LEARNING OBJECTIVES
-
Understand what a systematic review is and how to perform a critical appraisal of its strengths and limitations, including identifying the potential sources of bias
-
Understand what a meta-analysis is and when to use it, how to assess its internal and external validity, and the difficulties of clinical and statistical heterogeneity
-
Appreciate advanced methodologies (e.g. individual patient data meta-analysis and network meta-analysis) used to individualise treatment response and evaluate comparative effectiveness
Keeping up to date with current knowledge of the comparative efficacy of different treatments is an essential part of making good clinical decisions during everyday clinical practice (Reference CiprianiCipriani 2013a). To make the best clinical decisions, physicians must combine their own clinical expertise and training with high-quality scientific evidence and the patient's views (Reference Guyatt, Haynes and JaeschkeGuyatt 2000). This combination can create a powerful diagnostic and therapeutic alliance that optimises patients’ quality of life and clinical outcomes. Doctors have a professional obligation to ‘keep [their] professional knowledge and skills up to date’ (General Medical Council 2013). However, keeping abreast of current evidence is a Herculean task. Over 2 000 000 articles are published every year in 20 000 biomedical journals, and even if a clinician were to restrict their reading to high-yield clinical psychiatry journals, they would need to read over 5000 articles a year (Reference Geddes, Wilczynski and ReynoldsGeddes 1999), a task that is simply not feasible for busy clinicians.
To keep up to date efficiently, the clinician needs a system to summarise primary research findings in a form that gives a reliable and easy-to-read synthesis of current knowledge. However, like any other form of research, these summaries (or reviews) vary in quality and are susceptible to various forms of systematic error, or bias. To use reviews effectively, clinicians need to be aware of the potential advantages and limitations of the different types of review, so that they can weigh up the results and the relative merits of the methodology, and thus critically appraise the conclusions of the review.
What is a systematic review?
Systematic reviews synthesise primary research studies using specific methodological strategies to limit the risk of bias. In a systematic review, authors pose a clearly formulated question and use systematic and explicit methods to identify, select and critically appraise all the relevant evidence to address this question (Reference Higgins and GreenHiggins 2011a). Systematic reviews differ significantly from the more traditional (or narrative) reviews (Table 1).
TABLE 1 Comparison of the characteristics of systematic and narrative reviews
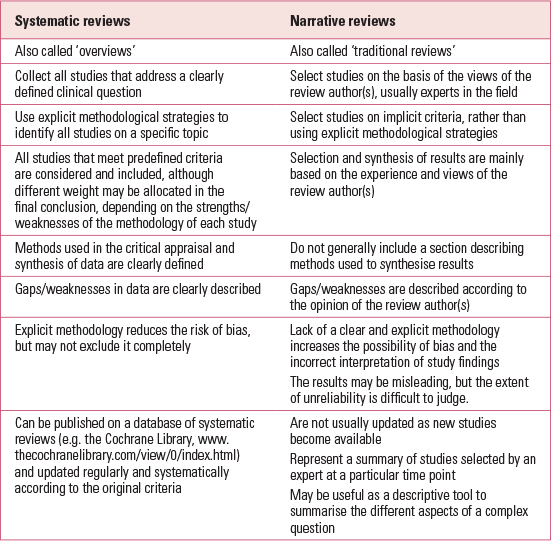
In a systematic review, all methods are described and clearly specified in a review protocol, so that the reader understands exactly which strategies have been used. The methods should be described in sufficient detail to allow anyone else, using the same methodology, to reproduce the same results. This improves the reliability and accuracy of the conclusions.
The first step is to identify a specific question to be addressed by the review. The range of this question needs to be narrow, as it is neither possible nor useful to retrieve all the available evidence on a topic that is too broad or wide-ranging. The nature of the question determines the type of research evidence that will be reviewed (for instance, randomised versus observational studies) and which studies will be included or excluded according to explicit criteria, predefined in the review protocol. For example, a question regarding the efficacy of treatments (Which treatment is better? Which dose is more effective?) is usually best answered by reviewing evidence from randomised controlled trials (RCTs), because randomisation protects against selection bias (Table 2). However, RCTs are not the appropriate trial design for all questions. Questions of aetiology (Does stroke predispose to later depressive disorder?) are better answered by cohort and case–control studies. Diagnostic questions (How well does a screening tool pick up cases of early psychosis?) are best studied with cross-sectional and prospective studies of patients at risk of the disorder. Such studies are called diagnostic validity studies when one diagnostic method is compared with an existing comparator or gold standard.
