In 2016, the WHO estimated that 39 % of the global adult population were overweight and predicted an increase to 50 % by 2030(1,Reference Dobbs, Sawers and Thompson2) . Excessive fat accumulation is a major public health concern that increases one’s risk of cardiometabolic multi-morbidity and mortality by up to two and twenty-three times, respectively(Reference Guo, Moellering and Garvey3–5). Concurrently, the yearly cost of treating obesity and its consequential diseases was estimated to reach US$1·2 trillion by 2025(6). While pharmacotherapy (e.g., orlistat) and surgical interventions (e.g., bariatric surgery) have been effective and prompt in inducing weight loss, individuals often experience subsequent weight regain due to poor lifestyle habits(Reference Kushner7). Therefore, cheaper and safer diet and exercise programmes remain the preferred method for weight loss where up to 55 % of weight loss programme participants could lose ≥5 % of their initial body weight within a year(Reference Christian, Tsai and Bessesen8). However, studies have shown that weight loss often culminates after 6 months and individuals often regain up to 100 % of their initial weight within 5 years(Reference MacLean, Wing and Davidson9,Reference Daley, Jolly and Madigan10) . Failure to sustain weight loss has been attributed to the poor adherence to behaviour change plans(Reference MacLean, Wing and Davidson9), lack of motivation(Reference West, Gorin and Subak11), knowledge(Reference Masood, Alsheddi and Alfayadh12), coping skills and self-efficacy(Reference Latner, McLeod and O’Brien13), and central to weight loss failure is the lack of self-regulation(Reference Montesi, El Ghoch and Brodosi14,Reference Hartmann-Boyce, Johns and Jebb15) .
Self-regulation refers to the self-monitoring and self-control of automatic thoughts, emotions and behaviours to achieve a long-term goal (e.g., weight loss)(Reference Baumeister16). Common self-regulation strategies for behaviour change include identifying discrepancies between current behaviours and future goals(Reference Epton, Currie and Armitage17), self-monitoring of behaviour and behavioural outcomes(Reference Burke, Wang and Sevick18), action planning(Reference Benyamini, Geron and Steinberg19), goal setting(Reference Pearson20), habit change(Reference Cleo, Beller and Glasziou21) and behavioural substitution(Reference Booth, Prevost and Wright22). However, as compared to old habits which are largely automatic and effortless, such strategies are intentional, effortful and cognitively demanding(Reference Bargh and Morsella23). This often leads to the temporal erosion of behaviour change adherence, causing a well-known yo-yo weight effect (weight increases back to baseline)(Reference Moroshko, Brennan and O’Brien24). Therefore, individuals trying to lose weight often attempt to either increase self-regulation capacity through sheer willpower(Reference Johnson, Pratt and Wardle25) or reduce the self-regulation effort needed through weight-loss mobile apps(Reference Everett, Kane and Yoo26), clinical weight management programmes(Reference LeCheminant, Gibson and Sullivan27) and commercial weight-loss programmes(Reference Gudzune, Doshi and Mehta28). However, such methods are often expensive, resource-intensive and unsustainable(Reference Forman, Kerrigan and Butryn29). An emerging strategy to tackle this problem of poor self-regulation is to apply artificial intelligence (AI)(Reference Russell and Norvig30).
AI refers to the mimicry of human intelligence through machine learning to attain and apply knowledge and skills for processes such as pattern recognition and decision-making. The popularity of AI stems from its potential to solve real-world problems with rationality, efficiency, cost-effectiveness and accuracy. In obesity research, AI has been used to examine aetiologies(Reference Bouharati, Bounechada and Djoudi31), perform risk profiling(Reference Chatterjee, Gerdes and Martinez32), standardise diagnosis (decision support system)(Reference Cruz, Martins and Dias33), personalise weight management programmes(Reference Rachakonda, Mohanty and Kougianos34), perform remote monitoring(Reference Chatterjee, Gerdes and Martinez32) and predict prognoses(Reference Stead35). However, to the authors’ best knowledge, there are limited academic publications that explored the use of AI to improve behaviour change self-regulation for weight loss(Reference Duan, Edwards and Dwivedi36).
Therefore, we conducted a scoping review to present an overview of the possible applications of AI to regulate eating and dietary behaviours, exercise behaviours and weight loss. Unlike a systematic review that aims to answer a specific research question, a scoping review aims to map out the ‘breath, nature and extent of research’ done on a topic without dwelling into the literature or assessing its methodological quality(Reference Brien, Lorenzetti and Lewis37). This aims to provide a comprehensive collection of articles on a specific topic, elucidate research gaps in their underexplored aspects and inform the worth of conducting a systematic review. In 2017–2018, approximately 45 % of middle-aged adults (40–59 years old), 43 % of older adults and 40 % of younger adults were obese(Reference Hales, Carroll and Fryar38). This indicates that weight management should begin at a younger age before the onset of obesity and chronic diseases, which commonly occurs during middle-age due to a slower metabolism, increased food consumption and an increasingly sedentary lifestyle(Reference Prasad, Sung and Aggarwal39–Reference Tchernof and Després41). Therefore, the literature search was narrowed down to adults from 18–64 years old to enhance the focus and clarity of this inquiry.
Methods
This scoping review was structured according to the five-step framework by Arksey and O’Malley, and results were presented according to the Preferred Reporting Items for Systematic Reviews and Meta-Analyses extension for scoping reviews (PRISMA-ScR) guidelines (online supplementary material, Supplemental Table S1)(Reference Arksey and O’Malley42,Reference Tricco, Lillie and Zarin43) .
Step 1: Identifying the research question
We used the Population, Intervention, Comparison and Outcomes (PICO) acronym to develop our research question, ‘what is known about the potential of AI for weight loss and weight-related behaviour change’.
