In the past decade, the prevalence of cardiometabolic diseases such as obesity, diabetes and CVD have increased dramatically in both industrialised countries and developing countries with emerging economies(Reference Jaacks, Vandevijvere and Pan1–Reference Saklayen4). Cardiometabolic diseases are caused generally by the interaction of lifestyle factors and genetic susceptibility(Reference Vimaleswaran and Loos5–Reference Heianza and Qi7). Dietary factors play an important role in the development of obesity, diabetes and CVD. Studies have shown that under-nutrition during the perinatal period can lead to an 85 % reduction in expression of brown fat biomarkers and genes involved in the citric acid cycle and fatty acid oxidation(Reference Kozak8), providing evidence for gene–diet interactions (i.e. nutrigenetics) on cardiometabolic diseases. Although several studies have examined the interactions between genes and dietary intake on cardiometabolic traits, the findings have been inconsistent because of two main challenges: (i) genetic heterogeneity, which is the systematic differences in the allele frequencies across various ethnic groups and (ii) insufficient sample size, and hence, it is unable to develop a personalised diet for each ancestral population without reliable information on gene–diet interactions.
Obesity can predispose individuals to several diseases including type 2 diabetes and CVD(Reference Guh, Zhang and Bansback10–Reference Papaetis, Papakyriakou and Panagiotou12). Furthermore, central obesity has shown to be associated with increased risk of mortality compared to common obesity(Reference Hamer, O'Donovan and Stensel13). Obesity is a multifactorial condition caused by a complex interplay between environmental (unhealthy diet and physical inactivity) and genetic factors (genetic susceptibility)(Reference Vimaleswaran and Loos5). Candidate gene and genome-wide association studies have identified several common SNP associated with obesity(Reference Vimaleswaran and Loos5,Reference Bradfield, Taal and Timpson14–Reference Vimaleswaran, Angquist and Hansen17) . Of these, the fat mass and obesity-associated gene (FTO) variants were found to be consistently associated with obesity traits in various populations and have been the strongest common genetic predictor of obesity known so far(Reference Vimaleswaran and Loos5,Reference Bradfield, Taal and Timpson14,Reference Willer, Speliotes and Loos18,Reference Vasan, Karpe and Gu19) . To date, FTO has shown the strongest association with BMI, where the FTO SNP increased the risk of obesity 1·20–1·32-fold in Europeans(Reference Speliotes, Willer and Berndt20) and 1·25-fold in Asians(Reference Li, Kilpelainen and Liu21). A recent meta-analysis of data from eight Indian studies showed that the FTO variant, rs9939609, increased the risk of obesity 1·15 times, which is equivalent to BMI increase by 0·30 kg/m2 per effect allele(Reference Vasan, Karpe and Gu19). Likewise, candidate gene and genome-wide association studies have shown the transcription factor 7-like 2 (TCF7L2) gene as the strongest candidate for type 2 diabetes(Reference Bodhini, Gaal and Shatwan22–Reference Franklin, Aulchenko and Huffman24). Besides FTO and TCF7L2, there are other genes/SNP, which have also been shown to be associated with obesity and other cardiometabolic diseases such as CVD and type 2 diabetes in various populations(Reference Bradfield, Taal and Timpson14,Reference Willer, Speliotes and Loos18,Reference Speliotes, Willer and Berndt20,Reference Warrington, Beaumont and Horikoshi25) .
Several studies in European populations have shown that physical activity and dietary intake may modify the association of SNP with cardiometabolic disease-related traits(Reference Ortega-Azorin, Sorli and Asensio26–Reference Du, Vimaleswaran and Angquist29). Increased physical activity levels have been shown to attenuate the effect of genetic variants (such as FTO and NOS3) on cardiometabolic traits in several populations(Reference Kilpelainen and Franks27,Reference Vimaleswaran, Franks and Barroso28,Reference Vimaleswaran, Li and Zhao30,Reference Kilpelainen, Qi and Brage31) ; however, gene–diet interactions have shown conflicting results(Reference Du, Vimaleswaran and Angquist29,Reference Vimaleswaran, Bodhini and Lakshmipriya32–Reference Sonestedt, Roos and Gullberg34) which could be attributed to genetic heterogeneity and various dietary factors (macronutrients and micronutrients). Given that the genetic make-up varies from one ethnic group to another, it is important to explore gene–diet interactions in multiple ethnicities, which will enable us to personalise diet according to each ethnic group. To address all these issues, the Gene–Nutrient Interactions (GeNuIne) Collaboration(Reference Vimaleswaran9) has been initiated to investigate the effect of GeNuIne on cardiometabolic disease-related traits using population-based studies from various ethnic groups in in lower-middle income countries (LMIC).
Role of the British Nutrition Foundation in GeNuIne Collaboration
The British Nutrition Foundation provided the start-up funds to initiate the GeNuIne Collaboration(Reference Vimaleswaran9), where the funds were used to undertake the pilot work required to generate data that can be used for conducting a large-scale study. The British Council Researcher Links travel grants obtained to establish collaborations with researchers in LMIC such as India, Brazil, Morocco, Turkey, Thailand, Sri Lanka, Indonesia and Pakistan. Although GeNuIne have been examined extensively in the western population, very few studies have been carried out in the LMIC and, hence, the GeNuIne Collaboration has been established to address this missing gap in human subjects’ nutrition in these countries.
Findings from GeNuIne Collaboration
Nutrigenetic studies in South Asia
After China, India has the highest number of people with type 2 diabetes in the world and the Indian Council of Medical Research–INdia DIABetes study has shown that type 2 diabetes cases have reached 62·4 million and 77·2 million people are pre-diabetic(Reference Anjana, Pradeepa and Deepa35). Asian Indians have unique clinical and biochemical characteristics that are collectively referred to as the South Asian phenotype (higher waist circumference, higher levels of total and visceral fat, hyper-insulinaemia, insulin resistance, and a greater predisposition to diabetes)(Reference Mohan and Deepa36,Reference Prabu, Rome and Sathishkumar37) , which confers increased susceptibility to diabetes and premature CVD.
