Policy Significance Statement
Policy makers currently lack a framework to balance the potential of technology against the risks it also poses. This is perhaps especially true in the rapidly changing field of data science, where new horizons are being charted on a regular basis. The purpose of this paper is to provide policy makers—and others—with the outlines of an emerging framework. We outline 10 areas for data responsibility that policy makers can use to guide decisions at every stage of the data life cycle. The paper is exploratory. It is intended more as an exercise in agenda-setting than as a definitive statement. Nonetheless, these ten areas may prove useful in evaluating data uses and applications, and they may also help identify areas that merit further research or resources within the data ecology.
Data, data science, and artificial intelligence can help generate insights to inform decisions and solutions for some of our most intractable societal challenges, including climate change, global pandemics and health, food insecurity, and forced migration. The possibilities are immense—but only if the associated technologies, practices, and methodologies are used responsibly and appropriately.
While we have witnessed rapid innovation in how data are being leveraged, existing policies and tools for data responsibility have struggled to keep up. Most of the existing data governance frameworks and laws are still based upon the Fair Information Practice Principles (FIPPS) which were designed in the 1970sFootnote 1 for a different data environment. As a result, many consider the FIPPS as the necessary baseline but not sufficient or applicable when considering new data challenges.Footnote 2 As concern grows among policy makers, civil society and citizens about the harmful (and often unanticipated) effects of data and technology, it is imperative to reimagine data responsibility by developing and expanding new approaches and concepts for how data could be harnessed responsibly toward the public good.