Step 2: Identifying relevant studies
Studies were first searched across eight electronic databases (CINAHL, Cochrane–Central, Embase, IEEE Xplore, PsycINFO, PubMed, Scopus and Web of Science) for papers published from inception till 22 July 2020. Initial search terms such as ‘artificial intelligence’ and ‘weight loss’ were iteratively derived from the PICO framework and medical subject heading through multiple rounds of database searching by the HSJC in consultation with LY. The final search terms used were ‘artificial intelligence’; ‘machine learning’; ‘computational intelligence’; ‘computer heuristics’; ‘expert system’; ‘fuzzy logic’; ‘knowledge bases’; ‘natural language processing’; ‘neural networks’; ‘weight loss’; ‘weight management’ and ‘weight control’ (see online supplementary material, Supplemental Table S2 for search terms used in different databases). Upon mapping the existing studies into three broad categories, we found that weight-related changes were centralised around diet and exercise. Therefore, we conducted another search for literature published up till 15 December 2020 using additional keywords such as ‘diet’, ‘eating’, ‘physical activity’, ‘sedentary’ and ‘exercise’.
Step 3: Study selection
After the database searching, duplicate articles were removed and the remaining titles and abstracts were screened for eligibility. Full texts of the articles were independently screened for eligibility by HSJC and WHDA where discrepancies were resolved through discussions. Studies were included if they described the use of AI for weight loss or weight loss-related behaviour change in adults aged 18–64 years. Studies were excluded if they: (1) did not describe the use of AI (e.g., purely data scraping); (2) were grey literature including conference, opinion, protocol or technical/theoretical papers; (3) were on people undergoing surgery (e.g., bariatric surgery) or with underlying diseases (excluding pre-diabetes) that affect weight status; (4) were unrelated to self-regulation and (5) were not written in the English language. Additional studies were then identified using forward and backward reference searching of the included articles. The search process and results are shown in Figs 1 and 2.
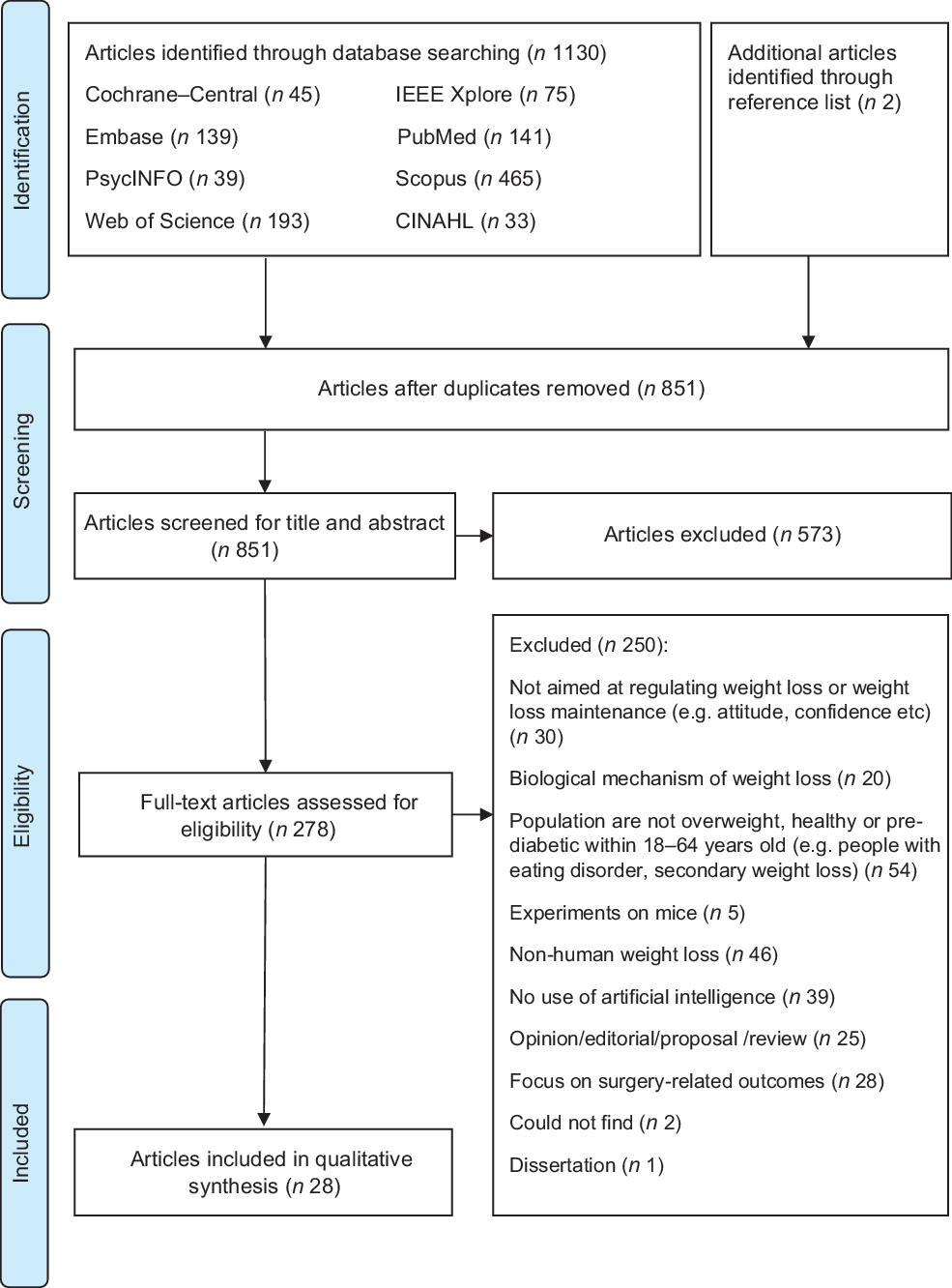
Fig. 1 PRISMA 2009 flow diagram for first search

Fig. 2 PRISMA 2009 flow diagram for second search
Step 4: Charting the data
Data extraction was performed according to a form developed by HSJC, which was pilot tested on five articles and refined accordingly before use. Information extracted was categorised under the headers – author, year, country, type of publication, study design, aim, population, sample size, age, sex, BMI, self-regulation tenets (e.g., self-monitoring), AI functions (e.g., recognise eating behaviours), AI features (e.g., gesture recognition and predictive analytics), weight loss-related behaviours (e.g., dieting), machine learning techniques, data collection methods and important results. The resultant information was then charted as shown in Fig. 3.

