For a large number of adolescents, attending college is a time of transition when they are first learning to manage their own energy intake and expenditure without parental oversight. In the USA, many students obtain their meals on campus, where, in the typical ‘all you can eat’ dining hall set-up, they are confronted with a wide array of food choices without any limitation on options or portion sizes. This set-up can also be found in other developed countries such as Canada and Australia, although most schools offer multiple dining options. Not surprisingly, researchers have documented a consistent weight gain in first-year undergraduates averaging 1–4 kg, such that a substantially greater proportion of freshman in several developed countries (e.g. Canada, the UK, Belgium and the USA) are classified as overweight at the end of the first semester compared with the beginning( Reference Deforche, Van Dyck and Deliens 1 – Reference Vadeboncoeur, Townsend and Foster 4 ). Thus, the first year of college is considered a critical period for risk of excess weight gain and consequently a target for obesity prevention( Reference Finlayson, Cecil and Higgs 2 , Reference Gropper, Newton and Harrington 5 ).
Although some obesity prevention programmes targeting first-year college students have shown success (e.g. ( Reference Hivert, Langlois and Bérard 6 – Reference Stice, Rohde and Shaw 8 )), most have investigated courses or seminars designed to educate students about obesity risk. Implementing these interventions on a large scale could be both costly and impractical. Interventions in the dining hall which focus on education (e.g. energy (calorie) information) show modest effects( Reference Cioffi, Levitsky and Pacanowski 9 ). At the same time, several short-term interventions based on principles derived from behavioural economics, such as manipulation of the size of dishes, utensils and portions( Reference Stroebele, Ogden and Hill 10 , Reference Wansink, Ittersum and Painter 11 ), have been shown to influence food choices and consumption( Reference Radnitz, Loeb and DiMatteo 12 ). Many such strategies can easily be employed in a college dining hall setting with relatively little cost or effort. For example, several colleges have gone ‘trayless’, simply eliminating the availability of trays that enable students to carry larger quantities of food, and have reported a reduction in both the amount of food students take and waste levels( Reference Thiagarajah and Getty 13 ). Intervening via payment method, specifically a restricted debit card v. cash v. unrestricted debit, resulted in a reduction in energy consumed in a dining hall( Reference Just and Wansink 14 ); however, utilizing restricted debit cards may not be easily generalizable to some dining hall systems. Hence, systemic changes in the food delivery systems on college campuses have the potential to reach a large number of students at low cost, rendering them appealing targets for obesity prevention efforts. To provide support for these changes, research is needed to establish their feasibility and effectiveness.
One strategy for reducing weight gain during college is to change the format of food selection in dining halls from one emphasizing free choice to one which ‘nudges’ students towards choosing lower-energy, lower-fat foods while still offering a full range of menu options( Reference Thaler, Sunstein and Balz 15 , Reference Wilson, Buckley and Buckley 16 ). A logical paradigm for instituting this format is one based on defaults, where more nutritious low-energy foods are featured prominently in menus and are more visible and easily accessible than energy-dense foods. In this scenario, to access the more energy-dense foods, individuals must make a special request and possibly encounter other inconvenience factors, such as a delay.
The appeal of defaults is that they can have a powerful impact on behaviour without restricting choice( Reference Carroll, Choi and Laibson 17 ). Individuals consistently choose defaults over opting out although the reasons for this are unclear. Several mechanisms for how defaults work have been proposed including inattention (lack of awareness that a decision can be made)( Reference Dhingra, Gorn and Kener 18 ), loss aversion (fear that opting out will entail a cost)( Reference Camerer 19 , Reference Kahneman, Knetsch and Thaler 20 ), information leakage (communication that the default is the ‘normal’ choice in novel decisions)( Reference McKenzie, Liersch and Finkelstein 21 , Reference Sher and McKenzie 22 ), inertia (unwillingness or inadequate opportunity to investigate other options)( Reference Madrian and Shea 23 ) and implied recommendation (perception that the default is the ‘best’ option)( Reference Johnson and Goldstein 24 ). Thus, the term ‘optimal defaults’ refers to the positioning of choices to increase the likelihood of a positive selection (i.e. one that benefits the individual or public).