Given the increased prevalence of type 2 diabetes among Asian Indians, the first study of GeNuIne Collaboration examined the interaction between two commonly studied FTO SNP and lifestyle factors such as diet and physical activity on obesity traits and type 2 diabetes in 1618 Asian Indians(Reference Vimaleswaran, Bodhini and Lakshmipriya32). The participants for this study were recruited from the urban component of the Chennai Urban Rural Epidemiology Study, a cross-sectional epidemiological study conducted on a representative sample of the population of Chennai in southern India(Reference Deepa, Pradeepa and Rema38). Dietary intakes were assessed using a previously validated and published(Reference Sudha, Radhika and Sathya39) interviewer administered semi-quantitative FFQ containing 222 food items to estimate food intake over the past year. Physical activity was estimated using a previously validated self-report questionnaire(Reference Mohan, Sandeep and Deepa40). The study identified a significant interaction between FTO SNP rs8050136 (Table 1) and carbohydrate intake (% energy) (P interaction = 0·04), where the high obesity risk A allele carriers had 2·46 times increased risk of obesity than those with low obesity risk CC genotype (P = 3·0 × 10−5) among individuals in the highest tertile of carbohydrate intake (% energy, mean: 71 %). A significant interaction was also observed between FTO SNP rs11076023 and dietary fibre intake (P interaction = 0·0008), where individuals with AA genotype in the third tertile of dietary fibre intake had, on average, 1·62 cm lower waist circumference than those with low obesity risk T allele (P = 0·02) (Fig. 1). Furthermore, the A allele carriers of the SNP rs8050136 had 1·89 times increased risk of obesity than those with CC genotype (P = 4·0 × 10−5) among those who were physically inactive. In summary, these findings indicate that Asian Indians with at least one copy of the FTO obesity-risk allele who consume a high carbohydrate diet or are physically inactive are at a particularly high risk of obesity, while high-fibre intake may protect against obesity risk in this group. Given that India leads the world in prevalence of type 2 diabetes and 28–44 % of Asian Indians carry at least one copy of the FTO risk allele(Reference Li, Kilpelainen and Liu21), our study highlights the need to discourage consumption of foods high in sugars and refined carbohydrate and encourage intake of high-fibre foods and increased physical activity levels, as following such advice could substantially reduce the genetic risk of obesity and type 2 diabetes among Asian Indians.
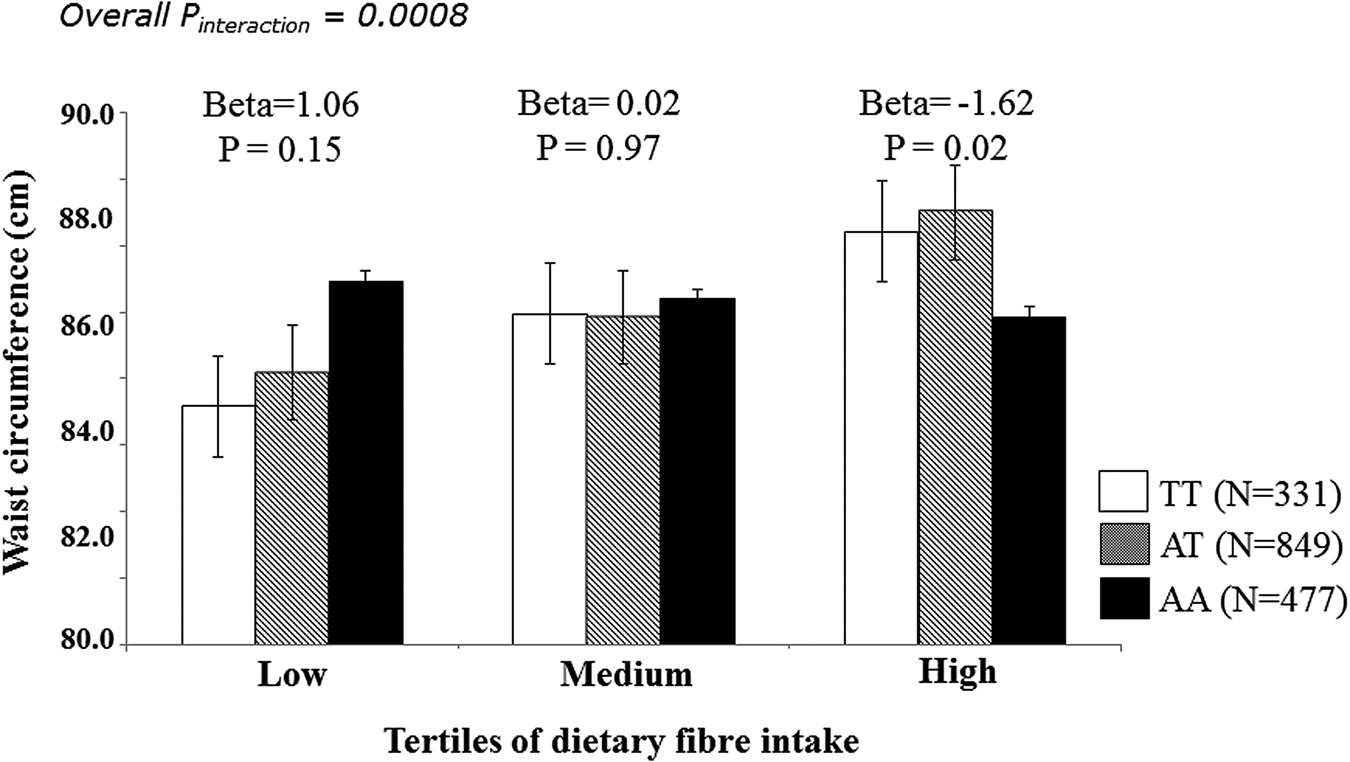
Fig. 1. Interaction of the FTO SNP rs11076023 with dietary fibre intake on waist circumference in Asian Indians. The individuals with AA genotype are in the third tertile of dietary fibre intake have a 1·62 cm decrease in waist circumference compared to those with T allele carriers (P = 0·02).