Fig. 3 Data mapping of AI features used for different self-regulation components (n 66)
Step 5: Collating, summarising and reporting the results
Study characteristics
As shown in Fig. 1, 1132 potential articles were retrieved from the first database search, 851 titles and abstracts were screened, 278 full-text articles were assessed and twenty-eight articles were included. As shown in Fig. 2, 4441 articles were retrieved from the second database search, 3959 titles and abstracts were screened, ninety-six full-text articles were assessed and sixty-five articles were included. The kappa statistic (k) indicated good interrater reliability (k = 0·96) where discrepancies were resolved upon discussion. During the screening of full-text articles, two articles were unable to be retrieved even after seeking help from the university librarian and hence were excluded(Reference Lo44,Reference Goldstein, Zhang and Forman45) . Two separate journal articles included in this review were published from the same dissertation(Reference Goldstein46–Reference Goldstein, Thomas and Foster48). Among the sixty-five included articles, one reported two studies and hence a total of sixty-six studies were presented in this scoping review. Representing more than 2031 participants, 56·1 % of the studies were from the USA, 87·9 % were experimental studies, 81·8 % had a sample size of < 100 participants, 89·4 % included participants from both sexes and 56·1 % reported the baseline BMI of the participants (Table 1). Study characteristics are detailed in online supplementary material, Supplemental Table S3.
Table 1 Study characteristics (n 66)*

* One included article consisted of two studies; hence, the total number of studies is 66.
† Includes ten studies that provided age ranges.
Self-regulation of weight loss-related behaviours
Three tenets of self-regulation were identified, namely self-monitoring (n 66, 100 %), optimisation of goal setting (n 10, 15·2 %)(Reference Everett, Kane and Yoo26,Reference Forman, Goldstein and Crochiere49–Reference Dijkhuis, Blaauw and van Ittersum55) and self-control (n 10, 15·2 %)(Reference Everett, Kane and Yoo26,Reference Forman, Goldstein and Crochiere49–Reference Liu, Li and Liu51) . Details on the use of AI for the self-regulation of weight loss-related behaviours are shown in Table 2. Of the studies on enhancing self-monitoring, twenty-nine (43·9 %) were on eating behaviours(Reference Amft and Troster58–Reference Jiang, Qiu and Liu76), seven (10·6 %) were on energy intake(Reference Rachakonda, Mohanty and Kougianos34, Reference Korpusik and Glass77–Reference Aswani, Kaminsky and Mintz82), thirty-three (50 %) were on physical activity(Reference Everett, Kane and Yoo26,Reference Liu, Li and Liu51–Reference Dijkhuis, Blaauw and van Ittersum55,Reference Chung, Oh and Baek60,Reference Zhang, Stogin and Alshurafa74,Reference Arif, Kattan and Ahamed81–Reference Zhou, Fukuoka and Goldberg105) and nine (13·6 %) were on energy expenditure(Reference Aziz, Zihajehzadeh and Park83,Reference Bouarfa, Atallah and Kwasnicki85,Reference Kang, Shin and Jung92,Reference Lin, Wang and Hwang94–Reference Mo, Liu and Gao97,Reference Sazonov, Hegde and Browning100,Reference Tao, Burghardt and Mirmehdi101) . Of the studies on optimising goal setting, five were on optimising eating behaviour goals (e.g., eating at a certain time of the day and energy intake)(Reference Goldstein, Thomas and Foster48,Reference Forman, Goldstein and Crochiere49,Reference Stein and Brooks53) and six were on optimising physical activity goals (e.g., type of physical activity and energy expenditure)(Reference Everett, Kane and Yoo26,Reference Liu, Li and Liu51–Reference Dijkhuis, Blaauw and van Ittersum55) . Of the ten studies on self-control, five were on controlling eating behaviours(Reference Goldstein, Thomas and Foster48,Reference Forman, Goldstein and Crochiere49,Reference Juarascio, Crochiere and Tapera107) , three were on controlling physical activity performance(Reference Everett, Kane and Yoo26,Reference Rabbi, Pfammatter and Zhang52,Reference Zhou, Fukuoka and Mintz54) and two were on both(Reference Liu, Li and Liu51,Reference Stein and Brooks53) . Only six of these studies reported weight loss of which two were significant(Reference Everett, Kane and Yoo26,Reference Juarascio, Crochiere and Tapera107) . With only 15·2 % of the included studies examining strategies to exert self-control over weight-related behaviours, more research is needed to explore the potential of AI on improving weight-related behavioural changes for weight loss.
Table 2 Functions of AI in self-regulation of weight management in healthy and overweight populations (n 66)

Functions of artificial intelligence in self-regulation of weight loss-related behaviours
We categorised the included articles into three AI applications, namely machine perception (n 50), predictive analytics only (n 6)(Reference Goldstein, Zhang and Thomas47,Reference Dijkhuis, Blaauw and van Ittersum55,Reference Aswani, Kaminsky and Mintz82,Reference Zhou, Fukuoka and Goldberg105,Reference Juarascio, Crochiere and Tapera107,Reference Lemon, Rosal and Zapka111) and real-time analytics with personalised micro-interventions (n 10)(Reference Everett, Kane and Yoo26,Reference Forman, Goldstein and Crochiere49–Reference Zhou, Fukuoka and Mintz54,Reference Juarascio, Crochiere and Tapera107) (Fig. 3). Briefly, machine perception refers to the use of machine learning to detect, extract features, classify and interpret (recognise) information that is received through wearable/non-wearable devices – akin to our vision (camera), proprioception (gestures) and audition (sound)(Reference Boek, Bianco-Simeral and Chan112). Predictive analysis refers to the use of historic data and statistical methods (e.g., data mining and modelling) to predict future events. Studies on predictive analytics focused on building predictive models based on behaviour data (eating and exercise), nutrition, goal achievement rates, anthropometric data, perspectives (e.g., blog posts) and ecological factors to predict weight loss and behaviour lapses. Real-time analytics refers to the instantaneous analysis of past and present data to train, test and optimise predictive models and provide corresponding prompts of behavioural lapse risks and recommendations as micro-interventions. Only one of the studies explored the use of all three AI applications in enhancing weight loss(Reference Liu, Li and Liu51). A summary of the AI features, instruments/sensors used, sensing domains and their corresponding functions relevant to weight management is shown in Table 3.
