Listeners’ perceptual judgments of second language (L2) speech are well-studied, especially in reference to the global speech dimensions of comprehensibility (ease of understanding) and accentedness (degree of nativelikeness; Derwing & Munro, Reference Derwing and Munro2015). Such dimensions are thought to be general in the sense that they account for qualities of speech that are not bound to communicative context or purpose – just the listener – despite an emerging body of evidence that communicative demand influences listener judgments through associated linguistic and temporal cues (Crowther et al., Reference Crowther, Trofimovich, Isaacs and Saito2015a, Reference Crowther, Trofimovich, Isaacs and Saito2018). Less well-studied are listener perceptual judgments that are more explicitly situated in particular communicative contexts and associated demands. In this study, we investigated the dimension of academic acceptability, which can be defined as acceptability for spoken communication in a tertiary academic setting. Though Thomson (Reference Thomson, Kang and Ginther2018) proposed that, in a perfect world, comprehensible speech should also be acceptable speech, little research has been conducted on the relationship between these two global speech dimensions. In our investigations, we explore the influence of linguistic (phonology, accuracy, complexity) and temporal (i.e., fluency) speech stream characteristics on listener judgments of comprehensibility and academic acceptability across four open-ended speaking performances elicited through the Duolingo English Test, a high-stakes English proficiency exam used for university admissions purposes. In addition to providing greater insight into the association between comprehensibility and academic acceptability, the inclusion of multiple speaking performances allows us to explore the extent to which L2 speakers’ speech stream characteristics and associated listener-based dimensions (acceptability, comprehensibility) are consistent across performances.
Literature review
L2 speech and perceptual judgments
Derwing and Munro (Reference Derwing and Munro2009) argued that listeners’ perceptions of L2 speech serve as the gold standard for assessment, as “what listeners perceive is ultimately what matters most” (p. 478). While listener perceptions have a longstanding history in applied linguistics research (e.g., Lambert et al., Reference Lambert, Hodgson, Gardner and Fillenbaum1960), L2 pronunciation research has drawn heavily on dimensions established in Munro and Derwing (Reference Munro and Derwing1995a): accentedness, comprehensibility, and intelligibility. The key finding of their study, one frequently replicated (e.g., Huensch & Nagle, Reference Huensch and Nagle2021), was that foreign-accented speech could at the same time be understandable. To measure understanding, Munro and Derwing included listener transcriptions as a measure of intelligibility (i.e., the accuracy with which a listener understands an L2 speaker’s intended utterance) and subjective scalar ratings as a measure of comprehensibility (or the perceived ease or difficulty of understanding an utterance). Interestingly, accuracy of understanding has been shown to not necessarily entail ease of understanding (see Derwing & Munro, Reference Derwing and Munro2015), indicating that the two measures of understanding could be argued to be partially independent dimensions of L2 speech.
Following Levis (Reference Levis2005), L2 speech scholars have consistently advocated for understandable over native-like speech as the target of pronunciation acquisition and pedagogy. Though interest in intelligibility as a measure of understanding remains high (e.g., Kang et al., Reference Kang, Thomson and Moran2018), there has been a noticeable shift towards comprehensibility as the measure of primary interest (e.g., Crowther et al., Reference Crowther, Holden and Urada2022). Beyond methodological advantages – scalar ratings common to comprehensibility research allow for more practical (i.e., quick) and reliable collection of listener judgments (Kennedy & Trofimovich, Reference Kennedy and Trofimovich2019) – Saito (Reference Saito2021) highlighted how such an approach “has strong ecological validity, as it is assumed to reflect the instant and impressionistic judgements made by interlocutors during oral communication in real-life contexts” (p. 86). As an additional reason for considering comprehensibility over intelligibility as a measure of understanding, consider that comprehensible speech is almost always assessed as intelligible (Derwing & Munro, Reference Derwing and Munro2015), which indicates that the acquisition of intelligible speech may outpace the acquisition of comprehensible speech. Thus, a pedagogical focus on comprehensible speech may have the added benefit of improving intelligibility (Thomson, Reference Thomson, Kang and Ginther2018).
Comprehensibility
Though not the earliest use of the term comprehensibility (e.g., Varonis & Gass, Reference Varonis and Gass1982), Munro and Derwing (Reference Munro and Derwing1995a) served to establish comprehensibility as it is generally investigated today (Crowther et al., Reference Crowther, Holden and Urada2022). That is, as the “[p]erceived degree of difficulty experienced by the listener in understanding speech” (Munro & Derwing, Reference Munro and Derwing2015, p. 14). By definition, comprehensibility is a perceptual measure (Munro & Derwing, Reference Munro and Derwing2020), with listeners’ assessments of ease of understanding a reflection of the processing difficulties faced while attending to foreign-accented speech (e.g., Munro & Derwing, Reference Munro and Derwing1995b). As a consequence, speech rated as less comprehensible, beyond generally being less intelligible, tends to elicit less favorable emotional reactions and social judgments (e.g., Dragojevic & Giles, Reference Dragojevic and Giles2016; Lev-Ari & Keysar, Reference Lev-Ari and Keysar2010).
One area of particular interest in L2 comprehensibility research has been to understand how measures of phonology, fluency, syntax, and lexicon influence listeners’ comprehensibility judgments, as understanding these influences enriches understanding of the dimension and helps to distinguish it from other listener perceptions. As the majority of comprehensibility studies rely on audio-only stimuli, listeners’ judgments are necessarily based on the speech stream itself (though see Trofimovich et al., Reference Trofimovich, Tekin and McDonough2021; Tsunemoto et al., Reference Tsunemoto, Lindberg, Trofimovich and McDonough2022, for recent, interaction-based judgments of comprehensibility). While the strength of a given measure’s influence is variable across studies, the general pattern that has emerged is that listeners, when attending to comprehensibility, attend to a range of phonological (e.g., segmental accuracy, word stress accuracy), temporal (e.g., speech rate, pausing phenomenon), and lexicogrammatical (e.g., grammatical accuracy, lexical appropriateness) measures; this is in contrast to their ratings of accentedness, where they primarily attend to measures of phonology when making judgments (e.g., Crowther et al., Reference Crowther, Trofimovich, Saito and Isaacs2015b, Reference Crowther, Trofimovich, Isaacs and Saito2018; Trofimovich & Isaacs, Reference Trofimovich and Isaacs2012; see Saito’s, Reference Saito2021, meta-analysis). Saito et al. (Reference Saito, Webb, Trofimovich and Isaacs2016) proposed that this difference stems from listeners’ need to make use of all available linguistic information to derive meaning from an utterance when judging comprehensibility. Finally, it has been well-documented that the demands of a given speaking task can influence listeners’ comprehensibility ratings. Comprehensibility of speech elicited using a more cognitively complex speaking task tends to be lower than that elicited using a less complex task, with different profiles of linguistic measures associated with each set of ratings (e.g., Crowther et al., Reference Crowther, Trofimovich, Isaacs and Saito2018).
