1. Introduction
Violence in inpatient psychiatric wards is a major problem for health services, with effects on patient and staff psychiatric morbidity [Reference Wildgoose, Briscoe and Lloyd1], wider implications on stigma for patients and recruitment in psychiatric hospitals, alongside costs associated with injury, staff sickness, and potential litigation by victims. There are higher reported rates of violence on forensic psychiatric wards compared to general psychiatry; a review of nearly 70,000 psychiatric patients from 122 studies in high income countries found that 48% of patients on forensic wards were violent over a mean follow-up of 31 months, which was almost double that for acute psychiatric wards (26%, mean time period: 19 months) and over two-fold that for other less acute psychiatric inpatient settings (22%, mean time period: 16 months) [Reference Bowers, Stewart and Papadopoulos2].
Despite its importance, few instruments have been designed for the prediction of violence specifically for inpatient populations. Current guidelines from the National Institute for Health and Care Excellence (NICE) [3] in England recommend the use of the Brøset Violence Checklist (BVC) [Reference Almvik, Woods and Rasmussen4, Reference Linaker and Busch-Iversen5] or the Dynamic Appraisal of Situational Aggression (DASA) [Reference Ogloff and Daffern6] for the prediction of inpatient violence, although US and Australasian guidelines do not appear to recommend any such tools for acute management of schizophrenia inpatients [Reference Galletly, Castle and Dark7, Reference Lehman, Lieberman and Dixon8].
Previous work has typically combined forensic psychiatric patients with other psychiatric populations and prisoners when assessing the predictive accuracy of risk assessment instruments [Reference Campbell, French and Gendreau9–Reference Whittington, Hockenhull and McGuire12]. A meta-review of violence risk assessment systematic reviews and meta-analyses found that 90% of reviews published before 2010 included mixed samples of different populations, and thus the overall findings may not be informative to specific patient groups [Reference Singh and Fazel13]. In addition, inpatient or institutional violence is often grouped together with community or offending outcomes in reviews [Reference Fazel, Singh and Doll10, Reference Singh, Grann and Fazel11, Reference Whittington, Hockenhull and McGuire12]. As violence base rates and possible interventions, and also the strength of risk factors, are different between inpatients and community-dwelling individuals, there is a need for a review specifically on inpatient violence.
Thus, we have aimed to systematically review and meta-analyse the performance of structured risk assessment instruments used to predict inpatient violence in forensic psychiatric samples. In addition, we have investigated sources of variation between individual studies using meta-regression analyses.
2. Methods
2.1. Review protocol
This review followed the Preferred Reporting Items for Systematic reviews and Meta-Analyses (PRISMA) statement [Reference Moher, Liberati and Tetzlaff14]. A review protocol was published on PROSPERO on 23/11/16: (https://www.crd.york.ac.uk/PROSPERO/display_record.asp?ID=CRD42016049789).
2.2. Risk assessment tools
Based on recent reviews and questionnaire surveys [Reference Hurducas, Singh and de Ruiter15–Reference Singh, Desmarais and Otto17], the 11 most commonly used instruments for forensic inpatient violence risk prediction were identified. Actuarial instruments included the Brøset Violence Checklist (BVC) [Reference Almvik, Woods and Rasmussen4, Reference Linaker and Busch-Iversen5], the Classification of Violence Risk (COVR) [Reference Monahan, Steadman and Silver18, Reference Monahan, Steadman and Robbins19], the Dynamic Appraisal of Situational Aggression (DASA) [Reference Ogloff and Daffern6], the Level of Service Inventory-Revised (LSI-R) [Reference Andrews and Bonta20], the Psychopathy Checklist Revised (PCL-R) [Reference Hare21], the Psychopathy Checklist Screening Version (PCL:SV) [Reference Hart, Cox and Hare22], the Violence Risk Appraisal Guide (VRAG) [Reference Quinsey, Harris, Rice, Quinsey, Harris, Rice and Cormier23, Reference Quinsey, Harris and Rice24] and the Violence Risk Scale (VRS) [Reference Wong and Gordon25]. Structured professional judgement (SPJ) tools included the Historical Clinical Risk Management-20 (HCR-20) [Reference Douglas, Hart and Webster26, Reference Webster, Douglas and Eaves27], the Short-Term Assessment of Risk and Treatability (START) [Reference Webster, Martin and Brink28, Reference Webster, Martin and Brink29] and the Violence Risk Screening-10 (V-RISK-10) [Reference Bjorkly, Hartvig and Heggen30, Reference Hartvig, Østberg and Alfarnes31]. Tools developed specifically for sexual violence were not included in this review as they are very rarely used in inpatients. Our systematic search returned no eligible studies focusing on the LSI-R or the V-RISK-10. Further information on each of the 9 included instruments can be found in Table 1.