Table 3 Summary of AI features (that uses machine learning), instruments/sensors, sensing domains and functions about weight management
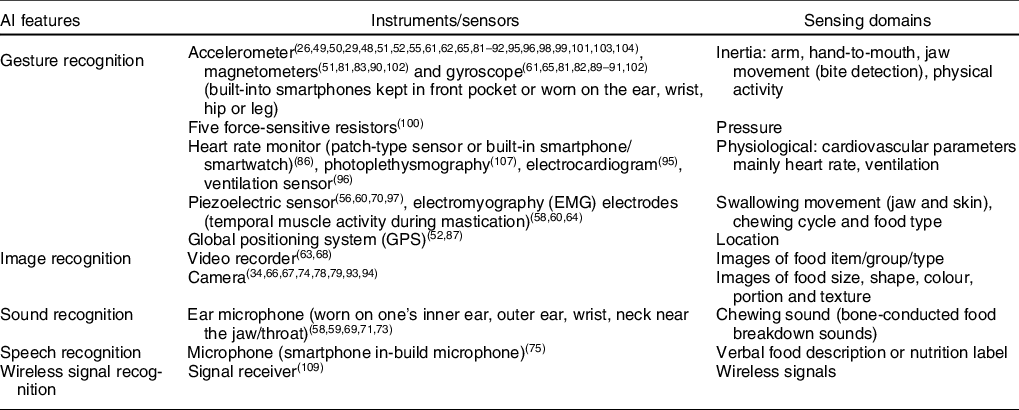
Machine perception: self-monitoring
Studies on machine perception were focused on examining the use of machine learning techniques to recognise (1) food items/groups (e.g., fruits or meat)/types (e.g., liquid or solid), (2) eating behaviours/habits (e.g., eating behaviour lapses), (3) physical activities types (e.g., aerobic and strength-training exercises)/intensity (e.g., sedentary to vigorous exercise)/habits and (4) estimate energy balance (energetic intake and output) (Table 2). The studies reported recognition accuracies ranging from 69·2 to 99·1 %. Machine recognition techniques used in the included studies were gesture (n 32)(Reference Liu, Li and Liu51,Reference Alshurafa, Kalantarian and Pourhomayoun56,Reference Amft and Troster58,Reference Chung, Oh and Baek60–Reference Fontana, Farooq and Sazonov62,Reference Huang, Wang and Zhang64,Reference Kyritsis, Diou and Delopoulos65,Reference Sazonov and Fontana70,Reference Zhang, Stogin and Alshurafa74,Reference Arif, Kattan and Ahamed81,Reference Aziz, Zihajehzadeh and Park83–Reference Kim, Barry and Kang93,Reference Lin, Yang and Wang95–Reference Wang, Redmond and Ambikairajah104) , image (n 14)(Reference Rachakonda, Mohanty and Kougianos34,Reference Hossain, Ghosh and Sazonov63,Reference Liu, Cao and Luo66–Reference Lopez-Meyer, Schuckers and Makeyev68,Reference Zhang, Stogin and Alshurafa74,Reference Jiang, Qiu and Liu76,Reference Pouladzadeh, Shirmohammadi and Al-Maghrabi78–Reference Yunus, Arif and Afzal80,Reference Fullerton, Heller and Munoz-Organero88,Reference Kim, Barry and Kang93,Reference Lin, Wang and Hwang94,Reference Tao, Burghardt and Mirmehdi101) , sound (n 7)(Reference Amft, Kusserow and Troster57–Reference Bi, Lv and Song59,Reference Päßler and Fischer69,Reference Sazonov, Makeyev and Schuckers71–Reference Walker and Bhatia73) , speech (n 2)(Reference Hezarjaribi, Mazrouee and Ghasemzadeh75,Reference Korpusik and Glass77) and wireless signal (n 1)(Reference Krukowski, Harvey-Berino and Bursac113) recognition. Four studies used both gesture and image recognition(Reference Zhang, Stogin and Alshurafa74,Reference Fullerton, Heller and Munoz-Organero88,Reference Kim, Barry and Kang93,Reference Tao, Burghardt and Mirmehdi101) while one used gesture and sound recognition(Reference Amft and Troster58). Wearable sensors were used in all the included studies on machine perception except those that used image and wireless signal recognition (which use cameras and Wi-Fi receivers). Energy intake was mostly estimated using image and speech recognition(Reference Rachakonda, Mohanty and Kougianos34,Reference Hezarjaribi, Mazrouee and Ghasemzadeh75–Reference Yunus, Arif and Afzal80) while the other AI recognition techniques were used to detect eating behaviours and food types. Gesture/image recognition was mainly used to detect and estimate physical activity and energy expenditure(Reference Aziz, Zihajehzadeh and Park83,Reference Bouarfa, Atallah and Kwasnicki85,Reference Kang, Shin and Jung92,Reference Lin, Wang and Hwang94–Reference Mo, Liu and Gao97,Reference Sazonov, Hegde and Browning100,Reference Tao, Burghardt and Mirmehdi101) while the other AI techniques were used only for physical activity recognition.
Predictive analytics: goal setting and action planning optimisation
Six studies showed the use of AI to predict weight loss (n 1)(Reference Aswani, Kaminsky and Mintz82), adherence to personalised physical activity goals (n 2)(Reference Dijkhuis, Blaauw and van Ittersum55,Reference Zhou, Fukuoka and Goldberg105) , dietary lapses (n 2)(Reference Goldstein, Zhang and Thomas47,Reference Juarascio, Crochiere and Tapera107) and episodes of emotional eating (n 1) (Table 2). Only one study collected primary data using the ecological momentary assessment (EMA), which was also the only one that reported a mean dietary lapse frequency of 3·5 per week. EMA refers to the ‘repeated sampling of subjects’ current behaviours and experiences in real-time, in subjects’ natural environments’. None of the studies examined the applicability of these predictive models to stimulate weight loss. The sample sizes of the included studies on predictive analytics ranged from 12 to 210, of which only 83·3 % of the studies reported their participants’ BMI. Mean BMI of these studies ranged from 22·1 to 33·6 kg/m2, which were higher than those studies on machine perception and hence possibly more applicable to overweight adults. 83·3 % of the articles reported mean ages that ranged from 22·1 to 55·2 years old, one study included only female participants and the proportion of females in the remaining studies ranged from 77 to 91·7 %. Two studies explicitly reported the recruitment of only adults who were overweight, which elucidates the unique weight loss trajectory in one who is overweight although it does not indicate strategies that are effective in weight loss(Reference Goldstein, Zhang and Thomas47,Reference Aswani, Kaminsky and Mintz82) .
