Introduction
Second language acquisition (SLA) is studied under a wide range of perspectives, and depending on the perspective and the research question pursued, different methods are meaningfully employed to empirically ground the research. This rich landscape is also reflected in instructed SLA (ISLA, Loewen & Sato, Reference Loewen and Sato2017), but the ISLA focus on the best way to teach and learn a second language (L2) brings with it a particular concern for the generalizability of laboratory research to classroom contexts (cf. Loewen, Reference Loewen, Phakiti, De Costa, Plonsky and Starfield2018, p. 672). The generalizability and replicability of results from experimental research is increasingly also a concern throughout the field of SLA, as recently illustrated by the call for replication studies with nonacademic subjects (Andringa & Godfroid, Reference Andringa and Godfroid2019) designed to broaden the traditional focus on experiments with academic, college-age subjects.
Combining those two lines of thought, a population that arguably is underresearched in SLA are school children (K–12) in their authentic learning context. In 2016, there were almost 22 million school children in upper secondary schools (ISCED level 3, age ≈ 14–18) in Europe, with 94% learning English and almost 60% of them studying two or more foreign languages.Footnote 1 Conducting more research in regular foreign language classrooms arguably could help increase the impact of SLA on real-life language teaching and learning in school, which so far seems to be rather limited. While in many countries the language aspects to be taught at a given grade level are regulated by law, where are school curricula actually based on empirically grounded L2 research? Where is it informed by what can be acquired by which type of student at which time using which explicit/implicit instruction methods? In the same vein, textbook writers in practice mostly follow publisher traditions rather than empirical research about developmental sequences, effective task and exercise design, or the differentiation needed to accommodate individual differences. While political and practical issues will always limit the direct throughput between research and practice, scaling up SLA research from the lab to authentic classrooms to explore and establish the generalizability and relevance of the SLA findings in real-life contexts would clearly strengthen the exchange. Note that scaling up as a term from educational science is not just about numbers, but about “adapting an innovation successful in some local setting to effective usage in a wide range of contexts” (Dede, Reference Dede and Sawyer2005, p. 551), which requires “evolving innovations beyond ideal settings to challenging contexts of practice.” This has much to offer, in both directions, given that the data from such ecologically valid formal education settings could arguably be an important vehicle for more integration of SLA perspectives focusing on aspects of learning at different levels of granularity. In real-life learning, all social, cognitive, task, and language factors are simultaneously present and impact the process and product of learning. In sum, we conclude with Mackey (Reference Mackey, Loewen and Sato2017) that “in order to better understand the relationship between instructional methods, materials, treatments, and L2 learning outcomes, research needs to be carried out within the instructional settings where learning occurs” (p. 541).
But how can we scale up ISLA research to real-life contexts where many factors cannot be controlled and the intervention itself is carried out by others, with many practicality issues and a range of educational stakeholders (teachers, students, parents, administrators, teacher educators, and politicians)? While it seems crucial to establish that the effects piloted in lab studies still show up and are strong enough to be relevant under real-world conditions, how can we methodologically deal with the loss of focus and control this entails and successfully set up intervention experiments that support valid interpretations related to SLA theory when carried out in a real-life setting?
Fortunately, this type of challenge is already being tackled by clinical research and educational science, where randomized controlled field trials (RCTs) are increasingly the method of choice for conducting empirically informed research in the field, supporting experimentally controllable, generalizable results and ecological validity. Hedges and Schauer (Reference Hedges and Schauer2018) concluded their recent survey with the vision of a “promising future for randomised trials,” arguing that a “growing focus on interdisciplinary research has provided a richer context to both the implications and the role of education research” (p. 273). For ISLA, a look at the What Works Clearing House (https://ies.ed.gov/ncee/wwc) seems to indicate, though, that we are at the very beginning of this process. So far, the database seems to include no RCTs targeting foreign language learning. Where education and psychology researchers start to address foreign language learning, such as in a recent RCT on English language learners in U.S. schools (Vaughn et al., Reference Vaughn, Martinez, Wanzek, Roberts, Swanson and Fall2017), the focus is on content knowledge and comprehension, to which the breadth and depth of perspectives on language learning in SLA would clearly have much to add.
While conducting RCTs comes at a significant organizational, conceptual, methodological, and financial cost, for some aspects of ISLA these costs can be significantly reduced (at least in the long run) by developing interactive and adaptive technology that readily scales individualized learning opportunities to large numbers of learners. A range of technologies are being used for ISLA (cf. Plonsky & Ziegler, Reference Plonsky and Ziegler2016), though interestingly the authors explicitly pointed out the need for more research on learners in K–12 school contexts (p. 31). In terms of technologies lending themselves to scaling up interventions, there are more than 50 years of history of intelligent tutoring systems (ITS) in education (Corbett, Koedinger, & Anderson, Reference Corbett, Koedinger, Anderson, Helander, Landauer and Prabhu1997). Yet foreign language learning is absent from the meta-analysis of ITS effectiveness by Kulik and Fletcher (Reference Kulik and Fletcher2016), for which they systematically collected all studies reporting on any kind of ITS, though some research has targeted writing and literacy development in the native language (Nye, Graesser, & Hu, Reference Nye, Graesser and Hu2014). In the tutoring system field, language is often characterized as an “ill-defined domain” (Lynch, Ashley, Aleven, & Pinkwart, Reference Lynch, Ashley, Aleven and Pinkwart2006) in contrast to mathematics, computer science, the natural sciences, and other subject domains for which such ITS have been developed. This characterization is rooted in the difficulty of explicitly characterizing the space of possible paraphrases and variation offered by human language. Computationally analyzing the even more variable interlanguage of L2 learners poses additional challenges. As argued in Meurers and Dickinson (Reference Meurers and Dickinson2017), learner language analysis requires integration of the language analysis with task and learner modeling. In line with this argumentation, the only ITS that, as far as we know, are used in regular foreign language teaching—the E-Tutor (Heift, Reference Heift2010), Robo-Sensei (Nagata, Reference Nagata2010), and TAGARELA (Amaral & Meurers, Reference Amaral and Meurers2011)—were all developed by researchers who were also directly involved in foreign language teaching at the university level, allowing them to integrate computational, linguistic, task, and learner knowledge. With increasing appreciation of the relevant concepts across the fields, methodological advances in computational linguistics and machine learning, and the widespread use of mobile phones and internet-connected computers by children, the time seems ripe to build on this line of work by scaling up web-based intervention research integrated into real-life school education.