Acceptability
Beyond comprehensibility (and accentedness), a perceptual dimension that has gained increased attention in L2 speech research is acceptability, though it has yet to reach the same level of interest as other dimensions (Thomson, Reference Thomson, Kang and Ginther2018). A consistent definition of acceptability is elusive. Szpyro-Kozłowska (Reference Szpyro-Kozłowska2014) referred to the “amount of irritation caused by a given accent” (p. 83), while Fayer and Krasinski (Reference Fayer and Krasinski1987) discussed both distraction (i.e., the extent to which the speech diverts attention from the message) and annoyance (i.e., the extent to which the listener experiences a negative, subjective reaction to the speech) as detrimental to acceptability judgments. However, a more appropriate way to view acceptability may be along two common streams of inquiry, as identified in Isaacs (Reference Isaacs2018). The first stream considers the acceptability of L2 speech in reference to a specified norm, often one associated with social power (see Levis, Reference Levis and Hughes2006). As such, a judgment of acceptability necessitates a comparison to what a speaker believes to sound proper, raising questions as to whether acceptability in this sense is distinct from accentedness (Flege, Reference Flege and Winitz1987). We may view this stream as measuring acceptability compared to what. The second stream makes reference to speakers’ acceptability, based on their speech, to meet the performative demands of a given role, such as serving as an ITA at an English-medium university (e.g., Ballard & Winke, Reference Ballard, Winke, Isaacs and Trofimovich2017; Kang, Reference Kang2012). This second stream situates acceptability as a perceptual measure of a speaker’s ability to fulfill a functional role within a given target language use (TLU) domain (e.g., English-medium academic study). Here, the emphasis of measurement is on acceptability for what. In comparison to the first stream, listeners need not access their belief on what sounds proper (though they may still do so), but instead should focus on what they believe is necessary for performance in a given TLU domain. We note that, in reference to both streams discussed above, researchers have used a range of adjectives, including not only “acceptability” (Ballard & Winke, Reference Ballard, Winke, Isaacs and Trofimovich2017; Sewell, Reference Sewell2012), but also “suitability,” “appropriateness” (Prikhodkine, Reference Prikhodkine, Evans, Benson and Stanford2018), and “effectiveness” (Kang, Reference Kang2012; Kang et al., Reference Kang, Rubin and Lindemann2015; Plakans, Reference Plakans1997).
Acceptability and the TLU domain (English-medium academic study)
Acceptability in reference to TLU domain task fulfillment has primarily emphasized English-medium academic study (hereafter academic acceptability, to reflect the TLU domain which these judgments reference). Even within this TLU domain, research has remained primarily constrained to the rating of ITAs by undergraduate students. For example, Kang (Reference Kang2012) asked 70 native and nonnative undergraduates to rate the instructional competence of 11 ITAs based on a 5-minute audio-only excerpt from a course lecture. She found that while ratings of ITAs’ instructional competence were informed by prosodic and fluency measures of speech (e.g., speech rate, pausing), additional variance in ratings came from background variables specific to the undergraduate listeners (e.g., teaching experience, time spent engaging with nonnative speakers). In another example, Dalman and Kang (Reference Dalman and Kang2023) considered the TOEFL iBT elicited speech of 20 high-proficiency English speakers. Despite near ceiling TOEFL iBT performance, 55 native-speaking undergraduates rarely judged speakers as being perfectly comprehensible nor highly acceptable for either university-level teaching or classroom-based group work. Of additional note is that in multiple linear regression analyses, the global dimensions of comprehensibility and accentedness were found to have only a moderate association with listeners’ assessments of acceptability for teaching (adjusted R 2 = 0.47) and acceptability for group work (adjusted R 2 = 0.29), though listeners did prioritize comprehensibility over accentedness when assigning their ratings. A moderate association between comprehensibility and acceptability is similarly seen in Hosoda and Stone-Romero (Reference Hosoda and Stone-Romero2010), who investigated college students’ speech perceptions of French- and Japanese-accented job applicants across four jobs (customer service representative, manager trainee, underwriter, data entry). The correlations between “understandability” and suitability ratings ranged from moderate (r = 0.56) to non-existent (r = 0.00). So, while Thomson (Reference Thomson, Kang and Ginther2018) has proposed that comprehensible speech should be seen as acceptable speech, it appears that such an ideal is not fully substantiated in existing research.
An emphasis primarily on ITAs’ instructional competence overlooks the fact that L2 English users at English-medium universities interact with a much wider range of stakeholders while engaging in a number of different tasks. Non-instructional roles that may occur on any given day may include engaging with a peer (or two or three) during course-based group discussion or post-class/office hours interactions with instructors. Both stakeholder groups (student peers, instructors) likely hold specific expectations regarding what qualifies as acceptable English for these given interactions. Extending inquiry into academic acceptability beyond the instructional competence of ITAs will allow for a more in-depth understanding of what constitutes acceptable language during English-medium academic study, and how such perceptions do, or do not, align with more commonly employed perceptual judgments of L2 speech (i.e., comprehensibility). Examining the influence of speech stream characteristics will also help determine the degree of overlap between academic acceptability and comprehensibility. Aside from Dalman and Kang (Reference Dalman and Kang2023), who examined three fluency-related variables and overall pitch range, we know little about how speech stream characteristics influence acceptability judgments.
Consistencies in speech across encounters
The dimensions of comprehensibility and acceptability are defined and operationalized with respect to listener perceptual judgments of speech. In this sense, it has become common to point out that comprehensibility, as a measure of understanding, is co-constructed in the encounter between speaker and listener (Levis, Reference Levis2005, Reference Levis2020). Listener ratings are a quantification of these encounters. An open question, however, is whether and to what degree underlying characteristics of individual speakers’ speech and listeners’ reactions are consistent across encounters. In other words, can some portion of the dimensions of comprehensibility and acceptability be seen as relatively stable individual traits that have consistent effects across encounters? Methodologically, speaker-listener encounters can be seen as interactions between two types of sampled units, speech samples and listeners, with speech samples being isomorphic to or potentially nested within sampled speakers (Barr, Reference Barr, Rueschemeyer and Gaskell2018). In research on comprehensibility and other listener-based global speech qualities, it is quite common for listeners to react to multiple speakers, and it is in turn common (and uncontroversial) to discuss differences among listeners in terms of judgment severity (e.g., some listeners make more generous judgments than others in general, across speakers). It is less common, though, to see research where (a) multiple speech samples (especially more than 2) are collected from speakers and (b) listener judgments of those samples are analyzed simultaneously, both of which would allow for a more general comprehensibility of speakers, across speech samples, to be described. While limited in number of studies and number of speech samples per speaker analyzed, studies of L2 Korean (Isbell et al., Reference Isbell, Park and Lee2019) and Spanish (Huensch & Nagle, Reference Huensch and Nagle2021) have demonstrated that variation associated with speakers meaningfully accounts for comprehensibility ratings across samples (i.e., by-speaker random effects contributed to conditional variance explained in mixed-effects models).
The current study
The current study draws upon speech elicited through the Duolingo English Test (DET). Despite ongoing debate regarding to what extent the DET truly reflects academic practices (Wagner, Reference Wagner2020), the DET has developed into a commonly used assessment tool for university admissions (https://englishtest.duolingo.com/institutions). In this sense, speech elicited through the DET, though not in situ academic performances, can serve as a barometer of L2 users’ preparedness for study in the TLU domain of English-medium academic study. Using speech samples from admissions tests facilitates the inclusion of individuals with varied first languages (L1s) and lower levels of academic language proficiency, in turn allowing for a more comprehensive examination of the dimensions of interest.
The 204 listeners in the current study represented stakeholders within the TLU domain of English-medium academic study. Each listener judged the comprehensibility and academic acceptability of speech elicited from 200 DET test takers. Test takers’ speech, across four speaking performances, was additionally coded for 13 speech stream characteristics, spanning phonology, complexity, accuracy, and fluency considerations. Through analyses of this data, we set out to investigate the following research questions:
RQ1: What is the relationship between judgments of academic acceptability and comprehensibility, in terms of (a) association between judgments and (b) influences of speech stream characteristics on judgments?
RQ2: To what extent do judgments of academic acceptability and comprehensibility, and the influence of speech stream characteristics, vary across speaking performances?
Method
We adopted a cross-sectional associative design involving speech samples elicited in the DET, a high-stakes English proficiency test, and ratings of speech provided by layperson listeners recruited specifically for the study.
