation of Openness to Ideas, whereas models with gene–environment interactions in addition to the environmental effects were less supported by the data than the model of environmental effect of the number of different NLE alone. Please see the results based on the standard approach: in cases where the effects of the NLE variables approached or even surpassed the nominal level of significance in the standard approach, the Bayesian analysis also provided at least suggestive evidence.
In contrast, moderate to very strong evidence for the null model was obtained for NFC, BF10≤0.17 for the absolute number of NLE, and BF10≤0.12 for the number of different NLE, except for the main effect of the number of different NLE where evidence against the null model was only anecdotal, BF10=0.41, that is, this effect is only about 2.4 times less likely than the null model and thus cannot readily be discarded.
5.3. Discussion
Using the standard approach, NLE did not significantly explain variance in NFC. Likewise, at the adjusted level of significance, Openness to Ideas was not related to NLE. Some suggestive evidence was obtained at the nominal significance level, and this evidence was supported by the estimation of Bayes factors that argue for a moderate to decisive evidence in favor of a role of NLE in the modulation of Openness to Ideas. While adding gene–environment interactions to the environment main effect also yielded moderate to strong evidence in favor of these models, they were less supported by the data than the inclusion of the environmental effects alone.
Taken together, these results suggest a relation of NLE with intellectual investment that appears to be specific for Openness to Ideas. This could be explained by the assumption that even negative events—or coping with them—might be conducive of a higher Openness to alternative views on life. Conversely, higher Openness to Ideas could also be associated with a higher propensity to attribute significance to life experiences, which could result in better recall performance. Furthermore, the specificity of the findings of Investigation 2 for Openness to Ideas once again points to some so far elusive differences between NFC and Openness to Ideas. Anyway, our initial hypothesis that carriers of the DRD4 exon III 7-repeat allele would exhibit lower scores in investment traits with increasing number of reported adverse life events and vice versa was not supported by our data. However, so far we had examined only NLE, while in previous reports on DRD4×environment interactions, both adverse and beneficial environmental influences had been examined. Thus, we re-examined our sample and invited the participants to fill in a newly developed questionnaire on both, PLE and NLE encountered so far.
6. Investigation 3: The role of PLE and NLEFootnote 2
6.1. Method
6.1.1. Sample
The sample comprised a subset of the original sample who responded to our follow-up request to fill in a questionnaire assessing both PLE and NLE (n=252, 47% men, age mean±SD: 35.9±11.4 years, age range: 18–60 years). The sample had a slightly higher self-reported educational level as compared with the total sample analyzed in Investigation I, with 69% holding a university entrance diploma (about 10% more than in the total sample). Again, the genotypes were in HWE, χ 2=0.06, df=1, p=.813 for COMT Val158Met, and χ 2=4.12, df=5, p=.532 for DRD4 exon III.
6.1.2. Procedure
The assessment was done online using LimeSurvey (LimeSurvey Project Team & Schmitz, 2015), and participants could win 20 EUR out of a pool of 50×20 EUR upon leaving their email addresses that were kept apart from the questionnaire data in a separate LimeSurvey database. Participants responded to a 79-item self-report questionnaire loosely based on the Munich Event List (Wittchen, Essau, Hecht, Teder, & Pfister, Reference Wittchen, Essau, Hecht, Teder and Pfister1989). Items assessed life events across 12 domains, six of them related to relationships (e.g., with parents/carers, siblings, partners) and six related to one’s life situation (e.g., health and trauma, education, and work). Participants had to indicate whether, when, and for how long they had experienced each of these events, and how they evaluated these experiences on a 7-point scale (ranging from −3=‘very negative,’ to+3=‘very positive’). Importantly, participants were also asked to consider a possibly positive impact of at first glance negatively valenced life events and vice versa. We computed the sum of PLE (median=27, range=6–68) and NLE (median=12, range: 0–51) encountered so far.
6.1.3. Statistical analyses
All analyses were performed using the same software as in Investigation 2. Again, the NFC and Openness to Ideas scores were normalized to yield normally distributed measures, S–W tests, p≥.737. With regard to covariates, again only age was considered because of theoretical reasoning and its empirical impact on the number of PLE and NLE, B≥0.21, p≤.001. Therefore, we partialled out age from the number of PLE and NLE. As in Investigation 2, all continuous variables were mean-centered for better interpretation and visualization, and simple slope analysis was based on regressions of the intellectual investment traits on the PLE and NLE variables, separately for the different genotype groups. As the number of PLE and NLE were only weakly correlated, r=.23, p<.001, we ran two ANCOVAs with both types of life events as well as the DRD4 and COMT genotypes as independent variables and Openness to Ideas and NFC as dependent variables. This resulted in 15 effects (four main effects, six two-way interactions, four three-way interactions, and one four-way interaction) per ANCOVA, hence, the Bonferroni-adjusted level of significance was set to .05/30~.002. At this level, our sample size enabled us to detect effects accounting for about 7% variance at 1−β=.80.