Table 1 Characteristics of the nine included violence risk assessment instruments.
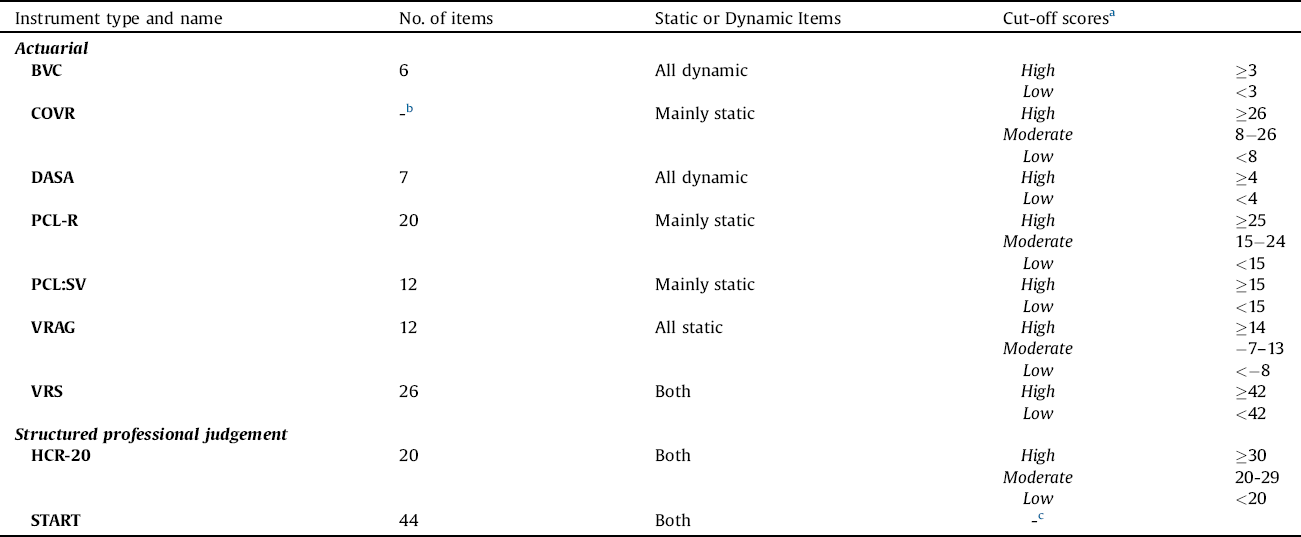
a Information on cut-off scores relates only to those samples who reported a cut-off score; in some cases cut-off scores were unknown or a clinical risk judgement may have been used instead.
b COVR has a varying number of items depending on answers given to previous items.
c No cut-off score was used for START classifications, as the low, moderate and high risk categorisation was given from the violence risk estimate section.
2.3. Systematic search
A systematic search was conducted to identify studies that measured the predictive validity of the nine instruments in forensic psychiatric settings for the outcome of inpatient violence. We searched five databases (CINAHL, Embase, Global Health, PsycINFO and PubMed) from the earliest available start date up to January 2017, using a keyword search of titles and abstracts with the following search terms: (PCL-R OR Psychopathy Checklist Revised OR HCR-20 OR Historical Clinical Risk Management OR PCL:SV OR Psychopathy Checklist Screening OR VRAG OR Violence Risk Appraisal Guide OR COVR OR Classification of Violence Risk OR LSI-R OR Level Service Inventory OR VRS OR Violence Risk Scale OR START OR Short Term Assessment Risk Treatability OR BVC OR Br?set Violence Checklist OR DASA OR Dynamic Appraisal of Situational Aggression OR V-RISK-10 OR Violence Risk Screening 10 OR risk assess*) AND inpatient* AND violen* AND risk AND (predict* OR valid*).