Recently, research in the area of health-care delivery has shown that defaults can be utilized to influence the decision making of both patients (e.g. HIV screenings, flu shots)( Reference Chandisarewa, Stranix-Chibanda and Chirapa 25 , Reference Chapman, Li and Colby 26 ) and providers (e.g. ordering tests, prescribing generic medications)( Reference Patel, Day and Small 27 , Reference Walmsley 28 ). These studies suggest that default options can be strategically manipulated to positively influence health behaviour.
While long-term, applied studies testing defaults as an obesity prevention strategy are lacking, archival research( Reference Anzman-Frasca, Mueller and Sliwa 29 – Reference Wansink and Hanks 31 ) and field experiments( Reference Dalrymple, Radnitz and Loeb 32 – Reference Wisdom, Downs and Loewenstein 34 ) in restaurant settings have shown that altering menus to feature lower-energy options as defaults is associated with a reduction in the energy content of food orders compared with free array, standard conditions. Indeed, proof-of-concept studies investigating the effect of defaults in younger children( Reference Loeb, Radnitz and Keller 35 , Reference Loeb, Radnitz and Keller 36 ) and in selecting exercise courses in college students( Reference DiMatteo, Radnitz and Loeb 37 ) have demonstrated that defaults have consistent and strong effects.
Choice architecture using defaults often includes an inconvenience factor that naturally occurs as opting out may require added time and effort( Reference Hollands, Shemilt and Marteau 38 ). For example, in restaurants and other food preparation settings, ordering off menu might involve additional food preparation time. A delay in obtaining a choice has been found to affect it by reducing its value (‘delay discounting’). Delay discounting is a similar concept to delay of gratification in that both involve the study of how people naturally value rewards available immediately more than equivalent rewards available in the future( Reference Epstein, Salvy and Carr 39 ) and how manipulating this relationship (e.g. increasing the value of future rewards) impacts choice. Earlier studies of delay of gratification found that this ability to forego immediate rewards in favour of a delayed larger reward was correlated with persistence in goal-directed behaviour and achievement of desired outcomes( Reference Mischel, Shoda and Rodriguez 40 ).
The ability to delay gratification has implications for individuals who are obese as they may be less willing to forego an immediate reward (e.g. high-energy palatable foods and sedentary behaviour) in favour of a delayed one (e.g. improved health and nutrition including a leaner BMI), due to increases in food cravings( Reference Epstein, Salvy and Carr 39 ). In fact, compared with normal-weight women, obese women have demonstrated greater delay discounting that may manifest in making more immediate, less healthy choices( Reference Davis, Patte and Curtis 41 , Reference Weller, Cook and Avsar 42 ). Delay discounting and food reinforcement interact, such that those high in delay discounting tend to consume greater amounts of more palatable foods( Reference Appelhans, Woolf and Pagoto 43 , Reference Rollins, Dearing and Epstein 44 ). In the present study, the decision to include a delay as part of the alternative choice for an optimal default can be seen to counteract the possible preference for more energy-dense food choices. In other words, in the optimal default condition, delaying the availability of the higher-fat, more-energy-dense choices likely diminished their perceived value, making them less attractive compared with more immediately available less-energy-dense choices.
By combining two powerful tools from behavioural economics (defaults and delays), the goal was to test the effectiveness of an intervention that reflects manipulations with strong translational capacity to procedures within a college dining hall setting.
How defaults and delays impact food intake has not been studied in a college setting even though the first year of college is a high-risk period for weight gain. Consequently, a proof-of-concept experiment is warranted to determine whether default menus including a delay impact choice and energy intake.
The purpose of the current investigation was to use an experimental paradigm to study default plus delay presentations on menus in a ‘real-world’ college dining setting. Specifically, college students were randomized to one of three conditions: (i) an optimal default lunch, (ii) a suboptimal default lunch or (iii) a free-choice array lunch. We hypothesized that making the default options more optimal (less obesogenic) would lead to more frequent choice of lower-energy/fat, more-nutrient-dense foods than either the suboptimal default or free array lunches. Percentage of optimal choices, quantity of food consumed and energy intake in kilocalories were the primary outcome variables. The secondary outcome variables were percentage of total energy coming from fat, saturated fat, protein, carbohydrate and sugar ingested, as well as amount of fibre in grams. In addition, we examined likability ratings of study foods, as this measure might inform whether the effect of defaults would persist over the long term.