With regard to Bayesian analyses, we repeated the approach taken in Investigation 2 and tested models with the life events main effects as well as all gene–environment interactions up to the NLE×DRD4×COMT interaction. In addition, for persuasive models that included interactions, but also the life events main effect, the former models were tested against the latter to examine incremental evidence over the simpler life events main effect model.
6.2. Results
Figure 3 illustrates the results of the analyses on the impact of PLE and NLE and their interaction with genetic variation on NFC and Openness to Ideas by providing the slopes for the DRD4 genotypes as well as for their mean, that is, for the main effects of PLE and NLE. Effects for COMT genotypes are not displayed due to a lack of evidence for such effects.
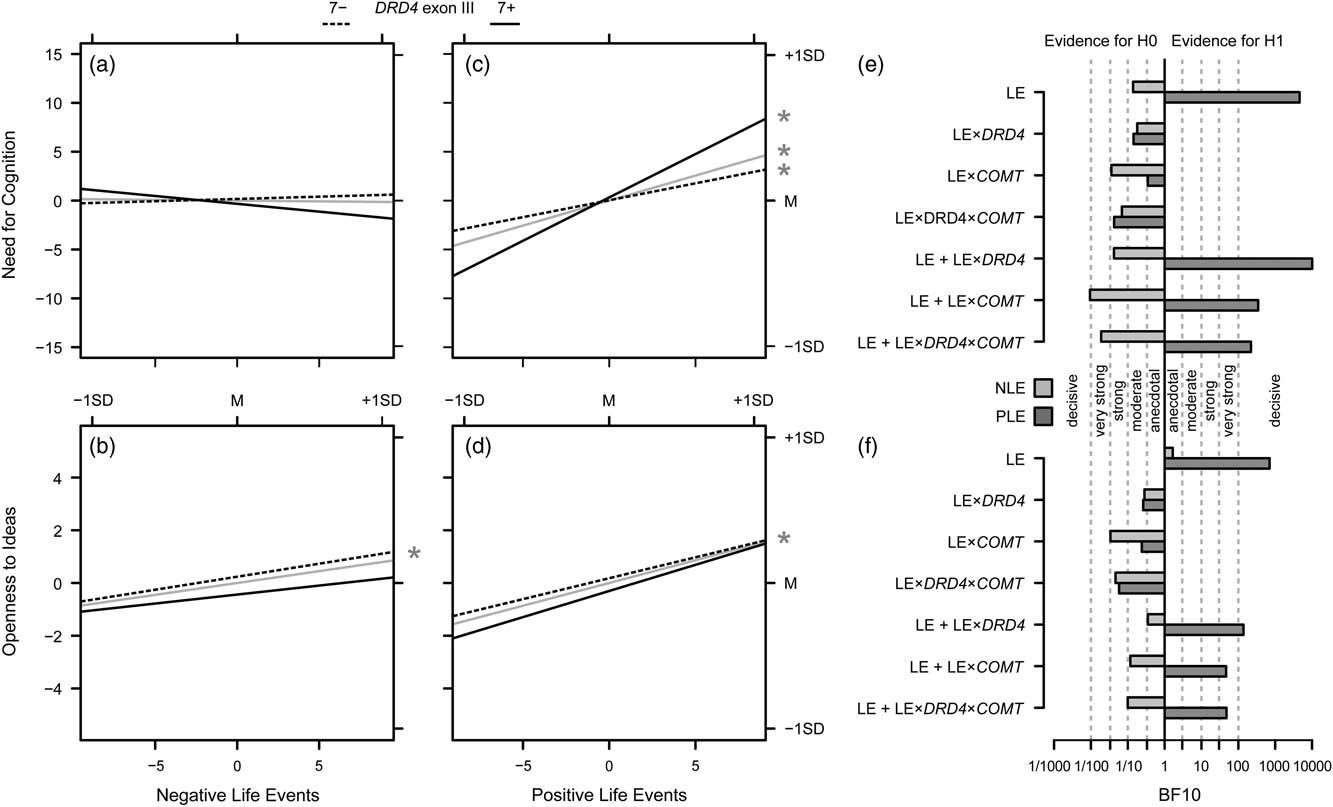
Figure 3 Overview on the results of Investigation 3. (a) Effects of the number of negative life events (LE), both for the total effect on Need for Cognition (gray solid lines), and separately for non-carriers (7−; dashed lines) and carriers (7+; solid lines) of the DRD4 exon III 7-repeat allele. (c) Same for the effects of the number of positive LE; the asterisk in the margin indicates significant slopes. (e) Bayes factors for models of interest (i.e., the LE and LE main effects, the LE×gene interactions and the additive effects of the LE variables plus the respective interactions) drawn at the y-axis versus the null model (i.e., no effect); verbal labels denote the magnitude of evidence in favor of the H0; light gray bars give the Bayes factors against the null model for models including the number of negative LE, dark gray bars provide the information for models including the number of positive LE; b, d, f). Same as (a, c, e) for Openness to Ideas.
