1 Introduction
Old and Middle English witness a switch in verb phrase headedness from a final to an initial configuration. The phrase structure trees in (1) sketch the resulting change from object (O) verb (V) to verb–object order.
(1)
Despite the fact that the development has received great attention in the literature (e.g. Canale Reference Canale1978 and much subsequent work), researchers have only recently begun to model the variation statistically. ‘There have been surprisingly few attempts at a comprehensive quantitative study’ (Fischer et al. 2000: 160). The purpose of this article is therefore to add to the ongoing endeavour to quantify the change by constructing a regression model predicting OV/VO order specifically for the Middle English (ME) period taking into account important determinants that have been identified in the literature.
The study of early English syntax is impeded by the paucity and low quality of available texts. The widely used ME periodisation introduced by the Helsinki corpus, M1 (1150–1250), M2 (1250–1350), M3 (1350–1420), M4 (1420–1500), shows a large gap in prose texts in M2. Furthermore, early prose texts like the Continuation of the Anglo-Saxon Chronicle (c. 1131, 1154), the Katherine Group (early thirteenth century) or the Ayenbite (c. 1340) display well-known idiosyncrasies, such as constructions specific to the genre of annals, AB language features, translation effects, etc. The ‘religio-juridico-belletristico-commercial nature … [of] high-style written language’ in ME may be far removed from ‘the vernacular engine driving changes in progress’ (Joseph & Janda Reference Joseph and Janda2003: 141). These problems are not always considered as carefully as they should be and a certain carelessness in the interpretation of the surviving text material may have contributed to the postulation of overly rapid or inaccurate time courses for the change in object verb order (e.g. ‘catastrophic’ reanalysis in the twelfth century in Lightfoot Reference Lightfoot1991; see criticism in Kiparsky Reference Kiparsky1996, fn. 10). A necessary precautionary measure against naïve generalisations is the inclusion of a comprehensive set of texts in the analysis. The Penn Parsed Corpus of Middle English (PPCME2; Kroch & Taylor Reference Kroch and Taylor2000a) is a tool that fulfils this requirement as well as can reasonably be expected and it has now become a standard resource in the field. However, researchers should also consider another beneficial methodology, namely the addition of poetry to their datasets. Most written transmission of the vernacular took place through the medium of verse during the earlier parts of the Late Middle Ages (c. 1050–1400). Poetic texts thus form an important source of evidence for the language use at the time. The Parsed Corpus of Middle English Poetry (PCMEP; Zimmermann Reference Zimmermann2015) is a supplementary parsed corpus that makes this best practice possible.Footnote 2 Accordingly, the present study uses data from both the PPCME2 and the PCMEP to study the change from OV to VO thus exemplifying how both genres can be made fruitful in the study of ME syntax.
The article is structured as follows. Section 2 introduces the PCMEP. Section 3 explains how the corpus data were retrieved and coded. I present a mixed-effects regression model for the rise in VO based on the data thus obtained in section 4 and discuss the central findings in the wider context of the background literature in section 5. Section 6 concludes.
2 The Parsed Corpus of Middle English Poetry
The Parsed Corpus of Middle English Poetry (PCMEP; Zimmermann Reference Zimmermann2015) is a fully annotated and syntactically parsed corpus of ME verse texts. At the time of writing (2021), it includes c. 216,000 words in 50 poems and continues to grow at a rate of about 20,000 word tokens per year. It is parsed according to the same guidelines as its much larger sister corpus, the Penn-Parsed Corpus of Middle English (PPCME2; Kroch & Taylor Reference Kroch and Taylor2000a). Researchers familiar with the PPCME2 thus do not have to learn a new annotation scheme but can use their search queries directly on both corpora.
As an illustration, consider the corpus annotation for sentence (2a) shown in (2b). Note in particular the OV structure relevant to this paper in the last line. It uses the label for direct object, NP-OB1, sandwiched between a modal, MD, and a non-finite main verb, VB.
(2) (a) Men, to say well of wymen / yt is best, //
Men, to say well of women it is best
and nat to displeen hem / ne deprauve //
and not to displease them nor deprave
yf that they wol hir honour / kepe and saue. //
if that they will their honour keep and save
‘Men, it is best to speak well of women // and neither to displease nor deprave them // as long as they keep and save their honour.’ (LetterCupid,80.189.85.[Stanza_27]Footnote 3)

The main purpose of the PCMEP is to help to close a substantial gap in the transmission of English prose texts between c. 1250 and 1350 with available poetry from the same time. Figure 1 shows that the poetry successfully bridges the prose gap.