This article takes a step in this direction. First, we discuss the issues involved in setting up an ITS for English, the FeedBook, that can serve as an experimental ISLA sandbox fully integrated into regular school classes. Second, we report on the first results of an RCT currently running in a full-year intervention in 12 seventh-grade classes in German secondary schools. Complementing the specific results of this study, we conclude with a discussion of the challenges and opportunities that this perspective opens up.
Addressing the Challenge With an ICALL Web Platform Supporting RCTs
There is a clear challenge we want to address: We want to conduct SLA interventions in an ecologically valid classroom setting to ensure that the effects generalize to this important real-life context of language learning. Being ecologically valid also means that noise and practical concerns reduce the accuracy and consistency of an intervention, and that a wide range of properties of students, teachers, parents, schools, and curriculum are simultaneously at play and interact. To be able to observe a reliable effect under such conditions requires large numbers of subjects in representative contexts, together with random assignment to control and treatment groups, as the gold standard for establishing internal validity. Large-scale RCTs thus are generally seen as a necessary step toward the successful scale-up of education interventions (McDonald, Keesler, Kauffman, & Schneider, Reference McDonald, Keesler, Kauffman and Schneider2006). McDonald et al. (Reference McDonald, Keesler, Kauffman and Schneider2006) also pointed out that for successful implementation of such a study, it is crucial to understand the context in which interventions are implemented and student learning occurs.
The school context in which foreign language teaching and learning happen is regulated, and teacher training and materials are (in principle) aligned with those standards. In contrast to setting up a laboratory experiment, the starting point for setting up a field study has to be the current state curriculum, which in essence constitutes the baseline that we then can set out to modify in our intervention. We therefore started our 3-year research transfer project, FeedBook (October 2016 to September 2019), with the current practice of teaching English in secondary, academic-track schools (Gymnasium) in Germany and established a collaboration with the school book publisher Diesterweg of the Westermann Gruppe, who agreed to provide us with the government-approved textbook, Camden Town Gymnasium 3, and its workbook materials. We focus on the seventh-grade curriculum, given that at this stage, some aspects of the linguistic system of the target language are in place, supporting a range of meaning- and form-based activities, but the curriculum still explicitly builds up the language system. The English curriculum for seventh grade in academic-track secondary schools in the state of Baden-Württemberg refers to building the linguistic resources needed to talk about facts, actions, and events that are in the present, past, future, or hypothetical; to compare situations; and to report on actions from different perspectives (Kultusministerium, 2016, p. 33).
The Camden Town book was inspired by Task-Based Language Teaching (TBLT; Ellis, Reference Ellis2003). The book for seventh grade includes six chapters, called themes, with each theme fostering particular competencies and including spelled-out target tasks. The grammar topics required by the state's English curriculum are integrated into these themes. The first four themes of the book are generally covered by every class in the seventh grade and form the basis of the FeedBook:
• Theme 1. On the move: problems growing up, leaving home
– Competencies: expressing problems, feelings, speculations, opinions; giving and evaluating advice
– Target tasks: writing a letter, a report from another perspective, contributing to an online chat
– Integrated grammar focus: tenses, progressive aspect, gerunds
• Theme 2. Welcome to Wales: moving, selecting a new school
– Competencies: obtaining, evaluating, and comparing information from different sources, describing hypothetical situations
– Target tasks: giving a presentation on Wales, writing a diary entry and an email discussing school differences
– Integrated grammar focus: comparatives, conditional clauses, relative clauses
• Theme 3. Famous Brits: time travel, British history, theater
– Competencies: identifying information in reading and listening, taking notes, expressing preferences, motivating opinions, paraphrasing
– Target tasks: creating and presenting a collage, a short presentation, a theatre scene
– Integrated grammar focus: past perfect, passive
• Theme 4. Keep me posted: internet communication, relationships
– Competencies: skimming texts, conducting a survey, presenting results, reporting on experiences, voicing conjectures, speculating, providing feedback
– Target tasks: comment on social media posts, design and perform a role play, create a web page, write the end of a photo story
– Integrated grammar focus: reported speech, reflexives
Since we aimed at developing an experimentation platform integrated with the regular teaching in seventh grade for the entire year, we implemented 230 exercises from the workbook, plus 154 additional exercises from a supplemental workbook of the same publisher offering exercises at three levels of difficulty. We left out 36 exercises that require partner work or are otherwise unsuitable for individual web-based work. The exercises we implemented include the typical spectrum from form-focused fill-in-the-blank (FIB) activities to more open formats, such as reading and listening comprehension questions requiring full-sentence answers.
The basic FeedBook system functionality described in Rudzewitz, Ziai, De Kuthy, and Meurers (Reference Rudzewitz, Ziai, De Kuthy and Meurers2017) was piloted in the school year 2017–2018. It included a student interface as well as a teacher interface that allows the teacher to manually provide feedback to students with the help of some system support, such as a feedback memory recognizing recurring student responses and inserting the feedback that was given before (inspired by translation memories supporting human translators). Developing the system took substantially longer than originally planned. Students, parents, and teachers essentially expect technology used in daily life to work on all types of devices, including mobile phones and tablets, all operating systems, and all browser types and versions, and to provide the functions they are used to (though we ultimately convinced the students that adding a feature to invite your friends was not as essential as they thought). We then turned the FeedBook from a web-based exercise book into an ITS by adding an automatic, interactive form feedback (Rudzewitz et al., Reference Rudzewitz, Ziai, De Kuthy, Möller, Nuxoll and Meurers2018). While our original motivation for the FeedBook project was the decade of research we spent on the automatic meaning assessment of short-answer activities in the CoMiC project (http://purl.org/comic), the real-life focus of homework assignments in workbooks in our experience clearly is on practicing forms, even when the textbook itself is TBLT-inspired. To satisfy this demand, the FeedBook system started out providing traditional focus on forms, in the technical sense of this term in SLA (Ellis, Reference Ellis2016).