Methods
Participants
Participants were first-year college students attending one of two campuses of a private university located in the Northeastern USA. They were recruited through posted flyers, emails, classroom announcements and on-campus in-person promotion. The study included students in late adolescence (aged 18–19 years) in their first year of college at the university. Students were excluded if they reported any dietary restrictions that would interfere with eating the foods served in the experiment (e.g. food allergies, religious or other dietary restrictions) or medical conditions that would preclude fasting between consuming a standardized breakfast and the experimental lunch. A total of 215 students were screened by telephone to be included in the study, and 168 met criteria and were scheduled for study sessions. The primary reasons students were not entered into the study were either food allergies or scheduling conflicts. One hundred and thirty-six participants signed consent and completed evaluation procedures, and 129 of these completed the full experiment. The seven who dropped after the first evaluation were no-shows and were not responsive to efforts to reschedule them.
Procedure
The study utilized a randomized, between-subjects design with three conditions: optimal default (OD), free array (FA) and suboptimal default (SD). Data were collected between February 2014 and May 2015. Investigators worked with the university’s food-service vendor, who prepared and provided all study foods. Menus were crafted by our collaborating nutritionist (K.L.K.). Percentage of energy from total fat in the optimal meal was 27 % and from saturated fat was 6 % (both within the guidelines from the American Heart Association)( 45 ). The suboptimal meal had 38 % of energy from fat and 10 % from saturated fat, which exceeded the American Heart Association guidelines.
Research assistants were trained on experimental and food safety procedures. After telephone screening, participants were seen on two occasions. During the first session, they gave informed consent, completed questionnaires, and had their weight and height measured. Participants were given a brief description of study procedures, but the specific hypotheses were not disclosed. To equate hunger levels among participants, they each received a standardized pre-packaged breakfast, consisting of an energy bar (250–260 kcal (1046–1088 kJ)) and a 10-ounce (295 ml) milk-based protein beverage (180–190 kcal (753–795 kJ)), with instructions to consume it at a prescribed time the morning of their scheduled experimental lunch.
The condition for each date was determined using a randomized block design and participants could schedule days for the lunch based on convenience. The randomization code was managed by a researcher outside the study team, who conveyed the condition for each date after participant scheduling had occurred. All experimental lunch sessions (a total of thirty-three) were scheduled at the same time of day. The number of participants in attendance ranged from one to fourteen (mean 3·94 (sd 3·2)). Participants were directed to non-adjacent seating in a private room. In the default conditions, foods were clearly displayed next to a menu shown prominently in a clear Lucite stand (see online supplementary material, Supplemental File, for a depiction of all three menus). Optimal menu items were depicted in list form using 26-point font (Calibri), centred, with directions for opting out at the bottom in left-justified 12-point font. The opt-out directions specified the alternative menu choices with the proviso of a 15 min wait time for delivery. In the free array condition, all menu items were displayed, immediately available and listed on a menu in centred, 26-point font. In all conditions, the foods were presented identically in pre-packaged individual servings (to easily permit item-for-item substitutions from the alternative menu choices in the two default conditions if desired). The optimal menu consisted of nutrient-dense, lower-fat/energy items (see Table 1 for menus) with less-nutrient-dense, higher-fat/energy items allowed as an ‘opt out’. On the suboptimal menu the depictions were reversed in that the default lunch consisted of higher-fat/energy, less-nutrient-dense items, and participants had to actively opt out and wait for the optimized menu items if desired. Participants could choose any combination of items from the default display or the alternative menu, to mimic the allowance of choice typical in a college dining hall. At a check-out station, research assistants recorded selected foods. Once participants chose their food items there were no further instructions regarding eating. No second helpings were allowed, but participants were allowed unlimited water. They were instructed to leave their trays at the end of the meal and the research assistants weighed the food (not water) remainders. Participants received $US 25 for taking part in the study. They were debriefed in writing after the entire experiment was completed.
Table 1 Nutrient composition of items on optimal and suboptimal menus
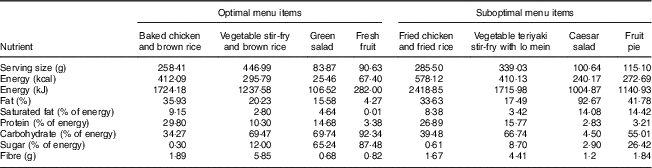
Total energy for meals including entrée, salad and dessert were the following: optimal chicken, 504·95 kcal (2112·71kJ); optimal vegetarian, 388·65 kcal (1626·11 kJ); suboptimal chicken, 1090·98 kcal (4564·66 kJ); suboptimal vegetarian, 922·99 kcal (3861·79 kJ). Nutritional information for salads was computed to include salad dressings.