Real-time analytics and personalised micro-interventions: self-control
Ten studies evaluated the use of AI-assisted weight management interventions that instantaneously optimise prediction models for behavioural risk profiling (e.g., low, medium and high risk) and enhance behavioural self-control through adaptive and personalised messages/feedback/prompts (Table 4). The interventions were all delivered through smartphone apps, namely OnTrack (used in three of the included studies)(Reference Goldstein, Thomas and Foster48–Reference Forman, Goldstein and Zhang50), Sweetech app(Reference Everett, Kane and Yoo26), Calfit app(Reference Zhou, Fukuoka and Mintz54), Lark’s AI health coach app(Reference Stein and Brooks53), Think Slim app(Reference Spanakis, Weiss and Boh106), SmartCare app(Reference Liu, Li and Liu51), MyBehaviour(Reference Rabbi, Pfammatter and Zhang52) and one without a name. In general, the mobile app interventions used either wrist-worn activity trackers, smartphone in-built accelerometers or EMA to track one’s physical activity. Manual food logging and EMA were commonly used to track one’s dietary habits (e.g., type, amount and triggers of food intake). Resultant data were then used to train the app’s machine learning technology to recommend optimised goals and action plans for better self-control, adherence and success in weight loss and weight loss maintenance. More details on each intervention are shown in Table 4. Intervention duration ranged from 3 to 16 weeks of which 50 % of the studies reported the inclusion of run-in periods of 1–2 weeks to collect baseline user data and assess user technological uptake and adherence(Reference Goldstein, Thomas and Foster48–Reference Forman, Goldstein and Zhang50,Reference Zhou, Fukuoka and Mintz54,Reference Spanakis, Weiss and Boh106) . Of the ten studies on real-time analytics, one used Chatbots(Reference Stein and Brooks53) and five used EMA(Reference Goldstein, Zhang and Thomas47–Reference Forman, Goldstein and Zhang50,Reference Spanakis, Weiss and Boh106) . EMA frequency ranged from six to ten times a day and the number of EMA questions ranged from 15 to 21 questions. Common questions were on timing (e.g., morning; afternoon; night), location (e.g., home; work), emotions (e.g., sadness; boredom; stress), activity (e.g., watching television; socialising) physical state/internal cue (e.g., hunger; cravings; fatigue) and situational triggers (e.g., visual food temptation/availability). The remaining three studies collected data on step count using accelerometers and food intake using manual logging through smartphone apps.
Table 4 Details of studies that used real-time analytics with personalised micro-interventions (n 10)

NR, not reported; IMU, inertial measurement unit.
* Included within intervention;
Three studies(Reference Everett, Kane and Yoo26,Reference Stein and Brooks53,Reference Zhou, Fukuoka and Mintz54) focused on only improving physical activity, four studies focused on only improving dietary behaviours(Reference Goldstein, Thomas and Foster48–Reference Forman, Goldstein and Zhang50,Reference Spanakis, Weiss and Boh106) and three studies(Reference Forman, Kerrigan and Butryn29,Reference Liu, Li and Liu51,Reference Rabbi, Pfammatter and Zhang52) focused on both. All five studies(Reference Forman, Kerrigan and Butryn29,Reference Goldstein, Thomas and Foster48,Reference Forman, Goldstein and Crochiere49,Reference Forman, Goldstein and Zhang50,Reference Stein and Brooks53) on dietary lapse prevention reported percentage increases in dietary adherence, but only one study reported statistically significant results (P < 0·05), suggesting mixed findings(Reference Forman, Goldstein and Zhang50). Two of the three studies on preventing exercise lapses reported significant (P < 0·05) increases in step count and metabolic equivalent task(Reference Everett, Kane and Yoo26,Reference Stein and Brooks53) . This could be attributed to the personalisation of goals that were coherent with each users’ lifestyle habits based on the information retrieved from their calendar apps (indicates availability for exercise) and health app (indicates activity patterns)(Reference Everett, Kane and Yoo26). Weight loss outcomes ranged from an average of 2·4 –4·7 %(Reference Forman, Kerrigan and Butryn29,Reference Goldstein, Thomas and Foster48–Reference Forman, Goldstein and Zhang50,Reference Spanakis, Weiss and Boh106) of which only two were statistically significant (P < 0·05)(Reference Everett, Kane and Yoo26,Reference Forman, Goldstein and Zhang50) . Three studies reported the use of Bluetooth enabled weighing machines that synchronise weight data to the users’ phone apps, while the rest used manually-input weight.
Sixty percentage of the studies were randomised controlled trials, while the rest adopted observational and quasi-experimental designs. One study only recruited adults who were overweight, while the rest also included healthy adults(Reference Spanakis, Weiss and Boh106). The study sample sizes ranged from 8 to 181 participants, with mean ages ranging from 28·3 to 56·6 years old, 47–86·0% of females and a mean BMI of 27·3–37·0 kg/m2. Three studies reported model accuracies ranging from 69·2 to 83·8% in predicting dietary lapses, which is lower than those in the studies on machine perception(Reference Goldstein, Thomas and Foster48–Reference Forman, Goldstein and Zhang50). This could be due to the inclusion of volatile complex human behavioural factors such as dietary lapse triggers into the prediction models that could have affected the model accuracies. Retention/completion rate ranged from 44 to 86% in eight of the nine studies, indicating varying levels of adherence(Reference Everett, Kane and Yoo26,Reference Goldstein, Thomas and Foster48,Reference Forman, Goldstein and Crochiere49,Reference Forman, Goldstein and Zhang50,Reference Stein and Brooks53,Reference Zhou, Fukuoka and Mintz54,Reference Spanakis, Weiss and Boh106) . Five studies assessed user acceptability/satisfaction using short surveys and validated instruments, namely Technology Acceptance Model Scales and Validated System Usability Scale(Reference Everett, Kane and Yoo26,Reference Forman, Kerrigan and Butryn29,Reference Forman, Goldstein and Crochiere49,Reference Forman, Goldstein and Zhang50,Reference Stein and Brooks53) . However, the cut-off score to indicate acceptable acceptability/satisfaction was unclear.