Additional studies were identified through hand-searching references of the identified studies, using the Google Scholar “cited by” function, scanning the annotated bibliographies for each instrument, and corresponding with researchers in the field. Studies in all languages and those that were unpublished were considered for inclusion. Studies were excluded if: (1) they measured the predictive validity of selected scales of a tool, as the aim was to test the accuracy of the tool as a whole; (2) they focused on a specific subgroup of the forensic population (e.g., those with a diagnosis of learning disability), as our aim was to focus on the most common forensic psychiatric populations; (3) instruments were coded retrospectively without blinding to outcomes, to avoid any possible observer biases in evaluating outcomes; (4) they were calibration studies for the actuarial tools, as such development samples will provide inflated accuracy. Where studies used overlapping samples, the sample with the larger number of participants was used in order to avoid double-counting. Using this search strategy, we identified 52 studies eligible for inclusion.
To be included in the full meta-analysis, studies were required to report numbers of true positives, false positives, true negatives, and false negatives at a given tool-specific cut-off score for the outcome of inpatient violence over a defined time period. We contacted study authors if this information was unavailable in the manuscript and they were asked to fill in a standardised form. The desired full range of outcome data were available in the manuscripts of 11 eligible studies (13 samples). Further data was requested from the authors of the other 41 manuscripts and data was obtained for an additional 8 studies (22 samples). Of the 52 eligible studies, 43 (78 samples) gave an overall performance measure (the area under the curve value; AUC) and thus were included for calculating the median summary AUC value for a wider sample. The final number of studies included in the meta-analysis of other performance measures (i.e. true and false positives/negatives with AUCs) was 19 (amounting to 35 samples).
2.4. Quality assessment
The QUADAS-2 tool, designed to assess methodological quality for systematic reviews of studies investigating diagnostic or prognostic accuracy, provided a risk of bias for each study, with low or high risk of bias categorisations. All included studies showed a low risk of bias.
2.5. Data analysis
Risk assessment instruments were divided into two groups: those designed for the prediction of imminent violence over a 24-hour period following the assessment (BVC and DASA) and those designed for the prediction of violence over a longer period (COVR, HCR-20, PCL-R, PCL:SV, START, VRAG and VRS). Given that instruments used for violence risk assessment in a clinical setting are primarily used to identify higher risk individuals that may need monitoring, we combined subjects who were classified as moderate risk with those classified as high risk, and compared these two categories to low risk patients.
2.5.1. Meta-analytic model
We followed guidelines in the Cochrane collaboration for systematic reviews of diagnostic and prognostic test accuracy [Reference Macaskill, Gatsonis and Deeks32]. We examined two central measures of accuracy: sensitivity (the proportion of violent patients that a risk assessment tool predicted to be higher risk) and specificity (the proportion of non-violent patients that an instrument predicted to be low risk). We then developed a bivariate random-effects model that jointly analyzed pairs of sensitivities and specificities, taking into account their correlation with one another [Reference Reitsma, Glas and Rutjes33]. Without covariates, this model is a different parameterisation of the hierarchical summary receiver operating characteristic (HSROC) model [Reference Rutter and Gatsonis34]. We then used summary receiver operating characteristic (SROC) plots to present the results of each study in receiver operating characteristic (ROC) space, with each study plotted as a single sensitivity-specificity point. This produced a SROC curve, with a summary operating point (showing summary sensitivity and specificity values), a summary AUC value, 95% confidence region and 95% prediction region. We obtained summary accuracy estimates for the sensitivity, specificity, positive predictive value (PPV; the proportion of patients classified as higher risk who went on to be violent), negative predictive value (NPV; the proportion of patients classified as low risk who went on to not be violent), diagnostic odds ratio (DOR; the ratio of the odds of violent patients having been classified as higher risk relative to the odds of non-violent patients having been classified as low risk) and the area under the curve (AUC) value.