Below we present 10 emerging approaches, grouped into three broad priority areas, that add up to a framework for data responsibility for policy makers, scholars, activists, and others working in the field. Taken together, these approaches and concepts can provide for more participatory processes, ensure greater equity and inclusion, and embed new practices and procedures in the way organizations across sectors collect, store, and use data. In arriving at these priorities, we begin from a recognition that data has both risks and potential. No technology is an unmitigated good; finding ways to maximize opportunity while limiting risks is key to generating new insights that can lead to societal benefits while simultaneously protecting individual and collective rights.
As indicated in Figure 1, the three priority clusters for responsible data that we identify are: Rethinking Processes and Systems, Rethinking Duties and Roles, and Rethinking Rights and Obligations.
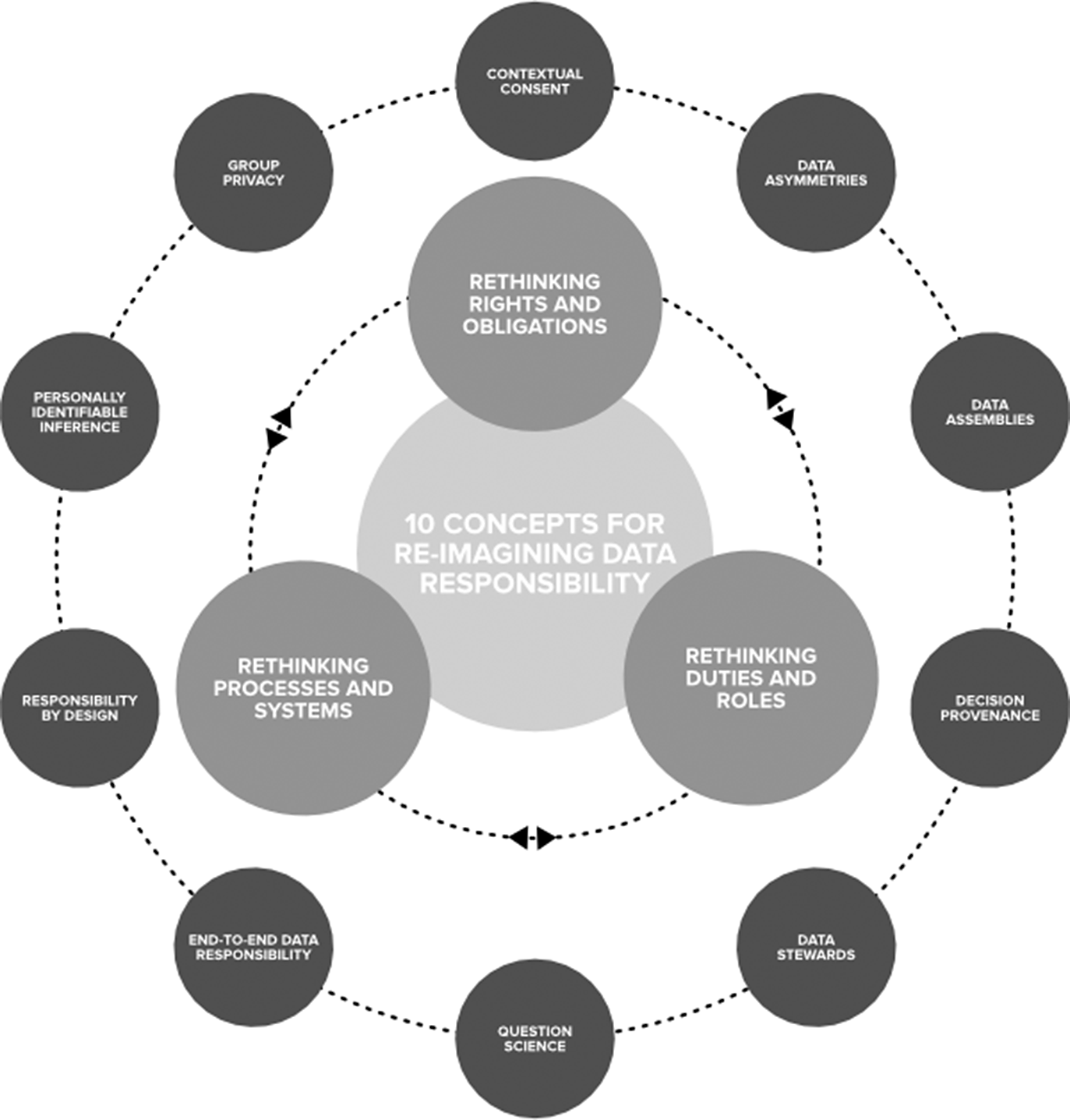
Figure 1. Re-imagining data responsibility.
While our discussion is preliminary and necessarily incomplete, these 3 priorities, along with the 10 components approaches we include, can help guide practices and policy-making surrounding the use of data, and they can also help identify areas for future research. The following discussion should be seen as an exercise in agenda-setting rather than a comprehensive survey; and is meant to give policy practitioners a rapid update of the emerging innovations. One of our primary goals is to identify which data responsibility approaches merit further attention (and resources) in order to develop or enhance a culture of trust in using and reusing data to address critical public needs.
1. Priority #1: Rethinking Processes and Systems
Data responsibility cannot be achieved in a piecemeal manner. Balancing risk and potential requires a systematic rethink of how organizations handle information across the data lifecycle. This systematic rethink can in turn be broken up into at least the following specific components.
1.1 End-to-end data responsibility
Using data and data science for the public good means promoting responsibility throughout the data lifecycle.