TABLE 2 Types of bias and the strategies used to minimise bias in RCTs

Once the question has been identified, the review proceeds to the systematic identification of all the relevant studies addressing that question (according to the methods described in the protocol). Published data are often accessed via electronic databases such as PubMed, Embase, PsycINFO and CINAHL. Care needs to be taken in the choice and arrangement of keywords used in the search, as this will have a significant effect on which papers are identified. Reviewers should search not only for published studies, but also for unpublished data and ‘grey literature’ (informally published written material, such as technical reports or working papers from research groups). Reviewers should make all practicable efforts to counteract any publication bias that may exist (see ‘Methods to reduce the effects of bias’ below).
Following identification of the studies, the reviewers critically appraise each one. The extent to which a systematic review can draw conclusions about the effects of an intervention depends on whether the data and results from the included primary studies are valid. A study's validity relates to whether it answers its research question ‘correctly’, that is, without bias (Reference Higgins, Altman and G⊘tzscheHiggins 2011b). The evaluation of the validity of the included studies is therefore an essential component of a systematic review, and should influence the analysis, interpretation and conclusions of that review. High-quality evidence is not always available for all outcomes of interest. In such a case, summary evidence can still be presented, together with a measure of quality to guide the reader, for example using the Grading of Recommendations Assessment, Development and Evaluation (GRADE) approach (Reference Guyatt, Oxman and VistGuyatt 2008). GRADE provides a system for rating the quality of evidence and the strength of recommendations that is comprehensive and pragmatic, and is increasingly being adopted worldwide. This can help to ensure that judgements about the risk of bias, as well as other factors affecting the quality of evidence (such as imprecision, heterogeneity and publication bias), are considered when interpreting the results of systematic reviews.
The Cochrane Library (www.thecochranelibrary.com) is possibly the best-known database of systematic reviews and the website contains within it several different databases. These include the Cochrane Database of Systematic Reviews (CDSR), Cochrane Central Register of Controlled Trials (CENTRAL), Cochrane Methodology Register (CMR), Database of Abstracts of Reviews of Effects (DARE) and Health Technology Assessment Database (HTA).
Meta-analysis
Meta-analysis refers specifically to the use of statistical techniques to summarise data quantitatively as part of a systematic review (Reference Higgins and GreenHiggins 2011a). However, the term is often used more loosely to refer to any systematic review that uses statistical methods to combine, weigh and summarise the results of several studies (Reference Cook, Sackett and SpitzerCook 1995). The results from the original studies (e.g. primary and secondary outcomes, rates of adverse effects) are extracted, put together and analysed statistically in a final pooled estimate. Various statistical software packages are available to perform these analyses, such as RevMan (http://tech.cochrane.org/revman) and Meta-DiSc (www.hrc.es/investigacion/metadisc_en.htm) (which are both free to use), Stata (www.stata.com) and Comprehensive Meta-Analysis (www.meta-analysis.com/index.php). A meta-analysis should take into account the characteristics of each of the primary studies, as the methodological quality of individual trials will affect the quality of recommendations that each meta-analysis can provide. It is important to note that the statistical methods of meta-analysis should only be undertaken following a systematic review (only a systematic review can guarantee transparent and comprehensive collection of all the available evidence, to avoid systematic biases in the selection of studies to be analysed). By contrast, meta-analysis is not an essential part of every systematic review: in some cases it may not be appropriate to combine the results of studies, for example if the original studies are too different from each other.
The overall results of meta-analysis give main treatment effects and relate to the average response in an average patient. Clinical practice, however, involves the assessment and treatment of an individual, and so the results of a subgroup analysis (according to different clinical or socio-demographic characteristics) may at first appear more relevant to the decisions made by clinicians. Subgroup analysis can be performed by combining data from specific subgroups in each study. However, results in subgroups are not always reported in original publications and the randomisation of treatments in the primary studies may not have been stratified according to the same subgroups. In addition, the more subgroup analyses that are performed, the more likely it is that a statistically significant, but incorrect result will be found purely by chance, as shown in Box 1. As a general rule, any subgroup analysis within a meta-analysis should be treated carefully and is best regarded as generating hypotheses for testing in the future, rather than providing reliable evidence about a particular subgroup.
BOX 1 The effects of chance on a subgroup analysis
Reference Counsell, Clarke and SlatteryCounsell et al (1994) conducted an investigation of the effects of chance on the results of a systematic review containing a subgroup analysis of a fictional treatment called DICE:
-
44 randomised trials were simulated by rolling dice – each roll of the die yielding the outcome for one ‘patient’
-
each investigator performed two trials to simulate the effect of gaining experience with the intervention
-
it was pre-specified that subgroup analyses would be performed to distinguish each investigator's first trial from their second.