Replication package
Research materials and data that can be made publicly available are accessible at https://osf.io/2ujfa/. Some study materials cannot be publicly shared: Original speech files, provided by Duolingo, and our transcriptions of those files, because of test taker privacy and test security concerns. Some research materials, including rating task instructions, questionnaires, are available in the same package as Online Supplement S1 and Online Supplement S2. Data used in statistical analyses are available in the file accept_comp_open_data.csv (with the codebook available in Comp_Accept_Codebook.csv). For the data we share, we ensured that all test taker information has been anonymized. The format of the “Speaker” variable used in the current study to delineate participants neither resembles nor correlates with any type of test taker identification system used by the DET. Our coding schema cannot be used to identify a given DET test taker. Instructions and code required to reproduce all analyses are available in the files 01_primary analyses.R and 02_primary analyses_no af.R.
Participants
Speakers
Speakers consisted of 100 DET test takers provided by Duolingo for the purposes of this study. The speakers’ language backgrounds reflected DET test taker demographic trends: Mandarin Chinese (n = 30), Arabic (n = 21), Spanish (n = 20), French (n = 14), Persian (n = 12), and English Footnote 1 (n = 3). Speakers reported taking the test for either graduate (N = 49) or undergraduate (N = 51) admissions. Official DET scores provide a standardized measure of speakers’ English proficiency. The 100 speakers had a mean DET score of 108 (out of 160; SD = 17.30, median = 110, range = 70–145), Footnote 2 closely approximating Duolingo’s global test taker score distributions (see Cardwell et al., Reference Cardwell, LaFlair and Settles2022). Figure 1 illustrates the sample distribution of speaker proficiency.

Figure 1. DET score histogram.
Listeners
Listeners included four sets of stakeholders from an English-medium university in the United States. All listeners completed a background questionnaire. Among students, 58 graduate students (34 female; mean age = 30.20, SD = 7.69) and 58 undergraduates (13 female; mean age = 23.00, SD = 5.58) agreed to participate, with a large proportion of each pursuing studies in engineering. A total of 47 faculty members (23 female; mean age = 43.0, SD = 11.00) spread across a range of academic disciplines agreed to participate. Finally, 41 administrative staff (28 female; mean age = 45.2, SD = 11.10) who were mostly involved in academic department support and student services also agreed to participate. The majority of listeners across groups were native users of English (76.6–87.8%), and all listener groups indicated similar levels of familiarity with foreign accents (mean = 5.51–6.26; 1 = not familiar at all, 9 = very familiar).
Materials – speech samples
Each test taker responded to four speaking prompts which comprised the extended speaking portion of the DET: Picture-Speak, Listen-Speak (x2), and Read-Speak, which were completed in a set order (see Table 1). For each DET speaking task type, test takers are directed to speak for at least 30s and may speak for up to 90s. As evident by the task labels, Picture-Speak required test takers to describe a picture, while Listen-Speak and Read-Speak required test takers to respond to an audio or written prompt, respectively. Example prompts for each task type can be found in Official Guide for Test Takers (Duolingo, 2022) and the DET practice test (https://englishtest.duolingo.com; registration required). Given the large item pool developed by the DET (Cardwell et al., Reference Cardwell, LaFlair and Settles2022), a variety of unique Picture-Speak (n = 90), Listen-Speak (n = 142), and Read-Speak (n = 70) prompts were included in our dataset. Each prompt for Listen-Speak and Read-Speak were additionally classified internally by the DET according to a communicative function (i.e., argument, explanation, description); prompts for Picture-Speak all shared a common function. All four speaking performances from each of the 100 speakers were provided by Duolingo (for a total of 400 speech files). Though we did not trim speech files for length (mean = 69.3s, SD = 20.7), we did remove any identifiable information (e.g., full name) and scaled speech files with low intensity to 70 dB.
Table 1. DET extended speaking task types

Linguistic coding
The 400 speech files were first transcribed via Amazon Transcribe (Amazon Web Services, n.d.), with manual corrections performed by the first and third author. A second set of pruned transcriptions was created, with false starts, filled pauses, and repairs removed to facilitate calculation of some complexity and speed fluency measures. Each speech file was then coded to derive a set of pronunciation, accuracy, complexity, and fluency measures (N = 13). Given that a vast array of speech measures have been shown to associate with L2 speech ratings to some extent, we have admittedly included only a small handful of measures, chosen for theoretical, analytical, and practical reasons. Theoretically, we drew upon Suzuki and Kormos (Reference Suzuki and Kormos2020), who considered a range of speech measures as predictors of listener ratings of comprehensibility and perceived fluency, as an initial guide to identify potential measures to include in our analyses. Analytically, to guard against overfitting and maintain interpretability, we limited the number of predictors included. This meant that several measures included in a preliminary analysis were removed (see descriptions below). Finally, given the volume of speech coded (>400 minutes, cf. ∼99 minutes in Suzuki & Kormos, Reference Suzuki and Kormos2020, and 24 minutes in Trofimovich & Isaacs, Reference Trofimovich and Isaacs2012), certain coding decisions were made to best make use of the available time of the research team members.
Pronunciation
Following Suzuki and Kormos (Reference Suzuki and Kormos2020), we included three measures of pronunciation: substitution rate, syllable structure error rate, and word stress error rate. Due to our extensive number of samples, we coded both substitution rate and syllable structure error rate at the word level, rather than the phoneme or syllable level. With this analytical choice, we sought to strike a balance between efficiency and fidelity for a relatively large amount of elicited speech, falling somewhere between previous approaches involving subjective scalar ratings of overall segmental quality (e.g., Crowther et al., Reference Crowther, Trofimovich, Isaacs and Saito2015a) and analytic coding at the level of individual segments (e.g., Trofimovich & Isaacs, Reference Trofimovich and Isaacs2012). This decision was also informed by Munro and Derwing (Reference Munro and Derwing2006), which found that for sentence-length utterances the presence of a single phonemic substitution had a notable effect on perceptions of comprehensibility, but additional errors had less impact. Though coding at the word level may not capture the total number of errors that speakers may produce, it can still reveal a general pattern of errors, as we would expect a given speaker to make relatively similar errors throughout their speech. We also note that while Suzuki and Kormos (Reference Suzuki and Kormos2020) included a measure of speech rhythm in their study, this measure was not predictive of either comprehensibility or perceived fluency in multiple regression analyses. Combined with the extensive time such coding would have required, we do not include rhythm as a measure in the current analyses.
-
1. Substitution rate: number of words with a phonemic substitution divided by total number of words produced;
-
2. Syllable structure error rate: number of words with an added/deleted syllable divided by total number of words produced;
-
3. Word stress error rate: number of polysyllabic words with missing/misplaced primary stress divided by total number of words produced.
The first and second authors initially manually coded 10% of all samples for all three measures (see Table 2). Overall percent agreement was high (≥94%) and Gwet’s AC1, a measure of inter-rater reliability argued to be more stable than either Pi (π) or kappa (κ) in the presence of high agreement and/or high prevalence (Gwet, Reference Gwet2008), similarly indicated high inter-rater reliability for each of substitution rate (AC1 = .94), syllable structure error rate (AC1 = .98), and word stress error rate (AC1 = .99). Footnote 3 Both authors subsequently collaboratively reviewed all files to identify and resolve all discrepancies in coding before the first author coded the remaining files.
Table 2. Intercoder agreement for hand-coded speech variables

Accuracy
Following Suzuki and Kormos (Reference Suzuki and Kormos2020), we included three measures of accuracy.