To generate value and effect change, data must be transformed into insight, and insight must be transformed into action. The sequences or stages of that enable value creation make up the data lifecycle(El Arass and Souiss, Reference El Arass and Souiss2018). Each phase of the data lifecycle—planning, collecting, processing, sharing, analyzing, and using—plays a formative role in generating value for stakeholders(Stodden, Reference Stodden2020). The respective phases also pose a number of risks and potential harms. Proactively assessing and mitigating risks (as well as capitalizing on opportunities) at each stage constitute the requirement of End-to-end data responsibility. (see Figure 2)
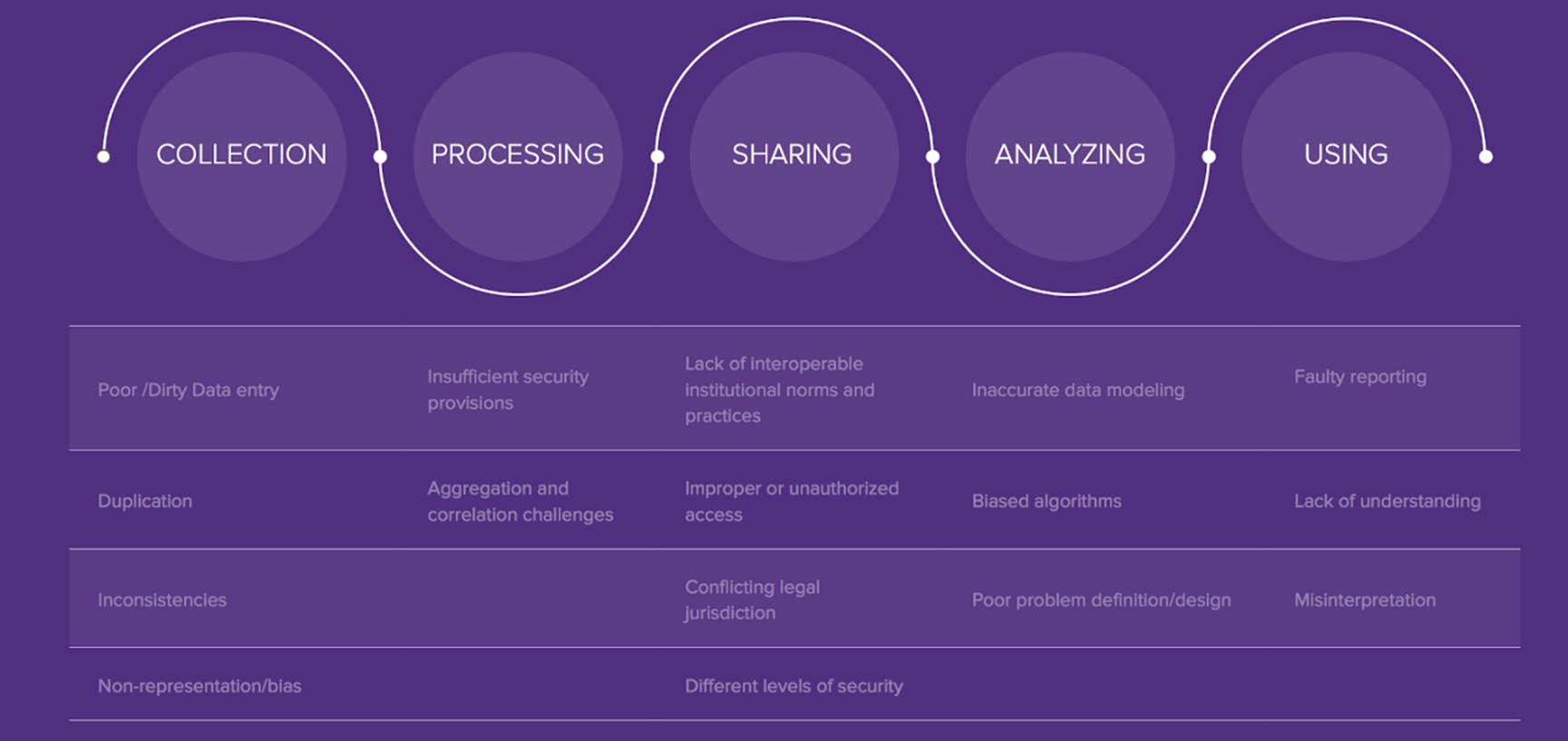
Figure 2. End-to-end data responsibility.