Overall, chance alone showed that ‘DICE treatment’ was non-significantly better than ‘control’, as measured by death rates. Overall, the analysis did not show a significant difference in death rates for DICE treatment. However, in a subgroup analysis looking only at ‘published’ trials (using a model of publication bias from real trials) performed by ‘experienced’ operators (second trials only), there was a significant 23% reduction in mortality. Thus, significant subgroup effects can be found due to chance alone.
Remember the meaning of the acronym DICE – Don't Ignore Chance Effects.
Strengths and potential pitfalls of meta-analysis
Strengths
Meta-analysis as a statistical tool has great strengths. Effect size is the estimate of the effect of a treatment in a study (e.g. the risk ratio or odds ratio for dichotomous outcomes and the mean difference or standardised mean difference for continuous outcomes (Reference Nikolakopoulou, Mavridis and SalantiNikolakopoulou 2014)), and the techniques of meta-analysis pool research data from a number of studies to provide an overall estimate of effect size in an easily digestible form. The results of a meta-analysis are usually presented in a forest plot or ‘blobbogram’ (Reference Cipriani and BarbuiCipriani 2006). In the plot the left-hand column lists the names of the studies (usually in chronological order) and the right-hand column shows the effect size for each of them (often represented by a square) incorporating confidence intervals represented by horizontal lines. The meta-analysed measure of effect is usually plotted as a diamond, the lateral points of which indicate confidence intervals for this estimate. By combining the effect sizes statistically, the meta-analysis produces much larger sample sizes, minimising random error and increasing the generalisability of the study results. In addition, the methods used in the analysis assess the quality of the included studies and thus the reviewers can indicate the strength of the summary evidence they report (Reference Higgins, Altman and G⊘tzscheHiggins 2011b).
Potential pitfalls
The methodology of the systematic review
Care should always be used in the interpretation of the results of a meta-analysis, as their validity is dependent on the methodology of the original systematic review. If this was not properly conducted, the results of the meta-analysis will be biased. When reading a systematic review it is important to be able to assess its merits, as not all systematic reviews use the same methodology (Box 2). The extent to which bias has been controlled gives a measure of the internal validity of the study. External validity (or generalisability) gives a measure of the extent to which the results provide a correct basis for generalisations to other circumstances.
BOX 2 How to appraise the merits of a systematic review and meta-analysis
-
What are the affiliations and financial support for the review and its authors?
-
What are the methods used to identify and select the primary studies on which the review is based?
-
What was the quality of the primary studies?
-
Were the analysis and synthesis appropriate?
-
Were possible sources of bias taken into account?
-
What was the statistical and clinical significance of the results?
-
Has there been an update of the literature search?
The quality of primary studies
The results of the analysis will also be affected by the quality of the primary studies. If the quality is poor, then it may not be possible to achieve meaningful results from meta-analysis: ‘garbage in, garbage out’. A meta-analysis needs to determine to what extent variations in study quality affect the decision to combine the data.
Many tools have been proposed for assessing the quality of studies for use in the context of a systematic review and meta-analysis. Most tools are either scales, on which various components of quality are scored and combined to give a summary score, or checklists, in which specific questions are asked (Reference Jüni, Altman and EggerJüni 2001). Many instruments contain not only items based on the generally accepted criteria for methodological quality (randomisation, allocation concealment, masking/blinding), but also items that are not directly related to internal validity, such as the presence of a power calculation (which relates more to the precision of the results) or whether the inclusion and exclusion criteria are clearly described (which relates more to applicability than validity) (Reference Moher and OlkinMoher 1995). Probably the best example of methods used for assessing quality in RCTs is CONSORT (www.consort-statement.org), but there are different methods for other study designs. These include QUADAS (www.bris.ac.uk/quadas) for studies of diagnostic test accuracy, STROBE (www.strobe-statement.org) for observational studies and TREND (www.cdc.gov/trendstatement) for nonrandomised studies.
These tools vary and some focus more on the quality of reporting than on the underlying study methodology. To address this problem, the Cochrane Collaboration recommends assessing study quality using its ‘risk of bias’ tool, which is neither a scale nor a checklist. It is a domain-based evaluation, in which critical assessments are made separately for different study-related issues: random sequence generation; allocation concealment; masking/blinding of participants and personnel; masking/blinding of outcome assessment; incomplete outcome data; selective reporting; and other sources of bias (Reference Higgins, Altman and G⊘tzscheHiggins 2011b).