Table 1. Minor allele frequencies (MAF) of the gene variants studies in the four ethnic groups
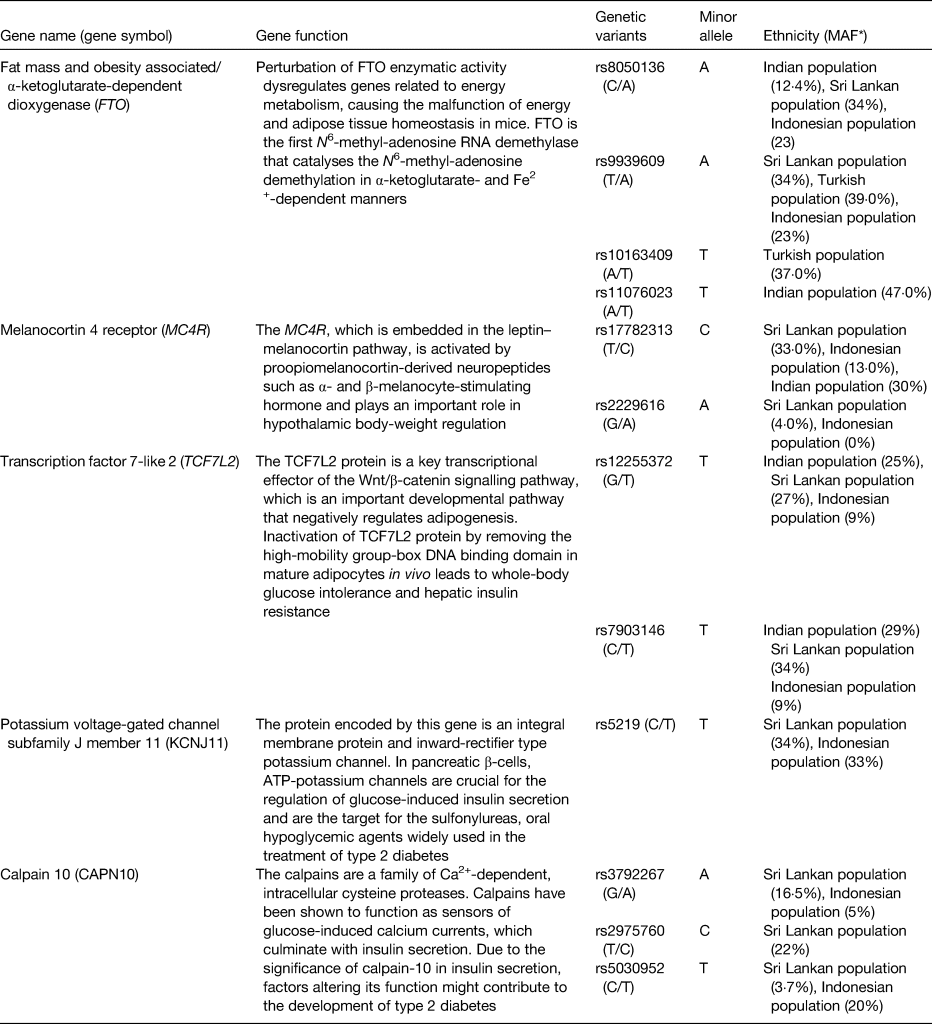
* MAF reported are from the studies investigated in the GeNuIne Collaboration.
The second study in Asian Indians examined whether the association of the melanocortin 4 receptor (rs17782313) and TCF7L2 (rs12255372 and rs7903146) SNP with cardio-metabolic traits is modified by dietary factors and physical activity in a random sample of participants who were normal glucose tolerant (n 821) and those with type 2 diabetes (n 861) recruited from the Chennai Urban Rural Epidemiology Study(Reference Bodhini, Gaal and Shatwan22). The study identified a significant interaction between the TCF7L2 SNP rs12255372 (Table 1) and fat intake (g/d) on HDL-cholesterol (P interaction = 0·0001), where the T allele carriers of the SNP had 2·26 mg/dl higher HDL-cholesterol level in the lowest tertile of fat intake (mean: 41 g/d) than the GG homozygotes (P = 0·008) and in the highest tertile of fat intake (mean: 95 g/d), HDL-cholesterol was 1·87 mg/dl lower in the risk T allele carriers in comparison with the GG homozygotes (P = 0·017) (Fig. 2). Further stratification to fat subgroups showed significant interactions between the TCF7L2 SNP rs12255372 and PUFA (g/d) on HDL-cholesterol (P interaction<0·0001), where the T allele carriers had 1·96 mg/dl higher HDL-cholesterol (P = 0·024) in the low PUFA tertile (mean: 9 g/d) in comparison with the GG homozygotes and in the third tertile (mean: 29 g/d), the HDL-cholesterol level of the T allele carriers was 1·64 mg/dl lower than the ‘GG’ homozygotes (P = 0·028) (Fig. 2). A similar interaction was also identified between the SNP rs12255372 and MUFA (g/d) on HDL-cholesterol (P interaction = 0·0003), where the T allele carriers had 1·77 (mg/dl) higher HDL-cholesterol in the lowest MUFA tertile (mean: 12 g/d; P = 0·03) and had 1·61 (mg/dl) higher HDL-cholesterol in the second tertile (mean: 18 g/d; P = 0·045) than the GG carriers, however in the highest MUFA tertile (mean: 29 g/d) the T allele carriers had 1·59 (mg/dl) decreased HDL-cholesterol (P = 0·041) than individuals with the GG genotype. PUFA was further stratified to linoleic acid and α-linoleic acid to investigate whether n-3 and n-6 fatty acids modified the association between the TCF7L2 SNP rs12255372 and HDL-cholesterol. Significant interaction was found between the SNP and α-linoleic acid on HDL-cholesterol (P interaction = 0·012), where the T allele carriers had 2·42 (mg/dl) higher HDL-cholesterol than the GG homozygotes (P = 0·004) in the lowest tertile (mean: 0·38 g/d). A similar interaction was also found between the SNP rs12255372 and linoleic acid (g/d) on HDL-cholesterol (P interaction<0·0001) (Fig. 2). These findings are of public health significance given that Asian Indians tend to have low HDL-cholesterol, which puts them at markedly increased risk for CVD(Reference Misra, Patel and Kotha41,Reference Joshi, Anjana and Deepa42) . The mechanism by which different fatty acids influence HDL-cholesterol levels and whether/how high-fat and high-PUFA intakes reduce HDL-cholesterol should also be established before public health recommendations and personalised nutrition advice can be developed for this Asian Indian population in order to reduce the burden of cardiometabolic diseases.