6.2.1. Genetic and environmental influences on Openness to Ideas
Only the number of PLE was significantly associated with Openness to Ideas, F(1, 228)=15.02, p<.001,
$$\eta _{p}^{2} {\equals}.07$$
, all other p≥.08,
$$\eta _{p}^{2} \leq .02$$
(Figure 3B and D). However, when analyzing the number of NLE alone for the purpose of visualization, at least a trend for the number of NLE emerged, B=0.09, p=.022 (Figure 2B).
6.2.2. Genetic and environmental influences on NFC
Also for NFC, there was evidence for an impact of PLE, F(1, 228)=21.14, p<.001,
$$\eta _{p}^{2} {\equals}.09$$
(Figure 3B), while again, there was no evidence for a role of NLE in the modulation of NFC, F(1, 228)=2.66, p=.104,
$$\eta _{p}^{2} {\equals}.01$$
(Figure 3A). Nominally significant effects were observed for the DRD4×COMT interaction, F(2, 228)=3.05, p=.049,
$$\eta _{p}^{2} {\equals}.03$$
, and for the interaction of DRD4 with PLE, F(1, 228)=4.45, p=.036,
$$\eta _{p}^{2} {\equals}.03$$
(Figure 3B), all other p≥.15,
$$\eta _{p}^{2} \leq .02$$
.
6.2.3. Bayes factors
There was anecdotal evidence for the hypothesis of a role of NLE in the modulation of Openness to Ideas, BF10=1.66, and moderate evidence against the hypothesis of a role of the number of NLE in the modulation of NFC, BF10=0.14. Moreover, there was moderate to strong evidence against the hypothesis that gene–environment interactions as such play a role in the modulation of investment traits, all BF10≤0.35 (Figure 3E and F). However, decisive evidence was obtained for the hypothesis of an impact of PLE on Openness to Ideas, BF10=705.66, and on NFC, BF10=4595.20 (Figure 3E and F, top row). That is, the data were about 700 times more likely under the assumption of a role of PLE in the modulation of Openness to Ideas, and over 4,500 times more likely under the assumption of a role of PLE in the modulation of NFC as compared with the null model. Moreover, also for the models with gene–environment interactions in addition to the PLE main effect, very strong to decisive evidence was obtained, with BF10 ranging from 46.70 (for the model with the PLE×COMT interaction for Openness to Ideas) to 10,113.04 (for the model with the PLE×DRD4 interaction for NFC). However, when comparing the respective models against the models with the PLE main effects only, no substantial incremental evidence of the gene–environment interaction effects over and above the environment effects could be obtained for Openness to Ideas: adding PLE×DRD4: BF10=0.20, adding PLE×COMT: BF10=0.07, adding PLE×DRD4×COMT: BF10=0.07. For NFC, the comparison was basically similar: adding PLE×COMT: BF10=0.08, adding PLE×DRD4×COMT: BF10=0.05. Yet, adding PLE×DRD4 to the PLE main effect resulted in BF10=2.20. That is, while the data were about 13–20 times more likely under the assumption of PLE main effect only as compared to models that included an interaction with COMT, the data were about twice as likely under the assumption of a PLE×DRD4 supplement to the main effect than under the assumption of a PLE main effect alone.
6.3. Discussion
Using the standard approach, the number of PLE was positively associated with both Openness to Ideas and NFC. No interaction between COMT Val158Met and life events was observed, neither for NFC nor for Openness to Ideas. However, for NFC, an at least nominally significant interactive effect of PLE and DRD4 exon III genotype was observed that mirrors previous findings: The increase in NFC with increasing number of PLE was most prominent in carriers of the DRD4 7-repeat allele.