Addressing the clinical and statistical heterogeneity of studies
Studies always vary, for example in terms of the types of participants involved, the methods used, the types of intervention used as a comparator, the length of follow-up and the outcomes measured. Therefore, there will need to be an element of selection of studies for inclusion. To avoid bias, before starting the review it is very important to specify the main criteria for selecting studies in the review protocol. Reviewers need to avoid over-inclusion of disparate studies, but also over-exclusion of studies that have relevant data. However, even if the inclusion/exclusion criteria are clear and coherent, sometimes the included studies differ significantly. This ‘heterogeneity’ can present challenges. It may not be possible to merge the results and perform a meta-analysis; where there is significant heterogeneity this has been likened to the error of ‘combining oranges and apples’ (Reference EysenckEysenck 1994). Even if it is possible to pool the studies, heterogeneity may well be found during the analysis. If so, usually a random effects model analysis is recommended, as this recognises that the observed differences in effect sizes between different studies reflect true heterogeneity as well as random error (Reference Nikolakopoulou, Mavridis and SalantiNikolakopoulou 2014). For this reason, pooled estimates from such an analysis have wider confidence intervals and results are more conservative than a fixed effects analysis.
An example of the difficulties involved in addressing heterogeneity in studies in psychiatry is the question of the effectiveness of community treatment, either intensive or standard, in improving the outcome of patients. The systematic reviews addressing this question have all struggled with similar issues. For example, the definitions of ‘community treatment’ and ‘control treatment’ vary significantly between the centres conducting the trials and have changed over the time that the studies have been conducted. Complex mental health interventions and services are difficult to standardise, and also the labels ‘standard care’ and ‘usual services’ used as the control treatment are often ill-defined and may overlap with the active treatment. In addition, studies have differed in their choice of the best indicator of outcome, with different measures used (Reference Dieterich, Irving and ParkDieterich 2010). One approach (e.g. Reference Marshall, Gray and LockwoodMarshall 2000a,Reference Marshall and Lockwoodb) is to rely on the labels (such as assertive community treatment, case management, standard care) given to each treatment arm by the investigators in the original studies. This is a practical solution, but may well mask an underlying ‘clinical heterogeneity’ in the different treatment arms. Some reviews (e.g. Reference Murphy, Irving and AdamsMurphy 2012) have found only small numbers of studies that meet their criteria. In addition, many of the studies in this area have small sample sizes (e.g. Reference Malone, Marriott and Newton-HowesMalone 2007), giving them inadequate power to detect statistically significant outcome differences, leading to ‘statistical heterogeneity’. Catty et al (2002) used broader inclusion criteria in order to include more studies and increase the overall sample size. They included all studies of ‘home treatment’, which encompassed any treatment outside hospital. Despite these broad inclusion criteria, and the choice of only one outcome measure (days in hospital) and intensive follow-up of the authors of the primary studies, they found that only 57% of the studies yielded data that were usable in their meta-analysis. This is typical for systematic reviews in this area, and severely limits generalisability.
Summary
The results of a meta-analysis rely not only on the methodology used in the systematic review and meta-analysis, but also on the quality of the studies used as the primary data source. Systematic reviews and meta-analyses on the same topic may produce conflicting results. For example, since the publication of the landmark paper by Caspi and colleagues (Reference Caspi, Sugden and MoffittCaspi 2003) suggesting that the serotonin transporter gene modifies the relationship between stressful life events and depression, a number of individual studies on the subject have been conducted. Meta-analyses of those studies have been contradictory, with some (e.g. Reference Risch, Herrell and LehnerRisch 2009) not supporting and others (e.g. Reference Karg, Burmeister and SheddenKarg 2011; Reference Miller, Wankerl and StalderMiller 2013) supporting such a gene–environment interaction. So, even though meta-analysis is probably the most robust tool currently available to summarise the evidence, the results are rarely unequivocal and always need careful appraisal and interpretation.
Bias in systematic reviews and meta-analyses
Bias can occur during the selection, appraisal or synthesis of data and should be avoided, as it gives inaccurate or misleading results. Types of bias are summarised in Box 3.