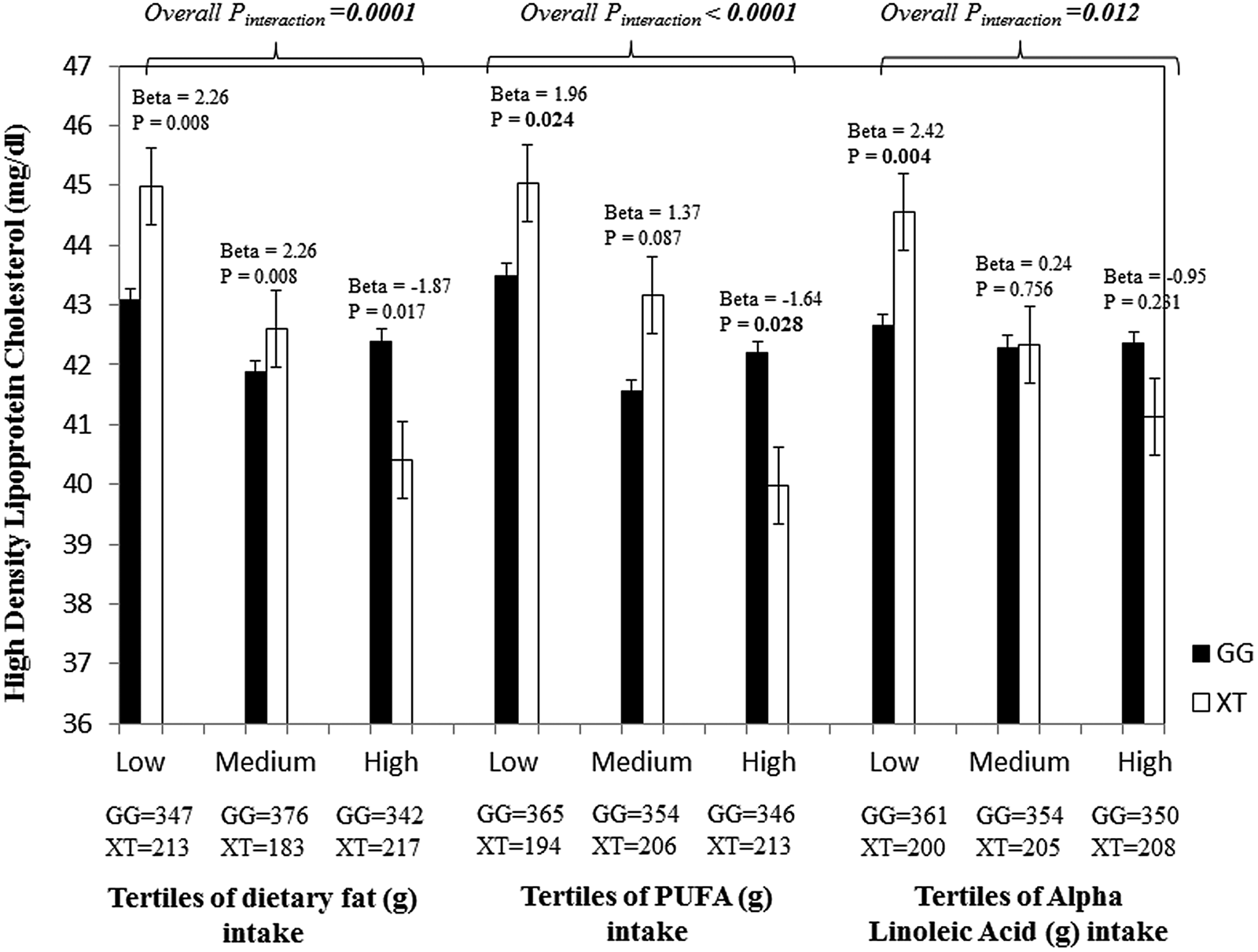
Fig. 2. Interaction of the TCF7L2 SNP rs12255372 with fat (g) intake, PUFA intake and α-linolenic acid (g) intake on HDL-cholesterol in Asian Indians. Individuals carrying the XT genotype had 2·26 mg/dl higher HDL-cholesterol in the lowest fat tertile (P = 0·008), while those in the highest tertile had 1·87 mg/dl lower HDL-cholesterol (P = 0·017) than those who carry the GG allele. Carriers of the XT genotype had 1·96 mg/dl higher HDL-cholesterol in the first tertile of PUFA intake (g) (P = 0·024), while those in the third tertile had 1·64 mg/dl lower HDL-cholesterol in comparison with the carriers of the GG genotype (P = 0·028). In the first tertile of α-linolenic acid intake (g), individuals with the XT genotype had 2·42 mg/dl higher HDL-cholesterol than the GG homozygotes (P = 0·004).
Given that, in recent years, the incidence of obesity in Sri Lanka has increased markedly(Reference Jayawardena, Byrne and Soares43), the third study of GeNuIne Collaboration was carried out in the city of Colombo, Sri Lanka. The Genetics of Obesity and Diabetes study is a cross-sectional study that was conducted in Colombo, Sri Lanka, between April and August 2017 to explore the interaction between genes and dietary intake on metabolic traits in 109 Sinhalese adults(Reference Surendran, Alsulami and Lankeshwara44). Dietary intakes were assessed using a previously validated and published(Reference Jayawardena, Byrne and Soares45) interviewer administered FFQ containing 85 food items. The global physical activity questionnaire, developed by the WHO, was used to measure physical activity(Reference Armstrong and Bull46). A genetic risk score (GRS) based on ten metabolic disease-related SNP previously associated with obesity and diabetes was constructed. The Genetics of Obesity and Diabetes study identified a significant interaction between the GRS and carbohydrate energy intake (%) on the waist:hip ratio (P interaction = 0·015) (Fig. 3). Individuals who carried eight or fewer risk alleles for the metabolic disease had 7·47 % lower waist:hip ratio measurements (cm) in the highest tertile of carbohydrate energy intake (%) (mean: 78·00 (sd 7·90) %) compared to those with nine or more risk alleles (P = 0·035). Interactions were also seen between the metabolic-GRS and carbohydrate energy (%) on log fasting insulin concentrations (P = 0·011) and log waist circumference (P = 0·031), and the metabolic-GRS and protein energy (%) on log fasting insulin levels and (P = 0·032) and log waist circumference (P = 0·011). Given that the total daily intake of carbohydrate is high in Sri Lankan adults(Reference Jayawardena, Thennakoon and Byrne47), our findings, if replicated in future studies using larger cohorts, might carry significant public health implications.