This approach is vital and goes beyond much recent thinking on data responsibility, which typically emphasizes risks at a single stage of the lifecycle—for example, algorithmic bias when analyzing data; secure cloud approaches when processing data or the need for consent at the collection stage. Mitigating risk at a single stage may be a good start, but it is not sufficient to accomplish true data responsibility. For example, we could envision a scenario in which collection stage risks are addressed by proactively collecting data on minority communities to mitigate bias; however, if acceptable sharing and use protocols are not put in place, there could be downcycle risk through exposure of sensitive demographic data. This is just one (hypothetical) illustration of why data responsibility must be prioritized more systematically, throughout the entire data lifecycle.
1.2 From data science to question science
Data-driven projects should arise as a result of genuine need, and not simply because an organization wants to access or use data that happens to be available. Toward that end, we need a new science of asking questions in order to identify priorities.
When using data for the public good, organizations too often focus on the supply side of the data equation (“What data do we have or can access?”) rather than the demand side (“What problems do we face, and what problems can data solve?”). As such, data initiatives often provide marginal insights and generate privacy risks while utilizing data that may not in fact be relevant to our most important societal problems (Auxier and Lee, Reference Auxier and Lee2019). What is required is a new approach that begins by asking the right questions about priorities and needs. In fact, we need a new science (and practice) of questions—one that identifies the most urgent needs in a participatory manner,Footnote 3 and that matches data supply and demand so as to create a more targeted approach to problem solving (Verhulst, Reference Verhulst2019).
In order to identify the questions that really matter, domain knowledge and data science expertise must be integrated. This can be done by targeting “bilinguals”: individuals who are proficient in a given domain and also skilled in the fields of data science and/or statistics (Meer, Reference Meer2015). The expertise of these individuals can be combined with the inputs of a wide range of users and citizens, helping formulate questions that are both relevant to societal issues and technically feasible. By ensuring the relevance of data projects, such an approach increases the likelihood of their impact and also conserves human and financial resources.
The Governance Lab’s 100 questions initiative seeks to do just this, developing a process that takes advantage of distributed and diverse expertise on a range of given topics or domains so as to identify and prioritize questions that are high impact, novel, and feasible, in the process ensuring that already scarce data resources are adequately used.Footnote 4 In this work, we have identified 10 domain fields of interest, such as migration, gender, and climate change, and identify and engage bilinguals who are tasked with identifying 10 important questions in their respective fields. The goal of this initiative is to create a template for future efforts that seek to reinvent how we ask questions about data.
1.3 Responsibility by design
Responsibility by design is a practice that seeks to introduces fairness, diversity, transparency, equality and data protection principles into all aspects and methods of data science.
The tools and methods of data science—including dataset selection, cleaning, preprocessing, integration, sharing, algorithm development, and algorithm deployment—span the data lifecycle. However, the need to ensure responsibility in all these processes is often overlooked, leading to biases and inequities in the way data are collected, preprocessed and processed, correlated, and reproduced. Such problems are exacerbated by the rise of machine learning and artificial intelligence techniques, as well as by the era of big data. As Julia Stoyanovich, founder of the Data, Responsibly consortiumFootnote 5 explains: “If not used responsibly, big data technology can propel economic inequality, destabilize global markets and affirm systemic bias.”Footnote 6 More recently, she and her colleagues have called for the need to consider data equity more broadly—as it relates to representation equity; feature equity; access equity; and outcome equity.Footnote 7 In order to mitigate such problems, it is necessary to utilize the principles and practices of responsibility by design. Although similar in some respects, responsibility by design goes beyond the earlier fairness, accountability, and transparency (FAT) concerns that are now widespread in the data community and that seek to address algorithmic bias (Lepri et al., Reference Lepri, Oliver, Emmanuel, Pentland and Vinck2018). Responsibility by design focuses on the full data science lifecycle—not just the design and deployment of particular algorithms (Stoyanovich et al., Reference Stoyanovich, Howe, Abiteboul, Miklau, Sahuguet and Weikum2017). It also borrows much of what is now known as privacy by design (Kingsmill and Cavoukian, Reference Kingsmill and Cavoukiann.d.; Cavoukian et al., Reference Cavoukian, Taylor and Abramsn.d.) and embraces privacy enhancing technologies.Footnote 8
Responsibility by design—and more recently the FAT community—suggests a “holistic consideration” of the sociotechnical systems that introduce the above concerns. As a framework, it ensures a well-planned and intentional approach to data science, and contributes to end-to-end data responsibility.