BOX 3 Types of error and bias in systematic reviews and meta-analyses
-
Poor quality of the primary studies (which tends to over-represent a favourable outcome)
-
Selective reporting within the primary studies (usually of significant and favourable results)
-
Bias in the selection of included studies for the review:
-
publication bias – large positive studies are more likely to be published
-
language bias – English language articles are more likely to be selected by the reviewers
-
studies listed on electronic databases are more likely to be identified
-
the preferences of the reviewers in selecting the included studies
-
-
Bias in the statistical methods used to extract and pool data
-
Bias in the assumptions/simplifications made by the authors in extracting and/or pooling the data
-
Funding/sponsorship bias (which tends to favour the treatment arm supported by the sponsor)
A key source of bias in systematic reviews is publication bias, which occurs as a result of the tendency for authors, reviewers and editors to publish preferentially studies that have a clearly defined, statistically significant result (Reference Mavridis and SalantiMavridis 2014). Studies where the treatment has a similar or lesser effect than placebo, or than the current well-established treatment, are less likely to be published. Publicly funded research is more likely to be published whatever the results, whereas commercially funded research shows a significant bias towards publication when the findings are positive (Reference DickersinDickersin 1990). A meta-analysis based purely on published results may well be misleading as the published set of data may not be a representative sample of the overall evidence (Reference Higgins, Altman and G⊘tzscheHiggins 2011b). For example, Reference Turner, Matthews and LinardatosTurner et al (2008) obtained reviews from the US Food and Drug Administration (FDA) of unpublished studies of antidepressants submitted for regulatory approval. The authors matched results from unpublished reports with the corresponding publications, if available. Interestingly, 31% of studies were not published. Positive results were much more likely to be published and, of the negative studies that were published, the majority were presented in a way that conveyed a positive outcome. As a result of selective reporting, the published literature conveyed an effect size nearly a third larger than that derived from the FDA data. Reference Whittington, Kendall and FonagyWhittington et al (2004) also highlighted the different recommendations in prescribing practice that could be deduced from analysing only the published data, using the example of studies of selective serotonin reuptake inhibitors (SSRIs) versus placebo in the treatment of depression in children aged 5–18. As the majority of clinical decisions weigh efficacy against risk of harm/side-effects, the non-reporting of negative studies could make a significant difference. When reading a meta-analysis, it is important to check whether the authors did search for unpublished studies and unpublished supplementary data.
To address the suboptimal reporting, in meta-analyses, of methodological problems such as potential publication bias, an international group developed guidance called the Quality of Reporting of Meta-Analyses (QUOROM) Statement, which focused on the reporting of meta-analyses of RCTs (Reference Moher, Cook and EastwoodMoher 1999). More recently these guidelines have been revised and renamed Preferred Reporting Items for Systematic Reviews and Meta-Analyses (PRISMA; Reference Moher, Liberati and TetzlaffMoher 2009). The PRISMA guidelines contain a checklist to assess the various elements of quality of a systematic review (with or without meta-analysis) and also to guide authors when reporting their findings. Following publication of the PRISMA statement, the UK Centre for Reviews and Dissemination (CRD) at the University of York developed the international Prospective Register of Systematic Reviews with Health-Related Outcomes, or PROSPERO (Reference Booth, Clarke and DooleyBooth 2012; www.crd.york.ac.uk/PROSPERO). The objectives are to reduce unplanned duplication of reviews and provide transparency in the review process, with the aim of minimising reporting bias.
Methods to reduce the effects of bias
The example of publication bias
Prevention of publication bias (a prospective method) is likely to be the most effective strategy. One approach is to create trial registries in which the details of trials are recorded before they commence, to capture data from all studies, whether eventually published or not (Reference De Angelis, Drazen and FrizelleDe Angelis 2004). Another suggestion is a trial amnesty, where researchers are encouraged to submit for publication reports of previously unpublished trials (Reference HortonHorton 1997). However, these systems are difficult to implement and many trials pre-date trial registries by some years and their data are not available to the public (Reference GoldacreGoldacre 2013). Overall, although prospective strategies may reduce the problem of publication bias in the future, it is likely to remain an issue that will need to be addressed to a greater or lesser extent in all meta-analyses for some time to come.
Retrospective methods attempt to compensate for publication bias after the event. For example, reviewers should make every effort to find all available data, including unpublished and non-English language published studies. As well as electronic searches, they should also hand search, check references and conference abstracts, and communicate directly with authors. Other sources of data include the websites of regulatory agencies (e.g. the FDA (www.fda.gov), the European Medicines Agency (www.ema.europa.eu)), pharmaceutical companies (e.g. the GlaxoSmithKline Clinical Study Register (www.gsk-clinicalstudyregister.com) and independent organisations such as the World Health Organization (http://apps.who.int/trialsearch), ClinicalTrials.gov (https://clinicaltrials.gov) and the European Union Clinical Trials Register (www.clinicaltrialsregister.eu). However, efforts to include unpublished data can present a double-edged sword. The data can be difficult to retrieve or can be incomplete, not representative of the sample being studied and may not have been peer reviewed. The methods of a meta-analysis should recognise that, despite the best efforts of the reviewers, there is likely to be a degree of publication bias in the studies selected for a systematic review.