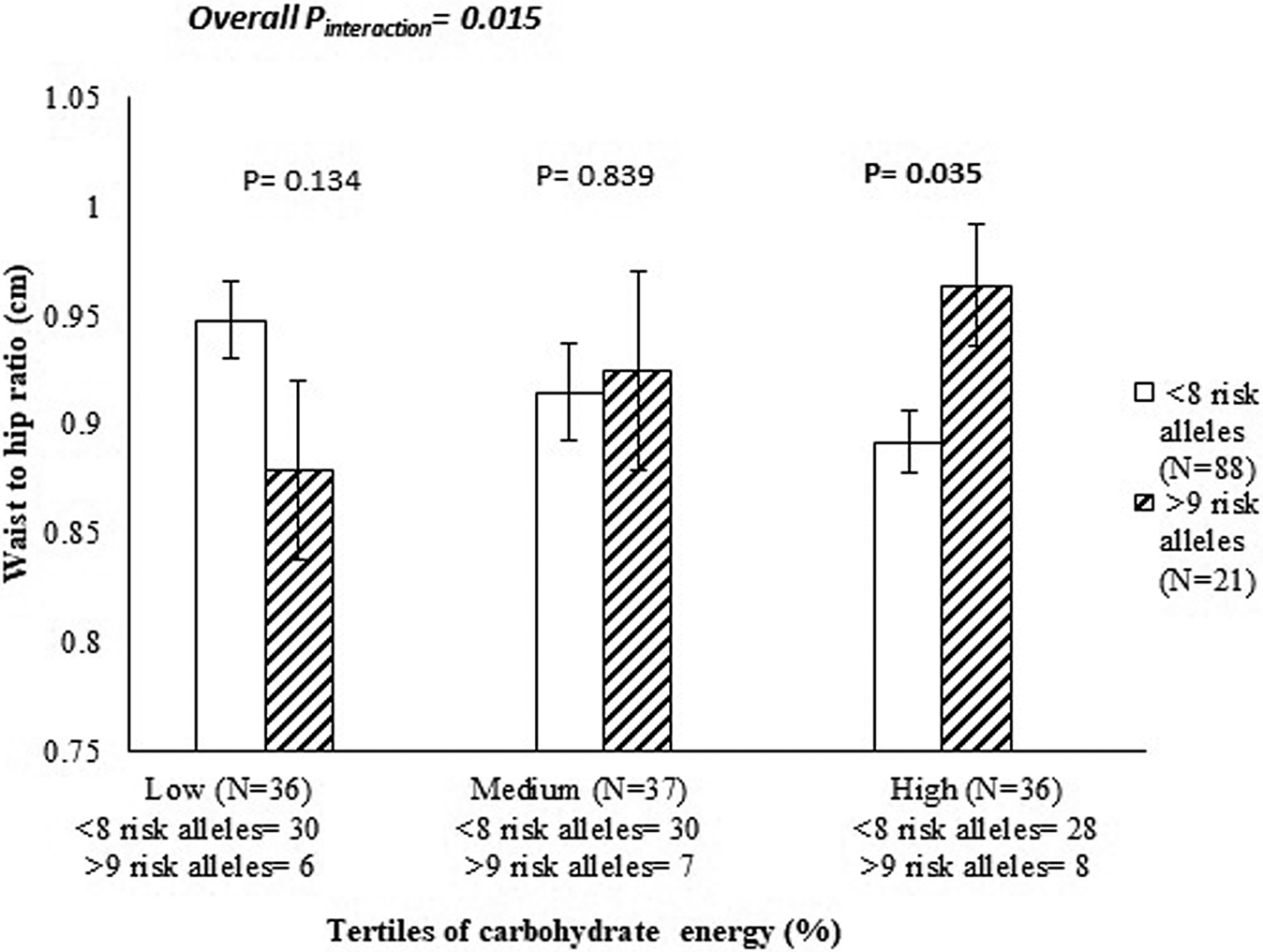
Fig. 3. Interaction between the genetic risk score and carbohydrate energy intake (%) on waist:hip ratio (cm) (P interaction = 0·015) in Sinhalese adults, where among those who consumed a high-carbohydrate diet, individuals who carried nine or more risk alleles had significantly higher levels of waist:hip ratios compared to individuals carrying eight or fewer risk alleles (P = 0·035).
Nutrigenetic studies in South East Asia
Indonesia has the seventh largest number of diabetic patients (7·6 million), despite relatively low prevalence (4·8 %) in 2012(Reference Soewondo, Ferrario and Tahapary48). It is estimated that the Western Pacific has more than 138·2 million people with diabetes in 2013, and the number is expected to rise to 201·8 million by 2035(49). In Indonesia, non-communicable diseases are estimated to account for 63 % of the total number of deaths(50). Of the total, CVD contributed 30 % followed by cancers (13 %), and diabetes (3 %)(50). Indonesia is a multi-ethnic country with over 300 ethnic groups. It has been reported that the West Sumatera province, where most of the Minangkabau ethnic group lives, had the highest proportion of inpatients with CVD among thirty provinces in Indonesia(Reference Boedhi-Darmojo51). The Minangkabau is a matrilineal society, where women hold greater power in both family and society(Reference Stark52). Food supply is centred around women and compelling evidence suggests that adequate nutrition protects against metabolic disorders related to obesity(Reference van Baak53), as a result understanding the dietary patterns of this sub-group of women in relation to their genetic susceptibility is of great importance.
The Minangkabau Indonesia Study on Nutrition and Genetics is a cross-sectional pilot study that was conducted in the city of Padang, West Sumatra, Indonesia, between December 2017 and January 2018. This study was conducted as part of the on-going GeNuIne Collaboration. A total of 117 women were recruited from community health centres in two sub-districts in Padang City to represent both urban (50 % Padang Timur) and rural (50 % Kuranji) areas of Padang population. Dietary intakes were assessed using a previously validated and published semi-quantitative FFQ containing 223 food items(Reference Lipoeto, Agus and Oenzil54). The global physical activity questionnaire was used to measure physical activity(Reference Armstrong and Bull46). Nine metabolic disease-related SNP (Table 1) were selected for the Minangkabau Indonesia Study on Nutrition and Genetics study based on the previously published candidate gene and genome-wide association studies for metabolic disease-related traits(Reference Bodhini, Gaal and Shatwan22,Reference Illangasekera, Kumarasiri and Fernando55–Reference Gloyn, Weedon and Owen62) and a GRS was generated from these nine SNP. The study identified a significant interaction between the GRS and protein (energy %) on log-transformed waist circumference (P = 0·032) (Fig. 4), where individuals who carried five or more risk alleles for metabolic disease had 2·15 % lower waist circumference measurements (cm) in the lowest tertile of protein energy intake (mean: 1·91 (sd 0·06) %) compared to those with four or less risk alleles (P = 0·027). This finding was in accordance with a study in 711 individuals of Caucasian ancestry(Reference Mohan and Deepa36), which had also shown an interaction between total protein intake and a GRS of sixteen obesity/lipid metabolism polymorphisms on body fat mass. Given that several SNP were analysed in the study, correction for multiple testing was applied. After Bonferroni correction, none of the interactions were statistically significant; hence, further replication studies utilising larger sample sizes are needed to confirm these findings, before public health recommendations and personalised nutrition advice can be developed for Minangkabau Indonesian women.