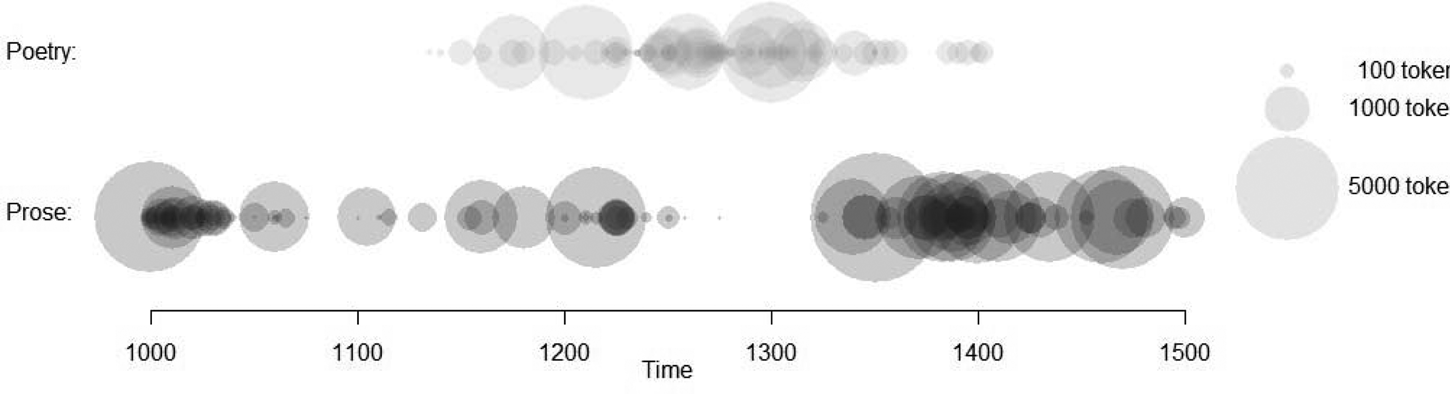
Figure 1. Temporal distribution and size of poetry and prose texts (from Zimmermann Reference Zimmermann2020: 11)
The PCMEP holds great potential for researchers working on ME syntax. The addition of its texts can (i) reduce the risk of erroneous generalisations based on a small number of texts with strong idiosyncrasies, (ii) give a fuller picture of a point of variation, for instance with respect to genre or dialect, and (iii) provide more realistic quantitative estimates for factors influencing a linguistic development. It is therefore advisable that ME poetry should be used to supplement the prose not just for the variation in OV/VO order discussed here, but for studies on ME syntax more generally.
3 Data collection and coding
Following the reasoning in Kroch & Taylor (Reference Kroch and Taylor2000b), studies on the variation between OV/VO should only consider cases in which a direct object co-occurs with a non-finite main verb following a finite auxiliary or modal.Footnote 4 I therefore collected all such clauses from the PPCME2 and PCMEP.Footnote 5
I used the coding function of CorpusSearch 2 (Randall Reference Randall2004) to annotate the data automatically for the following factors. (a) The dependent variable was either conservative OV or innovative VO.Footnote 6 Examples are presented in (3).
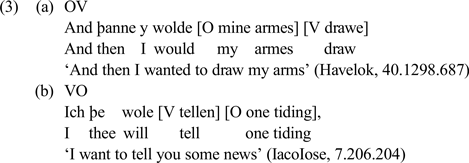
The following independent variables were included: (b) Every text file was coded for its genre, prose versus poetry. The genre distinction will be a major focus of the subsequent modelling. (c) Every text was assigned an approximate date of composition.Footnote 7 (d) Clause type was a binary variable with the levels main versus subordinate clause. The corpus annotation guidelines involve some ambiguities (e.g. for-clauses can be parsed as either), but serve as an objective and replicable standard. (e) The presence or absence of an overt subject was registered. (f) Object type distinguished between pronominal, definite, indefinite, negative and quantified objects. Pronoun objects consisted of a single personal pronoun. Definite objects contained a th-based determiner, possessor or proper name. Indefinite objects involved other determiners, bare nouns or the word other. Negative objects included a quantifier starting with n- (e.g. no), quantified objects other quantifiers or numerals. These conditions were checked in the order pronoun > negative > quantified > definite > indefinite. Hence, conflicts are resolved according to this cascade. For instance, (4) involves both a quantifier, al, and a determiner, this, but the object was classified as quantified because this type appears earlier in the coding sequence.

(g) The weight of the object was measured as the number of words it contains. (h) Lastly, I recorded the text source for every example.Footnote 8
In total, the dataset comprises 12,419 examples. Of those, 1,955 have conservative OV order and 10,464 innovative VO order. A total of 9,570 instances are found in prose and 2,849 in poetic texts.
4 Results
I fitted mixed-effects logistic regression models to the coded data. The model I would like to propose is given in table 1. I present models for pronominal and nominal objects separately. They predict the word order variation between VO and OV from the independent variables outlined in the last section and control for clustering within texts. Every predictor is given a short abbreviation, which will be referenced in the subsequent discussion.
