What are the consequences of committing violent attacks for the terrorist organizations? In the end, do they attract more supporters? Scholars and pundits alike generally refer to the existence of two opposing effects in their description of the consequences of violent terrorist activity. On the one hand, terrorist attacks may signal organizational capacity or fulfill members’ ideological goals to an extent that solidifies and broadens the base of supporters within the organization (Acosta Reference Acosta2014b; Doosje et al. Reference Doosje, Moghaddam, Kruglanski, de Wolf, Mann and Feddes2016). On the other hand, attacks might also lead supporters to believe that the organization is excessively violent or involvement may become too dangerous, which might make violence counter-productive for the size of their support base and reduce their supporters (Denardo Reference Denardo1985). In this article, we provide an empirical test of the broader argument over the utility of terrorism for militant groups by focusing on the impact of deadly attacks on the organizational support base of militant groups. Specifically, we systematically analyze the influence of terrorism on the number of supporters in the context of the Islamic State (IS). We contribute to the empirical literature of terrorism by providing an answer about which effect dominates among those segments of the population that are on the cusp of being outsiders and insiders regarding a terrorist organization. We do so by exploring the dynamics in those groups relative to IS on their most important communication and recruitment tool: Twitter.
We argue that the number of followers of IS-linked Twitter accounts reveals crucial information about the (de)mobilization dynamics regarding the relationship between terrorist organizations and societies. While we are not able to empirically move beyond the follower–non-follower dichotomy, we provide a useful framework from which to theoretically think about who moves across the insider–outsider boundary when we observe shifts in the overall number of followers. Hence, we begin by providing a theoretical mapping of Twitter followers into three types: (1) organization supporters, such as leaders, active members who unconditionally support the organization and latent sympathizers; (2) observers, non-supporters who follow IS-linked accounts to acquire information, such as media reporters, or individuals fighting against IS, but who would never support the organization; and (3) non-followers. The first group are the insiders of the organization in the sense that they have shown a degree of sympathy toward it; the second group – as well as a third group composed of the entire population of Twitter non-followers – constitute the outsiders. Hence, the overall change in the number of followers before and after an attack reflects three types of effects depending on the type of individuals who move across categories. Terrorist attacks may have: (1) attentional effects, non-followers may become observers: (2) mobilization effects, non-followers may turn into insiders; (3) demobilization effect, insiders may become outsiders after an attack either because of a disengagement effect, a change in beliefs leads to a reduction in support, or a deterrence effect, an increase in the fear of being tracked and prosecuted for their links with radical or violent activity.
To test the theoretical inequality of interest, we have implemented an automatic routine that collected the daily reports made by Anonymous on IS-related Twitter accounts and extracted key information on these accounts over 127 days–from 14 March to 22 July 2016. Altogether, we collected information on 300,842 observations, account-date data points, on 13,321 unique Twitter accounts.Footnote 1 Then, we merged these with the data on real-world data on terrorist attacks around the world that occurred during our period of study. Using this constructed dataset, we examine whether terrorist attacks linked to IS exert an impact on the number of followers of IS-related accounts by combining observational and quasi-experimental research designs.
Different methodological perspectives provide a strong empirical regularity: IS terrorist attacks decrease the number of followers of IS-related Twitter accounts in the aftermath of an attack. The estimated effect regarding Twitter followers is both statistically and quantitatively significant. We employ two empirical strategies with identical conclusions. First, we test our main hypothesis by using an interrupted time series analysis. In this case, we explore the discontinuous changes in the number of followers around two major attacks in our dataset: the Brussels bombing on 22 March 2016 and the attack in Nice on 14 July 2016. Second, we exploit the panel structure of our dataset to examine the effect of the intensity of a terrorist attack on the number of followers in their accounts using several model specifications, including random and fixed effect models, which exploit only variation in the number of followers within each account. By doing this, we observe that terrorist attacks precede fluctuations over time in the number of followers in IS terrorist attacks; this is true especially for attacks that occurred on European soil as compared to other attacks in Asia and Africa for which the effect is still negative and significant, but smaller in magnitude. Though we detect a surge in the number of accounts that are reported to Twitter for their potential links to IS, our estimated effects of terrorist attacks remain negative and significant after adjusting for such activity. Hence, both analyses consistently point to the negative effect of the attacks on the number of followers of the terrorist organization on Twitter.
Overall, this article speaks to several strands of literature. First, we offer a critical theoretical account on the types of effects that terrorist attacks may have on a terrorist organization, as well as its relationship to the population. Second, we present novel systematic evidence on the impact of terrorist attacks on the disengagement of probable terrorist supporters. Though there is some prior empirical research on the consequences of terrorism on some relevant political outcomes, including people’s attitudes and ideology (see Peffley, Hutchison and Shamir Reference Peffley, Hutchison and Shamir2015) and governmental policies (Abrahms Reference Abrahms2012), this study contributes further to this research strand by systematically examining the impact of the use of violence on potential supporters of terrorist organizations. Third, our work contributes to the growing literature that employs big data to answer long-standing questions in political science (Barbera Reference Barberá2015; Barbera et al. Reference Barberá, Jost, Nagler, Tucker and Bonneau2015; King, Pan, and Roberts Reference King, Pan and Roberts2014) by using a novel dataset on IS-related Twitter accounts. Methodologically, our work combines several features of computational research in social science pinpointed by Salganik (Reference Salganik2018) as we combine ‘always-on’ data from Twitter with a ‘traditional’ dataset on the timings of the terrorist attacks. Also, our work ‘approximates experiments’ connecting to the heated debate in political science on whether big data and causal inference are contradictory trends by employing big data in a quasi-experimental framework (Monroe et al. Reference Monroe, Pan, Roberts, Sen and Sinclair2015).