2.5.2. Heterogeneity
Heterogeneity is expected in meta-analyses of diagnostic or prognostic test accuracy due to the bivariate nature of the analysis and variation in cut-off scores; therefore, the standard Q and I2 statistics are not recommended [Reference Jackson, White and Thompson35–Reference Zhou and Dendukuri39], but with no consensus on what to use [Reference Naaktgeboren, Ochodo and Van Enst40]. Thus it is recommended that visual evaluation of the scatter of points from the SROC curve and the size of the ellipse of the prediction regions be used to assess heterogeneity. A greater scatter of points from the SROC curve and a larger prediction region are indicative of greater levels of heterogeneity [Reference Macaskill, Gatsonis and Deeks32].
2.5.3. Meta-regression and subgroup analyses
Meta-regression analyses were conducted to investigate the relationship between an overall accuracy estimate (the AUC value) and pre-specified study and sample characteristics, to test whether any had a moderating effect on the AUC. Sample-related variables included sample size, gender, mean age of participants, and proportion of patients with psychotic disorder, personality disorder, or violent index offence. Study-related variables included temporal design of the study (prospective vs. retrospective), type of instrument (actuarial vs. structured professional judgement), follow-up period post-assessment, and definition of violent outcome used (interpersonal violence vs. interpersonal violence and verbal aggression). Meta-regression analysis was performed for studies included in the meta-analysis. We planned to investigate any significant findings on meta-regression using subgroup analyses. We also performed an additional analysis of the alternative binning strategy (low/medium vs. high) for the longer-term tools.
All analysis was conducted on Stata [Reference StataCorp41], using the midas command to generate summary statistics and a SROC curve and the metareg command for meta-regression analyses. Summary PPVs and NPVs were not produced by the midas command and were therefore calculated as medians. Summary AUC values for the wider group of eligible samples were also calculated as medians.
3. Results
3.1. Descriptive characteristics
For the wider sample of studies that reported on AUC values, information was collected for 6,840 participants in 78 samples from 43 independent publications. There were 5,680 (83%) male patients and 1,150 female patients. In the meta-analysis of all performance measures (with additional information on sensitivity and specificity), information was collected for 3,306 participants in 35 samples from 19 independent publications (Table 2). Standardised outcome information on numbers of true and false positives and negatives for 24 samples was obtained directly from study authors. When investigating all performance measures, there were 2,645 (80%) male patients and 661 female patients and the overall mean age of patients was 36.6 years (standard deviation [SD] = 3.5). There was some variation in both sample size (mean = 94.5; SD = 120.4) and rate of violence over the study period (mean = 31% of the sample being violent; SD = 16.1). Each risk assessment instrument had between one and four studies assessing predictive validity, with the exception of the HCR-20, which was investigated in 13 studies. Studies were conducted in 12 different countries: Australia, Belgium, Canada, Denmark, Hong Kong, Ireland, Japan, the Netherlands, Norway, Spain, the UK and the USA.
Table 2 Descriptive and demographic characteristics of samples for imminent and longer-term instruments included in the full meta-analysis (k = 35).

Note: Data are number (%) of samples, unless stated otherwise. Percentages are reported in relation to only those samples where information was available for the variable in question. SD = standard deviation.
Table 3 Summary accuracy estimates produced by two categories of violence risk assessment instruments.

Note: Median AUC values calculated from wider samples (k = 78): 10 samples for imminent tools and 68 samples for longer-term tools.
a Median (interquartile range).
3.1.1. Comparison between groups
In the meta-analysis of all performance measures, there were 1,394 patients in the 6 imminent tool samples (reported in 4 publications), compared to 1,912 patients in the 29 longer-term tool samples (15 publications). Both sample groups had approximately 80% male patients (Table 2) and there was little difference in mean age (37.0 and 36.4 years, respectively). Sample sizes for imminent tool studies ranged between 38 and 530 patients, while for longer-term tool studies, they spanned from 29 to 185. Follow-up length for all imminent tool samples had a 24-hr follow-up, while for longer-term tool samples, it was a mean of 692 days (SD = 979). The mean rate of violence over the defined follow-up period was 23.8% in the imminent tool sample compared with 32.6% for longer-term tools.