Table 1. Logistic regression model predicting the occurrence of VO (versus OV) from year, genre and clause type for pronominal objects (top), and from year, genre, weight, clause type and object type for nominal objects (bottom)
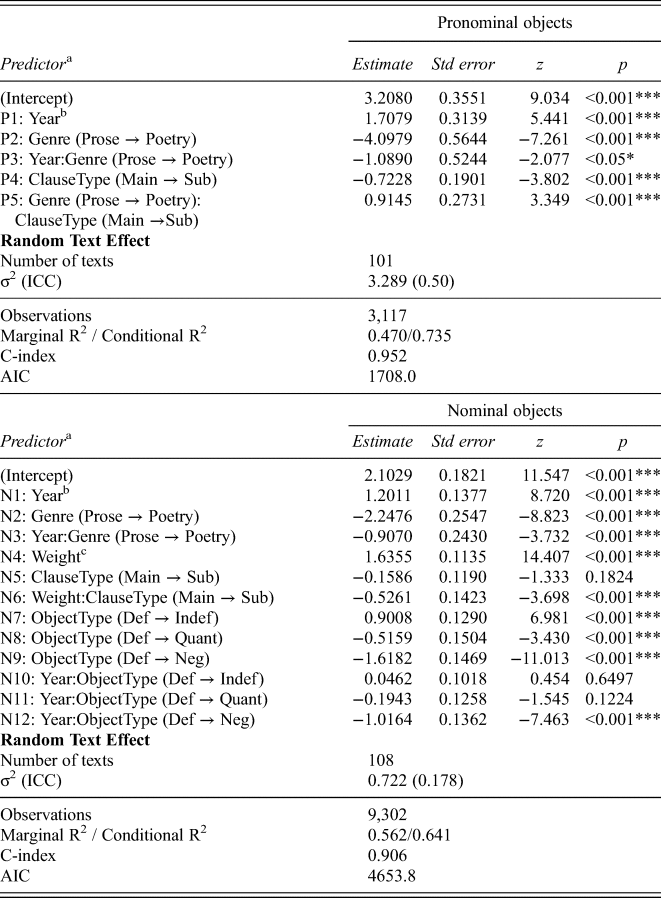
a effects on innovative variant VO
b ‘Year’ variable standardized to z-scores
c ‘Weight’ variable measured in log-words (natural logarithm)
‘Year’ is a highly significant predictor for the occurrence of VO order. As time passes, VO gradually becomes more probable (P1, N1). However, not only does the significant ‘Genre’ effect indicate greater conservativeness of the poetry overall, more pronounced for pronoun objects (P2) than for nominal ones (N2), but the significant ‘Year:Genre’ interaction also shows that the rate of change is slower in that genre. The poetry ‘slow-down’ is substantial for pronominal objects (P3), and so great that it almost seems to stall the development altogether for nominal objects (N3). Figure 2 illustrates these points.
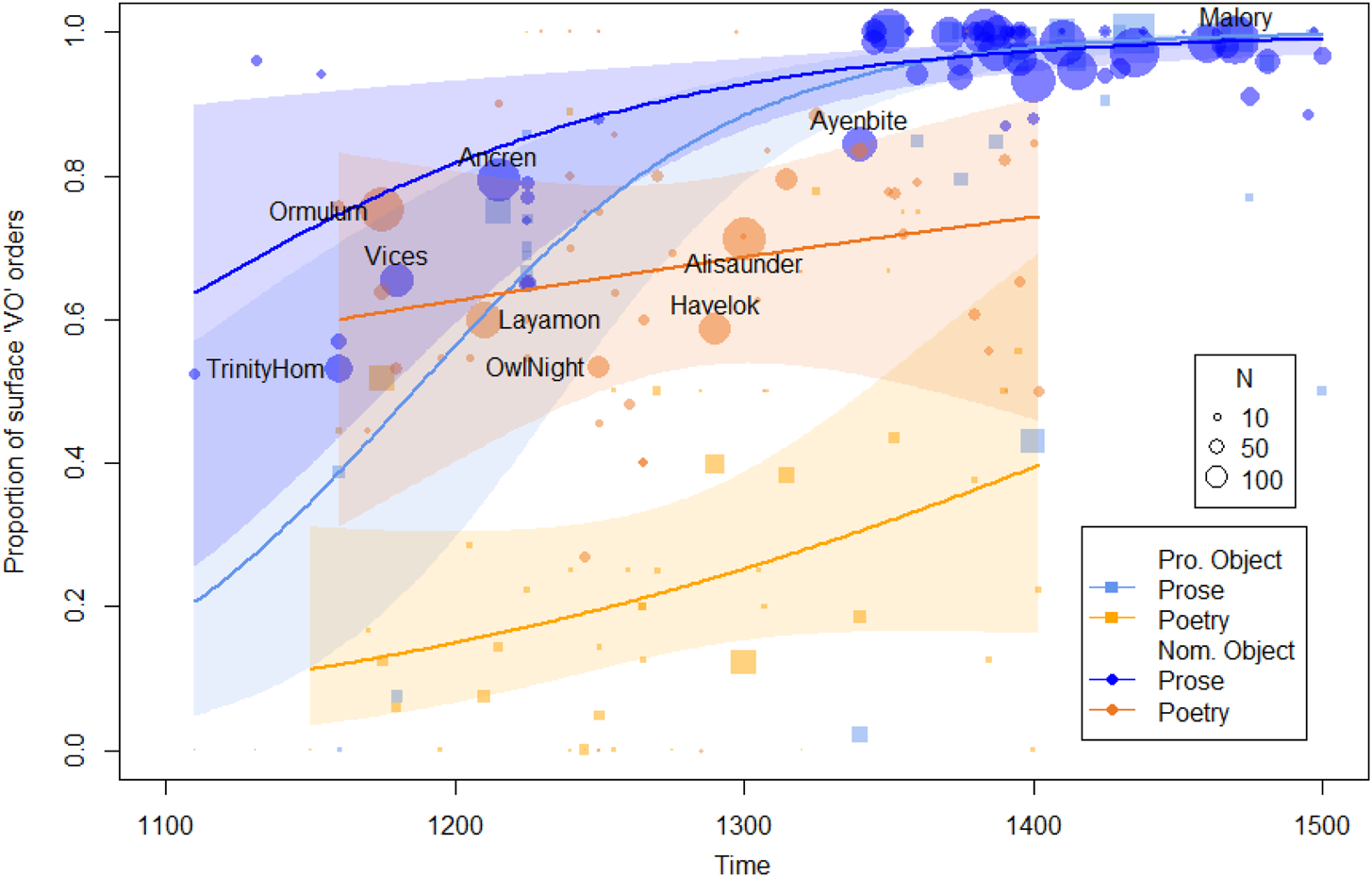
Figure 2. The increase of VO order across time in prose and poetry texts for pronominal (Pro.) and nominal (Nom.) objects. Every dot represents a text with the size proportional to its number of tokens. The shaded areas indicate 95%CIs.