Terrorist Attacks: Mobilization, Disengagement and Deterrence
There are several possible explanations for the relationship between terrorist attacks and the support base of terrorist organizations. A number of factors, including the psychology of individual terrorists (Horgan Reference Horgan2005; Victoroff and Kruglanski Reference Victoroff and Kruglanski2009), adherence to religious ideals (Pargament, Magyar-Russell, and Murray-Swank Reference Pargament, Magyar-Russell and Murray-Swank2005), socialization processes (Turk Reference Turk2004), or their contextual socio-economic factors (Mitra et al. Reference Mitra2008; Piazza Reference Piazza2011) may explain the variation in the activities of terrorist groups. In this article, we focus on the influence of deadly terrorist attacks on the support base of terrorist organizations.
An influential view on the purpose of terrorist attacks in political science suggests that terrorist groups use violence as a costly signal to show strength or capacity (Hoffman and McCormick Reference Hoffman and McCormick2004; Kydd and Walter Reference Kydd and Walter2006; Siegel and Young Reference Siegel and Young2009). Even though some scholars argued that terrorism may be effective at achieving policy concessions from the targeted states (Pape Reference Pape2003, Reference Pape2005), an emerging strand of literature shows that violent radical groups tend to be less likely to achieve their larger political ends than groups using non-terrorist strategies (Abrahms Reference Abrahms2012; Acosta and Childs Reference Acosta and Childs2013; Fortna Reference Fortna2015).
Rather than coerce policy concessions, other scholars propose that ideological drivers, the fulfillment of cultural expectation of particular identity groups, the pursuit of nonmaterial objectives or even the outright elimination of adversaries constitute the major goals of modern terrorist organizations (Abrahms Reference Abrahms2008; Acosta Reference Acosta2016; Grygiel Reference Grygiel2013; Moghaddam Reference Moghaddam2005). Regardless of their objectives, terrorist attacks certainly change the political position of the group through the provision of new information about them to governments, people or both.Footnote 2 For instance, Lyall, Blair, and Imai (Reference Lyall, Blair and Imai2013) showed how patterns of wartime violence strongly affected civilians’ support for combatant groups in the context of Afghanistan. Following this line of inquiry, we argue that terrorist activities provide new information about the effectiveness and objectives of the organization, which may shape the support for militant groups through two opposed processes: mobilization and demobilization.
On the one hand, terrorist attacks may mobilize supporters by increasing the perceived group efficacy. Terrorist violence is a strategic mobilization tool that aims at ensuring a ‘self-sustaining rate of political mobilization’ (Acosta Reference Acosta2014a), solidifying the loyalty of the already militant members, and broadening the base of supporters to ultimately institutionalize the organization (Acosta Reference Acosta2009, Reference Acosta2014a, Reference Acosta2016; Bueno de Mesquita and Dickson Reference Bueno de Mesquita and Dickson2007). In this sense, Doosje et al. (Reference Doosje, Moghaddam, Kruglanski, de Wolf, Mann and Feddes2016) argue that an increase in the levels of perceived group efficacy in their strategic or ideological goals is a crucial determinant in the micro-level process of radicalization. The declaration of the Islamic States in Iraq and Syria, for instance, arguably increased the perceived capacity of the IS, which helps to explain the large number of foreign fighters who traveled to IS-controlled areas.
On the other hand, not only does the nature of the attacks signal strength to achieve their ends, but it also conveys relevant information to their supporters about the kind of strategies they use, the character of their members and the goals of the organization (Bueno de Mesquita and Dickson Reference Bueno de Mesquita and Dickson2007; Hoffman and McCormick Reference Hoffman and McCormick2004). In this context, we can expect demobilization processes among supporters of terrorist groups after attacks. In light of excessively violent attacks, sympathizers might become morally repulsed (disengagement mechanism) and/or fearful of the personal costs from reprisal (deterrence mechanism). We discuss each mechanism in turn.
Violent terrorist attacks provide new information about the organizational capabilities, the character and the goals of the organization that might backlash and lead to disengagement. Specifically, some individuals who might have sympathized with the organization in the past may come to realize that they cannot cope with new levels of violence. Though idealistic expectations about the organization may have led some to follow or actively engage in its activities, terrorist acts may lead once-supporters to believe that violence has gone too far. This argument is not new. In his seminal contribution on the effectiveness of violent tactics, Denardo (Reference Denardo1985) suggested that most people find violence repulsive and regard political strategies that involve the use of violence as reprehensible. He also argued that radical organizations that ‘let themselves go on a rampage of destruction and killing often find their political base withering away’ (Denardo Reference Denardo1985, 192–93). Similarly, Lyall, Blair, and Imai (Reference Lyall, Blair and Imai2013) show how wartime violence generally decreases people’s support for combatant organizations.Footnote 3 Therefore, political movements may reduce moral attractiveness for some of their sympathizers or would-be sympathizers if their use of violence is excessive.
Recent qualitative empirical evidence suggests that disengagement may be a common process among members of terrorist organizations. In work with exit programs in northern Europe, Bjørgo (Reference Bjørgo2011) showed that individuals who belonged to extreme-right movements often exited those organizations because they felt that there had been too much violence. Studies of the determinants that led to a reduction in the number activists linked to the Basque terrorist group Euskadi Ta Askatasuna (ETA), Alonso (Reference Alonso2011) and Reinares (Reference Reinares2011) report evidence showing that many fighters exited the organization because of disagreements with the violent tactics of the organization. In a similar vein, Moghadam’s (Reference Moghadam2012) in-depth study of the decline of the West German Red Army Faction (Rote Armee Fraktion, RAF) revealed that disagreements over tactics and strategies led to an update of beliefs about the organization that initiated their disengagement with the organization.