However, the fact that an ITS such as the FeedBook supports immediate learner interaction provides the opportunity to build on feedback research arguing for the effectiveness of scaffolded feedback (Finn & Metcalfe, Reference Finn and Metcalfe2010). While in sociocultural SLA research, corrective feedback is conceptualized as a form of scaffolded interaction in the zone of proximal development (ZPD, Aljaafreh & Lantolf, Reference Aljaafreh and Lantolf1994), Bitchener and Storch (Reference Bitchener and Storch2016, p. 93) pointed out that almost all of this research is based on oral dialogue. The immediate, fully controlled interactivity supported by an ITS thus provides an interesting opportunity to explore the effectiveness of systematic scaffolded feedback in the written mode (complementing research on the written mode in synchronous computer-mediated communication; cf. Ziegler & Mackey, Reference Ziegler and Mackey2017). Given the good fit, we designed the FeedBook to incrementally provide cues in order to scaffold the use of forms that the learner could not yet handle entirely on their own for successful completion of an exercise. Since variables at all the different levels of granularity play a role in real-life language learning, it is unproductive to maintain a divide between sociocultural and cognitive-interactionist perspectives on language learning. Opting to conceptualize the interaction offered by an ITS as scaffolded feedback in the learner's ZPD is cognitively well-grounded (Finn & Metcalfe, Reference Finn and Metcalfe2010) and, as far as we can see, compatible with our plans to later investigate the impact of a range of individual difference measures.
In the next step, we expanded the automatic feedback in the FeedBook to also provide feedback on meaning, as needed for meaning-based reading/listening comprehension exercises (Ziai, Rudzewitz, De Kuthy, Nuxoll, & Meurers, Reference Rudzewitz, Ziai, De Kuthy, Möller, Nuxoll and Meurers2018). Including such meaning-based activities in the FeedBook also provides opportunities for the system to give (incidental) focus on form feedback (Ellis, Reference Ellis2016). In the current system, meaning feedback is always prioritized over form feedback, though in the future we plan to individually prioritize feedback for a given learner and task using machine learning based on information from learner and task models and learning analytics.
In contrast to traditional computer-assisted language learning (CALL) systems, the FeedBook does not require explicit encoding of different answer options and linkage to the feedback. As transparently illustrated by Nagata (Reference Nagata2009), manual encoding would not be feasible for many exercise types where the potential paraphrases and error types quickly combinatorially explode into thousands of learner responses that the system needs to be able to respond to. In line with the intelligent CALL (ICALL; Heift & Schulze, Reference Heift and Schulze2007) perspective, we therefore employ computational linguistic methods to characterize the space of possible language realizations and link them to parameterized feedback templates. Different from typical ICALL approaches generalizing the language analysis away from the task properties and learner characteristics, for the reasons depicted in Meurers and Dickinson (Reference Meurers and Dickinson2017), we would argue that valid analysis and interpretation of learner language requires task and learner characteristics. This is reflected by the FeedBook in two ways: First, two active English teachers with experience teaching seventh-grade students in this school form were hired on secondment as part of the project, one after the other, to ensure a firm link to the real-life teaching context. This includes the formulation of 188 types of feedback messages designed to express the scaffolding hints that teachers would give students on the language targeted by the seventh-grade curriculum. In addition to the learner characteristics implicitly encoded in the exercise materials and feedback templates, an inspectable learner model was developed to record individual competency facets. Second, the exercise properties are directly taken into account by the computational modeling of the well-formed and ill-formed variability of the learner language. The approach in Rudzewitz et al. (Reference Rudzewitz, Ziai, De Kuthy, Möller, Nuxoll and Meurers2018) derives the structure of the answer space that the system can respond to, which is based on the target hypotheses provided by the publisher in the teacher version of the workbook, combined with a flexible online matching mechanism.
More discussion of the technical side of the FeedBook development can be found in Rudzewitz et al. (Reference Rudzewitz, Ziai, De Kuthy and Meurers2017) and Ziai et al. (Reference Ziai, Rudzewitz, De Kuthy, Nuxoll and Meurers2018). We focus here on the conceptual side of the system and its use as an experimental platform, which we can parametrize in different ways to study the effect on language learning of school children in their regular formal education setting. The curriculum and the design of the FeedBook as a tool interactively supporting individual homework that prepares the student for the classroom sessions delineates the type of research questions that can be explored on this platform. Considering the breadth of research perspectives ISLA is engaged with (Loewen & Sato, Reference Loewen and Sato2017), this naturally only covers a small part of that spectrum—but this subspectrum arguably still includes a substantial number of research issues that such a platform can help settle in an empirically rich way. This includes the effectiveness of different types of feedback in different types of exercises, the reality and impact of developmental sequences and teachability (Pienemann, Reference Pienemann2012) on what can be taught to learners at what point, precise parametrization of exercise and task complexity including alignment with learner proficiency characteristics supporting adaptive individual differentiation, the impact of input materials differing in linguistic complexity and input enhancement in reading comprehension, or the role of individual learner differences and aptitude-treatment interactions, including measures of cognitive ability, motivation, self-regulation, and social characteristics of the students and their families—a broad range of issues at the heart of individual differences in ISLA and classroom research (Li, Reference Li, Loewen and Sato2017; Mackey, Reference Mackey, Loewen and Sato2017).