-
4. Lexical error rate: number of words deemed inappropriate for the context, malformed, or drawn from the speaker’s first language divided by the total number of words produced (excluding nonlinguistic filler);
-
5. Morphological error rate: number of words containing a deviation from standard academic English divided by total number of words produced (excluding nonlinguistic filler). Examples of deviations included errors in plural marking, subject-verb agreement, pronoun choice, and articles/determiners;
-
6. Syntactic error rate: number of clauses containing a deviation from standard academic English divided by total number of clauses produced. Examples of deviations included word order violations, missing words/constituents, misapplication of tense or aspect, and subordination errors.
As with the pronunciation measures, the first and second authors manually coded 10% of the speech files for all three measures, with percent agreement again high (≥91%; see Table 2). Gwet’s AC1 indicated high inter-rater reliability for lexical error rate (AC1 = .97), morphological error rate (AC1 = .98), and syntactic error rate (AC1 = .89). After reviewing all files to resolve discrepancies in coding, the second author completed all remaining coding.
Complexity
In Suzuki and Kormos (Reference Suzuki and Kormos2020), two measures of syntactic complexity, mean length of AS-units and mean number of clauses per AS-unit, were found to associate strongly with comprehensibility (rs > .60). We chose to include only one, Clauses/AS-unit, as it more directly addresses the notion of syntactic complexity in spoken language (Biber et al., Reference Biber, Gray and Poonpon2011). For lexical complexity, we drew on two measures similar to those used in Suzuki and Kormos (Reference Suzuki and Kormos2020) that capture two aspects of lexical complexity: lexical sophistication and lexical diversity.
-
7. Clauses/AS-unit: number of clauses divided by number of AS-units per sample. An AS-unit was defined as “a single speaker’s utterance consisting of an independent clause, or sub-clausal unit, together with any subordinate clause(s) associated with either” (Foster et al., Reference Foster, Tonkyn and Wigglesworth2000, p. 365). For example, in the single AS-unit the boy saw that the girl was holding a bag, there are a total of 2 clauses (the boy saw ___, the girl was holding a bag). Both measures were manually coded by the third author, and verified by the second author during the coding of accuracy measures. In total, only 9.5% of files (N = 38) required adjustment.
-
8. COCA spoken mean log frequency: A log-transformed measure of average word frequency, based on the raw tokens of all words in a corpus. Calculated using the Tool for the Automatic Analysis of Lexical Sophistication (TAALES; Kyle et al., Reference Kyle, Crossley and Berger2018);
-
9. Measure of textual lexical diversity (MTLD): A measure of the range of words used in a given sample, calculated “as the mean length of sequential word strings in a text that maintain a given TTR [type-token ratio] value” (McCarthy & Jarvis, Reference McCarthy and Jarvis2010; p. 384). Measured using the Tool for the Automatic Analysis of Lexical Diversity (TAALED; Kyle et al., Reference Kyle, Crossley and Jarvis2021).
Fluency
Following Suzuki and Kormos (Reference Suzuki and Kormos2020), we included measures of speed, breakdown, and repair fluency. Though we began with three measures of speed fluency, preliminary analyses indicated high correlations (rs > .83) between speech rate, articulation rate, and mean length of run. As such, we include only one measure, speech rate, in our current analyses.Footnote 4 Though Suzuki and Kormos (Reference Suzuki and Kormos2020) included five measures of breakdown fluency, as previously stated, we wished to keep our overall number of speech stream measures down in order to allow for greater model interpretability and guard against overfitting. As such, we included only two measures of breakdown fluency, filled pauses per minute and mid-clause silent pauses per minute. A focus on mid-clause, rather than end clause, pauses was chosen, since it was silent pauses in this location that predicted both comprehensibility and perceived fluency ratings in Suzuki and Kormos (Reference Suzuki and Kormos2020). Finally, we included a composite measure of repair fluency. All fluency measures were frequency, as opposed to duration, based.
-
10. Speech rate: number of syllables divided by total duration of sample;
-
11. Mid-clause silent pauses/minute: number of silent pauses occurring mid-clause divided by 60 seconds;
-
12. Filled pauses/minute: number of filled pauses (e.g., uh, um) divided by 60 seconds;
-
13. Repairs/minute: number of repairs divided by 60 seconds. Repairs included self-corrections, false starts and reformulations, and partial or complete repetitions.
Fluency coding was conducted by the third author, who made use of both hand coding and automated coding of pauses using Praat (Boersma & Weenink, Reference Boersma and Weenink2022). For speech rate and articulation rate, number of syllables was calculated automatically using the pruned transcripts (i.e., syllable counts did not include filled pauses, false starts, and hesitations). Following standard conventions (e.g., Suzuki & Kormos, Reference Suzuki and Kormos2020), silent pauses consisted of any silence ≥250ms and were automatically identified using de Jong and Wempe’s (Reference de Jong and Wempe2009) Praat script. All silence boundaries, initially identified using Praat, were then manually reviewed and adjusted as needed, with filled pauses and pause location additionally coded (based on analyses of unpruned transcripts). Repair measures were derived from those items removed for the pruned transcripts.
Listener procedures and judgment scales
Listeners received an email invitation to complete an online experiment implemented in Gorilla (Anwyl-Irvine et al., Reference Anwyl-Irvine, Massonnié, Flitton, Kirkham and Evershed2020), which they could access at home on a personal computer. Given the large number of speech samples, our experiment utilized a sparse rating design (see Isbell, Reference Isbell, Kang and Ginther2018). We divided the 400 DET speech samples into 40 blocks of 10 files each, with each block composed of speakers representing at least four different L1 backgrounds and roughly one speaker per DET score decile. At least two different speaking task types (among Picture, Listen-Speak, and Read-Speak) were represented in each block, with most blocks featuring all three. Each of a speaker’s four speaking performances was assigned to different blocks, which were then divided into two sets, A and B. Sets were designed to ensure no speaker had a response in both sets. Each listener was assigned to one block from Set A and one block from Set B, with half of the listeners starting with a block from Set A and vice versa. As such, no listener heard the same speaker more than once. Ultimately, due to randomization and a technical error related to block composition that was remedied partway through data collection (see Online Supplement S2 for details on block assignment and composition), a varied number of listener ratings for each of the 400 audio files were collected, with a mean of 10.40 per file (SD = 7.03, min = 2, max = 37).
Listeners made several judgments about files related to comprehensibility and academic acceptability. For comprehensibility, listeners provided three judgments using 6-point scales drawn from Schmidgall and Powers (Reference Schmidgall and Powers2021), with prompts as follows:
-
How certain are you that you understood the speaker?
-
How easy was it to understand the speaker?
-
How comprehensible was the speaker?
Though less common than the use of a single scale, the use of a multi-item scale for comprehensibility is not unheard of. For example, Kang et al. (Reference Kang, Rubin and Pickering2010) made use of a five-item scale (easy/hard to understand, incomprehensible/highly comprehensible, needed little effort/lots of effort to understand, unclear/clear, and simple/difficult to grasp the meaning), with reliability across items high (Cronbach α = .94). In Isbell et al. (Reference Isbell, Crowther and Nishizawa2023), we investigated the relationship between stakeholders’ speech perceptions and DET speaking performance using the same speech samples analyzed here. As Schmidgall and Powers’ (Reference Schmidgall and Powers2021) reported a similar analysis focused on TOEIC speaking performance, we made use of their three-item scale as a measure of comprehensibility.
Listeners subsequently provided four judgments on 6-point scales that targeted the acceptability of a given speaker’s English across different academic roles. Given that acceptability is a less well-established dimension in the literature, we drew inspiration from several previous studies. The first acceptability item targeted acceptability for university teaching, as this has been the primary focus of academic acceptability research (e.g., Dalman & Kang, Reference Dalman and Kang2023; Kang, Reference Kang2012). The second item, following Dalman and Kang (Reference Dalman and Kang2023), focused on group work in classes. The final two items, acceptability for undergraduate and graduate study, were included since the DET serves as a tool for assessing academic preparedness for university study. The four final prompts were as follows:
-
How acceptable would this speaker’s English be for undergraduate studies?