Another driver of behavioral changes after terrorist attacks is deterrence. Those people who belong to radical organizations must face the personal consequences of violent action (Denardo Reference Denardo1985). As governments exert greater pressure over terrorist groups after violent activity, the personal costs for organizational belonging may increase, or at least, the perception among supporters of terrorist organizations of prosecution and surveillance. In this regard, Alonso (Reference Alonso2011) reports changes in beliefs among members and sympathizers of ETA in the aftermath of violent attacks due to fear from the attack or successful police prosecution in its aftermath. However, deterrence is less likely to work in cases where terrorists do not have a known address (Schmid Reference Schmid2013). However, the effectiveness of deterrence is not the same across all countries, but it depends on the credibility of a state to punish and threaten to retaliate against members of the organization (Wenger and Wilner Reference Wenger and Wilner2012). Hence, the credibility to exert deterrence on terrorists is likely to depend on the national material capabilities of states to allocate resources in security, defense and intelligence services (Hendrix and Young Reference Hendrix and Young2014). In the context of the modern terrorist organizations that execute a great share of their activity using online tools of communication and recruitment all users can potentially be tracked by intelligence services. However, states’ ability to selectively punish terrorists after an attack depends on their bureaucratic capacity for controlling, monitoring and collecting intelligence on suspects (Blankenship Reference Blankenship2018). Consequently, post-attack deterrence processes are likely to change the behavior of some supporters of the organizations by leading them to go offline out of fear of being tracked, especially in those countries where intelligence services are more credible to threaten retaliation.
Islamic State on Twitter
An increasing number of terrorist groups make an intensive use of mass media and social media to disseminate their messages (Rudner Reference Rudner2017). The availability of these new technologies has made it far easier to distribute the groups’ films and images: terrorist attacks and violent scenes are broadcast uncensored, which has led to an unprecedented stream of online violence. Besides the use of regular television channels to promote their films in the news, terrorist organizations have begun to be highly involved in a number of decentralized digital platforms through social media networks like Twitter or Facebook; peer-to-peer messaging apps like Telegram and Surespot; and content sharing systems like JustPaste.it (Clark Reference Clark2016). While this has been the case for a large number of terrorist organizations worldwide, the Islamic State has intensively used Twitter for both propaganda and recruitment purposes as it offers great technical advantage for the global dissemination of text and image messages (Klausen Reference Klausen2015). In this regard, FBI Director James Comey argued in July 2015 that IS strategy consists in ‘broadcasting on Twitter, get people to follow them, then move them to Twitter Direct Messaging’. After a short screening of whether the follower is a likely recruit, then ‘they’ll move them to an encrypted mobile-messaging app so they go dark to us’ (Aspen 2015).
In this article, we argue that the number of followers of IS-linked Twitter accounts reveals crucial information about the (de)mobilization dynamics regarding the relationship between terrorist organizations and societies. To see how changes in IS-related accounts reflect support of IS, we first classify users into three categories according to their relationship with IS Twitter-related accounts: (1) IS leader members, fighters, active supporters and sympathizers – they constitute owners of IS-related accounts and the followers of the IS-related accounts; (2) the observers: the people who do not support, but follow their accounts because they are consumers of IS information (for example, media reporters who follow the latest news in Syria through IS Twitter accounts) or people fighting IS; and (3) the non-followers: they are all other users on Twitter who do not follow and do not support IS activities currently, yet all of them could turn into IS passive or active supporters in future periods. This group constitutes the vast majority of Twitter users.Footnote 4
Therefore, terrorist attacks are shocks that reshuffle the number of people in each of the three types of users, which map into the types of movements that we described in the theoretical discussion above.Footnote 5 First, mobilization effects occur when a non-follower becomes an explicit supporter of the organization by following it on some of their related accounts. In a second place, attentional effects define aggregate movements of observers toward IS-related Twitter accounts; they are non-followers who begin following their accounts with the simple purpose of obtaining first-hand information on the organization and their activities (such as media reporters), yet they have no chance of becoming supporters of the organization. If terrorist attacks either generate mobilization and/or attentional effects, then we should expect an increase in the number of followers after deadly IS terrorist attacks. This leads to the following hypothesis:
Hypothesis 1.1: (mobilization and/or attention hypothesis): Terrorist attacks increase the number of followers on IS-related accounts.
As we theoretically discussed above, terrorist attacks may also demobilize supporters via disengagement or deterrence. On the one hand, some supporters may update their beliefs over the organization and may realize that the organization does not fulfill their desires, so they withdraw their support from the organization and un-follow IS-related accounts. In addition, some previous IS followers may stop following IS-related accounts not because they change their preferences or beliefs but out of fear of being tracked and associated with terrorist organizations. If either effect or both are at work after terrorist attacks, then we should expect a decrease in the number of followers after deadly IS terrorist attacks. These effects lead to the following hypothesis:
Hypothesis 1.2: (demobilization hypothesis): Terrorist attacks decrease the number of followers on IS-related accounts.
The nature of the treatment analyzed in this article – deadly terrorist attacks – should not be interpreted as the attacks alone, but should be conceptualized in a broader sense. The actual treatment includes the entire causal chain that a terrorist attack triggers, including reactions to the attack by other citizens, media, politicians, institutional figures and the like. Hence, this compounded treatment is obviously larger as the salience of the attack is greater. In brief, it is reasonable to expect that attacks that produce a larger number of deaths and geographically located in Western countries both provide a more costly signal and generate greater international turmoil, we should expect that if the attacks are the actual treatment in our empirical findings, then these two indicators should be influencing the changes in the number of supporters to the organization. In sum, the logic of the heterogeneous strength of the attacks gives rise to the following empirical expectation:
Hypothesis 2: (salience hypothesis): Terrorist attacks influence the number of followers on IS-related accounts, especially after attacks in Western countries.
The crucial distinction between disengagement and deterrence in the demobilization theoretical argument is whether the change in individuals’ behavior is the result of learning from the attacks and, thus, a shift in beliefs; or the consequence of fear or threat of online or offline prosecution. Though we acknowledge that teasing out the mechanisms behind the demobilization effect is out of the scope of this article, and unfeasible with our empirical data, we might provide some preliminary evidence for either disengagement or deterrence mechanisms by exploiting the heterogeneity in our data.
According to the deterrence effect, terrorist attacks increase the beliefs among followers that their behavior may become noticeable and tracked by security agencies, which leads to a change in behavior but not in beliefs. In the context of IS on Twitter, IS supporters may ‘go dark’ on Twitter by unfollowing IS-related accounts after a terrorist attack, which would explain a potential decrease in the average number of supporters. If the deterrence effect dominates the potential negative result, then we should observe that those followers located in the countries with a more credible threat to prosecute or retaliate against online activists, or the countries with greater national material capabilities, should be more likely to drop from following IS-related accounts in the aftermath of an attack. Testing this hypothesis means testing for the relevance of the deterrence over the disengagement mechanism conditional on the confirmation of the demobilization hypothesis.