Machine learning techniques
Classifiers used included decision trees (n 5)(Reference Liu, Li and Liu51,Reference Huang, Wang and Zhang64,Reference Dobbins, Rawassizadeh and Momeni86,Reference Pärkkä, Cluitmans and Ermes99,Reference Spanakis, Weiss and Boh106) , random forests (n 8)(Reference Goldstein, Zhang and Thomas47,Reference Forman, Goldstein and Crochiere49,Reference Forman, Goldstein and Zhang50,Reference Alshurafa, Kalantarian and Pourhomayoun56,Reference Thomaz, Zhang and Essa72,Reference Zhang, Stogin and Alshurafa74,Reference Hua, Chaudhari and Johnson90,Reference Lin, Wang and Hwang94) , rotational forests (n 1)(Reference Arif, Kattan and Ahamed81), Bayesian (n 8)(Reference Goldstein, Zhang and Thomas47,Reference Forman, Goldstein and Crochiere49,Reference Forman, Goldstein and Zhang50,Reference Walker and Bhatia73,Reference Aswani, Kaminsky and Mintz82,Reference Bastian, Maire and Dugas84,Reference Dobbins, Rawassizadeh and Momeni86,Reference Dong, Scisco and Wilson110) , k-nearest neighbour (n 5)(Reference Dobbins, Rawassizadeh and Momeni86,Reference Bouarfa, Atallah and Kwasnicki85,Reference Fullerton, Heller and Munoz-Organero88,Reference Jain and Kanhangad91,Reference Vathsangam, Schroeder and Sukhatme103) , clustering (n 1)(Reference Spanakis, Weiss and Boh106) and support vector machines (n 14)(Reference Chung, Oh and Baek60,Reference Lopez-Meyer, Schuckers and Makeyev68,Reference Sazonov and Fontana70,Reference Sazonov, Makeyev and Schuckers71,Reference Walker and Bhatia73,Reference Pouladzadeh, Shirmohammadi and Al-Maghrabi78,Reference Pouladzadeh, Shirmohammadi and Bakirov79,Reference Dobbins, Rawassizadeh and Momeni86,Reference Jain and Kanhangad91,Reference Liu, Gao and John96,Reference Mo, Liu and Gao97,Reference Sazonov, Hegde and Browning100,Reference Zhou, Fukuoka and Goldberg105,Reference Juarascio, Crochiere and Tapera107) . Deep learning classifying techniques used were convolutional neural network (n 7)(Reference Kyritsis, Diou and Delopoulos65,Reference Liu, Cao and Luo66,Reference Jiang, Qiu and Liu76,Reference Korpusik and Glass77,Reference Yunus, Arif and Afzal80) of which two were region-based convolutional neural network(Reference Rachakonda, Mohanty and Kougianos34,Reference Kyritsis, Diou and Delopoulos65) , artificial neural network (n 4)(Reference Fontana, Farooq and Sazonov62,Reference Ermes, PÄrkkÄ and MÄntyjÄrvi87,Reference Kang, Shin and Jung92,Reference Montoye, Pivarnik and Mudd98) , generalised regression neural network (n 1)(Reference Lin, Yang and Wang95), probabilistic neural network(Reference Liu, Li and Liu51), hidden Markov model (n 4)(Reference Bi, Lv and Song59,Reference Päßler and Fischer69,Reference Tao, Burghardt and Mirmehdi101,Reference Zhang, Smeddinck and Malaka109) and natural language processing (n 2)(Reference Hezarjaribi, Mazrouee and Ghasemzadeh75,Reference Korpusik and Glass77) . One study used reinforcement learning(Reference Forman, Kerrigan and Butryn29), five used liner/logistic regression(Reference Aziz, Zihajehzadeh and Park83,Reference Hegde, Bries and Swibas89,Reference Lin, Wang and Hwang94,Reference Sazonov, Hegde and Browning100,Reference Vathsangam, Emken and Schroeder102) and other classifiers with more unique machine learning algorithms include multi-armed bandit(Reference Rabbi, Pfammatter and Zhang52), radial basis function network(Reference Lin, Yang and Wang95), behavioural analytics algorithm(Reference Zhou, Fukuoka and Mintz54) and Sojourn(Reference Kim, Barry and Kang93).
Discussion
Through this systematic scoping review, we found and included sixty-six studies that showed the potential uses of AI in regulating eating and dietary behaviours, exercise behaviours and weight loss. We conceptualise the AI use cases as (1) machine perception to enhance self-monitoring efficiency; (2) predictive analysis to optimise weight loss goal setting and action planning and (3) real-time analytics and personalised micro-interventions to prevent behavioural lapses. In general, the third themes seemed to be the most homogeneous where all studies described the use of a mobile phone app to monitor eating/dietary/exercise behaviours, optimise goal setting based on real-time data and delivery nudges/prompts to recommend a healthier behaviour. Predictive analytics was conducted on a wide variety of variables such as step count, energy intake, dietary lapse triggers, emotions and heart rate variability. It is noteworthy that we only found six studies that focused only on predictive modelling which could explain the heterogeneity. Machine perception was the most diverse with various recognition techniques that could be used to estimate energy intake and output. However, the accuracy of recognition technology and tracking device (e.g., in recognising food items and tracking heart rates), ease of data collection (e.g., syncing from various devices to a common data storage server for computing), degree of automaticity (i.e., risk of privacy infringement), user uptake (i.e., how adherent are the users to question prompts or machine-generated recommendations), machine learning modules (e.g., steps to prepare and analyse data and selecting the most suitable model for different datasets) and the comprehensiveness of such techniques (e.g., the number of food types that can be recognised) remains challenging. This hinders the practical implementation of AI into weight management programmes in a free-living condition, which could explain why most of the included studies are at the machine perception stage and only ten are real-life use cases for weight management. Readers should note that heterogeneity tests such as Q and I2 were not conducted and the aforementioned observation was derived iteratively through perusal.