3.2. Predictive accuracy
3.2.1. Summary statistics
The studies included for the production of these summary statistics were those for which information on true and false positives and negatives was available (k = 35).
Predictive accuracy was different for the two groups of instruments (Table 3). In studies of imminent instruments, sensitivity was 0.59 (95% confidence interval [95% CI]: 0.29–0.83), while for longer-term instruments, it was 0.75 (95% CI: 0.65–0.83). The summary specificity for imminent tools was 0.99 (95% CI: 0.80–1.00) and for longer-term tools was 0.56 (95% CI: 0.46–0.66). A summary DOR for imminent tools could not be accurately calculated due to the number of zero-value categories (2 of the 6 samples included had one or more cells with zero values). The summary diagnostic odds ratio (DOR) for longer-term tools was 4.0 (95% CI: 3.0-6.0). The median PPV for imminent instruments was 0.36 (Interquartile range [IQR]: 0.10–0.93) and the median NPV was 0.99 (IQR: 0.85-1.00). The median PPV for longer-term instruments was 0.55 (IQR: 0.30-0.75) and the median NPV was 0.75 (IQR: 0.58-0.95).
Two different summary estimates of AUC values are reported based on different sample sizes. The first were calculated as median AUCs from all eligible studies that reported AUC values; this amounted to 78 samples and a total of 6,840 patients from 43 publications, based on 10 imminent tool samples (1,666 patients) and 68 longer-term tool samples (5,174 patients). The median AUC for imminent instruments was 0.83 (IQR: 0.71-0.85), while for longer-term instruments it was 0.68 (IQR: 0.62-0.75) (Table 3).
The second summary AUC value reported is that from the samples included in the meta-analysis (k = 35), as for the other reported performance measures. The summary AUC value for imminent tools in the meta-analysis sample was 0.90 (95% CI: 0.87-0.92) and for longer-term tools it was 0.71 (95% CI: 0.67-0.75).
3.2.2. HSROC curves
Figs. 1 and 2 show the hierarchical summary receiver operating characteristic (HSROC) curve formed from the meta-analysis of imminent and longer-term instruments, respectively. On both curves, the summary sensitivity, specificity point is plotted, along with a 95% confidence contour and a 95% prediction contour. The HSROC curve for imminent tools is approaching the top left-hand corner of the graph, indicating high accuracy, but the prediction contour is large, indicating high levels of between-study heterogeneity (Fig. 1). For longer-term tools, the HSROC curve is closer to the y = x diagonal that would indicate an uninformative test than it is to the top left-hand corner of space (Fig. 2). The prediction contour is also large, again indicating high levels of between-study heterogeneity.

Fig 1. Summary receiver operating characteristics (SROC) curve from bivariate analysis of imminent violence risk assessment instruments for forensic inpatient violence.
Note: Summary operating point = best fit for sensitivity and specificity. 95% confidence contour represents within-study heterogeneity. 95% prediction contour represents between-study heterogeneity.

Fig 2. Summary receiver operating characteristics (SROC) curve from bivariate analysis of longer-term violence risk assessment instruments for forensic inpatient violence.
Note: Summary operating point = best fit for sensitivity and specificity. 95% confidence contour represents within-study heterogeneity. 95% prediction contour represents between-study heterogeneity.
3.2.3. Individual tool performance
Within the wider group of 78 samples, the majority of samples assessed the performance of the HCR-20 (k = 27) and the PCL-R (k = 10). These tools performed moderately for the prediction of inpatient violence with median AUCs of 0.70 (IQR: 0.62-0.80) and 0.64 (IQR: 0.61-0.69), respectively. Imminent instruments had higher AUC values; the BVC (k = 5) had a median AUC of 0.83 (IQR: 0.75–0.87) and the DASA (k = 5) also had a median AUC of 0.83 (IQR: 0.65-0.90). See Appendix Table 2 in Supplementary material for all accuracy measures for each instrument.