-
How effective would this speaker’s English be for group work in classes?
-
How acceptable would this speaker’s English be for graduate studies?
-
How suitable would this speaker’s English be for university teaching?
Figure 2 illustrates how these judgment scales were displayed in the online experiment platform Gorilla, which includes the descriptors for each point of the seven scales. Listeners received training on each target dimension and completed two practice ratings prior to beginning the experiment. Two attention checks, which required listeners to click on a specified rating on three rating scales, were included in the experiment.
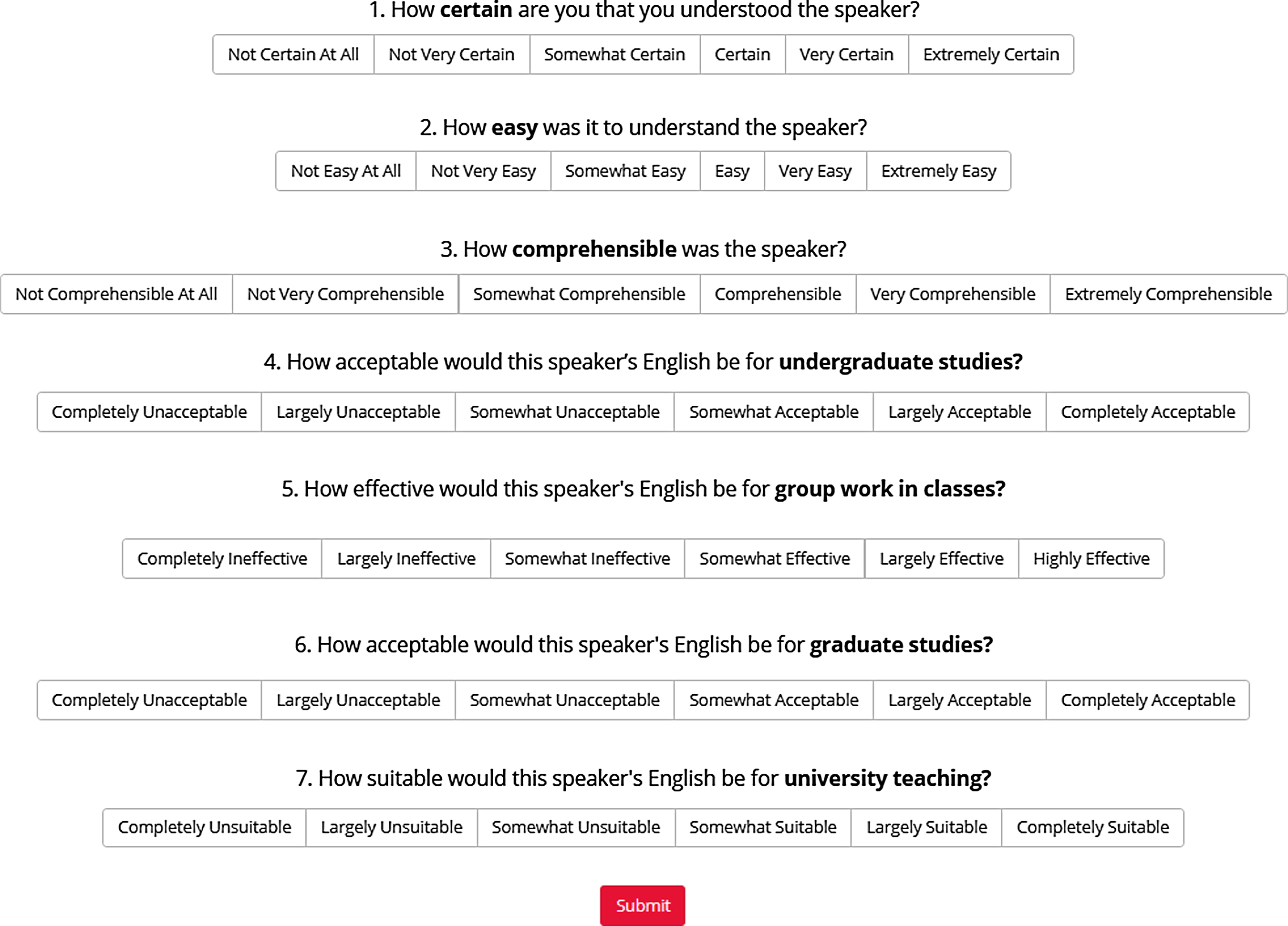
Figure 2. Speech judgment questions and interface.
To get a sense of how well the judgment items worked together as measures of the overarching construct, we aggregated judgments across listeners for each file and examined inter-item correlations (Table 3). The items for each scale were highly intercorrelated, indicating coherence of the dimensions being measured. Though the strongest correlations among items were generally those within the same scale (e.g., the correlations among the three comprehensibility items), it is also worth noting the high correlations among items across the two scales; we return to the relationship between comprehensibility and acceptability later in the manuscript. Additionally, in Isbell et al. (Reference Isbell, Crowther and Nishizawa2023), many-facet Rasch models yielded strong evidence of unidimensional measurement and good fit of judgment items for both comprehensibility and acceptability.
Table 3. Pearson’s correlations among comprehensibility and acceptability items (scores averaged across listeners)

Note. N = 400 speech files, all p-values < .001.
Reliability of each judgment scale and for listener averages across all scales for comprehensibility and acceptability were estimated using 2-way random effects intraclass correlations (ICC, McGraw & Wong, Reference McGraw and Wong1996) using the irrNA package (v.0.2.2, Brueckl & Heuer, Reference Brueckl and Heuer2021) in R to accommodate missingness in a sparse rating design. As shown in Table 4, absolute agreement among listeners’ single scores was low (.27 – .34), indicating variability in listener scale use (i.e., some listeners were more lenient in judgments while others were stricter, see Isbell et al., Reference Isbell, Crowther and Nishizawa2023). However, the degree of consistency for average ratings from k randomly selected raters was considerably higher (.79 – .84). Our primary analyses are based on the average of all judgments for each dimension from each listener, and the ICCs for raters’ aggregated comprehensibility and acceptability scores were both .84 and .85, respectively.
Table 4. Intraclass correlations of speech judgments

Note. A, 1 = agreement of single scores assigned to a file. C, k = consistency of scores averaged across raters for files (equivalent to Cronbach’s α, see McGraw & Wong, Reference McGraw and Wong1996).
Analyses
An initial check of listener judgment quality appeared satisfactory, suggesting that most listeners were paying attention throughout the experiment. Overall, 1160/1230 attention checks were passed (94%); 192 listeners responded correctly to 4/6 checks (94%), and 176 of these listeners responded correctly to every attention check (86%). Of concern, however, were 12 listeners who answered ≤3/6 attention checks correctly. A closer inspection revealed no clear aberrant response patterns (e.g., uniform responses, such as selecting the most positive category for all judgments). Parallel analyses to those presented below without these 12 listeners differed little (see Online Supplement S3: Tables S3.1–S3.5). As such, we report our analyses inclusive of all 204 listeners but make notes of minor differences when relevant.
Based on Isbell et al. (Reference Isbell, Crowther and Nishizawa2023), only minor differences existed between academic listening groups (undergraduate students, graduates, faculty, and administrative staff) in responses to judgment questions. While faculty tended to be most lenient in their ratings, and administrative staff most harsh, the magnitudes of differences between group average scores were small (less than half a point in most cases). Importantly, the four groups used the scales similarly, and assessed both comprehensibility (certainty > comprehensibility > ease) and acceptability (undergraduate > group work > graduate > teaching) items following the same hierarchy. As such, and in line with our research questions in the present study, we treated listeners as a single group and made use of composite scores from each listener, averaged across judgment questions, as dependent variables for both comprehensibility and acceptability models.