Using the Bayesian approach, the impact of PLE on both Openness to Ideas and NFC was confirmed. With regard to the interactive effect of the number of PLE and DRD4 exon III, there was evidence that the data were about twice as likely when adding this interaction to the main effect of PLE than when considering the main effect alone. Given the above-mentioned intervals for evidence in favor of one model against another (Wetzels et al., Reference Wetzels, Matzke, Lee, Rouder, Iverson and Wagenmakers2011), this would be considered as only anecdotal evidence. However, as Rouder, Morey, Verhagen, Swagman, and Wagenmakers put it, “Bayes factor evidence should not be evaluated by strict criterion, but in reference to the context and goals of the researcher” (Reference Rouder, Morey, Verhagen, Swagman and Wagenmakers2017, p. 314). In the present case—the convergence of the PLE×DRD4 interaction being significant at the nominal level using the standard ANCOVA approach, a two-fold increased probability of the data under the assumption of adding the PLE×DRD4 interaction to the PLE main effect using a Bayesian approach, and the multitude of reports of a beneficial effect of positive environments on controlled behaviors especially in carriers of the DRD4 7-repeat allele (e.g., Sheese et al., Reference Sheese, Voelker, Rothbart and Posner2007; Bakermans-Kranenburg & van Ijzendoorn, Reference Bakermans-Kranenburg and van Ijzendoorn2011; Sheese et al., Reference Sheese, Rothbart, Voelker and Posner2012)—we propose this evidence as tentative support for the assumption of an interactive effect of PLE and genetic variation in DRD4 function. We will attempt to provide an interpretation of this finding below in order to further justify that replication attempts are warranted.
7. General discussion
In the present study, we examined possible causal influences of genetic variation in prefrontal dopaminergic function and of environmental variation on the intellectual investment traits Openness to Ideas and NFC. Using a standard approach that relied on linear models and null-hypothesis significance testing, we found that, first, two dopaminergic genetic variants interacted in modulating individual differences in NFC, but not in Openness to Ideas (Investigation I); that, second, NLE played a role in the modulation of Openness to Ideas, but not of NFC (Investigation 2); and that, third, NLE as assessed using another measure were not substantially related to Openness to Ideas, while PLE were associated with both Openness to Ideas and NFC, with the latter effect also being dependent on DRD4 exon III genotype: increasing NFC scores with increasing number of PLE were especially observed in 7-repeat allele carriers, who reportedly show enhanced plasticity depending on environmental conditions (Investigation 3). However, using a Bayesian approach, the assumption of a gene–gene interaction effect on investment traits had to be discarded, while to some degree leaving open the question of an effect of COMT variation in carriers of the DRD4 7-repeat allele (Investigation 1). There was evidence for a role of NLE in the modulation of Openness to Ideas (Investigation 2), that, however, could not be corroborated using another instrument (Investigation 3), while there was unequivocal evidence for a role of PLE in the modulation of investment traits in general, with a tentative increment in predictive power by adding an interaction of PLE with DRD4 variation to the main effect of PLE. In the following, we will first provide a summary of the effects obtained by the standard approach, then a critical reexamination of these effects in light of the results of the Bayesian analyses, followed by a summarizing conclusion.
7.1. The role of genetic variation in prefrontal DA function
Encouraged by earlier results (Fleischhauer, Enge, & Donsbach, Reference Fleischhauer, Enge and Donsbach2008) and the findings of DeYoung et al. (Reference DeYoung, Cicchetti, Rogosch, Gray, Eastman and Grigorenko2011) on the role of two genetic polymorphisms relevant for prefrontal DA system function in Openness/Intellect, the potential impact of COMT Val1587Met and DRD4 exon III on intellectual investment traits was examined. We found an interactive effect, with the hypothesized increasing NFC scores with increasing number of COMT Met alleles being only observable in carriers of the DRD4 exon III 7-repeat allele. Thus, we could not straightforwardly corroborate the findings of DeYoung et al. (Reference DeYoung, Cicchetti, Rogosch, Gray, Eastman and Grigorenko2011), who—while also observing an interactive effect of COMT×DRD4 on Openness/Intellect in an adult sample—found that in carriers of the DRD4 exon III 7-repeat allele, COMT Met homozygotes showed the highest scores in Openness/Intellect. Apart from the different interaction pattern, we did not find the COMT×DRD4 interaction effect to be significant for Openness to Ideas (and we also did not observe such an effect when examining the broader construct Openness to Experience). Nevertheless, the general conclusion drawn by DeYoung et al. that these genetic variants influence individual differences in intellectual investment are backed at the basis of the classical statistical approach: DeYoung et al. (Reference DeYoung, Cicchetti, Rogosch, Gray, Eastman and Grigorenko2011) and our ANOVA results converge in the conclusion that genetic variation in prefrontal DA system function impact individual differences in, as DeYoung et al. (Reference DeYoung, Cicchetti, Rogosch, Gray, Eastman and Grigorenko2011) put it, “cognitive exploration.”