2. Priority #2: Rethinking Duties and Roles
The above priorities and steps require widescale and systematic transformation of organizations involved in the data lifecycle. But who will implement and oversee such change? In an era of data responsibility, we need to reconceptualize our notions of key actors and stakeholders, as well as the nature of their duties and roles. Here, we suggest three specific steps toward achieving the required transformation.
2.1 Decision provenance
To implement data projects with accountability, legitimacy and effectiveness, it is necessary to keep track of the decision flow - who makes decisions when - known as decision provenance, throughout the data lifecycle.
Data projects are complex undertakings that often involve disparate chains of authority and expertise applied to large, diffused problems. Often, the roles and responsibilities of different actors are poorly understood, and their actions poorly tracked; this complexity makes accountability difficult. Systematic data responsibility requires what is known as decision provenance—defined as a framework to “provide information exposing decision pipelines: chains of inputs to, the nature of, and the flow-on effects from the decisions and actions taken (at design and run-time) throughout systems” (Singh et al., Reference Singh2019). More specifically, decision provenance requires that organizations log each decision, and that all involved stakeholders have a clear understanding of how decisions are made across the data lifecycle, by whom, and for what purposes. For example, in the Governance Lab’s Responsible Data for Children Initiative (RD4C), we created a decision provenance mapping tool to support actors designing data investments for children to identify key decision points in the data ecosystem, advancing professionally accountable data practices. The tool requires users to identify specific data activities undertaken, note policies and laws impacting those activities and record all parties supporting decision making across the data lifecycle. And perhaps most importantly, the tool seeks to provide transparency into who is responsible and accountable at each stage, and who should be engaged when making decisions.Footnote 9
Decision provenance bolsters coordination and effective governance because it lets organizations keep track of what happened, and who was involved in data-related decision making. Decision provenance also establishes accountability and creates a “paper trail” (often virtual) in case something goes wrong. This is essential because a lack of accountability results in the potential for data misuse. Insufficient documentation of decision making can also lead to “missed opportunity,” or cases when data are not applied in a situation where they could contribute to problem solving. Finally, by exposing decisions that led to successes and failures, decision provenance can contribute to sustainability and scalability, helping identify ways to expand existing data projects and create new ones.
2.2 Professionalizing data stewardship
Organizations may consider creating a new role to establish data responsibility: the Chief Data Steward.
The complexity of data projects means that they typically involve large numbers of people. Often, the roles and responsibilities of these people are unclear, and projects suffer due to a lack of clear definition surrounding roles, hierarchies, and responsibilities. Responsible data handling requires building out a professionalized human infrastructure. In particular, it requires establishing a clear framework for what we call “data stewardship.”
Data stewards, as the Governance Lab defines them, are “individuals or teams within data-holding organizations who are empowered to proactively initiative, facilitate, and coordinate data collaboratives toward the public interest.” Stewards have three core responsibilities: collaborate, working with others to unlock the value of data; protect all actors in the data ecosystem from harm that might come from the sharing of data; and act, monitoring that data users are appropriately generating value and insights from the data shared. In addition, we have outlined five key roles a data steward must fill, to partner, coordinate, assess risk, disseminate findings, and nurture sustainability within collaboration efforts.Footnote 10 Data stewards need to be multidisciplinary to meet the evolving needs of the data ecosystem, as well as fulfill these roles and responsibilities in an agile manner. Data stewards are critical in making data collaboration efforts more systematic, sustainable, and responsible.