3.3. Investigation of heterogeneity and subgroup analyses
Meta-regression analyses were only performed for longer-term instrument samples, as there were too few imminent instrument samples (k = 6). No study- or sample-related variables were associated with between-study difference in AUCs (Appendix Table 3 in Supplementary material). When we used an alternative binning strategy (low/medium vs. high), the performance of the longer-term tools was marginally improved with regards to PPV and AUC (Appendix Table 4 in Supplementary material).
4. Discussion
This systematic review and meta-analysis examined the predictive accuracy of 9 violence risk assessment instruments for inpatient violence in forensic psychiatric hospitals from 78 samples involving 7,705 patients from 14 different countries. The main finding was that instruments designed for the prediction of imminent violence performed better at predicting inpatient violence than instruments designed for longer-term follow-up periods, based on a range of performance measures. As a measure of overall accuracy, the median AUC for imminent tool studies was 0.83, compared to a median AUC of 0.68 for longer-term tools. Generally, AUC values greater than 0.8 indicate a highly accurate test and those below 0.7 indicate poor to moderate accuracy [Reference Tape42]. Imminent instruments performed particularly well for screening out low risk individuals: 99% of those who went on to not be violent were correctly predicted to be low risk (specificity) and 99% of those who were predicted to be low risk went on to not be violent (NPV).
4.1. Individual tool performance
The HCR-20 is the most widely-used violence risk assessment instrument internationally, yet our findings from this review show that it has at best moderate accuracy across a range of performance measures, with regard to the prediction of inpatient violence. These lower levels of accuracy are likely a consequence of how the HCR-20 has been developed, as it is a general violence risk assessment instrument with applications and recommendations for use in a broad range of contexts, populations and follow-up periods. Similarly, the PCL-R and VRAG performed poorly for the prediction of inpatient violence. Although their performance may be acceptable for some populations in the community, the current evidence does not support their use for the prediction of inpatient violence in forensic psychiatry.
The two instruments designed specifically for imminent inpatient violence prediction (the BVC and the DASA) performed with higher accuracy for a number of measures. However, there were few studies (k = 10) despite being recommended by NICE. There were more studies focused on the poorer performing tools, such as the HCR-20, suggesting a need to move towards research examining short-term tools, and possibly optimizing them by considering novel risk factors [Reference Eriksen, Bjørkly and Lockertsen43].
4.2. Clinical implications
Our findings indicate that the use of instruments designed for the imminent prediction of violence over the 24-hour period post-assessment yielded higher accuracy for multiple measures of performance. In clinical practice, consideration should be given to the use of the BVC and the DASA, both of which are recommended tools in one clinical guideline for short-term management of violence and aggression in inpatient mental health settings [3]. Furthermore, the narrow 24-hour window within which violence is predicted allows for prevention and management strategies to be implemented when they may be most needed. Both the BVC and DASA are brief checklists (6 and 7 items, respectively), have the advantage of scalability and can easily be integrated into routine practice.
However, other clinical contexts will exist where longer-term instruments may be more relevant or appropriate; the high sensitivity (0.75) and moderate PPV (0.55) suggest these instruments may have a role for some patients. Considering the brevity of the BVC and DASA, they could act as a screen before a longer term tool is used considering the expense involved in administering time-consuming and resource-intensive instruments [Reference Rosenfeld, Foellmi and Khadivi44].
However, for both imminent and longer-term tools, it is important for there to be a link with clinical interventions and outcomes to link the risk prediction element with subsequent management of risk. One randomised controlled trial (RCT) has been conducted finding a positive effect (reduction in inpatient violent incidents) when the BVC was used in a forensic psychiatric sample combined with implementation of a violence management strategy and training [Reference Abderhalden, Needham and Dassen47].