Therefore, we can utilize the known geographic location of some accounts to test for the following deterrence effect. Note that if deterrence is the only driver of a demobilization effect, we should observe evidence for Hypothesis 3. If we do not find evidence for a deterrence effect, this implicitly suggests that the demobilization effect is likely to be due to a disengagement effect.
Hypothesis 3: (disengagement/deterrence hypothesis): Demobilization effects are especially strong among those accounts that are geographically located in countries with strong states; that is, countries with sufficient capabilities to allocate resources in security, defense and intelligence services.
Data Extraction on IS-Related Accounts
We construct our dataset by following ‘the reports’ of the anti-IS Twitter bots set up by the international hacker initiative Anonymous. This hacker group declared cyberwar on IS almost immediately after the IS attack in Paris on 13 November 2015. After that horrible event, the anti-IS initiative started to work in multiple directions, one of which was to report IS-related accounts in Twitter.Footnote 6 This article makes use of 300,843 reports from the anti-IS Anonymous bots collected over the period from 16 March 2016 to 20 July 2016. Each report is a Twitter account. Most of the accounts in this dataset have been reported multiple times and eventually got suspended (hence, there is a clear life-cycle of the accounts in the sample: they were reported the first time, then they were reported a couple of more times, then they were suspended). Each time when a certain Twitter account was reported, its profile information was collected. The gathered data are an unbalanced panel, in which n is a reported Twitter account and t is the date of this report. Overall, the final dataset has information on 13,321 unique accounts.Footnote 7 Each entity in the data contains information on the number of followers, date of the report, language of the account, time-zone and the number of friends, favorites and statuses on each reported account for every time the account was reported. Our dependent variable is the number of followers of a twitter account on date t.Footnote 8
The cumulative lagged measure of terrorist attacks
We complement the information extracted from the accounts reported by Anonymous with data on daily terrorist incidents linked to IS. We include an incident in our dataset if an authoritative figure, such as a country’s president or vice-president, addresses the nation by stating or strongly suggesting that an attack is committed by the IS. In the absence of direct information from a governmental authoritative figure, we include episodes that have been connected to IS by reliable media sources within a few hours of the attacks. Notice that the inclusion criterion of a case depends on the general perception of the attack in the immediate aftermath of the attack, as opposed to the result of formal investigations.Footnote 9
Hypothesis 2 reflects the idea that the effect of attacks on (de)mobilization are greater as the general impact of the attack is larger. As argued earlier, this impact factor may be related to two components: the number of casualties and the location of the incident. For this reason, we construct two independent variables for each day in our period taking into account these two parameters. First, we compute the number of deaths from terrorist attacks linked to IS that occurred in the United States and Europe. Secondly, we do the same for the number of deaths outside the United States and Europe. Hence, our independent variables do not reflect just whether there is an attack on a particular day, but they weight each attack for the number of deaths that it generates, and differentiate between those in Europe and the US and elsewhere. If our empirical strategy reflects our theoretical reasoning, then we should observe that the effect of the number of victims in the US and Europe should be larger than the effect of casualties located geographically elsewhere.Footnote 10
However, the assumption of an instant effect of a terrorist attack lasting only one day is unrealistic. Hence, a smoothing temporal approach for effect is a logical decision. Indeed, media coverage and news stories linger for some days after an attack. Consequently, we should expect to observe the consequences of an attack on the same day of the attack and over its immediate aftermath, although, probably, decreasing in magnitude. For this reason, we have chosen to implement a smoothing decreasing curve after the shock by applying a discount factor of 50, 75 and 100 per cent to the number of deaths. For each day in our dataset, we compute the cumulative mortality parameter, cd t; it aggregates all previous deaths from the terrorist attacks with a discount factor:

where i is a terrorist attack on day t(i) and deaths(i) is the number of deaths it caused; r is the discount rate applied, and (t−t(i)) is the number of days since the incident. This is summed for all incidents before t.Footnote 11 In the online Appendix E, we estimate the cumulative death parameter around the Brussels bombings on 22 March 2016, with r=0.5, to show that our selected discount rate parallels the changes in the keywords ‘Brussels+bombing’ reported by Google Trends.Footnote 12
To illustrate the incidence of violence linked to IS within our period of study, Figure 1 reports the cumulative lagged measure of deaths from terrorist attacks linked to IS from our initial extraction date (16 March 2016) to the end of the data collection period (20 July 2016). Altogether, there are two major attacks in European soil: on 22 March in Brussels, Belgium; and on 14 July in Nice, France.Footnote 13 Outside European soil the major attack was the two coordinated bomb attacks in the Karrada district in Baghdad, Iraq, on 3 July, with over 300 deaths.

Figure 1. Cumulative lagged measure of deaths from terrorist attacks by IS (16 March 2016–20 July 2016).
Empirical Strategy
Exploiting the temporal variation in terrorist attacks throughout our period of study, we use a pre-post approach to estimate their effects on the number of followers in the IS-related Twitter accounts. More specifically, for account α on day t, we estimate the models of the form:

where Y at denotes the log of followers of account a on day t. As noted previously, cd t is the continuous variable that equals the number of terrorist victims in day t, smoothed with a discount rate of 50, 75 or 100 per cent. The parameter of interest in our model equation is β 1.Footnote 14
Our main specification includes user-account fixed effects (α a). With regards to the plausibility of the identification condition, account fixed-effects are important because there are strong differences across accounts – language, geographic location of the user, demographic characteristics, as well as other observed and unobserved covariates. The major danger of an empirical strategy without account fixed effects is selection bias in the sample before and after an attack. This can arise because of shifts in either the type of accounts that Anonymous targets, which may be different in a period before and after an attack, or shifts in the behavior of users, including the opening or closing of accounts. The inclusion of account fixed effects allows us to remove all time-invariant heterogeneity across accounts (average number of followers throughout the period, language, geographic location) and focus on the average variation within accounts over time.