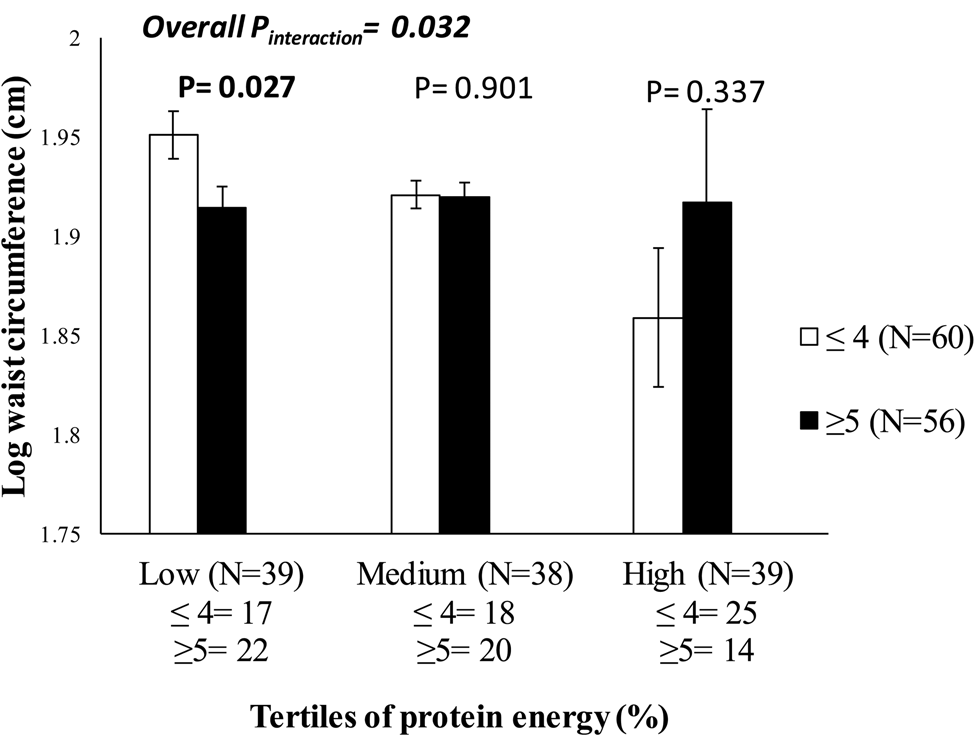
Fig. 4. Interaction between the metabolic-genetic risk score and protein energy (%) on log waist circumference (P interaction = 0·032) in Indonesian women, where among those who consumed a low-protein diet, individuals who carried five or more risk alleles had significantly lower waist circumference measurements compared to individuals carrying four or fewer risk alleles (P = 0·027).
Nutrigenetic studies in Western Asia
In Turkey, a transcontinental country located mainly in Western Asia, the prevalence of obesity has significantly increased by 40 % from 1998 to 2010(Reference Satman, Omer and Tutuncu63). In 2017, the overall prevalence of overweight and obesity in Turkish adults was 64·4 and 28·8 %, respectively(64). Turkish adults have distinctive characteristics compared to Europeans including low levels of total cholesterol and HDL-cholesterol, which confer an increased risk of CVD(Reference Onat65). In 2017, non-communicable diseases accounted for 88 % of deaths in Turkey, with CVD being the first cause of death accounting for about 48 % of all deaths(64). Several health promotion campaigns have been implemented in Turkey, including ‘move for health’ and ‘reducing portion sizes’, in order to reduce the prevalence of obesity which could eventually prevent CVD-related deaths(66,67) .
To date, no nutrigenetics studies have been conducted in a Turkish population. Given that gene–environment interactions might vary between populations because of genetic heterogeneity, it is important to investigate these interactions in different ethnicities to personalise healthcare according to each ethnic group. Hence, a total of 400 unrelated individuals (200 obese and 200 non-obese), aged 24–50 years, were recruited in Ankara, Turkey to determine whether FTO SNP, rs9939609 and rs10163409 (Table 1) were associated with obesity traits and whether these SNP interact with physical activity and dietary intake of macronutrients on obesity traits. Dietary intake was assessed using a 24-h dietary recall by trained research dietitians during a face-to-face interview with each participant. The Turkish version of the international physical activity questionnaire was used to assess the physical activity levels of the participants(Reference Saglam, Arikan and Savci68). The study identified a significant interaction between FTO SNP rs10163409 and protein intake (g) on the risk of increased waist circumference (P interaction = 0·044), where among individuals in the highest tertile of protein intake (mean: 138 (sd 38) g/d), carriers of the minor allele T of the SNP rs10163409 had a significantly higher risk of increased waist circumference (OR = 3·3 (95% CI 1·149, 9·478), P = 0·027) than those with AA genotype (Fig. 5). There was also a significant interaction between the FTO rs10163409 variant and dietary protein intake on waist circumference as a continuous variable (P interaction = 0·007). In addition, an interaction between the FTO SNP rs9939609 and physical activity levels on adiponectin concentrations were observed (P interaction = 0·027), where, among individuals with low-physical activity levels, carriers of the risk allele A of this SNP had significantly lower adiponectin concentrations than homozygous individuals for TT genotype (P = 0·006). These findings suggest that low levels of physical activity and a high-protein diet could increase the genetic risk of obesity in this Turkish population. Given that Turkish adults have low levels of physical activity and a sedentary lifestyle(64), our findings will have significant public health implications in terms of reducing the prevalence of obesity and CVD mortality(69).