2.3 Greater use of data assemblies and public deliberation to establish legitimate re-use
Secondary re-use of data is an effective and efficient approach to data-driven problem solving, but must be guided by public deliberation and policies in order to establish legitimacy and abide by contextual consent.
One area of particular concern in the emerging field of data science and ethics concerns secondary use—that is, the use of data that was originally collected with another purpose in mind. Examples include the use of Geographical Information Systems and mobile data for crisis response or urban planning, and tax return data for civil sector transparency and accountability. Reusing data can save time and resources while providing useful insights, but serious consideration must be given to the specific circumstances under which it is acceptable to reuse data. Absent such consideration and clear guidelines, secondary use runs the risk of violating regulations, jeopardizing privacy, and de-legitimizing data initiatives by undermining citizen trust. Among the questions that need to be asked are what types of secondary use should be allowed (e.g., only with a clear public benefit?), who is permitted to use them, are there any types of data that should never be reused (e.g., medical data?) and what framework can allow us to weigh the potential benefits of unlocking data against the costs or risks.(Young et al., Reference Young, Verhulst, Safonova and Zahuranec2020)
Answering these questions requires not only new processes, but also rethinking our notion of stakeholdership (OECD, 2020) and arriving at a new understanding of what types of actors are involved in the data lifecycle. One emerging vehicle for balancing risk and opportunity is the use of working groups or symposia where thought leaders, public decision makers, representatives of industry and civil society, and citizens come together to take stock of existing approaches and design methodologies. The data assembly, for example, is an initiative from The Governance Lab, supported by the Henry Luce Foundation, that seeks to solicit public input on data re-use for crisis response in the United States.Footnote 11 In the summer 2020, the Assembly hosted three “mini-public” deliberations with data holders and policy makers, representatives of civic rights and advocacy organizations, and New Yorkers to address the needs of varying stakeholders and move toward a consensus on data-driven response to COVID-19 and other emerging threats. As a result of these deliberations, a data responsibility framework was co-designed indicating the conditions and principles that should guide the re-use of data for pandemic response and recovery.Footnote 12
This data assembly offers just one possible model. Many others exist.Footnote 13 The broader point is that data responsibility requires a reconceptualization of what types of actors are involved, and how they are involved. Broadening our notions of stakeholdership and participation will not only lead to better policy, but also work toward flattening some of the inequities and asymmetries we highlight below.
3. Priority #3: Rethinking Rights and Obligations
New systems and new roles will inevitably result in new risks. Data responsibility seeks to address not only known and legacy challenges, but also more dynamic, emerging ones. As part of this process, we must work to secure new rights—and new types of rights--and define new obligations on the part of all actors in the data lifecyle. Here, we outline four such rights and obligations.
3.1 Contextual consent
Contextual consent aims to obtain and use consent in a meaningful way, and to give users a legitimate say in the data ecosystem.
Recent privacy scandals and the introduction of regulations such as GDPR and the Digital Services Act in Europe have brought consent to the center of conversations about data. While much (if not all) data are assumed to be collected with the consent of users, especially if this no other lawful basis, there remain many shortcomings in the way consent is understood and deployed (Richards and Hartzog, Reference Richards and Hartzog2019). Helen Nissenbaum, a professor at Cornell Tech known for her work in the fields of online privacy and security, argues that “consent as currently deployed” is a “farce” because it gives the “misimpression of meaningful control” (Berinato, Reference Berinato2018). Problems arise when data are collected for one purpose and later used for another, or when users grant consent (e.g., to cookies) without fully understanding the implications of doing so. Users may also grant consent because they feel there is no alternative. A more responsible approach would rely on the notion of contextual consent (Barkhuus, Reference Barkhuus2012).