Researchers attempt to detect publication bias using a number of statistical tests (e.g. Egger's test and funnel plots) that rely on the underlying theory that studies with small sample size will be more prone to publication bias, whereas larger studies are more likely to be published regardless of their findings (Reference Egger, Davey Smith and SchneiderEgger 1997). In a funnel plot, effect sizes are plotted on the horizontal axis against a measure of the weight/size of each study (e.g. standard error or sample size) on the vertical axis. A symmetrical funnel will be formed if publication bias is absent, but the funnel will be skewed or asymmetrical if it might be present (Reference Egger, Davey Smith and SchneiderEgger 1997). It is common, therefore, for a meta-analysis to show a funnel plot and perform tests such as the ‘trim and fill’ method to identify and adjust for asymmetry (Reference Duval and TweedieDuval 2000). Asymmetry is often interpreted as showing direct evidence of the presence of publication bias. However, this is too simplistic: asymmetry may also result from an essential difference (or heterogeneity) between smaller and larger studies (Reference Lau, Ioannidis and TerrinLau 2006). For example, small studies may focus on high-risk patients, for whom treatment may be more effective; or small studies may have a shorter follow-up. Variation in quality also affects the shape of the funnel plot, with smaller, lower-quality studies showing greater benefit of treatment.
Examples of advanced methodology
Individual patient data meta-analysis
As already mentioned (see ‘Meta-analysis’ above), subgroup analysis within a standard meta-analysis has significant limitations. Individual patient data meta-analysis (IPDMA) is a potentially useful approach in which a meta-analysis is conducted using the data on individual patients from primary studies (Reference ClarkeClarke 2005). This allows more accurate subgroup analyses because they can be based on common subgroup classification across studies. It is crucial that the meta-analysis preserves the original clustering of the patients within studies: it is inappropriate to analyse the data from all the patients as if they had all participated in the same study. However, an appropriate analysis can produce results that inform evidence-based practice, such as a pooled estimate of treatment effect across all studies, how the treatment effect varies between studies (e.g. with treatment dose or study location) and varies across types of patients (e.g. grouped by age or stage of disease). IPDMA has many potential advantages over meta-analyses using aggregate data, where the data are sometimes poorly reported, not available or presented differently across studies (Reference Riley, Lambert and Abo-ZaidRiley 2010). Use of individual data standardises study methods and often provides extra data (e.g. longer follow-up, more outcome measures) not included in the original aggregate publication. However, IPDMA is a highly time-consuming and resource-intensive approach, for both the reviewers and the original study authors; it requires advanced statistical methods and the original data may well be poor or missing. It has not been widely used in psychiatry as yet, although there are some examples of how IPDMA can help clinicians weigh up the benefits of psychiatric treatment in the individual patient (e.g. Reference Furukawa, Levine and TanakaFurukawa 2015). The proposal of Reference Tudur Smith, Dwan and AltmanTudur Smith and colleagues (2014) to start a central repository of individual patient data from trials would substantially reduce the time required to source the original data.
Network meta-analysis
Meta-analyses use as their standard statistical technique pair-wise comparisons of treatments. This means that when reviewing the data on the efficacy of all available treatments for a particular condition, the clinician is presented with an array of pair-wise comparisons, whereas they would rather compare the relative efficacy of all treatments simultaneously. In addition, some comparisons between treatments have not been studied directly and so there are no direct data on which to base a pair-wise comparison. Network meta-analysis (NMA) (also called multiple treatments meta-analysis or mixed treatment comparison) is a statistical method that can fill this gap as it allows multiple treatments to be assessed at the same time, using direct and indirect evidence from the comparison data available (Reference Caldwell, Ades and HigginsCaldwell 2005). The indirect evidence comes from inferring the relative efficacy of two drugs that have not been directly compared with each other, but that have each been directly compared with the same comparator drug. So for example, as shown in Fig. 1, if there are trials of drug A v. drug B, then this gives us direct information on their efficacy relative to each other. Trials of drugs A v. C and drugs B v. C can also supply indirect data on the relative efficacy of A v. B. The use of indirect evidence performs two functions: it provides data on comparisons for which no trials exist and it improves the precision of the direct data by adding indirect data (and therefore reducing the width of the confidence intervals of the estimate of efficacy provided) (Reference Cipriani, Higgins and GeddesCipriani 2013b).