Another concern is that the effects we observe may not be driven by changes in the behavior of ISIS’s online audience, but instead they might be a product of the time-variant aggressive account suspension efforts by Twitter and the Anonymous’ hacktivist group in the aftermath of terror attacks. We construct two variables that account for changes in the behavior of Twitter and Anonymous. First, we create a variable that indicates the total number of reports Anonymous sends to Twitter every day. Second, we generate a measure of daily behavioral changes of Twitter that captures the total number of suspended accounts in our dataset. Though they are both post-treatment, they allow us to assess whether our findings are driven by changes in the behavior of Twitter and/or the Anonymous in the aftermath of terrorist attacks.
For the interpretation of the parameter β 1 as a causal estimate of the effect of terrorist attacks on the number of followers, we need to assume that timing of the terrorist attacks is exogenous to the pre-attack number of followers in Twitter accounts linked to IS. While a violation of the assumption is more likely when using long periods of time, we believe the assumption of the exogenous timing of terrorist attacks is highly plausible because our sample period is comprised of a short time span of only 127 days. Even though it is plausible that radical groups may organize terrorist attacks to anticipate a downward trend in support base, it is less likely that organizational leaders can anticipate sudden decreases in the support base in precise dates or in short periods of time. In other words, the likelihood of a terrorist attacks in 2014 may differ from that of a terrorist attack in 2016 for reasons that we do not fully understand, and leaders may react by increasing the rate of attacks in 2016 compared to 2014. However, the likelihood of a terrorist attack in a given day within our 127-day period – from 16 March to 20 July 2016 – is likely to be approximately constant within short periods from the perspective of Twitter followers and non-followers. In practical terms, this means that the exact day of the attack is generally not known in advance.
In short, for the causal parameter that we estimate to be biased, not only does it require the chance of an attack to vary over time, but to vary as a function of the number of followers in the periods immediately before an attack, or without an attack.Footnote 15 Thus, the parameter β 1 can be interpreted as a causal estimate of the effect of terrorist attacks on the number of followers of IS-related accounts because the uncertainty related to the precise timing and nature of the attack provides an identification mechanism for our empirical tests.
To empirically tease out the mechanisms, we generate a variable that takes into account the capacity of the state in which the account is located. We measure a country’s material capabilities to credibly prosecute perpetrators and to retaliate using the National Material Capabilities (version 5) (Singer, Bremer, and Stuckey Reference Singer, Bremer and Stuckey1972). Ideally, we would like to predict the exact country in which an account is located and then match it to a country’s capabilities to retaliate. While we lack information to precisely geo-locate the accounts with their profile information,Footnote 16 we employ a supervised machine learning algorithm to train an ordinal regression model.Footnote 17 Using the characteristics of the accounts for which we have complete information, we generate five clusters of accounts based on the resources to retaliate by the country in which an account is located.Footnote 18
Results
The results section is divided into three subsections. We first implement an interrupted time series analysis on the two most important attacks in Europe that fall within our period of study: The Brussels bombing on 22 March and the Nice attack on 14 July, both in 2016. This allows us to provide a first empirical evaluation of the relationship by employing a quasi-experimental research design with strong internal validity. Second, we move to the panel data analysis where we combine daily Twitter data with terrorist attacks worldwide during our entire period. This design enables us to generalize the prior findings to all other terrorist events with models that convincingly estimate a causal effect. Finally, we explore the mechanisms of the negative association found in the first two subsections by separating disengagement from deterrence.
An interrupted time series analysis of two major attacks
To assess the causality of the relationship, we employ an interrupted time series analysis, a type of regression discontinuity design (RDD) in which the running variable is defined by time (Percoco Reference Percoco2014). The terrorist data and the information on Twitter accounts are ideal for this approach because of the well-defined moment of the attack and the large number of accounts that we have collected in every single day throughout our period of analysis, which statistically empowers small bandwidths.
In brief, there is a potential outcome Y j for each account-observation j – the number of followers of an account; a treatment assignment variable W j , observation observed in the pre- or post-treatment period – which determines the potential outcome we eventually observe, so Y jW j, that is, either Y j1 or Y j0; a forcing variable t j, which is a running covariate – time to the attack in our case – and, finally, a cutoff value c, which establishes the interruption in the time series, which is set at 0 (the attack date in our case). Hence, the treatment assignment is given by:

The primary quantity of interest is the immediate change in the number of followers represented by τ in the following equation:

where followers j indicates the number of followers in an account-observation, α is an intercept of the model, Attack j is an indicator whether an observation falls on the day of the attack or later, ft j is a smooth function of the running variable time in days from the date of the attack, which can be positive or negative, and εj is an error term. To estimate f, we use a non-parametric estimation (a local linear regression).
A crucial step in this approach is the decision about the bandwidth, the window period of analysis. In general terms, there is a trade-off between precision and bias. On the one hand, a strategy that uses a large bandwidth would allow us to precisely estimate the causal effect because it would use a large number of observations ranging from many days before and after the attack. Because it allows data points that are far from the attack in the estimation of the outcome in both the control and treated periods, differences across units may arise because they were generated in different environments. On the other hand, we would like to minimize the bias of our estimate by subsetting our temporal focus to a narrow temporal window, and thereby removing those observations that are far from the day of the attack. Yet the general downside of this strategy is that we work with a reduced sample size, which reduces the precision of our estimates.
We chose a baseline bandwidth following the parameters suggested by Imbens and Kalyanaraman (Reference Imbens and Kalyanaraman2012) (IK) and test the sensitivity of the estimates by using different bandwidths, ranging from that suggested by IK to only one day before and after the attack. In the estimation procedures, we use triangular kernel, as recommended by Lee and Lemieuxa (Reference Lee and Lemieuxa2010), which gives more weight to those observations closer to the cutoff point.
Attack on Brussels, Belgium
On 22 March 2016, two blasts hit the main terminal of Zaventem international airport, in the north-east of central Brussels, and another explosion struck the Maelbeek metro station in the area where several European institutions are located. These three coordinated suicide bombings killed thirty-two civilians and three of the perpetrators, and more than 300 people were injured. Shortly after the explosions, IS claimed responsibility for the attacks in a statement released via Twitter, Telegram and other social media.