To support research into such issues, it is not just the intervention that we must be able to scale up to hundreds (or, ultimately, thousands) of students, but also the collection of the relevant variables needed to address the research questions. We therefore started integrating web-based versions of questionnaires covering a range of learner variables and adapted web-based versions of two cognitive/aptitude tests based on the web-based versions for adults of Ruiz Hernández (Reference Ruiz Hernández2018), the MLAT5 and a version of the OSpan using Klingon characters, making it harder to cheat through note taking in the uncontrolled, web-based scenario (Hicks, Foster, & Engle, Reference Hicks, Foster and Engle2016).
FeedBook as a Platform for Studying Feedback
As the first study using the FeedBook, we are investigating the effectiveness of immediate formative feedback incrementally scaffolding the completion of homework, embedded in a regular school context. We chose this relatively traditional topic since it is well motivated by the challenges teachers and students face in real-life classroom, and there is a rich discussion of this topic in SLA pointing out the need for more systematic research, discussed below. Teachers typically are the only reliable source of feedback for foreign language students in secondary school, but their time and the time teachers and students spend together in class is very limited. So, there is little opportunity for students to obtain individual formative feedback, even though the substantial individual differences in aptitude and proficiency would make individual feedback particularly valuable. When students are systematically supported in homework exercises at their individual level, these exercises may also function as pretask activities allowing more students to actively participate in joint language tasks later in the classroom.
Throughout education, feedback is established as an important factor supporting learning, especially where it helps overcome insufficient or false hypotheses (Hattie & Timperley, Reference Hattie and Timperley2007). In its summary of evidence-based research on education, the Education Endowment Foundation includes feedback as the strongest factor influencing learning overall.Footnote 2 In SLA there is a long tradition of research and interest in feedback, for which the edited volume of Nassaji and Kartchava (Reference Nassaji and Kartchava2017) provides a current overview. They highlighted the need for further investigations and also mentioned the role that technology could play (p. 181). Sheen (Reference Sheen2011, p. 108) pointed out that empirical studies were often limited to corrective feedback on few linguistic features, limiting the generalizability. In a similar vein, Russell and Spada (Reference Russell, Spada, Norris and Ortega2006, p. 156) concluded their meta study on the effectiveness of corrective feedback for the acquisition of L2 grammar, stating that more studies investigating “similar variables in a consistent manner” were needed. Ferris (Reference Ferris2004) concluded years of debate started by Truscott (Reference Truscott1996) with a call for more systematic studies: “Though it may be difficult for the ethical and methodological reasons I have already described, we need to think of ways to carry out longitudinal, carefully designed, replicable studies that compare the writing of students receiving error feedback with that of students who receive none, as well as comparing and controlling for other aspects of error treatment” (Ferris, Reference Ferris2004, p. 60). Sheen (Reference Sheen2011) emphasized the broad practical and conceptual relevance and complex nature of the topic:
It also highlights the importance of examining CF [corrective feedback] in relation to language pedagogy (e.g., Ferris, Reference Ferris2010; Lyster & Saito, Reference Lyster and Saito2010), given that corrective feedback is one aspect of language teaching that teachers have to deal with on a daily basis. To repeat an earlier statement—CF is a highly complex phenomenon. To understand [it,] it is necessary to take into account multiple factors including feedback type, error type, interaction type, mode (oral/written/computer-mediated), the L2 instructional context, the learner's age, gender, proficiency, first language (L1), anxiety, literacy and cognitive abilities, and how the learner orientates to the correction. In short, the study of CF constitutes an arena for studying the issues that figure in SLA—and in language pedagogy—more broadly. (Sheen, Reference Sheen2011, pp. 174–175)
Linking these issues to the role of computer-generated feedback on language learning, Heift and Hegelheimer (Reference Heift, Hegelheimer, Nassaji and Kartchava2017, p. 62) discussed studies in tutorial CALL and concluded that the “key in the future development of computer-generated feedback is to equip the tools with mechanisms that allow for research of vast and reliable user data.” In sum, there is a rich landscape well worth exploring, both to investigate parameters and their interaction from the perspective of SLA research and to effectively support real-life teaching and learning. A software platform such as the FeedBook arguably can help research some of these issues by supporting systematic studies of different types of feedback in a range of exercises fully integrated in real-life school. The study in this article on scaffolded feedback supporting focus on forms to students working on their regular homework provides a first illustration of this, with the envisaged integration of individual difference measures and the functionality for (incidental) focus on form illustrating relevant and realistic next steps.
Let us illustrate the meta-linguistic cue feedback that the system currently provides to incrementally scaffold the student's work on homework exercises. All exercises are web-based versions of the workbook exercises of the Camden Town textbook made available by the publisher. Figure 1 shows a typical, minimally contextualized FIB activity targeting the formation of simple past forms. We see that the first gaps were already completed and automatically marked as correct by the system, indicated by a check mark and coloring the answer in green. Now, the student entered tryed, and immediately after leaving the gap, the system presented the message seen in the image, puts an x next to the student answer and colors it in red.

Figure 1. Feedback on simple past formation in a fill-in-the-blank exercise.
The meta-linguistic cue given as feedback points out the subregularity that needs to be understood to produce the correct word form. The term simple past, shown in light blue, is a link to the so-called Language in Focus (LiF) explanatory texts from the book, which we also included in the FeedBook. As described in Ziai et al. (Reference Ziai, Rudzewitz, De Kuthy, Nuxoll and Meurers2018), the space of possible learner responses and how they link to the parameterized feedback templates written by the English teachers on our project is automatically generated based on the solution for the exercises from the teacher workbook and computational linguistic models of the language and the learners covering the seventh-grade curriculum. So, the FeedBook feedback immediately becomes available for any new seventh-grade exercise entered into the system without additional encoding effort.