4.3. Strengths and limitations
To our knowledge, this is the first comprehensive review and meta-analysis of violence risk assessment instruments in the context of their predictive accuracy for inpatient violence in forensic psychiatric populations. There has been one previous review of risk assessment for inpatient violence in forensic psychiatric patients [Reference Hogan, Ennis and Assessment45]. However, it used mean correlation coefficients between violence risk assessment scores and inpatient violence, which is limited to examine predictive accuracy. Further, only three violence risk assessment instruments (the HCR-20, PCL-R and PCL:SV) were included in that review.
Recent criticism of risk assessment literature has stated that there is an insufficient focus on subpopulations in a specific context [Reference Douglas, Pugh and Singh46]. Unlike previous reviews of risk assessment tools, the current one investigates a particular patient group in one setting. In addition, the literature on predictive accuracy of violence risk assessment has been limited by relying on one or two measures of accuracy [Reference Douglas, Pugh and Singh46]. The AUC value, for example, is often reported in isolation; however, it does not indicate whether this discrimination is clinically useful, nor does it provide any information on the calibration of the instrument’s predictions with actual future violence [Reference Singh48]. To address this, we investigated a range of accuracy measures although none of the included studies reported calibration measures.
One limitation is that only studies reporting true and false positives and negatives could be included in the full meta-analysis. However, median AUCs were reported for the wider sample of eligible studies. Further, we corresponded with authors requesting unpublished data and increased the number of possible samples from 11 to 35 samples that report a range of performance measures. Another limitation is the large amount of between-study heterogeneity, perhaps due to variations in cut-off scores used for risk classifications. A number of other possible explanations were investigated in meta-regression and no associations were found to explain the variation between tools. This heterogeneity is expected, especially in prognostic (as opposed to diagnostic) studies, and the use of a random-effects model accounted for this variation. Further, where possible, the same cut-off scores were applied for each sample of the same instrument.
There were differences between the imminent and longer-term groups of studies with regard to the type of primary outcome used (interpersonal violence only vs. interpersonal violence and verbal aggression), which could explain their relative performance. Although this was investigated in meta-regression analyses and found to have no effect on the AUC accuracy estimate for longer-term tools, this analysis could not be performed for imminent instruments due to lack of available data. It is possible, therefore, that the better performance of the imminent tools (based on AUCs) is based on higher rates of softer outcomes (i.e. aggression), which will inflate base rates.
We also found marginally improved performance in some performance measures when we used a different binning strategy (low/medium vs high). Whether this merits a change in how these tools are used in practice and for which inpatient settings requires further work.
4.4. Future directions
Future research on violence risk assessment in forensic inpatient settings should focus more on imminent instruments as this meta-analysis found a smaller proportion of the research literature based on these instruments. Another useful direction for research would be further exploration of whether there should be a screen before longer-term instruments are used [Reference Rosenfeld, Foellmi and Khadivi44]. As the two imminent tools in this study rely predominantly on dynamic variables, research could investigate the role of novel dynamic variables to improve risk prediction, and whether adding static variables can add incremental performance. Further to this, new technologies that have been developed for the use of risk prediction and monitoring should be examined [Reference Gulati, Cornish and Al-Taiar49]. From a methodological perspective, future work in this area should report multiple estimates of predictive accuracy in order to provide a more complete picture of an instrument’s performance, including measures of calibration. Overall, this meta-analysis supports previous recommendations that future work in violence risk assessment requires the development and validation of tools designed for specific populations [Reference Douglas, Pugh and Singh46, Reference Fazel, Wolf and Larsson50, Reference Wolf, Fanshawe and Sariaslan51].
Acknowledgements
We thank the following study authors for providing tabular data for the analyses: Dr. Kaoru Arai, Dr. Oliver Chan, Professor Geoff Dickens, Dr. Óscar Herrero, Dr. Helen Miles, Professor Robert Snowden, Professor Lindsay Thomson and Dr. Vivienne de Vogel.
SF is a Wellcome Trust Senior Research Fellow in Clinical Science (202836/Z/16/Z). We would like to disclose no conflicts of interest of funding sources for this review.
Appendix A Supplementary data
Supplementary data associated with this article can be found, in the online version, at https://doi.org/10.1016/j.eurpsy.2018.02.007.
Comments
No Comments have been published for this article.