To evaluate the impact of the attacks on the number of followers, we took those accounts from our dataset that are observed more than once and fall within the window of fifteen days before and after the attack.Footnote 19 As our data collection procedures were initiated on 16 March, the pre-attack period is six days before the attack and the post-attack period is fifteen days after the attack.Footnote 20
Table 1, Panel A, presents the main estimates of the impact of the terrorist attack in Brussels on the number of followers in the accounts linked to IS. Table 1 shows the estimation equation by local linear regression using the number of followers as the dependent variable. Our treatment variable is Attack i, so the coefficient τ on Attack captures the effect on the number of followers of being in the pre-attack period versus the post-attack period, that is, it measures the effect of crossing the threshold – the attack date, from left to right – on the number of followers.
Table 1. Local Average Treatment Effects of Timing of the Attack on the Number of Twitter Followers
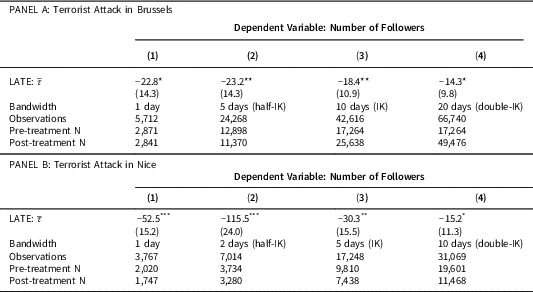
Note: *p<0.1; **p<0.05; ***p<0.01. One-tailed. IK refers to Imbens and Kalyanaraman’s (Reference Imbens and Kalyanaraman2012) bandwidth.
Under this specification, the attack in Brussels decreased the number of followers by twenty-one followers, which is equivalent to a 5.4 per cent decrease from the mean number of followers of the twelve-day period – seven days to the left and seven days to the right on the attack’s timeline. Importantly, the estimated negative effect is consistent significantly and quantitatively similar across different bandwidth specifications, whether doubling or halving the IK bandwidth. Moreover, even if we focus on the two-day period centered around the attack day (bandwidth = 1), the effect is also significantly negative. Figure 2 graphically shows the discontinuous jump around the attack date. This provides substantial evidence of the causal impact of the Brussels attack on the number of Twitter users following IS-related accounts.

Figure 2. Changes around the attack dates.
Attack on Nice, France
This subsection explores whether we see a similar effect for the largest terrorist attack on Western soil during our period of study. On the night of 14 July 2016, a man driving a 19-ton refrigerated truck and carrying an automatic pistol deliberately drove into crowds that were celebrating the Bastille Day on the Promenade des Anglais in Nice, France. The attack resulted in the deaths of eighty-six people and 434 injuries. On the morning of 15 July 2016, a few hours after the attack, the French president, François Hollande, addressed the nation in a televised speech, stating that the attack was terrorist in nature and linking it to the Islamic State by saying: ‘all of France is being menaced by Islamic fundamentalist terrorism’. Further evidence for this explicit link being made by the President was that he also used his televised speech to announce ‘the strengthening of […] the actions in Syria and Iraq’ (Mestre, Revault d’Allonnes, and Bissuel Reference Mestre, Revault d'Allonnes and Bissuel2016). On 16 July, IS, via its news agency, the Amaq News Agency, claimed responsibility for the attack by stating that a ‘soldier of the Islamic State’ executed ‘a new, special operation using a truck’, and warning that ‘no matter how much they enforce their security measures and procedures, it will not stop the mujahideen from striking’ (France 24 2016; Williams Reference Williams2016).
To evaluate the impact of this attack on the number of followers, we took only those accounts from our dataset that are observed more than once and fall within the window of fifteen days before and after the attack, as we did for the Brussels attack. In this case, however, our period of analysis stops a few days after the attack, on 20 July 2016, and, consequently, we have a fifteen-day pre-treatment period and a seven-day post-treatment period. Although the bandwidths that we will use are narrower than this time window, this will not affect our estimates.
Table 1, in Panel B, presents the main estimates of the impact of the terrorist attack in Nice on the number of followers in the accounts linked to IS.Footnote 21 As we did for the Brussels bombing, our favorite specification is reported in column (3) because it uses the bandwidth suggested by Imbens and Kalyanaraman (Reference Imbens and Kalyanaraman2012). Under this specification, the Nice attack decreases the number of followers by thirty, which is equivalent to a 10 per cent decrease from the mean number of followers throughout the eight-day period – four days to the left and four days to the right of the attack’s timeline. Additionally, the effect is consistently negative and statistically significant across bandwidths – the IK’s bandwidth, and its half –although the effect decreases as we incorporate data points that are further apart from the attack date until the estimate loses statistical significance at double the IK’s bandwidth. Interestingly, if we focus on the difference between the date before the attack and the attack date (bandwidth equals 1), the effect is also significantly negative. Figure 2 illustrates the discontinuous jump downwards that occurs between the date before and after the attack. Next, we explore whether these negative effects are consistent with a more general pattern of a causal and negative effect of attacks on the number of followers in accounts associated with IS using all the available data.
Main analysis
Table 2 reports the impact of the number of victims in IS attacks on the number of followers of IS in Twitter on the day of the attack and in its immediate aftermath. Panels in the table show the results across different discount rates. Models 1 and 3 report varying-intercept multilevel models where day-intercepts are modeled as a function of attacks perpetrated by IS. Additionally, models 2 and 4 include fixed effects at the level of the user-account. In addition, models 3 and 4 control for both Anonymous’ daily intensity of its reporting activities and Twitter’s daily intensity of its suspension activities.
Table 2. The Impact of the Number of Victims in Attacks on the Number of Followers in IS-Related Twitter accounts

Note: *p<0.1; **p<0.05; ***p<0.01. Constants omitted from the output. Columns 1 and 3: Mixed effects model with random effects at the user-account level. Columns 2 and 4: Fixed-effects models at the user-account level with standard errors clustered by user-account. The number of observations in the fixed effects models is lower because accounts with one observation are excluded from the dataset for identification purposes. In all models, we have excluded extreme observations in the dependent variable – the top 1 per cent, yet results are unaltered if we include them.