Figure 2 shows a more functionally motivated exercise designed to practice comparatives. The student is supposed to produce sentences comparing two airlines flying to Greece based on the information and the language material provided. In the screen shot, the student wrote, “Tickets at Air Con are expensiver than at Midair,” and as the cursor left the field, the system colored the learner answer in red and displayed the feedback explaining that adjectives with three or more syllables form the comparative with “more.” If the student cannot link this feedback to the sentence she wrote, a click on the little magnifying glass below the feedback message displays the sentence with the word “expensiver” highlighted.

Figure 2. Feedback on comparatives in a more contextualized activity.
A student can work on the exercise step by step, any number of times, with the system always showing a single feedback message at a time, or accepting the response. The system never shows the answer, and students naturally can submit answers still containing errors to the teacher. We are considering adding an option, to limit frustration, to peek at the answer after a number of attempts, though it is nontrivial to distinguish serious attempts from gaming the system.
For exercises such as the reading comprehension activity shown in Figure 3, the system prioritizes meaning feedback over incidental focus on form feedback (Long & Robinson, Reference Long, Robinson, Doughty and Williams1998). The answer given in the example here was grammatically correct, but it does not sufficiently answer the question. The system detects this using an alignment-based content assessment approach (Meurers, Ziai, Ott, & Bailey, Reference Meurers, Ziai, Ott and Bailey2011) and responds that important information is missing. At the same time, light green highlighting appears in the text to indicate the passage in the text that contains the relevant information, in the example at the beginning of the text. The system thus scaffolds the search for the relevant information. A click on the magnifying glass for such cases narrows down the highlighting to further zoom in on the key information.

Figure 3. Feedback on meaning in a reading comprehension activity.
Having illustrated the FeedBook system and the type of feedback and incremental work on exercises that it supports, we can turn to evaluating whether receiving such scaffolded feedback indeed fosters learning of the targeted grammatical concepts.
Study
In this first RCT using the FeedBook, we seek to answer the question:
RQ Does immediate scaffolded feedback on form given as part of the homework accompanying regular English classes improve learning of the L2 grammar?
Considering what constitutes a meaningful baseline against which the improvement is measured, an experiment comparing the effect of the feedback in a web-based environment against a baseline performance using the printed workbook would not be sufficiently focused on the research question investigating feedback. This is because media and novelty effects are likely to interfere where children use new digital tools and possibly new hardware. All students in this study therefore get to use the FeedBook, and we employ within-class randomization to distinguish the intervention from control students by parameterizing the feedback provided by the system as described below.
While the research question focuses on the impact of immediate scaffolded feedback on form, the study is also intended as a stepping stone, establishing a parameterizable platform to conduct large-scale interventions in an authentic school context to address a range of ISLA questions in the future.
Methods
Participants
We recruited 14 seventh-grade classes in four German high schools (Gymnasium), academic-track schools, grades 5 to 12. English is taught as the first foreign language from Year 5, with students generally having some initial exposure to English in primary school. All of the schools in the study regularly use the Camden Town textbook, one of the textbook series approved in the state of Baden-Württemberg. The schools and classes are quite varied in a number of other dimensions. Three of the schools are public, and one is a private school. One of the schools is designated as a science-focused school, another as an arts- and sports-focused school. Three of the classes are content and language integrated learning (CLIL) classes, one from Grade 5 on and the other two from Grade 7, receiving 2 additional hours of English per week. The average class size is 25 students, ranging from 15 to 31. The schools are coeducational, but one of the classes is all boys. Several of the classes are taught by trainee teachers for half of the year or the entire year. Two of the classes are tablet classes, where students are provided with a tablet computer and bring it to school on a daily basis. Overall, there is a real-life mix of characteristics, as would be expected of a study emphasizing the importance of conducting research in ecologically valid settings.
Two of the 14 teachers opted out of participating in the study due to work overload, and one dropped out after parents spoke out against participation. Technical problems with the wireless network in one class made it impossible to collect valid pre- and posttest data, eliminating that class for the study presented here. The study thus is based on 10 classes for which we obtained questionnaires, pretest, and posttest data.
In addition to the within-class randomization, we recruited two complete business-as-usual control classes in a fifth school as an additional reference. However, for such classes it turned out to be very difficult to motivate the teachers to reserve slots for testing. They stopped participating in the testing during the school year, though we hope they will still complete the tests at the very end of the year to support an overall comparison of general proficiency development, in addition to the construction-specific analysis of development that the within-class randomization provides.
In the 10 classes included in our analysis, there are 255 students, who were randomly assigned to groups A and B. Of those students, 222 consented to taking part in the study and were present in class to complete both the pre- and posttest. Of those students, 17 (7.66%) turned in tests that contained a substantial number of nonsense test answers (eight or more answers consisted of nonsense or swear words, random letter sequences, or pasted copies of web pages), so we removed the data from those students from the analysis, which left us with 205 students for the analysis presented here. Of those, 104 are in group A, which for the study presented here is the intervention group, and 101 in group B, the control group. Regarding gender, 116 of the students are male, 84 are female, and five did not provide the information. The average age of these seventh graders at the middle of the school year is 13.09 years (SD = 0.49), with six students not their reporting age.
Design
We first needed to determine how to do the random assignment. Randomizing at the class level, with different classes being taught by different teachers, is problematic since a substantial amount of variance in student achievement gains can result from variation in teacher effectiveness (Nye, Konstantopoulos, & Hedges, Reference Nye, Konstantopoulos and Hedges2004, p. 253). We would thus need a high number of classes to statistically factor out the differences between the teachers in the control versus those in the intervention classes. Fortunately, the use of a web-based tool designed to support individual interactive practice outside of class readily lends itself to another type of randomization avoiding this problem: within-class randomization.