As a nominal object becomes heavier, the probability of VO increases (N4). In fact, once an object is at least 9 words long, it almost categorically occurs after the verb. I found only two exceptions, the most extreme of which, an OV configuration with a heavy, 18-word object, is presented in (5).

I do not see sufficient justification to posit further interaction effects between ‘Weight’, ‘Year’ and ‘Genre’. The ‘Weight’ slopes are somewhat steeper in the poetry than in the prose, and the slope for prose levels off as VO becomes the norm. However, these effects may be spurious. The diachronic increase in the prose may be more pronounced simply because there is a lack of prose texts in the data between 1250 and 1350, precisely when the poetry texts are designed to supplement the gap. This might make the slopes of the prose texts appear more advanced simply because the prose texts are also on average younger. I will therefore model the effect of object heaviness as identical between the two genres and additive with time.
Main clauses have a higher chance of occurring with VO than subordinate clauses for pronoun objects (P4). Interestingly, this only holds for prose, not for poetry (P5).
The effect of clause type on word order is more complicated for nominal objects. Here, main clauses show a relatively linear relation between weight and word order. Subordinate clauses, on the other hand, have a lower probability of VO, but this is due in large part to greater conservativeness of 2-word objects. More research is needed to evaluate this surprising finding. The effect flattens the weight slope in subordinate clauses, leading to a significant interaction effect between ‘Clause Type’ and ‘Weight’ (N5, N6). These points are illustrated in figure 3.
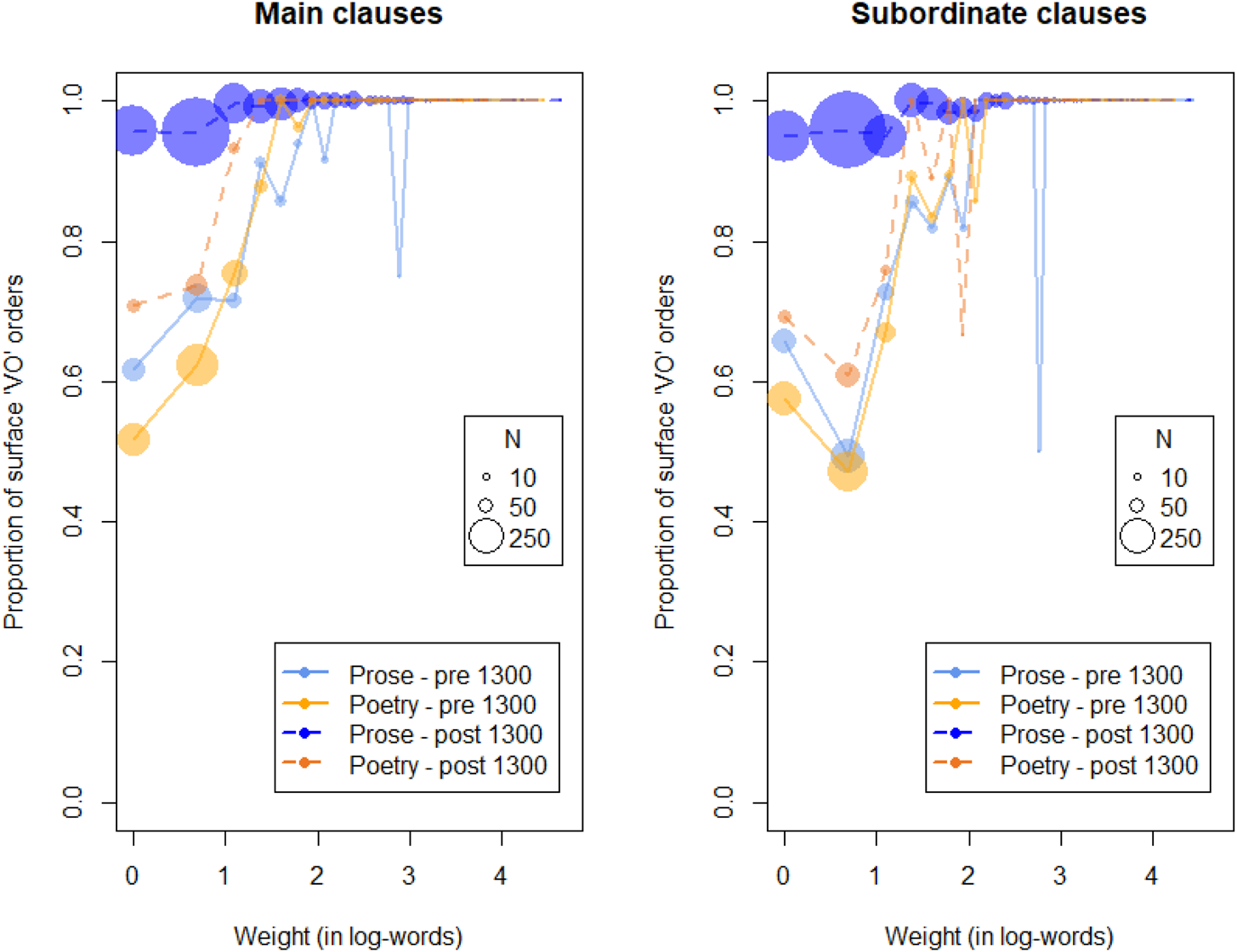
Figure 3. The proportion of VO order by object weight (in log-words) for nominal objects. The interactions with prose/poetry (blue versus orange dots) and time, shown for before/after 1300 (solid versus dashed line), are seen as accidental and are not included in the model. The interaction with main/subordinate clauses (left versus right panel), caused by a curious drop in VO for 2-word objects in subordinate clauses, is included in the model.