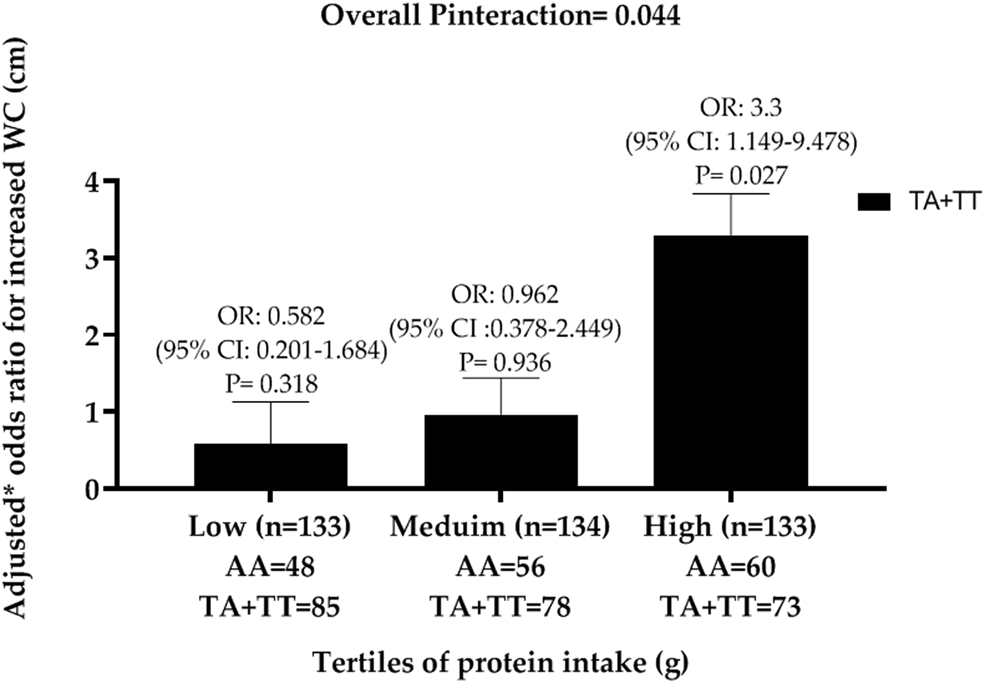
Fig. 5. Interaction between FTO SNP rs10163409 and protein intake (g) on central obesity (increased waist circumference (WC)) in a Turkish population. Black bars indicate the T allele carriers (TA + TT). OR are adjusted for age, sex, hypertension, CVD, total energy intake and obesity status.
Nutrigenetic studies in South America
CVD has remained the leading cause of mortality in Brazil since the latter part of the 1960s(Reference Schmidt, Duncan and Azevedo e Silva70,Reference Ribeiro, Duncan and Brant71) . Although effective tobacco control policies and access to improved healthcare have led to drastic improvements in cardiovascular health, an upward trend in unhealthy eating habits and physical inactivity has been observed in the Brazilian population(Reference Ribeiro, Duncan and Brant71). Epidemiological studies have shown that hyperhomocysteinaemia is a well-known independent risk factor for atherosclerotic vascular disease and hypercoagulability states(Reference Shenoy, Mehendale and Prabhu72). Studies have shown significant interactions between SNP involved in the C1 metabolism pathway and dietary factors on homocysteine concentrations(Reference Dedoussis, Panagiotakos and Chrysohoou73,Reference Steluti, Carvalho and Carioca74) . However, no studies, to date, have examined the interaction between C1 metabolism-related genes and lifestyle factors on vitamin B12, folate and lipid concentrations.
A cross-sectional study was conducted in a public school in the city of Goiânia, Goiás, Brazil, between March and May 2014(Reference Surendran, Morais and Abdalla75). A total of 113 adolescents (aged 10–19 years) were selected to answer a food frequency record and provided a blood sample for biochemical and DNA analysis. The global physical activity questionnaire was used to assess physical activity. Ten common SNP involved in the C1 metabolism pathway were selected based on the published reports(Reference Steluti, Carvalho and Carioca74,Reference Tanaka, Scheet and Giusti76–Reference Feng, Kalari and Fridley80) . The study identified significant interactions between the catechol-o-methyl transferase SNP (rs4680 and rs4633) and dietary carbohydrate intake on HDL-cholesterol concentrations (P = 0·011 and 0·036, respectively). In addition, an interaction was found between the catechol-o-methyl transferase SNP (rs4680) and dietary carbohydrate intake on oxidised-LDL concentrations (P = 0·005). Given that oxidised-LDL and hyperhomocysteinaemia are well-known independent risk factors for atherosclerotic vascular disease(Reference Shenoy, Mehendale and Prabhu72,Reference Papageorgiou and Tousoulis81) , our findings have significant implications for population health. These findings warrant confirmation in larger, well characterised and well-powered prospective studies/randomised controlled trials, before any public health recommendations and personalised nutrition advice can be developed for the adolescent Brazilian population.
Nutrigenetic studies in other developing countries
In several LMIC, nutrigenetics studies have not been carried out because expertise, infrastructure and funds are limited. As part of the GeNuIne Collaboration, nutrigenetics studies are currently being implemented in other LMIC including India (rural component), Peru, Ghana, Morocco, Thailand and Pakistan through funds from the Medical Research Council, Global Challenge Research Fund and the British Council Newton Fund. In addition, workshops on nutrigenetics and nutrigenomics are also being conducted supported by funds from local organisations in LMIC, the British Council and Newton funds to mediate knowledge- and technology-transfer to the LMIC.
Challenges and limitations in nutrigenetic research
Nutrigenetics is still quite a new research area and standardised protocols are not well-established in LMIC. Most often, results are difficult to replicate among populations due to population stratification, making conclusions difficult to draw. Furthermore, while most studies only consider one SNP in a single gene, personalised nutrition requires the knowledge of multiple GeNuIne to allow a more complete understanding of nutrigenetics. To overcome the challenges involved in examining single genes, several studies have examined the combined effect of genes/gene variants on metabolic outcomes in response to dietary intake(Reference Surendran, Alsulami and Lankeshwara44,Reference Vimaleswaran, Le Roy and Claus82) . Measurement accuracy is difficult to obtain as diet and nutrition are very complex to measure and inaccuracies of exposure measurements may introduce bias and make false conclusions about GeNuIne(Reference Pena-Romero, Navas-Carrillo and Marin83). Furthermore, GeNuIne studies need very large sample sizes; underpowered studies are responsible for poor reproducibility of GeNuIne outcomes. Therefore, a larger sample size is needed to find GeNuIne to identify an interaction effect of comparable magnitude.