Since we wanted to fully integrate the study into authentic classrooms for the entire school year, a design randomly assigning each child to either the control or the intervention group would be problematic in terms of being perceived as disadvantaging the students in the control group. That perception would likely have doomed the required parental approval (even though the point of the study is to test whether the intervention is effective at all). Since the English curriculum is explicitly regulated and the teaching and timing of material during the year in the real-life setting is up to the teacher, a waiting control group or counterbalancing repeated measures design also cannot be put into practice.
We decided on a type of rotation design, in which students are randomly assigned to groups A and B. The school year is split into the four textbook themes typically covered by the English classes. For the first theme, group A is the intervention, and B the control group. For the next theme, this is reversed, with group B becoming intervention, and group A the control. The same happens for the next two themes. Before and after each theme, we carried out pre- and posttests of the grammar topics primarily focused by that theme. The overall study design, including the questionnaire and ID tests, is summed up in Figure 4.

Figure 4. Overall design showing rotation of control and intervention during the school year.
The entire intervention, including all testing and questionnaires, is web-based, requiring only a web browser connected to the internet. To ensure high quality of the testing, for this first study we decided to conduct the tests in person in the schools using the computer classrooms there or laptops/tablets where available.
At the beginning of the school year, seventh-grade teachers often are newly assigned. The students started to use the FeedBook for the first time, and despite a year of piloting, the program still contained bugs that we ironed out while Theme 1 was being taught, including one allowing all students to access all feedback while submitting their homework to the teacher. This was an unintended crossover effect during Theme 1, but it worked well for students to get to know the system and its functionality.
For the analyses presented in this article, we focus on Theme 2, when the school year was humming along and the system mostly worked as intended. Specifically, we studied how well the grammar constructions that were focused on in Theme 2 (conditionals, comparatives, and relative clauses) were learned by comparing the student's pretest results for those constructions before Theme 2 was covered in class and homework with the posttest results for them afterwards. The group A students constituted the intervention group for this theme, and they received specific scaffolded feedback for its grammar focus, as described in the “Materials” section below.
Given that we wanted to interfere as little as possible with the regular teaching, while proceeding through the themes in class, the teachers assigned exercises in the FeedBook whenever they normally would have assigned exercises from the printed workbook, typically as homework to practice material introduced or revisited face to face in class. While the teachers were free to assign homework as they like, we asked them to include a small number of core exercises to ensure that there was some opportunity to practice the grammar topics of the theme. For example, for Theme 2, we asked them to include eight homework exercises (i.e., eight individual ones, not entire exercise sheets).
In within-class randomization, spillover effects can occur, where students in the intervention group convey information to those in the control group. Some students possibly did their homework together or discussed it, which is par for the course in an authentic school setting. One part where spillover became relevant was during the tests, for which all students were in the same classroom. It would have been impractical to divide the classes into two groups and test them separately, and it would have made the group distinction explicit to the students. We instead followed the common school practice of placing cardboard screens between the students to reduce spillover at test time (i.e., cheating.)
Materials
The intervention was carried out with the FeedBook, and the system was parameterized differently for the intervention and control groups so that specific feedback messages on selected grammar topics could only be seen by one of the groups. As introduced in the “Addressing the Challenge” section, each theme of the book targets general competencies, and the exercises in the workbook for that theme require a range of language aspects to complete them, not just the particular grammar aspect that the specific scaffolding feedback and the pre- and posttest for that theme focus on. For example, writing down a sentence naturally always involves making decisions about word order, agreement, tense, or aspect. To ensure a continuous interactive experience when using the system and to keep students motivated throughout the year, only the feedback specific to the particular target constructions of a given theme was switched off for the control group. Everyone always received meaning and orthography feedback, as well as default feedback, which was triggered when the learner response differs in a way outside the space of pedagogically envisaged type of answers modeled by the system. The system then responded with “This is not what I am expecting. Please try again.” Every student also received positive feedback in the form of a green check mark and coloring of the answer when giving a correct answer. There was one exception: For two narrowly focused exercises, the situation was so binary that we also switched off the positive feedback; that is, the control group received no positive or specific grammar feedback in those two exercises. In sum, as we will exemplify below, the vast majority of system feedback was given to both groups of students—the experience of the intervention and control students only differed with respect to the particular grammatical focus constructions of a theme.
As the pre- and posttest for the grammar constructions focused on a theme, the same test was used. It consisted of subtasks for each targeted grammar topic: one with true/false items, and the other with FIB items. We also considered a third item type with open-format, full-sentence answers, but found that the school students completed these in a highly variable way that made it difficult to score them in terms of their language competence for the targeted forms. The test format and most of the items were piloted in school by one of the teachers on our project. The test we used as the pre- and posttest for Theme 2 consists of 40 items in total, which are available from the IRIS repository.Footnote 3
In addition to the construction-specific grammar test, at the beginning of the school year we administered a C-Test as a general proficiency indicator, “one of the most efficient language testing instruments in terms of the ratio between resources invested and measurement accuracy obtained” (Dörnyei & Katona, Reference Dörnyei and Katona1992, p. 203). After Theme 2, the students also completed a questionnaire with sections providing general information about themselves, parental involvement in school and homework, and computer experience. In addition, the questionnaire contained items from a questionnaire successfully used in large-scale school studies in Germany (Gaspard, Häfner, Parrisius, Trautwein, & Nagengast, Reference Gaspard, Häfner, Parrisius, Trautwein and Nagengast2017) to measure student's expectancy (academic self-concept), intrinsic values, attainment values (personal importance, importance of achievement), utility values (such as utility of English for general life, job, and school), and effort and emotional cost. To obtain more individual difference measures, in a later part of the study we will also be asking the students to complete web-based versions of the MLAT5 and of the OSpan using Klingon characters (Hicks et al., Reference Hicks, Foster and Engle2016) to obtain the information needed to later address research questions targeting cognitive individual differences and aptitude-treatment effects. The goal is to provide a broad link to SLA research emphasizing the range of factors at stake (e.g., Mackey, Reference Mackey2012; Nassaji & Kartchava, Reference Nassaji and Kartchava2017).