Finally, indefinite objects have a significantly higher, quantified and negatively quantified a lower, probability of occurring with VO order than definite objects (N7, N8, N9). Furthermore, negative objects innovate VO order at a slower rate than the other object types (N10, N11, N12). Figure 4 illustrates. I assume, for the same reasons as above, that additional interactions, especially with ‘Genre’, are accidental and are therefore not included in the model.
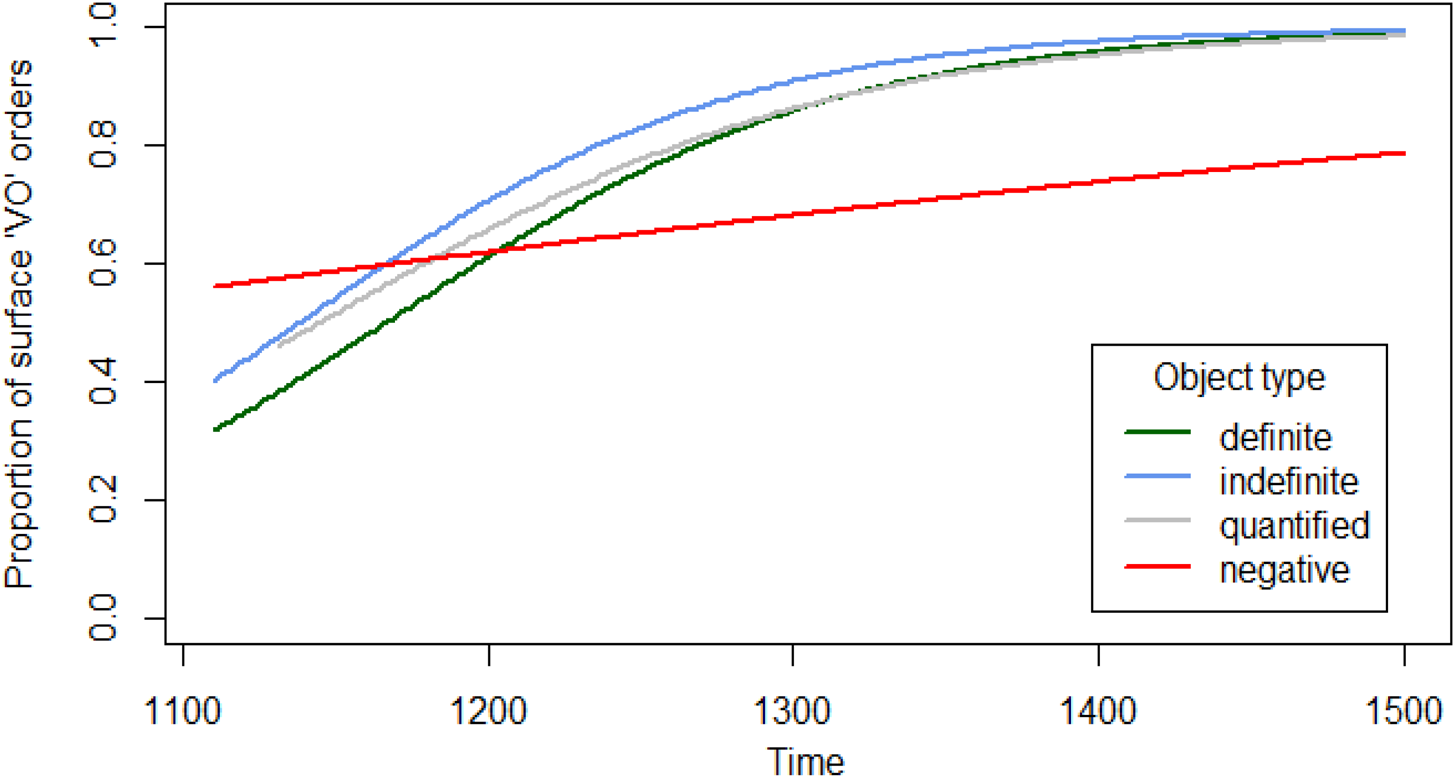
Figure 4. The rise of VO in Middle English by object type. The graph presents model estimates only for ease of exposition.