Measures
Anthropometrics
Participants were weighed and measured (height) twice in light clothing without shoes using a balance beam scale with stadiometer (Detecto) that was calibrated before each weighing.
BMI percentile for each student was calculated using the Centers for Disease Control and Prevention( 46 ) BMI percentile for age and gender formula.
Liking of foods
During the first session, all participants completed a measure of ‘liking’ for each food in the experiment using a 167 mm line marking scale with the anchors 0, 50 (83·5 mm) and 100 (167 mm)( Reference Simmen, Pasquet and Hladik 47 ). The level of liking was computed by measuring the length of the line up to where participants marked and dividing by the total length of the line.
Choice
Trained research assistants recorded (immediately) the food choices each participant made. The percentage of optimal choices variable was computed by summing the number of optimal choices each participant made (maximum = 3: salad, main dish plus side, dessert), dividing by 3 and multiplying by 100. Taking more than one portion per food category was not allowed.
Quantity of food consumed
Foods were served in standardized portion sizes for this age group, determined from the Continuing Survey of Food Intakes by Individuals( Reference Smiciklas-Wright, Mitchell and Mickle 48 ). All foods were weighed before the experiment using an Ohaus Scout Pro commercial-grade food scale with digital readout in grams. Once participants ate the experimental lunch, the remaining food on their plates was weighed and these amounts were subtracted from the pre-lunch weights. Gram intakes for each food were converted to fibre grams and macronutrient values (i.e. total energy and energy from fat, saturated fat, protein, carbohydrate and sugar) using Food Processor version 11.0.
Behavioural observations of participants during the lunch
Research assistants completed a behavioural observation of participants, noting whether they sat alone (yes or no) and whether they engaged in another activity at least 50 % of the time while eating (yes or no). Two additional questions asked the number of people participants sat with (if not alone) and specific activities the participant was engaged in while eating (research assistants checked any that applied: cell phone (not talking), talking on cell phone, talking to another participant, talking to a research assistant, reading, writing, or using a laptop).
Demographic questionnaire
Participants completed a demographic form as part of their initial packet of questionnaires providing sex, age, ethnicity, year in college, marital status, home campus, whether they commuted or resided on campus, and whether they were currently dieting.
Power calculations and statistical analyses
A power analysis indicated that forty-five subjects per cell would provide at least 80 % (P = 0·05) power to detect a moderate effect size of 0·6 (Cohen’s d) in our primary dependent variable between all pairwise comparisons of the three study conditions. We chose this effect size estimate based on results from a prior study from our lab using similar methodology( Reference Loeb, Radnitz and Keller 35 ), but with parents of young children as agents of food selection. Although analyses from that earlier study yielded large effect sizes, we powered the current study to detect a more modest effect recognizing differences in who is making the food choices (older adolescents, not parents) and other aspects of the current design (e.g. the addition of a free-choice array condition).
Data were analysed using the statistical software package IBM SPSS Statistics version 24 using full factorial ANOVA and Tukey’s honest significant difference (HSD) follow-up comparisons for the condition variable. We tested for main effects of condition and sex and their interaction on percentage of optimal choices, energy consumed in kilocalories and quantity of food consumed (primary outcome measures). The secondary outcome variables were percentage of energy from fat, saturated fat, carbohydrate, protein and sugar, as well as amount of fibre in grams. Partial η 2 was computed as a measure of effect size of study results.
Results
Demographic characteristics of the final sample are presented in Table 2. A majority of participants were female (60 %) and 18 years old (77·5 %). The sample was diverse with approximately 28 % African American, 29 % White and 24 % Latino. According to Centers for Disease Control and Prevention (2016) charts, mean BMI was healthy for participating females (24·99 (sd 4·70) kg/m2, 65·5th percentile) and overweight for males (27·18 (sd 5·80) kg/m2, 73·1th percentile). There were no significant differences among study conditions in BMI for either females (F (2,74) = 0·18, NS) or males (F (2,49) = 0·38, NS). Descriptive data for both primary and secondary dependent variables broken down by sex and condition can be found in Table 3.