Procedure
The pretest was administered in each class whenever the teacher signaled that they were starting with Theme 2, and the posttest when the teacher told us they were starting with the next theme. To motivate the teachers to let us know without delay, the different themes in the FeedBook were only unlocked for the students after the tests had been conducted. The time the teachers took to cover Theme 2 varied between 56 and 67 days (M = 63.2, SD = 3.71), including two weeks of Christmas school holidays. No form of feedback on the test performance was provided to the students or the teacher. The pre- and the posttest were scored automatically by comparing the learner response against the correct answer. Where responses were typed, we manually reviewed the different types of answers given and extended the correct answer keys with orthographic variants of the correct grammatical form.
An important reason for using a web-based system to scale up interventions to the authentic school setting is that the system can provide detailed logs of the individual learning process, so we can inspect what actually happened during the intervention. Despite the dramatic loss of control, focus, and compliance issues that scaling up to the field entails, the availability of the logs detailing the individual interaction and learning process supports detailed analyses of learning in an authentic setting. Based on this log, we can make the description of the intervention procedure more concrete. We mentioned the core tasks we asked the teachers to integrate into their routine, without knowing whether they assigned the tasks or which students actually did the homework—the typical kind of loss of control resulting from scaling up. In the log, we can see which tasks the students actually worked on and whether they saw feedback.
Figure 5 shows the exercises the students worked on between the pre- and the posttest for Theme 2 on the x-axis and the number of feedback messages they saw while working on that exercises on the y-axis. We see that the students primarily worked on the eight core exercises (B3, B6, ET2, ET5, CYP2, AP9, AP12, AP26), which are shown with red italics labels composed of the book section (A, B, C, Extra Training, Check Your Progress, Additional Practice) plus the exercise number, and there are three more exercises recording more than 500 practice steps with feedback (A3, B1, B2). The figure shows that students in both groups received a substantial amount of feedback, in line with the goal of providing a responsive, interacting system.

Figure 5. Overall number of feedback messages shown per exercise in Theme 2.
For our research question, the most interesting issue was who saw what kind of feedback. Figure 6 distinguishes feedback on (a) the grammar focus of Theme 1 (tenses, progressive aspect, gerunds); (b) the grammar focus of Theme 2; (c) the grammar focus of Theme 3; (d) other, that is, grammar not focused by a theme (e.g., selection of bare or to-infinitive); (e) orthography; (f) meaning feedback; and (g) cases where default feedback was generated, including (h) check marks indicating correct answers. On the left, we see the number of practice steps where feedback was shown and on the right the cases where it was hidden by the system (i.e., internally generated but not shown to the student). We clearly see that for orthographic, meaning, and default feedback, learners in both groups received a substantial and comparable amount of feedback. For the feedback messages targeting the grammar focus of Theme 2, the Theme 2 intervention group saw the feedback (solid red bar for Grammar 2 on the Shown side of the figure), whereas the control group did not (solid blue bar for Grammar 2 on the Hidden side). Interestingly, the right side of the figure shows that the number of interactions steps for which feedback on the grammar construction was hidden is higher than where it was shown, indicating that students kept trying until they were shown the green check mark confirming that they got it right. When not receiving the specific feedback, this apparently required more attempts. Orthography, meaning, and default feedback was shown to both groups. Learners in both groups also saw the vast majority of the positive feedback (green check marks). As shown on the far right, some positive feedback was hidden from the control group in two exercises (ET5, CYP5) that were so binary in the choices that specific grammar feedback and correct feedback amounted to two sides of the same coin.
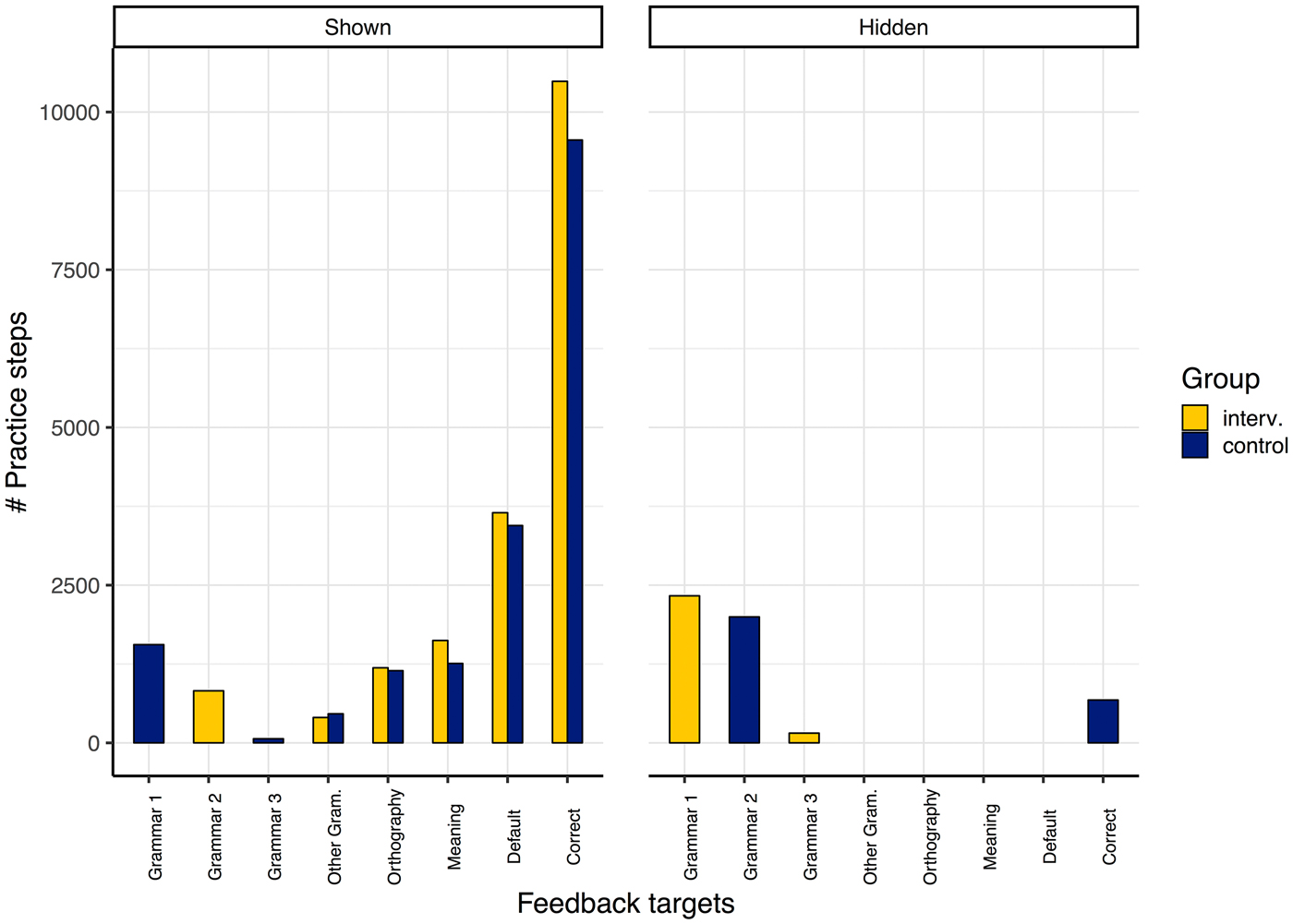
Figure 6. Number of practice steps by feedback targets and who saw which feedback.