Yet, the results of the Bayesian analyses provide moderate to strong support for a model without genetic effects, that is, for the null hypothesis. Interestingly, however, when only considering the subgroup of DRD4 7-repeat allele carriers, strong evidence for a COMT effect was obtained. Thus, the plausibility of such an effect deserves closer examination.
There is evidence that genetic variation in COMT function (1) influences prefrontal control functions such as shifting and updating (Frank & Fossella, Reference Frank and Fossella2011; Mier, Kirsch, & Meyer-Lindenberg, Reference Mier, Kirsch and Meyer-Lindenberg2010); that (2) the COMT Met allele is dose-dependently associated with a higher degree of uncertainty-based exploration, interpreted as indexing a higher motivation to show exploratory behavior in order to reduce uncertainty and thereby improving performance in the long run (Frank, Doll, Oas-Terpstra, & Moreno, Reference Frank, Doll, Oas-Terpstra and Moreno2009); and that (3) Met allele carriers dose-dependently show a stronger propensity for model-based learning (Doll, Bath, Daw, & Frank, Reference Doll, Bath, Daw and Frank2016) that is characterized by an “evaluation of candidate actions using expected future outcomes according to a world model” (Doll, Simon, & Daw, Reference Doll, Simon and Daw2012, p. 1075). These striking conceptual similarities between uncertainty-based exploration and model-based learning for the sake of performance improvements in the long run on the one hand and the investment of cognitive resources into problem solving on the other could provide a plausible explanation of the effect of COMT effect on NFC observed here.
With regard to a biologically plausible explanation of the observation that the COMT effect on NFC was driven by carriers of the DRD4 exon III 7-repeat allele, evidence seems to favor the assumption of lower D4 receptor function in the presence of the 7-repeat allele (Pappa, Mileva-Seitz, Bakermans-Kranenburg, Tiemeier, & van Ijzendoorn, Reference Pappa, Mileva-Seitz, Bakermans-Kranenburg, Tiemeier and van Ijzendoorn2015). A recent study suggests an overall balancing influence of D4 receptor activation on prefrontal network activity (Zhong & Yan, Reference Zhong and Yan2016). Wang et al. (Reference Wang, Ding, Flodman, Kidd, Kidd, Grady and Moyzis2004) argued that the suboptimal function of D4 receptors favors behaviors and environments that result in elevated dopaminergic tone, and indeed, our own work supports the assumption of a differential role of tonic DA function in carriers and non-carriers of the DRD4 7-repeat allele (Dreisbach et al., Reference Dreisbach, Müller, Goschke, Strobel, Schulze, Lesch and Brocke2005; Strobel et al., Reference Strobel, Debener, Anacker, Muller, Lesch and Brocke2004). Together with the COMT-mediated modulation of tonic DA function (Bilder et al., Reference Bilder, Volavka, Lachman and Grace2004), these results could form a basis for an explanation of the COMT×DRD4 interaction observed here: the lower D4 receptor function in 7-repeat allele carriers could be amended by elevated extrasynaptic levels of prefrontal DA in the presence of the low-functioning COMT Met allele. This could eventually result in adaptive exploratory tendencies instead of maladaptive impulsive behaviors (cf. Wang et al., Reference Wang, Ding, Flodman, Kidd, Kidd, Grady and Moyzis2004).
However, despite the plausibility of the potential mechanisms behind the genetic effects obtained by the classical statistical approach as outlined above, our demonstration of strong evidence for a COMT effect on NFC at least in the subgroup of DRD4 7-repeat allele carriers was no planned contrast. Thus, we have to conclude for now, that our data are more probable under the null hypothesis that there is no effect of COMT Val158Met and DRD4 exon III on cognitive exploration, or it is very small or only present for certain genotype groups. We therefore hope for independent replication attempts.
7.2. The role of NLE
As mentioned in the Introduction section, a modulating role of positive environments on DRD4 exon III mediated effects on controlled behavior has been shown (e.g., Bakermans-Kranenburg & van Ijzendoorn, Reference Bakermans-Kranenburg and van Ijzendoorn2011; Sheese et al., Reference Sheese, Rothbart, Voelker and Posner2012), which is why we assumed that NFC as a propensity for controlled processing might be higher in carriers of the DRD4 exon III 7-repeat allele who had experienced less adverse or even beneficial environments. Investigation 2 showed that NLE were not related to NFC, neither as main nor as gene–environment interaction effects. In turn, Openness to Ideas was significantly related to both the total number of NLE and the number of different NLE, while again, gene–environment interactions were also absent for Openness to Ideas.