Participants in the studies on real-time analytics and micro-interventions were generally older (seven of eight studies reported mean age of 40–57 years old) and had a higher BMI (27–37 kg/m2) than the other included studies. While variables such as gender/sex/are well-known to influence the outcomes of weight management programmes due to differences in body image(Reference Lemon, Rosal and Zapka111), food intake choices(Reference Boek, Bianco-Simeral and Chan112), self-monitoring and self-control(Reference Krukowski, Harvey-Berino and Bursac113), we did not find studies that examined such differences. Future studies could include a subgroup analysis based on gender to identify gender-specific target variables that could enhance weight management outcomes. While all studies ascertained the benefits of AI in facilitating behavioural self-regulation, only two out of ten interventional studies showed statistically significant weight loss post-intervention. This could be due to the difference in intervention effects on a general compared with an overweight population(Reference Olander, Fletcher and Williams114,Reference Poppe, Van der Mispel and Crombez115) . Another reason could be due to the short interventional programme that lasted from 3 to 16 weeks, where clinically significant weight loss (> 5 % of initial body weight) is normally observed between 6–9 months post-intervention(Reference Nackers, Ross and Perri116). On the other hand, mixed findings could also be attributed to an underpowered sample size of 43 and 55 in the studies that showed significant weight loss results as compared with the rest that ranged from 52 to 181(Reference Forman, Kerrigan and Butryn29,Reference Goldstein, Thomas and Foster48,Reference Forman, Goldstein and Crochiere49,Reference Forman, Goldstein and Zhang50) . It is also possible that micro-interventions in the form of prompting affect different behaviours differently. For example, increasing physical activity may require prompts/reminders/cues to motivate an action while such prompts could have a counter-productive effect on reducing unhealthy eating as it cues the action of unhealthy eating(Reference Evans, Norman and Webb117). Therefore, although we recognise the potential of AI in enhancing the completeness and convenience of behaviour change self-monitoring and self-control, its additional efficiency cannot be established as yet. Moreover, the majority of the studies were on machine perception while only ten were on real-time analytics with micro-interventions. This suggests that we are still in the infancy stage of applying AI on self-regulating weight loss-related behaviours as studies are still focused on building accurate and valid behaviour self-monitoring systems before testing its effectiveness in predicting and promoting weight loss.
Machine perception
One obvious advantage of using wearable sensors for machine perception is its potential to enhance the completeness and accuracy of data collection as it reduces respondents’ self-reporting burden, a contributing factor of underreporting shown in up to 30 and 50 % of adults of normal and overweight(Reference Amft and Troster58,Reference Dong, Scisco and Wilson110) . This is commonly achieved through the automatic collection of objective behavioural data, eliminating the common barriers of adherence such as poor motivation, time constraints and negative moods(Reference Burgess, Hassmén and Pumpa118). However, none of the studies on machine perception evaluated its effects on weight loss nor behaviour change and most of the studies did not assess the accuracy of food energy estimations. This could be due to the focus on building an accurate and reliable machine perception system before assessing its validity on specific weight-related estimations. Nevertheless, studies have shown that off-loading the need for manual logging (e.g., keeping a food diary, taking pictures and scanning barcodes) reduces user burden and increases self-monitoring adherence(Reference Burke, Swigart and Warziski Turk119,Reference Hales, Turner-McGrievy and Wilcox120) . Of note, research has shown that the frequency rather than accuracy of self-monitoring is more significant in weight loss(Reference Turner-McGrievy, Beets and Moore121). Future studies could examine the efficiency and accuracy of triangulating gesture data with image and sound in self-monitoring for weight loss and actual weight loss.
Several limitations were reported including the lower accuracy of classifiers trained at a group rather than individual level(Reference Fontana, Farooq and Sazonov62,Reference Dong, Scisco and Wilson110) and assessing in a laboratory rather than free-living conditions(Reference Kyritsis, Diou and Delopoulos65,Reference Bastian, Maire and Dugas84,Reference Lin, Yang and Wang95) . Food recognition techniques by detecting chewing and swallowing gestures may be accurate enough to discriminate between hard and soft food items but not the exact food type especially for liquids that do not need chewing(Reference Huang, Wang and Zhang64,Reference Sazonov and Fontana70,Reference Pouladzadeh, Shirmohammadi and Al-Maghrabi78) . This would affect the accuracy of energy intake estimations and non-optimal recommendations were given. In terms of usability, the use of certain wearable devices such as placing electrodes over one’s skin surface for electromyography may not be comfortable and applicable in a free-living condition. Some of the devices also required the user to switch them on and off before and after an eating episode, placing a certain amount of burden on the users. Physical activity may also be misclassified when one performs different types of exercises within the same assessment time frame(Reference Fullerton, Heller and Munoz-Organero88,Reference Kim, Barry and Kang93) . Lastly, sample sizes were small and were comprised of mostly healthy young adults and hence models may not be representative of the entire population, although the data points collected were enough to develop an accurate model(Reference Hegde, Bries and Swibas89,Reference Kim, Barry and Kang93,Reference Mo, Liu and Gao97,Reference Montoye, Pivarnik and Mudd98,Reference Wang, Redmond and Ambikairajah104) . Future studies could take note of these limitations and address them when possible.
Predictive analytics
Positive dietary outcome expectations have been shown to significantly correlate with body fat loss(Reference Dennis, Potter and Estabrooks122), weight loss and weight loss maintenance in obese individuals(Reference Calugi, Marchesini and El Ghoch123). Studies included in this category predicted weight loss based on self-reported or accelerometer-measured exercise intensity (e.g., step count and duration), self-reported diet type (i.e., fat content and food items), the researcher measured anthropometrics, adherence to counselling interventions and socio-demographic profile (i.e., age and sex). Other predictors include weight energy consumption(Reference Babajide, Hissam and Anna124,Reference Babajide, Tawfik and Palczewska125) , initial body composition (mainly fat percentage), social interaction on social media, negatively worded emotional blog posts(Reference Chung, Jones and Liu126,Reference Wang, Liu and Tang127) , the historical success rate in diet and exercise goal achievement and food item consumed (eating poultry was found to be associated with better goal commitment than eating porcine). These studies used clustering, decision trees, bag of visual words approach and linguistic inquiry and word count to classify the data obtained. One study included the temporal closeness of weight loss-related blog posts (i.e., timestamp) and frequency of virtual social interaction (e.g., commenting on friends’ posts) into the predictive models to improve the accuracy of weight loss prediction(Reference Wang, Liu and Tang127). Another study developed an algorithm based on the utility-maximising framework to consider the irrationalities in human behaviour change in its weight loss predictive model(Reference Bastian, Maire and Dugas84). The inclusion of such behavioural concepts could inform the future development of predictive models of public health nutrition and weight loss.