For all analyses involving inferential statistics, we adopted an alpha of p < .05. When interpreting the magnitudes of correlations, we drew on Plonsky and Oswald’s (Reference Plonsky and Oswald2014) guidelines (small > .25, medium > .40, and large > .60). For mixed-effects models, we considered the standardized coefficient estimates, overall model R 2 (including marginal and conditional, with Plonsky & Ghanbar’s, Reference Plonsky and Ghanbar2018, guidelines informing interpretation of the former), and furthermore considered the degree to which speech stream fixed effects reduced the amount of random intercept variation associated with speakers (i.e., the degree to which Level 1 fixed effects reduced Level 2 random effect variation compared to a “null” random effects only model; Bryk & Raudenbush, Reference Bryk and Raudenbush1992). To check assumptions of linear mixed-effects regression models, we examined bivariate correlations among predictors to screen for collinearity and plotted model residuals (histograms, Q-Q plots) to assess normality.
Results
Descriptive statistics for study variables are provided in Table 5. These descriptive statistics are aggregated across all 400 spoken performances, with listener judgments aggregated across rating questions and listeners (descriptive statistics for each of the 4 task types are available in Online Supplement S4). The ICC characterizes how similar values of each variable were across speakers’ sets of performances. Notably, averaged listener judgments associated with a speaker were moderately similar across performances, as were segmental pronunciation characteristics (phonemic substitutions and syllable structure errors) and disfluencies (mid-clause pauses and repairs/repetitions). The speech stream characteristics most similar within speakers across all performances were speech rate and filled pauses.
Table 5. Summary of speech variables

Note. N = 400 speaking performances.
* ICC across all 4 speaking tasks.
† Based on the average of all items and all raters.
Figure 3 further illustrates the distribution of study variables (density plots on the diagonal) and presents bivariate scatterplot and Pearson correlation coefficients for all pairs of study variables. The averaged listener judgments of comprehensibility and acceptability were strongly correlated at r = .93, and several speech stream variables demonstrated moderate to large correlations with each judgment. Notably, speech stream variables tended to have similar correlations with both listener judgments. Correlations among speech stream variables were mostly small.

Figure 3. Correlations and distributions of study variables.
Linear mixed-effect regression models were used to examine the influence of speech stream characteristics on listener judgments for each speech performance. The dependent variable in each model, comprehensibility or acceptability, was the average of a single listener’s ratings for all questions (3 comprehensibility questions or 4 acceptability questions). Fixed effect predictors included functional demands of each speaking task type, pronunciation variables, and complexity, accuracy, and fluency indices. While the full model with all fixed effects was of primary interest, intermediate models that added each group of variables were run to examine changes in variance explained.
Random intercepts were included for speakers, to account for the nesting of spoken performances and judgments, and for listeners, to account for the nesting of judgments within each listener. In more substantive terms, the by-listener random effects account for differing levels of judgment severity. Attempts to include random slopes to account for possible variation across listeners in the influence of speech stream characteristics were unsuccessful, as the inclusion of more than a single random slope (uncorrelated with any intercept) resulted in singular fits or nonconvergence.
Model results are shown in Table 6. As indicated by R 2 values, fixed effects explained relatively little variation in judgments for both models while a substantial amount of variation (∼40–45%) was accounted for by-speaker and listener random intercepts. In other words, differences among speaker ability and listener severity were the most powerful influences on judgments across speaking performances. Speaker and listener random intercepts were also highly correlated across the two models, r speakers = .96 (p < .001) and r listeners = .85 (p < .001).
Table 6. Linear mixed-effect model results for comprehensibility and acceptability

Note. The ΔR 2 column represents changes in marginal R 2. Boldface indicates p-values <0.05.
Closer inspection of speaker random effects and task-level fixed effects indicated that several fixed effects had noteworthy explanatory power. Compared to intercept-only models (with no fixed effects), speaker random intercept variation in final models was reduced by 56% for comprehensibility and 65% for acceptability. Thus, Level 1 fixed effect variables associated with spoken performances could account for considerable amounts of variation in Level 2 random effects associated with speakers. Fixed effects influences on judgments were similar across both models, with speech rate, all lexicogrammatical accuracy variables, and phonemic substitutions having statistically significant standardized coefficients of comparable size. Most of these statistically significant coefficients were small and negative (as expected for error rates), though the magnitude of the speech rate coefficient was positive and notably larger. Syllable structure error rate was only statistically significant in the acceptability model. These results are largely consistent with models excluding listeners who passed fewer than 4/6 attention checks (see Online Supplement S3: Table S3.2), but with a lack of statistical significance for substitution rate (p = .05) in both models (coefficient magnitudes were nearly identical).
Listener perceptions by speaking task type
To examine how speech stream characteristics might influence listener judgments differently across speaking task types, we conducted linear mixed-effect model analyses separately for each task type: Listen-Speak 1, Picture-Speak, Read-Speak, Listen-Speak 2. In all task-specific models, random intercepts were included for speakers and listeners. As before, we were primarily interested in models with all relevant predictors, but intermediate models were constructed to investigate the incremental contribution of communicative function (except for Picture-Speak, for which communicative function is uniform), pronunciation, complexity, accuracy, and fluency variables.
Table 7 provides a summary of the task-specific models for comprehensibility and acceptability (full results tables, including all coefficients, are available in Online Supplement S4: Appendix B and C). Across all task types, speaker and listener random intercepts, accounted for in the conditional R 2 values, explained more variation in judgments (34–43%) than fixed effects (14–21%). In intercept-only models, the amount of variation in judgments explained by speakers and listeners was greater for acceptability than comprehensibility. For Read-Speak and Listen-Speak 1 and Listen-Speak 2, functional demands associated with prompts accounted for little variance explained in either listener judgment. Speech stream variables accounted for the greatest amount of variation in judgment for the two Listen-Speak performances. The complexity variables appeared to account for more variance in judgments for both Listen-Speak performances than for Picture-Speak and Read-Speak. Notably, the inclusion of fixed effects substantially reduced the amount of variation associated with speaker random effects (τS). Most drastically, the speaker random effect variance was reduced to .03 in the Listen-Speak 2 model for acceptability, down nearly 90% from the intercept-only model value of .24. The other acceptability and comprehensibility models with all variables reduced speaker random variation by 50–75%.
Table 7. Variance explained (R2) and speaker variance for task-specific comprehensibility and acceptability models
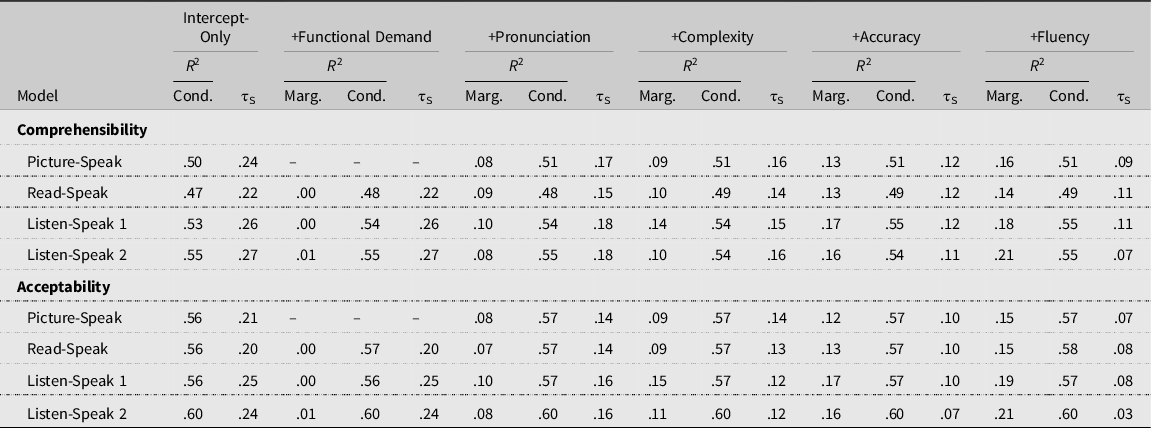
Note. +Fluency corresponds to the final model with all fixed effects predictors added.