Crucially, this impact of NLE on Openness to Ideas was backed by the Bayesian analyses: while these analyses again provide no evidence for the assumption of gene–environment interactions, there is moderate evidence for the hypothesis of an impact of the absolute number of NLE and decisive evidence for a role of the number of different NLE in the modulation of this intellectual investment trait. Thus, further inquiries into the mechanisms by which the encounter of and coping with adverse environments shape individual differences in intellectual investment traits are warranted.
Unfortunately, there is scarce evidence on the developmental influences and the role of live events on investment traits. DeYoung (Reference DeYoung2014) summarized evidence on developmental origins of Openness/Intellect that points to a role of child–parent attachment, infant curiosity, and exploration as well as orienting sensitivity. For NFC, we could not identify similar evidence apart from the theoretical claim of Cacioppo et al. that “need for cognition, at least in theory, should result from a person’s values and the competence feedback and feelings of personal satisfaction and mastery derived from cognitive challenges” (Reference Cacioppo, Petty, Feinstein and Jarvis1996, p. 246). In this context, they emphasized the importance of observing and experiencing success with a problem-solving-focused style of coping with the challenges of life.
With respect to coping, it has been shown that Openness to Experience is related to an engagement-focused coping style and to problem-solving-focused engagement (Carver & Connor-Smith, Reference Carver and Connor-Smith2010; Connor-Smith & Flachsbart, Reference Connor-Smith and Flachsbart2007). By inference, NFC should also be related to this type of coping. Indeed, one study on the role of NFC in coping with the challenges of the transition to retirement showed that higher NFC predicted higher positive affect during this transition, an effect that was mediated by the frequency of cognitive activity and problem-focused coping (Bye & Pushkar, Reference Bye and Pushkar2009). It can be assumed that this higher positive affect acts as reinforcing for NFC-related behavioral tendencies.
As we did not obtain information on the evaluation of the life events or on coping strategies and their success, an interpretation of the pattern of results remains speculative. Concerning the lack of main effects of NLE on NFC, but their presence for Openness to Ideas, one could argue that the occurrence of life events in general results in a higher Openness to alternative views on life or that, conversely, individuals high in Openness to Ideas regard the mere presence of NLE as challenge as has already proposed (cf. Carver & Connor-Smith, Reference Carver and Connor-Smith2010). For NFC, similar ideas have not been put forward, so our only conjecture is that the goal-oriented aspect of NFC—that seems less pronounced in Openness to Ideas—and the associated different coping strategies may make some difference. Anyway, an explanation of the divergent results for NFC and Openness to Ideas observed here must remain notional as long as there is no more profound knowledge on the role of life events in shaping intellectual investment traits.
7.3. The role of PLE
In Investigation 3, neither classical nor Bayesian analyses substantially favor the assumption of a role of NLE in the modulation of Openness to Ideas (this time using another instrument for NLE assessment) or NFC, but both approaches strongly support the assumption of a positive impact of PLE on intellectual investment traits. Moreover, the expected gene–environment interaction pattern is apparent for NFC when using the standard approach: the increase in NFC scores with increasing number of PLE is especially prominent among DRD4 7-repeat allele carriers. Bayesian analyses also at least tentatively favor an increment of adding the interaction of DRD4 exon III with PLE to the sole environmental main effect.
This suggests that PLE act as reinforcing for intellectual investment. However, it could also be the other way round: individuals high in intellectual investment traits may report a higher number of PLE, because (1) they appraise their life events as more positive or (2) remember more PLE because of their goal-directed attentional focus, which may also explain why this effect was much stronger for NFC that has been observed to be more strongly related to goal-directedness than Openness to Ideas (Fleischhauer et al., Reference Fleischhauer, Enge, Brocke, Ullrich, Strobel and Strobel2010). These issues cannot be resolved with our cross-sectional design. Therefore, a longitudinal assessment of life events that also utilizes, first, more objective measures of life events beyond mere self-reports, and second, more detailed assessments of the appraisals of specific life events is necessary to attain a clearer picture of the role that PLE and NLE play in shaping intellectual investment traits.