Contextual consent is based on the understanding that obtaining consent means more than simply soliciting a binary “yes” or “no.” The context of the interaction must be taken into account.Footnote 14 In some cases, the benefits of utilizing data without explicit consent may outweigh the potential harms. For example, at the onset of the COVID-19 pandemic, user location data was important to help decision makers at the state and local levels understand how citizens were responding to social distancing policies and to perform robust contact tracing (Wetsman, Reference Wetsman2020). This information also aided population cluster monitoring to inform the risk of COVID-19 spread in certain areas. It is important to note that GDPR does allow for organizations to (re)use personal data without consent if they can demonstrate a “legitimate purpose” and the “necessity” of processing personal data toward that endFootnote 15—which in essence a version of contextual consent. In other situations, contextual consent means engaging users in the data ecosystem in a more meaningful way—at the design, implementation, and review stage of data initiatives. Ideally, data subjects and community partners can be involved in all planning stages of public initiatives driven by local government bodies. Likewise, for cases in which user data will be used for efforts beyond that which it was originally collected, data subjects should be informed about ongoing processes on the retention and analysis of these datasets.
It is important to emphasize that, while contextual consent may in some cases allow for reuse without explicit approval, moving away from the current limited notion of consent does not imply disempowering users or enabling the unfettered reuse of personal data (Dunn, Reference Dunn2016). The goal is to give users a greater say in the data ecosystem and, in doing so, to encourage a more responsible approach to gathering, storing, and using data.
3.2 Righting data asymmetries through data collaboratives
Power asymmetries often result from unequal access to data and data science expertise. These data asymmetries threaten to restrict rights and freedoms in a broader socio-economic context.
A wide variety of imbalances exist when it comes to accessing data, and as well as in the ability to derive meaningful information and insights from it. This phenomenon is true across sectors, and equally applicable to individuals as organizations (Verhulst, Reference Verhulst2018). Marginalized citizens and traditionally disempowered demographic groups have less access to data (and its benefit) than those who are better resourced and educated. Similarly, in the private sector, data monopolies—companies with access to vast, almost limitless amounts of data—pose clear threats to competition and innovation. Even in the public sector and civil society, we see large discrepancies in the ability of governments and nonprofit groups to identify data opportunities, and to act on them in furtherance of better public policy or other social goods.
Data responsibility—and, indeed, social responsibility—requires us to address these asymmetries. There are many possible avenues, including increased “data portability”Footnote 16 and “data sovereignty”Footnote 17 but one of the most powerful tools we have at our disposal is a greater use of data collaboration. In particular, the emerging notion of “data collaboratives,” in which information is collectively accessed and acted upon across sectors (Susha et al., Reference Susha, Janssen and Verhulst2017), can help break down data silos and ensure that the right data gets to the people who can really benefit from it.Footnote 18 There is a growing body of literature, as well as several case studies (Verhulst et al., Reference Verhulst, Young, Winowatan and Zahuranec2019), to support the use of data collaboratives in mitigating inequalities and data asymmetries (Young and Verhulst, Reference Young, Verhulst, Harris, Bitonti, Fleisher and Skorkjær2020).
3.3 Transparency surrounding personally identifiable inference
There is a need for greater transparency regarding inferred personal data, or information that is interpreted about a person through multiple data points.
As we spend more time browsing the web, visiting social media platforms, and shopping online, we provide platforms and advertisers with growing amounts of personal data. Some of these data are explicitly disclosed, and some of them are observed (e.g., information about a user’s location or browser, which they may not have explicitly meant to share). An emerging third category is inferred data—information that is neither explicitly nor implicitly revealed by users, but that is arrived at through multiple pieces of disclosed or observed personal data. For example, a company may be able to infer a user’s gender, race, or religion based on content they are posting on social media platforms. They can then use this information to personalize marketing efforts toward certain users.(Barocas, Reference Barocasn.d.).