Marg. = Marginal R 2.
Cond. = Conditional R 2.
τS = Speaker random effect variance.
Looking at specific speech stream predictors, Fig. 4 compares the magnitudes of standardized coefficients across speakers’ performances for comprehensibility and acceptability, with error bars indicating 95% confidence intervals. Speech rate was clearly the most influential and consistent predictor across task types and judgments, with positive, statistically significant values in all models. Lexical error rate also had fairly consistent negative effects across task types and judgments, though not statistically significant in every model. Conversely, some predictors were consistently near zero in magnitude and failed to achieve statistical significance across task types and judgments, including word stress error rate, clauses per AS-unit, MTLD, and all fluency variables aside from speech rate.

Figure 4. Coefficient plot for task-specific judgment models.
Other predictors showed similar magnitudes across judgments, but differed by task type. Substitution rate showed larger effects for both comprehensibility and acceptability in Picture-Speak and Listen-Speak 1, though it was only statistically significant as a predictor of comprehensibility in Listen-Speak 1. Syllable structure error rate had no impact in Picture-Speak, but had notable negative effects in Read-Speak and both Listen-Speak performances for both judgments. Morphosyntactic accuracy predictors also differed by task type. Morphological error rate was a larger negative predictor on Listen-Speak 2 (and a similar trend was seen in Listen-Speak 1), but had no large or reliable effect in Picture-Speak or Read-Speak. Conversely, syntactic error rate was a statistically significant and negative predictor in the Picture-Read and Read-Speak tasks for both judgments, but had smaller, nonsignificant effects in the two Listen-Speak performances.
With regard to differences when listeners who passed fewer than 4/6 attention checks were excluded from analyses (see Online Supplement S3: Tables S3.3–S3.5), total amounts of variance accounted for (model R 2 and reductions in speaker random effect variance) were nearly identical. The magnitudes of fixed effect coefficients were also largely similar, but there was one instance where a speech stream predictor failed to achieve statistical significance: substitution rate in the comprehensibility model for Listen-Speak 1.
Discussion
We sought to understand in more depth the relationship between listeners’ perceptions of the well-researched speech dimension of comprehensibility and the less-understood dimension of acceptability. Importantly, acceptability in this study was conceptualized as listeners’ perception of a speaker’s ability to perform a specific role within a specific TLU domain (i.e., English-medium academic study, or academic acceptability). To shed light on this relationship, we not only considered the association between the two dimensions, but the linguistic profile of speech stream characteristics that appeared to influence listeners’ judgments of each. This relationship was explored across four speaking performances per speaker, allowing for consideration of speakers’ consistency across performances. We discuss the results of the study with respect to our two research questions, before concluding with additional considerations for the dimension of acceptability in L2 speech.
RQ1: the relationship between judgments of academic acceptability and comprehensibility
Given the strong association between the two dimensions (r = .93), and the similar speech stream profiles for each, it would seem that our findings constitute support for the idea that acceptability can be (almost) indistinguishable from comprehensibility, at least for this population of listeners judging speech in reference to particular TLU domain needs. The only speech stream characteristic which appeared to differ in the multi-task comprehensibility and acceptability models was syllable structure error rate, which had a statistically significant but small negative effect on acceptability judgments but nearly no effect on comprehensibility judgments. Nonetheless, the bivariate correlations between syllable structure error rate and comprehensibility and acceptability were similar in magnitude (r = −.32, r = −.35, respectively), which calls into question the meaningfulness of this difference.
Compared to previous studies on speech stream predictors of L2 English comprehensibility, the overall amount of variance accounted for by speech stream-based fixed effects in this study appears somewhat low on the surface. In the general models of comprehensibility and acceptability, inclusive of all four speaking performances, speech stream fixed effects accounted for only 8–10% of total variance in judgments. These amounts are comparable in magnitude to Dalman and Kang (Reference Dalman and Kang2023), which found adjusted R 2 values ranging from .04 to .08 for linear regression models with four predictors for comprehensibility and acceptability. In comparison, and towards the other extreme, a recent study by Suzuki and Kormos (Reference Suzuki and Kormos2020) found that a handful (5) of speech stream variables could explain over 92% of the variance in comprehensibility judgments made on argumentative speech elicited from 40 L1 Japanese speakers of English (see also R 2 values of .74–.87 in Crowther et al., Reference Crowther, Trofimovich, Isaacs and Saito2015a, Reference Crowther, Trofimovich, Isaacs and Saito2018; .50 in Kang et al., Reference Kang, Rubin and Pickering2010; .86 in Trofimovich & Isaacs, Reference Trofimovich and Isaacs2012, etc.). We note, however, that fixed effects in this study did explain a substantial amount of variation in by-speaker random intercepts, by 56–65% in the multi-task models. We elaborate on this finding shortly.
RQ2: variation in judgments of academic acceptability, comprehensibility, and speech stream characteristics across speaking task types
The overall effects of speaking task type on listener judgments were minimal. Average judgments of comprehensibility across task types ranged between 3.92 and 4.01 and average judgments of acceptability between 4.11 and 4.23. In terms of speech stream characteristics, there were also some broad similarities across task types and dimensions, namely, speech rate and lexical errors had fairly consistent influence on judgments and several other measures consistently had null effects. However, there were several interesting differences in speech stream influences across task types. For one, more variance overall was explained by speech stream fixed effects in both Listen-Speak performances for both dimensions. Furthermore, syllable structure errors had statistically significant effects on Read-Speak and both Listen-Speak performances, but not Picture-Speak. While we did not have access to the prompts speakers responded to (but see the example item in the Official Guide for Test Takers, Duolingo, 2022), it may be the case that the pictures used were less likely to elicit multisyllabic words in the first place. There was also an interesting dynamic where morphological errors had more pronounced influence on the Listen-Speak performances while syntactic errors had more influence on Picture-Speak and Read-Listen. While this finding is more difficult to explain and interpret, it nonetheless suggests that task types have some influence on speaker performance and/or ensuing listener judgments. These subtle differences are contrasted by studies such as Crowther et al. (Reference Crowther, Trofimovich, Isaacs and Saito2015a) and Crowther et al. (Reference Crowther, Trofimovich, Isaacs and Saito2018), where more distinct profiles of speech stream influences on comprehensibility emerged in tasks that varied in complexity. In both studies, lexicogrammatical measures appeared more influential for a more complex integrated task, in which speakers were required to employ greater reasoning and perspective-taking, versus less complex long turn and picture narration tasks. However, we note that whereas both Crowther et al. (Reference Crowther, Trofimovich, Isaacs and Saito2015a) and Crowther et al. (Reference Crowther, Trofimovich, Isaacs and Saito2018) compared distinctly different tasks, the tasks included in the current study might be considered more similar than they were different, with the exception of the picture description task. Both Read-Speak and Listen-Speak required speakers to respond to a prompt, with the only task differences being the modality of prompt delivery and the availability of the reading prompt during speakers’ response. As such, the largely similar profiles of speech stream influences may be due to speakers drawing on similar processes to respond to prompts.