Table 2 Demographic characteristics of the study participants: first-year college students (n 129) from a Northeastern US university, February 2014–May 2015
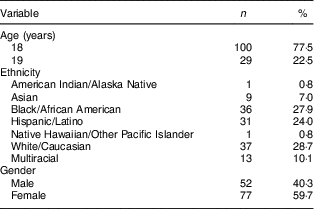
Missing ethnicity data for one participant.
Table 3 Means and standard deviations of primary and secondary outcome variables by condition and sex: college dining hall simulation study conducted among first-year students (n 129) from a Northeastern US university, February 2014–May 2015
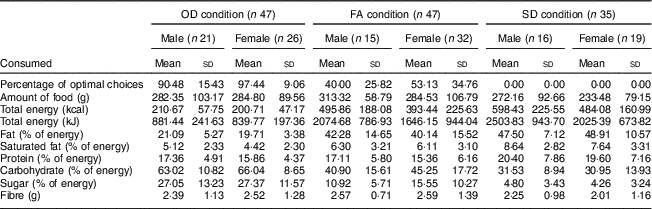
OD, optimal default; FA, free array; SD, suboptimal default.
Behavioural observations
Approximately one-third (34·4 %) of participants sat with others at a table, while the remainder sat alone. Over half (52·3 %) of the students were engaged in at least one other activity while consuming the lunch, with most of them (73·5 %) using their cell phones. A χ
2 analysis was not significant for condition with activity (active v. not active):
$\chi _{{(2)}}^{2} $
=0·55, P=0·759. However, it was significant for condition with seating (alone v. with others):
$\chi _{{(2)}}^{2} $
=13·96, P = 0·001. Further analyses to determine if seating was related to any dependent variables revealed no significant findings (see online supplementary material, Supplemental Table 1) for percentage of optimal choices, total energy consumed, amount of food consumed, percentage of energy from fat, protein, carbohydrate or sugar, or amount of fibre. However, there was a significant relationship between seating and percentage of energy from saturated fat. Consequently, we decided to repeat the analyses for percentage of energy from saturated fat using seating as a covariate (see below).
Food choices selected
Neither student sex nor the sex × condition interaction was significantly associated with the percentage of optimal choices selected (see Tables 3 and 4). However, the effect of condition on percentage of optimal choices was significant with follow-up Tukey HSD tests showing the largest percentage of optimal choices in the OD condition (94 %), which was significantly greater than that of the FA condition (47 %) which significantly exceeded that of the SD condition (0 %; see Table 5).
Table 4 Factorial ANOVA results for percentage of choices that are optimal, amount of food consumed, total energy and macronutrients consumed (percentage of total energy): college dining hall simulation study conducted among first-year students (n 129) from a Northeastern US university, February 2014–May 2015
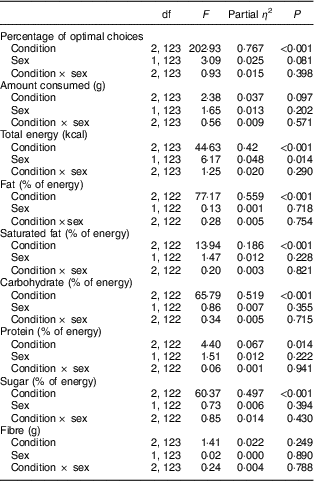
Table 5 Results of Tukey honest significant difference follow-up analyses: college dining hall simulation study conducted among first-year students (n 129) from a Northeastern US university, February 2014–May 2015
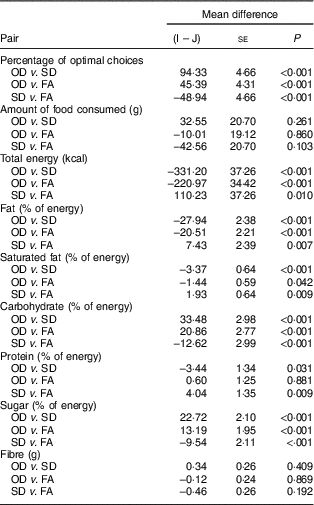
OD, optimal default; SD, suboptimal default; FA, free array.