Nevertheless, it is quite striking that our prediction of an interactive effect of PLE and DRD4 exon III genotype on intellectual investment was at least tentatively confirmed for NFC. As outlined in the Introduction section, DRD4 exon III has been suggested as a plasticity factor that, depending on environmental influences, modulates behavior for better or for worse (Bakermans-Kranenburg & van Ijzendoorn, Reference Bakermans-Kranenburg and van Ijzendoorn2011; Belsky et al., Reference Belsky, Jonassaint, Pluess, Stanton, Brummett and Williams2009; Sheese et al., Reference Sheese, Rothbart, Voelker and Posner2012; Sheese et al., Reference Sheese, Voelker, Rothbart and Posner2007). So far, accounts for such environmental influences on this plasticity to our knowledge have not been suggested. A possibly heightened sensitivity to significant environmental events in DRD4 exon III 7-repeat allele carriers (Gorlick et al., Reference Gorlick, Worthy, Knopik, McGeary, Beevers and Maddox2015) may, when these events are perceived as positive, act as somehow reinforcing and, by inference, DA-enhancing. In the long run, this could lead to enhanced tonic DA levels that, as we supposed above, could explain why the otherwise rather deficient D4 receptor functioning in 7-repeat allele carriers that normally would destabilize prefrontal functioning could nevertheless facilitate controlled processing. Taken together, it is hoped that our results stimulate further research on the interaction between positive environments and DRD4 variation, given the convergence of classical and Bayesian approach and the literature.
7.4. Limitations
Several limitations of the present study need to be mentioned. First, the sample size of the initial sample enabled us to detect effect sizes of at best 2% of explained variance. For the subsequent analyses with n=455, and n=252, respectively, the power was considerably reduced, but still enabled us to detect small effects with a power of .8. However, it may not be reasonable to assume genetic effects of this size. Rather, effect estimates from genome-wide association studies (GWAS) suggest effects accounting for less than 1% of variance (de Moor et al., Reference de Moor, van den Berg, Verweij, Krueger, Luciano, Vasquez and Consortium2015; Flint & Munafò, Reference Flint and Munafò2013). Thus, the lack of power to detect such small effects may have also been problematic for the Bayesian analyses. This points to a more general issue attributed to the candidate gene approach by many researchers in the community: a lack of replicability because of underpowered studies and no internal replication samples. The present contribution may be no exception, although it can be considered as an—at least conceptual—replication itself. However, we believe that the candidate gene approach still deserves attention and has its own right even with the advent of GWAS. At first glance, they seem by far superior to candidate gene research given the large sample sizes employed, the statistical rigor (e.g., by setting stringent thresholds for statistical significance) and the internal replication samples. Nevertheless, in line with Montag and Reuter (Reference Montag and Reuter2014), we deem results from GWAS itself as subject to replication difficulties which is likely due to the complexity of genetic modulation of complex traits, and—more importantly—even replicated GWAS signals need further analysis with regard to their theoretical implications for the traits under investigation. As an example, in a recent meta-analysis of GWAS for personality (de Moor et al., Reference de Moor, Costa, Terracciano, Krueger, de Geus, Toshiko and Boomsma2010), a genome-wide significant association with Openness to Experience was found for variation in the RASA1 gene region. This association could not unequivocally be replicated across the different samples used in this report, and the RASA1 gene appears to be highly expressed in bone and marrow, but only modestly in the brain (de Moor et al., Reference de Moor, Costa, Terracciano, Krueger, de Geus, Toshiko and Boomsma2010). Thus, given the genome-wide signal and the statistical rigor of the approach, further—candidate gene driven—research seems to be warranted to explain why a gene that is only modestly expressed in the brain should be related to an investment trait (see, e.g., Boyle, Li, & Pritchard, Reference Boyle, Li and Pritchard2017, for the so-called omnigenetic model). While candidate gene studies need of course be adequately powered to detect effects of less than 1% of variance, which by far too often has not been the case (Flint & Munafò, Reference Flint and Munafò2013), we believe that there is still a need for candidate gene based research to follow-up on GWAS results.
Moreover, the power of the study would further decrease with further corrections for multiple testing than that already performed. While we had rather clear hypotheses on the effects to be observed, we tested a number of other effects in order to pinpoint the effects of interest, hence, an even more rigorous approach to multiple testing would perhaps render some of the findings from the standard approach insignificant. However, we are at least confident that in the standard analyses, the results were not due to the impact of outliers or covariates, and we rest our final conclusions only on those results that were also obtained using a Bayesian approach to minimize the possibility of chance findings.