However, despite the strong influence of situational and environmental factors on behavioural self-regulation, only one study included the influence of such factors using EMA in its predictive model(Reference Goldstein, Thomas and Foster48). EMA has been shown to enhance the reliability and validity of data collected by reducing the risk of recall bias and reflect human responses in real-world settings(Reference Shiffman, Stone and Hufford128). Exercise lapses were predicted by the number of weeks one has participated in a weight loss intervention and the average daily steps in comparison to that of the previous week(Reference Goldstein, Zhang and Thomas47,Reference Zhou, Fukuoka and Goldberg105) . On the other hand, dietary lapses were predicted by food type (e.g., oil, pork, fruits) and self-reported EMA factors such as boredom, motivation, cognitive load and tempting food availability(Reference Forman, Goldstein and Zhang50,Reference Weber and Achananuparp129) . In a study on 469 overweight and obese participants who attended a behavioural weight loss programme, negative affect and social situations were identified as dietary lapse triggers at 9 months into the programme while affect, urges and situational dietary adherence were significantly associated with weight loss 12 months into the programme(Reference Swencionis, Smith-Wexler and Lent130). Neither affect, negative physical state, urges and temptations, time pressure, nor social situation was significantly associated with physical activity(Reference Swencionis, Smith-Wexler and Lent130). Suggestively, the predictors of physical activity and dietary adherence differ and future research and interventions should consider examining such differences to develop target and efficient intervention.
Real-time analytics and micro-interventions
Three studies reported significant improvements in participants’ diet and exercise lapse prevention after undergoing a micro-intervention that involved behavioural lapse self-monitoring through smartphone app nudges/prompts(Reference Everett, Kane and Yoo26,Reference Forman, Goldstein and Zhang50,Reference Stein and Brooks53) . This coincides with a study that found a 1 % decrease in the risk of exercise lapse with every additional 10 min of physical activity, suggesting that prior event/experience with self-regulation success increases the likelihood of preceding adherence(Reference Crochiere, Kerrigan and Lampe131). Only two studies reported a statistically significant weight loss in participants who underwent AI-assisted weight loss intervention. The randomised controlled trial with the largest sample size (n 181) only found a significant interventional effect when its interaction with diet type was considered(Reference Forman, Goldstein and Crochiere49). Concurrently, this study reported the lowest completion rate of 62·9 % as compared with the two aforementioned studies with higher completion rates of 86 %(Reference Everett, Kane and Yoo26) and 97·7 %(Reference Forman, Goldstein and Zhang50). Given that larger sample sizes reflect higher generalisability of results, this discrepancy suggests that interventional prompts could only be effective in inducing weight loss if the users react and adhere to the weight loss prompts and recommendations. This is especially when studies have shown that prompts and reminders could be deemed annoying and reduce app utilisation. Future studies should also note issues on legitimacy, privacy, the effort required and an ability to monitor behaviours and goals automatically in real-time(Reference Dennison, Morrison and Conway132).
Potential mechanism of how artificial intelligence can be used to improve self-regulation for weight loss and weight-related behaviour changes
Through this review, we highlight that a large gap in the evidence on how AI can assist in weight loss self-regulation is the lack of integration and synthesis of all three AI function categories. Therefore, we conceptualised the potential use of AI in self-regulation for weight loss based on the current findings and present it in Fig. 4. This mechanism is akin to how humans make behavioural decisions by firstly using our senses to detect and recognise certain behaviours, triggers and outcomes. Next, information is processed and learned in the brain by drawing linkages between past behaviours and current outcomes to anticipate future outcomes. Lastly, anticipations are updated based on new information while the brain decides and self-regulates behavioural outputs to achieve the desired goal(Reference Kennedy133).

Fig. 4 Proposed mechanism of AI-assisted self-regulation
There are several research gaps. Firstly, intervention effectiveness should consider the influence of sex, age and comorbidities which are well-known primary predictors of body weight. Secondly, future studies on AI-assisted weight loss interventions could consider the influence of an obesogenic environment that presents one with various temptations and sets one up for self-regulation failure. Moreover, affect, habit strength and motivation have been well-established to be significant predictors of behaviour change and could be considered in future studies. It is noteworthy that data could be stored and retrieved from a cloud (on-demand data centres over the internet) or edge computing (near the source, e.g., smartphone) devices to allow machine learning algorithms to optimise and personalise existing weight loss predictive models(Reference Kroese and de Ridder134).
Limitations
Firstly, the lack of Chinese database could have limited our search results on the use of AI, especially when China has been rapidly developing their technological capabilities in recent years. Future studies could examine the use of AI in studies published in other languages to facilitate further discussions on the potential of AI in self-regulation for weight loss. Next, as this scoping review aimed to present the potential of AI to enhance self-regulation for weight management, a broad and comprehensive scope of the review was needed. Therefore, although some AI applications were tested on small samples of a mixture of adults who were both healthy and overweight, such articles were included due to the consideration of feasibility that they are still at their infancy of development. Lastly, our search results could be limited to the AI applications published in academic journals and not those which have gone straight to consumer use.
Conclusion
In summary, the current study elucidated the potential use of AI to improve weight loss through a proposed framework that includes machine perception, predictive analytics and real-time analytics with micro-interventions. However, this is contingent upon other situational, environmental and emotional factors that have to be accounted for in the AI architectures. Future studies could compare the effectiveness of AI-assisted self-regulation weight loss programmes and existing behaviour change programmes to assess the resource efficiency of AI-assisted interventions.
Acknowledgements
Acknowledgements: Not applicable. Financial support: This research received no specific grant from any funding agency, commercial or not-for-profit sectors. Conflict of interest: None. Authorship: H.S.J.C.: conceptualisation, data curation, formal analysis, investigation, methodology, project administration, resources, software, supervision, validation, visualisation, writing – original draft, writing – review and editing. W.H.D.A.: formal analysis, resources, software, validation, writing – review and editing. Y.L.: conceptualisation, methodology, resources, software, supervision, writing – review and editing. Ethics of human subject participation: Not applicable.
Supplementary material
For supplementary material accompanying this paper visit https://doi.org/10.1017/S1368980021000598