Food consumed
The amount of food consumed (measured in grams) was not significantly different across conditions or between males and females (see Tables 3 and 4). As total energy is related to macronutrients, we computed percentage of energy from macronutrients relative to total energy consumed. Effects for the interaction of condition × sex were not significant for any analysis. The effect of sex was significant only for total energy consumed. There were significant effects of condition on total energy consumed and percentage of energy from fat, saturated fat, protein, carbohydrate and sugar. Those in the SD condition consumed significantly more total energy and significantly greater percentages of energy from fat and saturated fat compared with those in the FA condition, who consumed significantly greater total energy and percentages of energy fat and saturated fat than those in the OD condition (see Table 5). To ensure that seating (alone v. with others) was not a confounder for the saturated fat analysis, it was entered as a covariate into the equation examining the effects of condition and sex. The results were almost identical (see online supplementary material, Supplemental Table 2). Similarly, students randomized to the SD condition consumed a significantly greater percentage of energy from protein than those in the OD and FA conditions, who did not significantly differ from each other (see Table 5). However, for the analyses of percentage of energy from carbohydrate and sugar, significantly greater percentages of energy from carbohydrate and sugar were consumed by those in the OD condition followed by the FA and SD conditions, which were significantly different from each other. There were no significant differences in quantity of fibre consumed among conditions.
Liking
The ‘liking’ ratings of the low- and high-fat/energy versions of each food category (chicken main dish, rice side dish, vegetarian main dish, salad, dessert) were compared within subjects using pairwise t tests (e.g. likability of baked v. fried chicken). There was no significant difference in likability of the chicken dishes (see Table 6). However, students rated three of five of the higher-fat/energy foods as significantly more likable than their lower-fat/energy counterparts. The Caesar salad, fried pilaf and teriyaki stir-fry were all rated more likable than the green salad, brown rice pilaf and vegetable stir-fry, respectively. Only the lower-fat/energy dessert (grapes) was rated significantly more likable than the higher-fat/energy alternative offered (fruit pie).
Table 6 Comparison of study foods’ ‘liking’ ratings: college dining hall simulation study conducted among first-year students (n 129) from a Northeastern US university, February 2014–May 2015
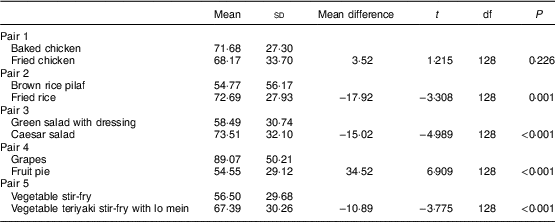
Values are based on percentage of line marked.
Discussion
The present study is the first to demonstrate in a college dining hall setting that an optimal default strategy resulted in food choices that were lower in energy/fat. There was a robust effect for condition with nearly all of those randomized to the optimized default menu remaining with the optimal choices, while none of the students who received the suboptimal default menu elected to switch to the optimal choices. The students given the free array menu chose almost half of the optimal choices. There were inherent energy and nutritional profile differences in the selections featured in the two default menus, as these were their defining characteristics. Because average amount of food consumption was comparable across groups, it was expected that significant differences in choice would manifest in significant differences in energy and macronutrient intakes. Specifically, those randomized to the optimal menu made less-energy-dense choices than those randomized to the free array menus, while those randomized to the suboptimal condition chose (and consumed) foods with more energy than those in the free array condition. This pattern of results was also found when macronutrients were analysed in terms of proportion of overall energy with two exceptions. For protein, we did not find that those in the OD condition consumed a significantly greater proportion compared with those in the FA condition, and for carbohydrate and sugar, we found that those in the OD condition chose and consumed foods with significantly more carbohydrate and sugar as a percentage of overall energy compared with the other two groups. The high percentage of energy from carbohydrate and sugar can be attributed to the relatively low energy and fat content of the OD food items (e.g. salad dressing and fruit). In contrast, SD items (e.g. fruit pie) contained a higher percentage of energy from fat than percentage of energy from sugar or other carbohydrates. Paradoxically, because these values are expressed as a percentage of total energy, items from the OD menu have a significantly greater percentage of energy from carbohydrate and sugar despite the fact that the actual number of kilocalories per serving from carbohydrate and sugar is lower compared with these values in the SD menu. Regardless, in future studies, it would be beneficial to include salad dressings with a lower quantity of sugar to increase the nutrient density of the OD items. Notably, we did find that those in the SD and FA conditions consumed a large share of energy from fat and saturated fat that was well above the American Heart Association guidelines. This may be of concern if similar choices are frequently made throughout students’ lives.