Column 1 shows that the effect of the number of victims in the US and Europe is negatively related to the log of the number of followers of Twitter-related accounts. However, this model may be biased due to distinct sample composition in the pre-attack and the post-attack periods. Column 2 also reports the significantly negative effect of attacks on followers after focusing on the within-account variation alone. This specification is closest to identifying the causal effect because it incorporates an intercept for each account and, consequently, controls for all between-account heterogeneity. Consistently, IS deadly attacks decreases the number of followers in IS-related Twitter accounts. Columns 3 and 4 report the same set of coefficients after controlling for daily changes in the intensity of the reporting activity by Anonymous and the suspending activity by Twitter. The intensity of Anonymous’ reporting activity seems to increase the number of followers and the intensity of Twitter’s banning activity seems to decrease the number of followers, yet these effects are not consistent across all model specifications and do not substantively alter our main finding.Footnote 22
If these models are capturing a true causal effect of bombing, we should expect that the effect of an extra victim in the United States and Europe should be stronger than the effect of a victim elsewhere. As expected, models 1 through 4 show that the effect of a victim outside Europe and the United States is negative but the magnitude of the effect is significantly smaller than a victim in Europe or the United States. In particular, the effect of 100 deaths in Europe and the US on the log of followers on that same day is between –0.07 and –0.05, yet the impact of a victim outside the US and Europe is close to –0.01; that is, between five and seven times smaller. In other words, a terrorist attack outside Europe and the US requires about five or seven deaths to achieve the equivalent effect to a single death within the US or Europe on the number of followers.Footnote 23
To strengthen our causal claims, we develop a placebo test to check the robustness of our models to several sources of endogenity. An adequate placebo test when dealing with interrupted time series is to derive the effects we would have been seen if alternative timings were examined. If the estimated causal effect is a result of an artifact rather than the actual attacks, we should expect the same relationship to emerge when picking random dates. In the online Appendix H, we show that the observed coefficient is significantly stronger than it would be under a random allocation of attacks. This alleviates concerns that our findings are the result of an empirical artifact.
Altogether, these results empirically support the thesis that the demobilization effect from the attacks dominates its mobilization effect since we are able to detect a significant negative effect on the number of followers in the aftermath of these events. While the results are statistically significant, we now turn to discuss whether the magnitude of the effect is substantive. Certainly, the large size of our sample allows us to detect effects that are very small in magnitude. Therefore, it is pertinent to discuss whether our parameter of interest captures a causal effect that is substantively meaningful. Before moving to the discussion of the magnitude of the effect, we should bear in mind that the estimated parameter does not capture the full impact of demobilization, but only the net effect of demobilization after subtracting it from a potential mobilization effect. If we assume that both effects may be strictly positive in absolute values, then the estimated demobilization effect should be interpreted as a lower bound of its true effect.
Figure 3 reports the predicted value of the number of followers in IS-related Twitter accounts as a function of the number of deaths associated with a particular day in the sample. The number of followers in Twitter accounts related to IS is predicted to be close to 137 if there has not been an attack on that day nor on the dates immediately following. However, an attack on the US or on European soil would imply a decrease in the number of followers on that day and in its aftermath, and this decrease depends on the intensity of and the proximity to the attack. Approximately, every twenty-five victims lead to an average decrease of two followers in their accounts. By contrast, elsewhere the magnitude of the decrease in the number of followers is much weaker because it requires an attack with 100 deaths to generate a change in the predicted value of one follower less in IS Twitter accounts.

Figure 3. Predicted values of the number of followers depending on the number of cumulative deaths and the location of IS terrorist attacks.
While interpreting these results, we should take into account that the variable ‘number of deaths’ does not truly account for the number of victims on a given day because the variable is measured in a way that allows for lingering effects of a given terrorist attack over time. Thus, to explore the actual impact of a discrete attack in the number of followers, we should assess not only the effect it has on a particular day but the cumulative effect it has throughout the period in the aftermath of the attack. To illustrate the shifts in the predicted values as a function of the number of deaths in an attack, Table 3 reports the five bloodiest attacks within our period of study, the date they occurred, their location, their death toll and the predicted change in the number of followers caused by an attack alone.
Table 3. Predicted Percentage Change in the Number of Followers After Some Important Terrorist Events within our Sample Period

Note: Simulated values are based on the fixed-effects models from column 3 in Table 3.
The values in the last column are the predicted cumulative percentage change in the number of followers as a result of the actual death toll related to attacks observed within our period of study. As a reminder, this means that the effect of the Brussels bombing had a value of 36 on 22 March 2016, 24 on 23 March, 11 on 24 March, 3 on 25 March, 1 on 26 March, and values indistinguishable from 0 thereafter. Hence, the simulation exercise involves summing these actual values in the variables produced by single attacks to obtain the change in the predicted value expected from each discrete attack. The results show that the largest impact was generated by the case of the attack in Nice with an average accumulative change of a 7.5 per cent decrease in the number of followers. Another important impact is caused by the 6.6 per cent decrease in the number of followers that would be expected from the Karrada bombings, a coordinated attack that killed 341 people on 3 July 2016 in Baghdad, Iraq. Even though not so important in magnitude, the attack in Istanbul and Brussels killing forty-four and thirty-six people would be expected to cause a 3.8 per cent and 3.2 per cent decrease.
This simulation exercise allows us to illustrate that the magnitude of the effects observed in our models are significant statistically, but also quantitatively. To put these percentages in perspective, a 7 per cent decrease from a starting point of 137 means an average of nine fewer followers. Taking into account that there are about 10,531 accounts related to IS throughout our period of study, this quantitatively means an expected drop of 94,779 in the absolute number of followings to IS.Footnote 24 This is a substantial reduction in the size of the IS Twitter audience. Overall, this finding is entirely consistent with the interrupted time series analysis provided above.
Exploring the mechanism: disengagement or deterrence?