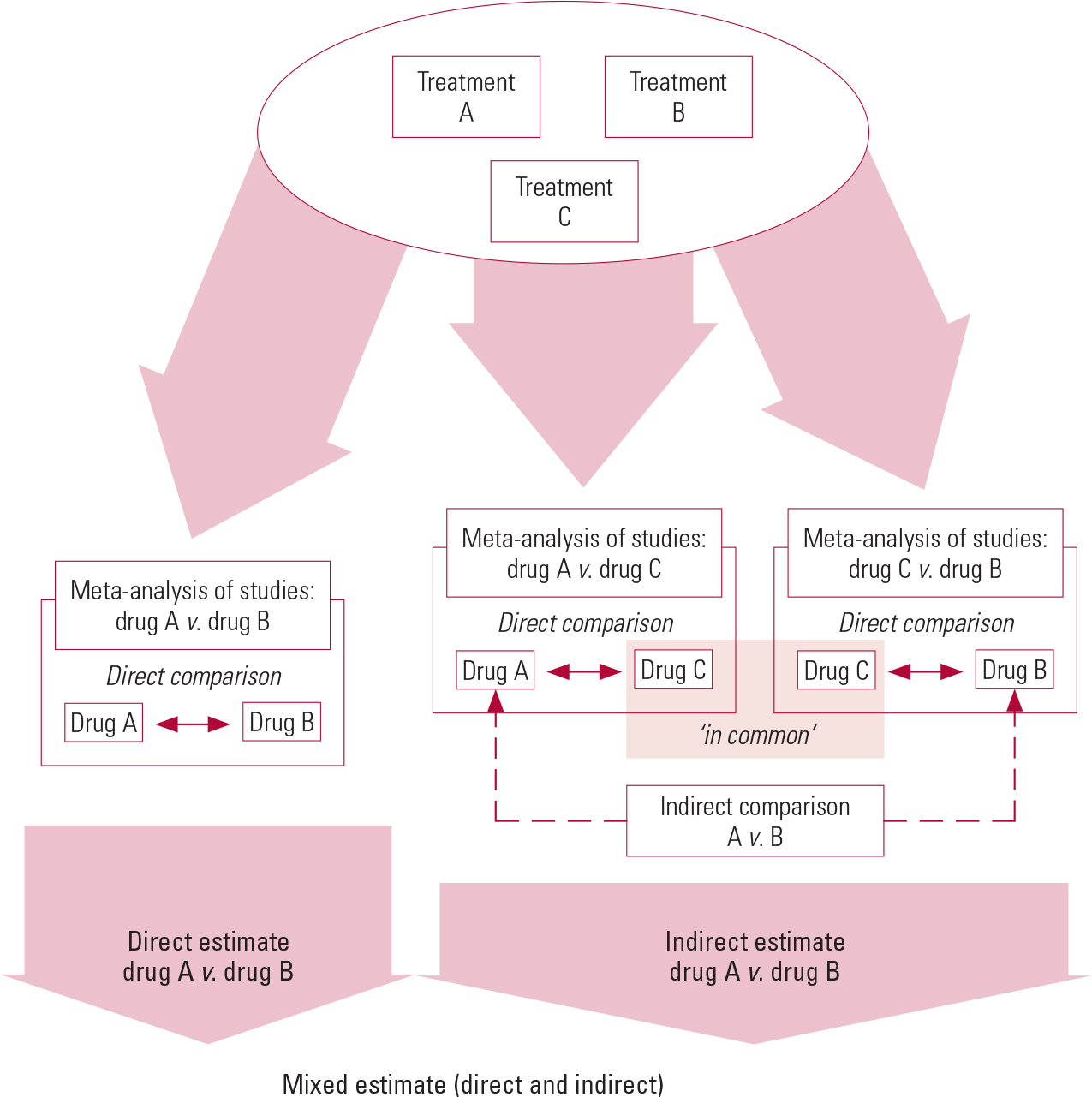
FIG 1 The combination of direct and indirect evidence into a single effect size for treatment A v. treatment B (mixed estimate).
NMA has a useful role, not only in strengthening the evidence base, but also in ranking treatments for specific disorders against each other according to an outcome of interest, for example efficacy and acceptability. This allows a summary of all treatments for which evidence, whether indirect or direct, is available, to be ranked against each other, producing a table (similar to a mileage table in a road atlas) showing the relative efficacy and tolerability of each agent. Examples of well-conducted NMA reviews with robust methodology include: antimanic drugs in acute mania (Reference Cipriani, Barbui and SalantiCipriani 2011; Reference Yildiz, Nikodem and VietaYildiz 2015), maintenance treatments for bipolar disorder (Reference Miura, Noma and FurukawaMiura 2014), antidepressants for acute treatment of unipolar depressive disorder (Reference Cipriani, Furukawa and SalantiCipriani 2009), augmentation agents in treatment-resistant depression (Reference Zhou, Ravindran and QinZhou 2015a), psychotherapies for depression in children and adolescents (Reference Zhou, Hetrick and CuijpersZhou 2015b), treatments for social anxiety (Reference Mayo-Wilson, Dias and MavranezouliMayo-Wilson 2014) and antipsychotic drugs for the acute treatment of schizophrenia (Reference Leucht, Cipriani and SpineliLeucht 2013). The advantages of this approach are clear, and the information is easy to understand and to apply to clinical practice. Thus, NMAs have increasingly been employed to support clinical guidelines and health technology appraisals (Reference Barbui and CiprianiBarbui 2011). However, despite the advantages, NMAs are not yet established practice. Some concerns have been expressed about the validity of the methods employed. Although randomised evidence is used and the indirect evidence preserves the original randomisation, the indirect evidence is not itself randomised evidence as treatments have originally been compared within but not across studies and such a comparison may therefore be subject to bias. Therefore, direct evidence is more robust and indirect evidence should ideally be used as a supplement to direct evidence. However, in the majority of cases, direct and indirect evidence are in agreement (Reference Song, Harvey and LilfordSong 2008).