These results are in line with our other studies( Reference Dalrymple, Radnitz and Loeb 32 , Reference Loeb, Radnitz and Keller 35 – Reference DiMatteo, Radnitz and Loeb 37 ) suggesting at the proof-of-concept level that default menu manipulation has consistent and strong effects that can be applied to a variety of settings. These settings include simulated and actual restaurant settings and a school lunch cafeteria. Other studies of archival data( Reference Anzman-Frasca, Mueller and Sliwa 29 – Reference Wansink and Hanks 31 , Reference McCluskey, Mittelhammer and Asiseh 49 ) and field experiments( Reference Downs, Loewenstein and Wisdom 33 , Reference Wisdom, Downs and Loewenstein 34 ) have shown defaults to have effects in restaurant settings. Moreover, the effect seems to be linear. As a greater number of lower-energy items are included as part of the default offering, the percentage of high-energy foods ordered diminishes( Reference Downs, Loewenstein and Wisdom 33 , Reference McCluskey, Mittelhammer and Asiseh 49 ). The one exception was a report from Just and Wansink( Reference Just and Wansink 14 ) describing a brief study of a summer programme for children of elementary school age where the defaults failed to shape behaviour. However, in their report, the defaults appeared to be presented verbally and in a lunchroom line, rather than on menus.
As no significant differences among experimental groups were obtained in the amount of food participants ate, those who chose less-energy-dense foods did not appear to be compensating by consuming greater quantities of those foods( Reference Rolls 50 ). Moreover, there was no evidence that participants refused the lower-energy options, in contrast to the widely held notion that young people avoid eating healthier foods( Reference Glanz, Basil and Maibach 51 – Reference Riddell, Ang and Keast 53 ). In fact, almost half (47 %) the foods chosen in the FA condition were from the optimized menu.
The study design included an opt-out instruction with a 15 min wait time, in addition to the provision of alternative foods. A waiting period was included to enhance the ecological validity of the manipulation as going ‘off menu’ may require diners to wait longer for their food. For students who remained with the default, it is not clear whether their decision was based on the default placement on the menus or a wish to avoid having to wait for their alternative choices, or a combination of these two factors. Convenience can affect decision making( Reference Wisdom, Downs and Loewenstein 34 ), so determining how including a wait time, as part of a default opt-out paradigm, affects decision making is an important area for further study.
Although the differences between males and females in percentage of optimal choices and amount of food consumed did not reach significance, there were significant differences in total energy consumed across experimental conditions. This is not surprising as males and females have different energy needs( 54 ). At the same time, there was no evidence that default menus affected males and females differently as none of the interactions were significant.
A pre-experiment comparison of the liking of foods across all participants revealed that students liked higher-energy foods significantly more than their lower-energy versions in three of five cases. In only one case (the dessert) was the lower-energy food higher in liking. Liking is associated with preference and choice, with the association between disliking and rejection higher than that between liking and selection( Reference Rasmussen, Krølner and Klepp 55 , Reference de Graaf, Kramer and Meiselman 56 ). However, when defaults were presented, students showed a strong tendency to remain with the defaults regardless of how much they liked the alternative foods. Nevertheless, although liking did not seem to affect choice in the current experiment, it is unclear how it might affect choice in the long term, in a paradigm where students are repeatedly presented lower-fat/energy, nutritious default menus with higher-fat/energy ‘opt outs’. Formulating menus with lower-fat/energy food choices that are comparable in liking to their higher-fat/energy counterparts may be important for maintaining the impact of defaults in the long term.
There are several strengths of the present study that should be acknowledged. The sample was ethnically diverse. In addition, our collaboration with food services from study inception meant that food selections were prepared by dining hall kitchen staff and were similar to foods typically served on campus. Moreover, the study design had further ecological validity, extending from early discussions with the vendor, in that its three conditions reflected dining service procedures that are ongoing or could be adopted. Other concessions to ecological validity, such as permitting – if initiated spontaneously by the participant – engagement in other activities while eating (such as checking one’s phone) and adjustment of seating arrangement, could have introduced confounding variables in the design. However, post hoc analyses revealed little effect of these participant behaviours. Specifically, seating (alone v. with others) turned out to be related to condition, probably because participants were scheduled in groups of varying size and were not randomized individually as this would not have been feasible. However, seating was not related to the dependent variables except for percentage of energy from saturated fat. Re-analysing the data with seating as a covariate did not change the results.