The variance of the random text intercepts is substantial especially for pronominal objects. This shows that it is essential to control for the clustering of examples within individual texts to avoid misleading coefficient estimates.
5 Discussion
I will now put the quantitative findings above into the context of existing studies. First, the rise of VO in Middle English can be measured in prose and poetry. Both sources of data are therefore useful as evidence. However, the change proceeds in a markedly different manner in prose and poetry. The latter genre is considerably more conservative (replicating Foster & van der Wurff Reference Foster and van der Wurff1995, Reference Foster and van der Wurff1997) and also innovates the new order more slowly (a new finding in this explicit form).
One could attempt to establish a typology of syntactic features that are more or less prone to sustained variation in poetry. Shorter, morphosyntactic features may be harder to manipulate consciously whereas longer, macrostructural patterns are easily exploited for poetic effect. This assumption could begin to explain why there is no marked genre-specific differential in the rise of when as a subordinator (Zimmermann Reference Zimmermann2020) or the development of Jespersen's Cycle (Truswell et al. Reference Truswell, Alcorn, Donaldson and Wallenberg2019), whereas the loss of null subjects (Walkden & Rusten Reference Walkden and Rusten2017) or the change in the relative order of verb and object clearly proceed differently between prose and poetry.
A related perspective on the diachronic genre differences is offered by the notion of ‘poetic function’ (Jakobson Reference Jakobson1960), poetry's focus on message formulation and linguistic creativity, utilising archaic features as readily as colloquial ones for aesthetic effect (e.g. Semino Reference Semino2002). Most importantly, conservative OV patterns may persist to accommodate rhymes on the verb, although other artistic uses are conceivable (e.g. Fisher Reference Fischer1992: 373). These poetic functions may obfuscate the true rate of VO order associated with a given time, thus adding noise not encountered in the prose. Indeed, the variance of deviance residualsFootnote 9 of the model for texts before 1400 indicates larger between-text variability and poorer predictivity for poetry (1.29) than for prose (0.43).
One can also think of the findings in terms of ‘archaisation’, a concept by which I mean a gradual divergence in the frequency of use of a linguistic feature between idiomatic usage, where the decline is faster, relative to a more marked genre, in which the loss is slower, leading to the feature's association with that genre and its eventual niche survival. Here, prose is closer to spoken language and loses VO more quickly, while poetry is less natural and abandons VO more slowly, so that OV patterns are re-evaluated as distinctly ‘poetic’.Footnote 10 The concept can be applied to a wide range of phenomena, e.g. the restriction of be come or thou to hyper-formal or biblical registers.
It bears repeating that VO order reaches a probability of more than 99 per cent of use in prose in the fifteenth century. VO should thus be regarded as the norm from that time on (pace some authors who sketch the time course differently, e.g. ‘the VO base was dominant by 1200’ (Kiparsky Reference Kiparsky1996: 144Footnote 11), ‘the change took place … through roughly the late 17th c.’ (Wallenberg et al. Reference Wallenberg, Bailes, Cuskley and Ingason2021: 3); see the excellent summary in Fischer et al. 2000: ch. 5). In fact, the data presented here allow a refinement of a plausible end point of the change in prose, datable to the early to mid fifteenth century.
The weight variable is implicated in a likely reason for the emergence of VO. The earliest medieval English is sometimes regarded as underlying OV plus a right-ward extraposition process (e.g. Lightfoot Reference Lightfoot1979; Kemenade Reference Kemenade1987). This early extraposition is mainly driven by weight. Indeed, the ancient poem Beowulf shows post-verbal objects predominantly with heavy objects / across metrical breaks (Pintzuk & Kroch Reference Pintzuk and Kroch1989; Taylor Reference Taylor2005). Subsequently, lighter objects begin to appear post-verbally and eventually a new underlying VO base is said to come about as a result. It would in principle be possible to derive a testable prediction from this account: if weight and time are merely additive, VO orders would emerge in addition to and independently of weight constraints. If on the other hand there is a significant interaction effect between weight and time such that VO rises faster with light objects, the new word order could be interpreted as feeding on extraposed objects, which would support a causal association. As stated above, the data are unfortunately too sparse and too noisy to ascertain with reasonable certainty if such an interaction effect should be postulated.
Ever since Lightfoot's (Reference Lightfoot1991) proposal for a learning bias leading children to acquire linguistic structures predominantly from unembedded clauses (degree-0 learnability), main clauses have been seen to be overall more innovative than subordinate clauses. A corresponding clause type effect has been identified in the literature for the rise of VO (e.g. Pintzuk & Taylor Reference Pintzuk and Taylor2006: 254–5). On the other hand, Stockwell & Minkova (Reference Stockwell and Minkova1991) articulate the claim that subordinate clauses lead the way in the transition from OV to VO. While my model does include effects that support the general innovativeness of main clauses, it also suggests that the relationship between word order and clause type is much more complicated. Pronoun objects in poetic texts do not provide evidence for the advanced status of main clauses. Nominal objects show a greater dependence on weight in subordinate than main clauses because, for unknown reasons, 2-word objects are more conservative than others in that environment. In general, it remains somewhat vague how the discovery of any distributional difference between clause types in historical corpora would impact on Lightfoot's proposal. There is thus room for a more detailed theory of clause type interactions of the type presented here. Perhaps it is possible to explain the complex influence of clause type on word order variation at least partially in terms of information density (Wallenberg et al. Reference Wallenberg, Bailes, Cuskley and Ingason2021).