A key characteristic of the current study was that, given the source of speech elicited (high-stakes English proficiency test [i.e., DET]), it was possible to include speakers representing a wide range of linguistic backgrounds and overall proficiency. That is, our population included both those who would and those who would not receive admission into English-medium university study (see Isbell et al., Reference Isbell, Crowther and Nishizawa2023), ranging in proficiency from roughly CEFR levels B1 to C1. This is in contrast to many existing studies, where the speaker population was more homogenous. For example, Suzuki and Kormos (Reference Suzuki and Kormos2020) included only L1 Japanese speakers of English, with most at the CEFR B1-B2 proficiency level. While Crowther et al. (Reference Crowther, Trofimovich, Isaacs and Saito2015a, Reference Crowther, Trofimovich, Isaacs and Saito2018) did include speakers from several different L1s, they had all achieved at minimum a proficiency level high enough to allow for undergraduate study at an English-medium university. As a final point of comparison, while speakers in the current study all completed the same battery of DET speaking tasks, the prompts they completed were drawn from a large item pool, minimizing the potential effects of prompt familiarity on listeners’ judgments. Given the earlier referenced differences in variance explained with previous speech rating research, from the listener’s perspective, speech stream characteristics may have a larger influence on judgments when repeatedly hearing speech elicited using the same prompt delivered by speakers of a common L1 background and similar range of proficiency. With a uniform prompt, the topic, content, and even some linguistic features (e.g., topical vocabulary, grammar structures, discourse markers) of responses may overlap more from speaker to speaker. This similarity may reduce listener cognitive efforts on comprehending meaning and allow for closer attention to fluency, disfluencies, and phonological form when judging such speech.
Alignment across speech performances
One notable contribution of this study is the examination of L2 speech dimensions based on listener judgments (comprehensibility, acceptability) as attributes of speakers that are generalizable across several speaking performances. Before accounting for speech stream characteristics, comprehensibility and acceptability scores showed moderate levels of consistency within speakers across performances (i.e., ICC values). We also observed notable consistencies of several speech stream characteristics within speakers, particularly related to fluency and segmental pronunciation phenomena, while other speech characteristics showed more within-speaker variation across performances, like lexicogrammatical accuracy and complexity. Accounting for these features as fixed effects in models showed how characteristics of individual spoken performances systematically explained variance in judgment outcomes within and across speakers, as inclusion of such variables as fixed effects reduced the total amount of speaker random intercept variation in the all-task general models (by 56–65%) and task-specific models (by 50–90%). In other words, both consistent and less consistent aspects of speaker performance have roles to play in determining the outcomes of speaker-listener encounters.
Of course, not all variation in judgments was explained. Remaining random variation associated with speakers may be accounted for by speech stream characteristics and other variables we did not include in this study, such as suprasegmental measures related to prosody (e.g., Trofimovich & Isaacs, Reference Trofimovich and Isaacs2012), discourse features (Suzuki & Kormos, Reference Suzuki and Kormos2020) or collocation use (Saito, Reference Saito2020; Saito & Liu, Reference Saito and Liu2022). Similarly, the substantial variation associated with listeners could also likely be explained by a number of factors, such as L1/L2 status of English, academic role, experience interacting with L2 English speakers, and so on (e.g., Isaacs & Thomson, Reference Isaacs and Thomson2013; O’Brien, Reference O’Brien2016). Variation unattributed to speakers and listeners is likely to be accounted for, in part, by differences in specific prompts.
Limitations
Due to the nature of the sparse rating design we employed (i.e., not all listeners rated all speech samples), we were limited in our ability to account for the potential effect of listener variables such as L1/L2 status or accent familiarity, both of which have been found to influence listener perception of global speech dimensions (e.g., Ballard & Winke, Reference Ballard, Winke, Isaacs and Trofimovich2017; Crowther et al., Reference Crowther, Trofimovich and Isaacs2016). As referenced, in Isbell et al. (Reference Isbell, Crowther and Nishizawa2023), the four listener groups (undergraduates, graduate students, faculty, staff), as a whole, tended to rate similarly across speech dimensions, which may help to alleviate concerns regarding such individual differences, though how such differences may influence ratings of academic acceptability should be considered in future research. An additional methodological limitation is that the simultaneous judging of comprehensibility and acceptability may have inflated the similarity of those judgments. Though O’Brien (Reference O’Brien2016) found that the rating of three perceptual dimensions (accentedness, comprehensibility, fluency) separately versus simultaneously resulted in only minor differences in listener judgments, the high association between aggregated comprehensibility and acceptability judgments (r = .93) found here may suggest that reconsideration and/or further investigation might be necessary. Finally, we acknowledge that the choice of linguistic measures, and the way in which these measures are coded, is variable across studies. Decisions made for the current study were made drawing upon existing research, but also simultaneously accounting for the need to code over 400 minutes of speech data. Refinement of our linguistic coding procedures could potentially lead to variations in our findings.
Conclusion
Findings pertaining to the association between comprehensibility and acceptability and the patterning of speech stream characteristics that influenced judgments of each construct support the idea that acceptability can be (almost) indistinguishable from comprehensibility (Thomson, Reference Thomson, Kang and Ginther2018). This finding, of course, comes with a pair of caveats. For one, the emphasis here was on academic acceptability, where elicited ratings were specific to the academic items presented to listeners. The same speech, presented for judgment to stakeholders representing different TLU domains (e.g., business, tourism), may be viewed quite differently. Any interpretation of acceptability, as operationalized in the current study, should remain within the specific TLU domain of interest. Second, the speech elicited was not representative of in situ language use. Thus, listeners’ judgments were not based on speech representative of actual academic production. This leaves a question regarding to what extent “acceptable” speech, as deemed in the current study, would predict success in the actual academic domain (see, for example, Bridgeman et al., Reference Bridgeman, Powers, Stone and Mollaun2012, or Schmidgall & Powers, Reference Schmidgall and Powers2021). Yet, despite such concerns, judgments across listeners were found to be highly reliable. As all listeners were stakeholders within the TLU domain of interest, this would provide support for a claim that even if speech may not be representative of actual in situ performance, stakeholders with knowledge of the TLU domain share, at least to some extent, a degree of criteria for what constitutes acceptable speech for a given academic role. An additional finding of interest, and one seemingly less explored in L2 speech research, was that speakers’ productions generated moderately similar listener perceptions across performances. Whereas prior studies (e.g., Crowther et al., Reference Crowther, Trofimovich, Isaacs and Saito2015a, Reference Crowther, Trofimovich, Isaacs and Saito2018) have emphasized how judgments of speech and linguistic/temporal influences on those judgments differ across tasks, we here highlight how consistencies in the characteristics of speakers’ performances, and presumably underlying competence, are identifiable and also play a role in predicting individual outcomes of listener judgments. Further research into these cross-task consistencies will greatly enhance our understanding of L2 speaking ability and what determines how listeners make judgments of comprehensibility and acceptability.
Data availability statement
All raw data/materials from this study, which includes official Duolingo English Test speaking performances and transcriptions, are not available. Data used in statistical analyses are available.
Replication package
Research materials and data that can be made publicly available are accessible at https://osf.io/2ujfa/.
Acknowledgments
We would like to thank Duolingo English Test personnel for reading and commenting on earlier versions of this article to verify our description of test content and procedures. As all data collection and analysis described in this study were conducted independently, any and all remaining errors are our own.
Funding statement
Funding from Duolingo awarded to Daniel R. Isbell and Dustin Crowther supported this study.
Competing interests
The authors have no conflicts of interest to disclose.
Ethics approval statement
This study was conducted in accordance with Applied Psycholinguistics informed consent guidelines. Study protocols were reviewed and approved by the University of Hawai‘i Office of Research Compliance Human Studies Program (Protocol ID 2020-00996).