Furthermore, although our sample was randomly drawn from the general population, this population was limited to a certain German area, and we had to rely on the respondents to our call for participation. The rather high level of education in our sample points to some self-selection bias. This is an inevitable problem of volunteer-based research. To partly overcome this issue and to also address the concern of non-representativeness (Henrich, Heine, & Norenzayan, Reference Henrich, Heine and Norenzayan2010), a cross-cultural approach may be a solution (Montag & Reuter, Reference Montag and Reuter2014; Montag et al., Reference Montag, Sindermann, Melchers, Jung, Luo, Becker and Kendrick2017).
Another limitation is the nature of the assessment of life events. Self-reports may be distorted by social desirability, and episodic memory may be systematically biased by individual differences in personality traits. Thus, future studies on the role of life events in shaping intellectual investment traits should strive for more objective measures such as informant reports, or, for shorter time scales, experience sampling via mobile devices.
A final issue needs to be discussed that relates to the genetic variables examined here: although we selected two polymorphisms that have been implicated in prefrontal functioning (Mier, Kirsch, & Meyer-Lindenberg, Reference Mier, Kirsch and Meyer-Lindenberg2010; Oak, Oldenhof, & Van Tol, Reference Oak, Oldenhof and Van Tol2000) and implicated in intellectual investment before (DeYoung et al., Reference DeYoung, Cicchetti, Rogosch, Gray, Eastman and Grigorenko2011), further variants relevant to prefrontal functioning should be examined in future studies on genetic influences on investment traits, with a polygenic score computed based on multiple genetic variants (e.g., Dudbridge, Reference Dudbridge2013; Montag & Reuter, Reference Montag and Reuter2014) probably being the most promising way of establishing a firm molecular genetics based link between individual differences in prefrontal functioning and intellectual investment traits.
As consequences of the issues discussed above, future candidate gene studies should adopt the rigorous methodological standards of GWAS. Moreover, they may be informed by GWAS results (Flint & Munafò, Reference Flint and Munafò2013) that could, for example, be used to (1) compute polygenic scores (see, e.g., Montag & Reuter, Reference Montag and Reuter2014) to explain more of the variance of the trait under investigation and account for small and/or inconsistent effects of single gene variants and (2) to follow-up on GWAS signals in order to establish biologically plausible causal links between novel gene variants and the trait in question. In this vein, candidate gene studies and GWAS should not be taken as antithetic, but as complementary approaches to the study of the role of genetic variation in individual differences. Furthermore, the samples of candidate gene research should be recruited from diverse ethnic backgrounds (as an example, see Montag et al., Reference Montag, Sindermann, Melchers, Jung, Luo, Becker and Kendrick2017), and different statistical approaches may be used in order to substantiate potential findings. In our view, the Bayesian approach is an ideal complement if not an alternative to classical null hypothesis significance testing as it can—among other benefits—provide evidence in favor of the absence of effects, just as in the case of the genetic main effects examined here. Finally, environmental effects need to be ascertained as objectively as possible and should ideally complemented by experience sampling procedures.
7.5. Conclusion and suggestions for future studies
Taken together, despite compelling previous evidence in favor of such effects, genetic variants impacting on prefrontal DA functioning could not be unequivocally demonstrated to be causally associated with individual differences in intellectual investment traits. Rather, Bayesian analyses favor the null hypothesis, but an effect of COMT may still exist in carriers of the DRD4 7-repeat allele. The experience of NLE may to some extent have a positive impact on Openness to Ideas, but not on NFC, while the experience of PLE appears to be substantially associated with both NFC and Openness to Ideas. Our results also suggest that the impact of PLE on NFC is particularly strong in carriers of the DRD4 exon III 7-repeat allele, being evident both in the standard and—albeit to a lesser extent—in the Bayesian analyses, and thus warrant further inquiry into gene–environment interactions. Whether the results for the environmental variables are due to a personality-based bias in remembering or appraising past life events or to causal influences of life events and their interaction with genetic predispositions on intellectual investment traits, remains to be pinpointed in future studies. These studies should ideally employ longitudinal designs with objective assessments of life events in adequately powered samples (and internal replication samples), ideally using polygenic scores derived from theory or from GWAS and having a cross-cultural design. Given the continuing interest in personality neuroscience, it is hoped that researchers with interest in intellectual investment traits join forces to realize this ambitious endeavor.
Acknowledgments
The authors wish to thank Dorothea Kluczniok for her valuable help in data acquisition and want to express their gratitude to Burkhard Brocke for his continuing advice and support for our efforts in engaging in research into the biological bases of personality.
Financial Support
This research was supported in part by grants from the German Research Foundation (DFG; SFB 940/2 and CRC 1193, Z03) and by the European Community’s Horizon 2020 Programme (H2020/2014-2020) under grant number 667302 (CoCA).
Conflicts of Interest
The authors have nothing to disclose.