We still do not fully understand the biological pathways between genes and cardiometabolic diseases, given that the identified SNP account only for a small proportion of the underlying metabolic variance, and hence genome-wide gene–diet interaction studies are required to identify novel loci(Reference van Leeuwen, Smouter and Kam-Thong84,Reference Gauderman, Zhang and Morrison85) . Another important challenge is the lack of appropriate statistical tools to accurately mine these ‘big data’, which represent enormous datasets. Further advancement in the field of statistics and bioinformatics is required to handle and integrate all the data generated by various analytical techniques. Developing such methods would significantly expand the power of large-scale studies and improve the possibility of discovering novel interactions. The next step would be to translate the large datasets generated by nutrigenetics studies into information that would form the basis for the identification of novel markers, which will lead to the development of personalised diets to reduce the burden of cardiometabolic diseases.
From nutrigenetics to personalised nutrition
Nutrigenetics studies have shown that genes and dietary factors can significantly influence the risk of developing cardiometabolic diseases(Reference Vimaleswaran and Loos5,Reference Vimaleswaran, Tachmazidou and Zhao86) . Although several SNP have been identified for cardiometabolic diseases using candidate gene(Reference Vimaleswaran, Tachmazidou and Zhao86) and genome-wide association studies(Reference Bradfield, Taal and Timpson14,Reference Willer, Speliotes and Loos18) , it has been shown that these SNP contribute to the development of the disease only under an obesogenic environment(Reference Rhee, Phelan and McCaffery87). While advances in the field of high-throughput genetic analysis have shown the contribution of SNP to cardiometabolic diseases, the molecular and pathophysiological mechanisms underlying these gene–lifestyle interactions remain unexplored. Functional studies are required to understand their biological significance and their potential application in personalised medicine. Besides genes and diet, the gut microbiota and gene–diet–microbe interactions can also modify the risk of developing cardiometabolic diseases(Reference Hu88,Reference Qi and Cho89) . Diet and gut microbiota are major components of the exposome that interact together with a genetic make-up in a complex interplay to result in an individual's metabolic phenotype. Given that gut microbiota also plays an important role in metabolic homeostasis, it is crucial to examine metagenome–hyperbolome–diet interactions to understand how nutrients can alter the metabolic phenotype and health outcome. Furthermore, foodomics approaches (such as nutrigenomics, nutrimetabolomics, nutritranscriptomics, nutriproteomics and metagenomics) are essential tools to assess an individual's optimal metabolic space(Reference Vimaleswaran, Le Roy and Claus82). Before this can effectively translate into clinical practice, and become available to health professionals, the data generated by these ‘omics’ approaches must be integrated to provide a full understanding of the systemic metabolism that results from these intricate relationships. The full potential of personalised nutrition requires in-depth knowledge of physiological pathways and several biomarkers, delivering a comprehensive platform picture of an individual's metabolic status. Furthermore, by taking into account the cultural and socio-economic status of the ethnic group under study, nutrition-specific interventions programmes can address the immediate determinants of nutrition status (e.g. inadequate diet and disease burden) and are found in a range of policy areas, such as health, humanitarian relief, and food processing(Reference Bhutta, Das and Rizvi90).
The use of an evidence-based approach is very important in nutrigenetics and in order to provide more scientific evidence between gene–diet interactions, there is a need for more studies and more variety in examined populations. The investment in intervention studies which will include more people from a diverse range of ethnic groups and extensive genotyping along with deeper, standardised phenotyping will give more promising results for the prevention and treatment of cardiometabolic diseases. Consideration of multiple gene–nutrient–environment interactions is important to provide accurate personalised nutrition recommendations in the future. Hence, the combined application of nutrigenetics and nutrigenomics with molecular and metabolite profiling to define an individuals' metabotype will be required to provide the basis for implementing personalised nutrition for cardiometabolic disease prevention.
Acknowledgements
Dr Karani S. Vimaleswaran acknowledges support from the British Nutrition Foundation and thanks the support from the GeNuIne Collaborators:
• Hugh Sinclair Unit of Human Nutrition and Institute for Cardiovascular and Metabolic Research, Department of Food and Nutritional Sciences, University of Reading UK: Professor Julie Lovegrove.
• Dr Mohan's Diabetes Specialties Centre, Chennai, India: Professor Mohan Viswanathan, Dr Anjana Mohan, Dr Guha Pradeepa.
• Department of Molecular Genetics, Madras Diabetes Research Foundation, Chennai, India: Dr Radha Venkatesan, Dr Bodhini Dhanasekaran.
• Department of Foods, Nutrition and Dietetics Research, Madras Diabetes Research Foundation, Chennai, India: Mrs Sudha Vasudevan.
• Faculty of Nutrition, Federal University of Goiás, Goiânia: Dr Maria Aderuza Horst, Dr Cris Cominetti.
• Faculty of Medicine, Andalas University, Padang, Indonesia: Professor Nur Indrawathy Liputo, Dr Finny Fitri Yani.
• Faculty of Medicine, Universitas Hasanuddin Makassar, Indonesia: Professor Nurpudji A. Taslim.
• Department of Nutrition, Medical School, Sam Ratulangi University Manado, Indonesia: Dr Nelly Mayulu.
• Grupo de Análisis para el Desarrollo, Lima, Peru: Dr Alan Sánchez.
• Instituto de Investigación Nutricional, Lima, Peru: Professor Mary E. Penny.
• Department of International Development, University of Oxford, UK: Dr Marta Favara.
• Faculty of Health and Social Care, University of Chester, Chester, UK: Professor Basma Ellahi.
• Biochemistry and Biotechnology Department, College of Science, Kwame Nkrumah University of Science and Technology, Kumasi, Ghana: Dr Reggie Annan.
• Department of Nutrition and Dietetics, Hacettepe University, Ankara, Turkey: Dr Zehra Buyuktuncer-Demirel.
• Department of Non-Communicable Disease Epidemiology, London School of Hygiene and Tropical Medicine, London, UK: Dr Sanjay Kinra.
• Université Hassan II de Casablanca, Laboratoire de Biologie et Santé, Faculté des Sciences Ben M'Sik, Unité de Recherche en Nutrition Humaine, Casablanca, Morocco: Professor Abdelfettah Derouiche, Dr Ali Jafri.
• Department of Food Science and Technology, Faculty of Agro-Industry, Kasetsart University, Bangkok, Thailand: Dr Parichat Hongsprabhas, Dr Sudathip Sae-tan.
• National Institute of Food Science and Technology, Faculty of Food, Nutrition and Home Sciences, University of Agriculture, Faisalabad, Pakistan: Dr Mian Kamran Sharif.
Financial Support
None.
Conflict of Interest
None.
Authorship
K. S. V. is the Principal Investigator of the GeNuIne Collaboration, where he had conceived, supervised, obtained funding and designed the studies in various ethnic groups.