The goal of inferred personal data, writes Katarzyna Szymielewicz, the co-founder of Panoptykon Foundation, is to “guess things that you are not likely to willingly reveal” such as “your weaknesses, psychometric profile, IQ level, family situation, addictions, illnesses…and series commitments” (Szymielewicz, Reference Szymielewicz2019). The resulting profiles can be invasive and often incorrect, and users typically have little insight into how they are created and how to correct them (or even that they exist). A more responsible approach would require greater transparency, explainability, and human oversight over how such data are collected and used. Users should have more insight into and control over their inferred personal profiles, and they should be able to rectify or otherwise address inferred information about them. More generally, we need more conversations about acceptable uses of inferred personal data (Viljoen, Reference Viljoen2020). While some uses (e.g., targeted public services) may be acceptable, others (e.g., determining loan or housing access) may cross a line and should be regulated or outright banned.
3.4 Ensuring group privacy
Data segmentation by demographic group (e.g., by gender or age) has the potential to offer useful insights but it also requires special considerations for group privacy.
Segmenting data by demographic groups can make insights derived from that data more specific and lead to better targeted policies and actions. In addition, Demographically Identifiable Information (DII) can also help address the problem of “data invisibles,” in which certain groups are traditionally excluded from data collection and the resulting insights and actions. At the same time, there is growing recognition that DII poses certain risks (Floridi, Reference Floridi2014). The focus on individual data harms—and rights—“obfuscates and exacerbates the risks of data that could put groups of people at risk” (Young, Reference Young2020). We need to think of rights and obligations, too, at the group level (Taylor et al., Reference Taylor, Floridi and van der Sloot2017).
A key task facing any attempt to create a responsible data framework is to balance the benefits and threats to group privacy. Attention must be paid to avoid the so-called “mosaic effect” (Office of the Assistant Secretary for Planning and Evaluation (ASPE), 2014). This phenomenon occurs due to the re-identification of data (including anonymized data) by combining multiple datasets containing similar or complementary information. The mosaic effect can pose a threat to both individual and group privacy (e.g., in the case of a small minority demographic group). In addition, groups themselves are frequently established through data analytics and segmentation choices (Mittelstadt, Reference Mittelstadt2016). Due to the algorithmically guided criteria for group sorting, it becomes unclear who can and should advocate for the groups and be empowered to seek redressal. Lastly, individuals are likely unaware their data are being included in the context of a particular group (Radaelli et al., Reference Radaelli2018). Decisions made on behalf of this group can limit data holders’ control and agency. Mitigation strategies include considering all possible points of intrusion, limiting analysis output details only to what is needed, and releasing aggregated information or graphs rather than granular data. In addition, limited access conditions can also be established to protect datasets that could potentially be combined (Green et al., Reference Green, Cunningham, Ekblaw, Kominers, Linzer and Crawford2017).
Conclusion
Put together, the above 3 priorities and 10 steps add up to the outlines of a more comprehensive framework for data responsibility in the 21st century. Needless to say, our discussion is preliminary, and much work remains to be done in order to flesh out these concepts, as well as to understand nuances and variations in the way they may be applied. Indeed, we believe that one of the cross-cutting principles of data responsibility must be greater attention to variance. What works in one setting (geographic, cultural, or demographic) may not in another. A more comprehensive framework would drill down into these various requirements, allowing us to better understand how to tailor policies and principles to different contexts.
Funding Statement
Stefaan G. Verhulst has received funding to work on data responsibility by Luminate, UNICEF, the Rockefeller Foundation and FCDO (UK).
Competing Interests
Stefaan G. Verhulst is an Editor-Chief of Data & Policy. This article was accepted after an independent review process.
Data Availability Statement
Data availability is not applicable to this article as no new data were created or analyzed in this study.
Acknowledgments
The author would like to recognize and thank Akask Kapur, Aditi Ramesh, Andrew Young, Andrew Zahuranec, and Uma Kalkar, all at The GovLab (NYU), for their editorial support and review.
Comments
No Comments have been published for this article.