Our core finding provides evidence of a demobilization effect of terrorist attacks on the followers who support the organization. This effect is particularly strong considering that we only observe the net effect: the demobilization effect is discounted by the opposite mobilization and attentional effects. Yet the demobilization effect is not entirely homogeneous but it may consist of two components: the disengagement effect, the action of un-following the organization due to a change in supporters’ beliefs in the aftermath of an attack; and the deterrence effect, the act of un-following the organization to avoid being tracked after an attack – so, by ‘going dark’.
Table 4 reports four model specifications. Model 1 shows that the effect of 100 European deaths on the log of followers is –0.40 for those accounts located in the weakest set of countries, so NMC equals 0, and the coefficient slightly strengthens to –0.48 for those located in the strongest set of countries. Yet, the difference in effect is not statistically significant across any of the models. Model 2 also reports a lack of significant moderation after including fixed effects at the level of the user-account. Similarly, models 3 and 4 also control for daily changes in the intensity of the reporting activity by Anonymous and the suspending activity by Twitter, yet results remain substantively unaltered. Overall, these results confirm the effect of terrorist attacks on decreasing the number of followers on IS-related Twitter accounts, but we find that this effect is consistent across all accounts regardless of the value of the material capabilities of the state in which an account is located.
Table 4. The Impact of the Number of Victims in Attacks Linked to IS on their Number of Followers in Twitter by Countries’ National Material Capabilities

Note: *p<0.1; **p<0.05; ***p<0.01. Constants omitted from the output. Columns 1 and 3: OLS regressions. Columns 2 and 4: OLS models with user-account fixed-effects and standard errors clustered by user-account. Models in columns 2 and 4 are also referred to as a hybrid model because they provide variation in a time-invariant variable (NMC of the account) and in a time-variant variable (number of victims), while maintaining any other characteristic of the account constant. The coefficient of the NMC score has to be excluded in the hybrid models because the constitutive term is time-invariant, so it cannot be separately estimated with the fixed effects. In all models, we have excluded extreme observations in the dependent variable – the top 1 percent, yet results are unaltered if we include them.
Overall, though we acknowledge that this is not an ideal test of the mechanisms, we can observe that the important prediction from the deterrence mechanism, in which accounts located in stronger countries should be more affected by the attacks, is not supported by the results. The effect is similarly strong across all accounts regardless of the strength of the state in which the account is geographically located. This suggests that the disengagement mechanism, rather than the deterrence mechanism, might likely be at work in our main analysis.
Conclusion
Twitter has become a crucial communication and recruitment tool for the Islamic State. This article offers an innovative approach to learning about the characteristics of IS by tracking the reports made by Anonymous on their Twitter accounts. The new data allow our study to explore an important question for political science in general and the terrorism literature in particular: what are the consequences of terrorist attacks?
While a full answer is beyond the scope of this research, we have provided new empirical evidence about the impact of terrorist attacks on the number of supporters of terrorist organizations. Our results shed light on the dynamics between those on the border between those inside and outside the organization. In particular, a strong empirical regularity has emerged, showing that terrorist attacks lead to a decrease in the size of IS Twitter audience in the aftermath of an attack that is both statistically and substantively significant.
These results speak directly to one of the hotly debated issues in terrorism studies; that is, whether terrorism helps groups that use it. Some political scientists argue that terrorism works (Kydd and Walter Reference Kydd and Walter2006; Pape Reference Pape2005), while others contend that it is counterproductive (Abrahms Reference Abrahms2012; Fortna Reference Fortna2015). Our results contribute to this debate by showing the detrimental effects of terrorism on the size of their support base. Hence, if an organization seeks to broaden its base of explicit supporters through violent activities, we show that this strategy is counterproductive because not only does the number of supporters not increase, but it significantly decreases.
If ideological drivers motivate terrorist organization (Abrahms Reference Abrahms2008; Acosta Reference Acosta2016), the reduction of the support base is a necessary cost to pay for the fulfillment of major organizational goals, including the pursuit of nonmaterial objectives or the outright elimination of adversaries. However, if we follow the strand of literature that assumes rationality among terrorists (Bueno de Mesquita and Dickson Reference Bueno de Mesquita and Dickson2007; Hoffman and McCormick Reference Hoffman and McCormick2004; Kydd and Walter Reference Kydd and Walter2006), then the unaddressed puzzle is: why would terrorist organizations engage in violent activities if this causes a decrease in the number of people that will be reached or potentially recruited? We can speculate that the reduction in their Twitter audience is the price terrorists have to pay to accrue two benefits from attacks. First, attacks may not aim at broadening the base of supporters (external mobilization), but to increase the loyalty of those who were already members (internal mobilization). In other words, they might trade quantity for quality by radicalizing existing supporters at the expense of losing moderate supporters. In our research design, this is something that is unobserved. We do not see whether supporters move from passive to active, or from active to leader, after terrorist attacks, because they are still followers of the observed accounts both before and after an attack.
Additionally, attacks may aim at infusing terror in the civil population. For instance, organizations may benefit in terms of exerting influence over foreign governments (Bueno de Mesquita Reference Bueno de Mesquita2005; Bueno de Mesquita and Dickson Reference Bueno de Mesquita and Dickson2007). Possibly, spreading terror among the population may increase the likelihood of governments providing policy concessions (Thomas Reference Thomas2014), or else provoke an excessive response of government to the advantage of the organization (Bueno de Mesquita Reference Bueno de Mesquita2005; Bueno de Mesquita and Dickson Reference Bueno de Mesquita and Dickson2007). From this perspective, the cost paid in a reduction in the number of people may be worth taking.
Finally, we acknowledge that further research is required to fill the theoretical and empirical gaps that remain in this literature to fully understand the incentives of pursuing violent activities. In addition, this study has focused on the terrorist organization that is perhaps the most influential in today’s politics, Islamic State. However, future research should explore whether our findings can generalize to other types of terrorist organizations.
Supplementary material
Data replication sets can be found in Harvard Dataverse at: https://doi.org/10.7910/DVN/H6RZA7 and online appendices at: https://doi.org/10.1017/S0007123418000340.