The object type effects largely replicate previous findings. The more advanced status of indefinite as compared to definite objects most likely reduces to information-structural constraints already present in Old English. Indefinite objects tend to be new, and newness is a strong predictor for VO order (e.g. Taylor & Pintzuk Reference Taylor and Pintzuk2012). Inversely, definite objects are often old and givenness predicts OV order (e.g. Struik & Kemenade Reference Struik and van Kemenade2020). The distinction appears to be less rigorous in my Middle English data than in previous studies (e.g. the repeated formulation of OV as ‘reserved’ for given objects in Struik & Kemenade Reference Struik and van Kemenade2020) perhaps because poetic requirements like rhyme can easily override general processing constraints. This is illustrated in (6), where an indefinite object, ‘a present’, new to the addressee, nevertheless appears with an OV configuration, quite possibly to enable a rhyme on the main verb.

The finding that quantified, and particularly negatively quantified, objects have a significantly higher probability of occurring with OV than other objects in Middle English ties in seamlessly with identical constraints in the previous Old English (Pintzuk & Taylor Reference Pintzuk and Taylor2004, Reference Pintzuk and Taylor2006) and subsequent Early Modern English periods (Moerenhout & van der Wurff Reference Moerenhout and van der Wurff2000; Ingham Reference Ingham2002). The constraint eventually leads to a narrowing of OV predominantly to these contexts in late Middle and Early Modern English. One interesting question that does not seem to have received great attention so far is to what extent this state of affairs was sustained by influence from French, as illustrated in (7).
(7) (a) French
car nul enuieulx ne peut [O riens] [V souffrir]
(b) Middle English translation
for an envious man may [O no thing] [V suffer]
(King Ponthus and the Fair Sidone, Mather Reference Mather1897: 64)
The issue might be resolved by separating negative indefinite pronouns (nothing) from negatively quantified nominals (no + noun).
It has been claimed that subjectless clauses are another context permitting OV alignments in late prose (van der Wurff 1997). However, ‘Subject’ (overt versus null) did not emerge as a significant variable in any of my models. Perhaps the special context can be explained as a late exaptation of remnant OV order for a new use (e.g. Traugott Reference Traugott2004). More generally, the finding raises the important methodological question of how to distinguish between unquantifiable and non-existent effects.
I will spend the remainder of my discussion on what I believe to be a fundamental and recurring problem arising when historical linguists attempt to map estimates of effects from quantitative models onto phrase-structural grammar models. I will illustrate the problem with reference to the results from the present study.
One can make a rough distinction between two types of studies of historical syntax, which I will call ‘statistical’ and ‘generative’. Statistical treatments attempt to predict the rate of a variant of a linguistic variable from quantifiable and additive factors. Generative treatments, on the other hand, attempt to build a grammar algorithm that can parse a string as a hierarchical phrase structure tree or reject it as ungrammatical. This article is an example of a statistical study, modelling variation in the form of a regression analysis, and not a generative study, as I have not contributed to the development of an explicit, phrase-structural model of Middle English syntax.
Generative analyses are important because they model syntactic structures in a computationally rigid way (e.g. through merge in Minimalism, context-free rewrite rules etc.). For example, the innovation in VO order can be modelled as the gradual emergence of speakers’ knowledge to put together an initial verb with a subsequent object to form a VP, as shown in (1b). In particular, generative models can reveal structural ambiguities. This is a crucial contribution to historical syntax, where it is often the case that linguistic examples may represent an innovative structure of interest, but may also instantiate an alternative parse. In the context of reanalysis, such cases are often referred to as bridge contexts. For example, the existence of ME patterns such as V-X-O (where X can be any constituent) shows that a realistic grammar algorithm for that language stage must be able to handle post-posing structures (extraposition, rightward raising, heavy NP shift etc.). Consequently, the string VO should also be parsable with such an option resulting in ambiguity between two hierarchies, say, underlying VO, […[VO]], and post-posed VO, [[…V]O].Footnote 12 The methodological question I wish to draw attention to is this: how can one reconcile the prediction of the frequency of word order patterns from statistical models with the insight that some tokens may instantiate extraneous rather than the relevant phrase structure parses from generative models? In the present case, a logistic regression modelFootnote 13 predicts VO order from a number of independent variables, but it is wholly uninformative about the frequency of distinct phrase structural parses of these VO patterns, such as an integral VP versus post-position.