Conclusions
Evidence-based medicine has developed substantially in the past few decades. Initially, the focus was to provide the best evidence available to answer specific therapeutic questions. Much time and effort have rightly been focused on the best way, incorporating the most rigorous methodology, to provide that evidence. Generating, summarising and understanding the best available evidence are essential for establishing the benefits and safety of interventions, and systematic reviews, often including meta-analyses, have become a valuable tool towards these ends.
However, systematic reviews as a study design have limitations and a number of issues need to be addressed before implementing evidence synthesis in clinical practice (Reference Berlin and GolubBerlin 2014). The clinical heterogeneity of psychiatric patients and the sometimes variable quality of the primary studies make some reviews difficult to interpret and to use. The questions posed in the clinic are often much more complex than those answered by a systematic review. Clinicians (but also researchers, guideline developers, journal editors and critical readers of the literature) should be aware of this, because understanding the limitations and the potential of meta-analytic evidence is crucial to delivering better care to patients. Clinicians need to develop the skills required to feel confident using evidence-based practice in their approach to clinical questions on a daily basis. Publications such as Evidence-Based Mental Health (http://ebmh.bmj.com) are changing their approach somewhat to address these needs (Reference Cipriani and FurukawaCipriani 2014), for example by including real-time online ‘clinical conferences’ via Google Hangout, which use evidence-based practice to demonstrate how to address complex clinical questions in a practical way, and a regular statistics section (an area often neglected when reading papers). Evidence-based practice will continue to provide challenges for clinicians, but as they gain confidence in the techniques required and incorporate them into routine clinical practice, those challenges will reap rewards that are well worth the effort.
MCQs
Select the single best option for each question stem
-
1 Which of the following is not true of a well-conducted systematic review?
-
a Studies on a specific topic are identified
-
b Studies that meet predefined criteria are included
-
c The methods used to appraise and synthesise the data are clearly defined
-
d The review is regularly updated using the original criteria
-
e The systematic and explicit methods used eliminate the possibility of bias.
-
-
2 Which of the following is not true regarding bias?
-
a The risk of bias is greater for narrative reviews than for systematic reviews
-
b One of the aims of guidelines such as the PRISMA statement is to minimise publication bias
-
c Masking/blinding of outcome assessors may help overcome selection bias
-
d The meta-analysis of a biased systematic review will also be biased
-
e The Cochrane Collaboration recommends a domain-based bias tool.
-
-
3 Which of the following is not true regarding meta-analysis?
-
a It pools data statistically from different studies to give an overall estimate of effect size with a greater sample size
-
b Heterogeneity is usually addressed using a fixed effects analysis
-
c It is the use of statistical techniques to quantitatively summarise data
-
d Meta-analyses of the same question can give significantly different conclusions
-
e Its results can be summarised in a forest plot.
-
-
4 Which of the following is not true of individual patient data meta-analysis?
-
a It can include data obtained, but not reported in the original studies
-
b It can investigate how treatment effects vary across centres
-
c It allows subgroup analysis if individual data are preserved in their original clusters
-
d The statistical methods are easy to use and data retrieval is not time-intensive
-
e It can analyse how treatment effects vary in different patient groups.
-
-
5 Which of the following is not true regarding network meta-analysis?
-
a It uses only indirect evidence to compare treatments
-
b Treatments can be ranked against a specific variable, e.g. efficacy or tolerability
-
c Indirect data can provide information where no direct comparison exists
-
d Indirect data can be added to direct data to increase the sample size of that comparison
-
e The results can be easily understood by clinicians and applied to clinical practice.
-
MCQ answers

1 | e | 2 | c | 3 | b | 4 | d | 5 | a |
Acknowledgements
K.A.S. and A.C. acknowledge support from the National Institute for Health Research (NIHR) Oxford cognitive health Clinical Research Facility. J.G. is an NIHR Senior Investigator. The preparation of this article was supported by the NIHR Collaboration for Leadership in Applied Health Research and Care Oxford. The views expressed are those of the authors and not necessarily those of the NHS, the NIHR or the Department of Health.
eLetters
No eLetters have been published for this article.