Limitations include the single meal exposure to defaults, which did not permit a study of the sustainability of optimal defaults nor their effect on weight status across the first year of college. Although data are accumulating that suggest defaults can influence choices on menus, defaults have not yet been tested as an effective obesity prevention strategy over the long term. While there is variability across college campuses in the range of college dining facilities, in some cases undergraduates might eat in their dining halls at least once per day, if not even three times per day. For optimal defaults to be an effective long-term strategy in a college setting, they would need to continue to have a repeated effect for as long as students eat at dining halls, ideally shaping eating behaviours and preferences via repeated exposure in the long term. Second, although the internal validity of the experiment was strengthened by having all participants ingest a standardized breakfast at a fixed time, this was likely not representative of the timing or content of their typical breakfast. Third, the scope of the study did not permit an investigation of how the intervention affected students with diverse BMI. This may be an important area for future research. Fourth, although there was no evidence that students consuming the lower-energy food compensated by eating more of the meal itself, we do not know if students compensated for the lower energy intake at the lunch by ingesting higher-energy snacks or larger meals later. Finally, the sample size (n 129) was slightly smaller than the n 135 we planned for due to attrition from the first to the second meeting. However, because the effect sizes (see partial η 2 values in Table 4 for percentage of optimal choices, total energy, and percentage of energy from fat and saturated fat) exceeded 0·14, which is considered large for partial η 2 ( 57 ), the study was adequately powered.
Even if the intervention was found effective over the long term, one intervention would not be sufficient to prevent weight gain in college students. Additional interventions will be needed, such as those addressing other avenues for accessing high-energy foods (e.g. vending machines, retail) and exercise promotion. Using nudges, a strategy derived from behavioural economics that is very similar to defaults, several demonstration projects( Reference Almy and Wootan 58 ) (defaults) and other research studies (e.g. ( Reference Van Kleef, Otten and van Trijp 59 )) have shown that changing offerings at checkout from candy to healthier foods can impact consumer buying habits. Defaults can be utilized to steer undergraduates towards registering for exercise courses( Reference DiMatteo, Radnitz and Loeb 37 ), although this research also requires replication and extension beyond a proof-of-concept demonstration.
Conclusions
In summary, these findings show that optimal default strategies result in undergraduates making lower-energy food choices. Additional research is necessary to investigate how this effect can be maintained over the long term and used in conjunction with other interventions that positively affect energy intake and expenditure.
Acknowledgements
Acknowledgments: The authors would like to acknowledge the contribution of food products from Clif Bar and Company, and the assistance of the following individuals: Abigail Altman, Alessandra Berardi, Emily Biggs, Ilana Brodzki, Jessica Dalrymple, Julie DiMatteo, Arielle Feldman, Lisa Hail, Cassandra Hoy, Katie Huryk, Samantha Lowman, Chelsea Lynch, Amy Parter, Jenna Schlein, Corinne Sweeney, Erica Valerio, Bob Valenti and George Kuzma. Financial support: This work was supported by a grant from the National Institute of Food and Agriculture’s (NIFA) Agriculture and Food Research Initiative’s (AFRI) programme on Childhood Obesity Prevention (programme code A2101; grant/award number 2013-69001-20416). NIFA had no role in the design, analysis or writing of this article. Conflict of interest: None. Authorship: C.R. contributed to the original idea for the study, study design, grant editing, procedure implementation and ongoing supervision of students running the study, statistical analyses and writing the paper. K.L.L. was the primary author of the original grant and contributed to study formulation and design, procedure implementation and ongoing supervision of students running the study, statistical consultation and editing the paper. K.L.K. provided consultation on the nutritional make-up of the meals and portion sizes, grant editing, nutritional analyses and editing the paper. K.B. edited the original grant, contributed to the study design and implementation, and edited the final manuscript. M.B.S. edited the original grant, contributed to the study design and implementation, and contributed to the writing and editing of the final manuscript. L.T. ran participants, trained research assistants, organized and did data entry, did the nutritional analyses and contributed to the writing of the paper. S.M. contributed to the study design and choice of statistical analyses. Ethics of human subject participation: This study was conducted according to the guidelines laid down in the Declaration of Helsinki and all procedures involving human subjects were approved by the Fairleigh Dickinson University Institutional Review Board. Written informed consent was obtained from all subjects.
Supplementary material
To view supplementary material for this article, please visit https://doi.org/10.1017/S1368980017004220