While I do not currently have a good solution for this problem, I can see a number of possible paths forward. A first attempt may be to break down the statistical model for specific constructions that are assumed to diagnose the phrase structure of interest unambiguously. For instance, pronominal objects cannot readily post-pose and are hence less problematic with respect to the ambiguity discussed. Generative models can make phrase structure rules sensitive to a categorical difference between pronominal and full nominal phrases. In this way, the grammatical difference can map directly onto separate statistical models. Indeed, I have presented separate models for pronominal and nominal objects above. This method, however, has a number of shortcomings. It is inadequate for distinctions that are probabilistic in nature. For example, the significant effect of object type cannot readily be addressed in this way. In fact, some linguists may not assume a categorical difference even for pronominal and full noun phrases, but rather regard the difference as falling at the extreme end of a spectrum regulating the probability of post-position, since demonstrably post-posed pronoun objects, although extremely rare, are not entirely absent in Middle English. Furthermore, there will often be so many structural ambiguities that no one sensible subdivision is obvious. Not infrequently may subdivisions require the isolation of some data that disambiguates a parse of interest and at the same time the inclusion of the same data in the combined dataset as it is ambiguous with respect to some other kind of syntactic ambiguity. For example, pronominal objects do not normally post-pose, but they frequently pre-pose, creating ambiguous parses for OV orders. At best, the separate model for pronominal objects therefore estimates a lower bound for the true rate of underlying VO order with pronouns.
Another avenue could be inspired by the idea to correct the total counts of an ambiguous syntactic structure by estimating the rates of their distinct parsing alternatives. For example, one could compare V-X-O structures, where O must be post-posed, to V-O-X structures, where O is unlikely to have post-posed, to arrive at an estimate of the rate of post-position. This estimate can be used to calculate the proportion of post-posed objects in ambiguous VO patterns. For an excellent example of this methodology in connection with information structure, see Taylor & Pintzuk (Reference Taylor and Pintzuk2011). However, this approach has problems as well. One cannot be sure that the rate of a particular parse estimated independently from one diagnostic will carry over to another environment. I agree that it is fruitful to work under the assumption ‘that postposing applies at a constant rate’ (Pintzuk & Taylor Reference Pintzuk and Taylor2006: 266) in all environments, but this is not a necessity. In the example above, the rate of post-position in contexts with an additional element X may be different from the rate of post-position in contexts without such an element. I also worry that the method may stretch the relatively small early English corpora beyond their limits so that fluctuations by random factors, like individual texts, may influence the results. I therefore believe that it may be more advantageous to attempt a principled mapping of regression coefficients to a probability for the application of a syntactic process.
Why do I contend that this is an important problem? First, generative analyses can make unfalsifiable claims about the prevalence and time of birth or death of underlying orders because they can freely interpret ambiguous tokens that could but do not have to be parsed according to the underlying order of interest. Exaggerating to illustrate the point, I could claim that VO order arises extremely late, say in 1400, and extremely fast, say within 10 years, by analysing all prior VO strings as results of post-position processes. Intuitively, the best way to inoculate against such absurdities is to tie historical generative models to quantitative corpus data. Yet statistical models do not actually resolve the issue in principle at the moment. Second, an enhanced synthesis of statistical and generative models can help to avoid ideological fragmentation in the field. Usage-based linguists may interpret the output from statistical models as directly representative of speakers’ knowledge thus paying little attention to the reality of structural ambiguity. Generativists may struggle to include probabilistic effects in their discrete phrase structure rules. If successful, a synthesis could build strong bridges between historical syntacticians of different persuasions and between their ideas.
6 Conclusion
This article investigated the ME change from OV to VO quantitatively with a particular focus on the distinction between prose and poetry. The main findings are as follows:
(i) The time course of the change is complex. Referring to positive objects in prose texts and abstracting away from syntactic pre- and post-posing processes, one can say that the transitional period comes to its end in the early to mid fifteenth century. Previous assessments that locate the end point of the change considerably earlier should be reassessed in light of the large textual base and conditioning factors considered here.
(ii) The prose–poetry contrast emerges as a central determinant for the change. Poetry is more conservative overall and innovates the new order more slowly. The variable is more important than object type (information structure) or clause type. Studies on the variation between OV/VO in early English should therefore not ignore this crucial variable. Furthermore, claims about the existence of OV word order after c. 1400 should explicitly refer to genre categories in order to avoid misleading statements.
(iii) ME poetic texts have great value as a bridge during M2, 1250–1350, when prose texts are exceedingly rare. At the same time, their inclusion must accommodate genre-specific patterns. Here, poetic texts lend strong support to a gradual rather than abrupt decline in OV throughout M2 but also behave so fundamentally differently from the prose that one cannot treat them as a mere supplement. Poetry is at once an illuminating and an obfuscating source of data.
I have mentioned limitations, possible extensions and open questions throughout the article. I will repeat only the most pressing issue: there is a great need to develop a methodology that can map precisely between frequency predictions for string-based levels of a dependent linguistic variable from regression models and their hierarchical, phrase-structural parses from explicit grammatical theories, which, crucially, may involve structural ambiguity.
The change from OV to VO in early English is a decades-old and well-studied research topic. Historical linguists have learned about its long-term development as well as its fine-grained expression in individual texts or examples, its potential causes, dialectal distribution and a host of significant predictors. With a lot of the low-hanging fruit picked, it is to be expected that progress will now be made more slowly, by incremental processes of revisiting and reconceptualising some of the relevant issues. I hope the present article will function as one piece in the ongoing effort to put this jigsaw puzzle together.