To interpret the Grammar 1 and Grammar 3 columns, we need to remember that homework exercises in Theme 2 involved all kinds of linguistic material, not just the specific constructions that are the particular grammar focus of that theme. The blue bar for Grammar 1 in the Shown feedback portion of the graph on the left indicates that the control group for the grammar focus of Theme 2 was the intervention group for the grammar focus of Theme 1; so they continued to receive feedback on those grammar constructions. The red bar for Grammar 1 feedback in the Hidden portion of the figure on the right shows that the Theme 2 intervention group still did not receive feedback on the grammar focus of Theme 1. The same holds for the grammar constructions that would be the grammar focus of Theme 3; where those constructions already occurred in exercises in Theme 2, students in the Theme 2 intervention group did not see the specific grammar feedback for those constructions, as they would be the control group in Theme 3.
While there is no space here to further analyze the log of student interactions with the system, the detailed, stepwise records of language learning in the wild arguably can provide important information for a wide range of research questions, with learning analytics and educational data mining as emerging fields providing relevant methods (Lang, Siemens, Wise, & Gašević, Reference Lang, Siemens, Wise and Gašević2017). As far as we can see, however, these will only become potent when combined with SLA models of learners, tasks, and feedback, and with operationalizations of SLA concepts such as uptake or emergence criteria (Pallotti, Reference Pallotti2007) supporting valid interpretations of the information available in such logs.
Results and Discussion
To address our research question about the effectiveness of scaffolded grammar feedback, we started by taking a look at the mean scores of the intervention and control groups at pretest and posttest. They are visualized in Figure 7, with the whiskers showing the 95% confidence intervals (CI) around the means. All analyses were conducted in the statistical computing language R, version 3.5.1 (The R Foundation for Statistical Computing, 2018).

Figure 7. Comparison of mean scores for pre- and posttest (with 95% CI whiskers).
The students of both groups performed comparably on the pretest, with a slightly lower score for the intervention group (M = 24.92, SD = 5.63) than the control group (M = 25.76, SD = 5.41), which did not amount to a significant difference (Welch t test: t(202.98) = −1.0881, p = 0.28). On the posttest, both groups had improved, with students in the intervention group outperforming the students in the control group. To test this explicitly, the difference between the posttest and the pretest results of a given student was computed: that is, the change score. A Welch t test was conducted to compare the change score in intervention and control condition, which showed a significant difference in the scores for intervention (M = 7.82, SD = 5.4) and control (M = 4.81, SD = 5.24); t(203) = 4.04, p < 0.0001). The learners who received the specific scaffolded grammar feedback thus learned 62% more than those who did not. Cohen's d = 0.56, indicating a medium-size effect.
Bringing the impact of the pretest score into the picture, Figure 8 visualizes the difference between the intervention and control groups when predicting the change score based on the pretest score.

Figure 8. Linear regression line for pretest score and group predicting change score (including 95% CIs).
In addition to the higher change scores for the intervention group already numerically established above, the increase was higher for students with a lower pretest. The difference between intervention and control group was almost eliminated for very high pretest scores, which could be an aptitude-treatment interaction or a ceiling effect given that the maximally achievable score on pretest and posttest was 40.
The results for the linear regression with the dummy coded group variable shown in Table 1 confirms the significance of the difference for both predictors. The adjusted R 2 of the model is 0.42. An interaction between group and pretest added to the model falls short of significance (p = 0.086).
Table 1. Linear regression model predicting change score based on group and pretest score

One aspect we have ignored so far is that students are part of classes, and teacher effectiveness may differ from class to class. To capture this, we can move to mixed-effect models and introduce the class as a random intercept, using the R package lme4 (Bates, Maechler, & Bolker, Reference Bates, Maechler and Bolker2012). At the same time, we can also address a concern that the linear regression setup predicting change score is based on a lot of aggregation that essentially loses information. The change score reduces the pretest and posttest behavior to a single value representing the difference between the sum of correct answers. When moving to mixed effects in order to determine and factor out the variance that is due to students being in different classes, we can move to mixed-effects logistic regression to directly model the student responses on the tests without aggregation. Instead of predicting the change score, in the mixed-effects logistic regression we can model the log odds of the binary outcome for each test item, that is, whether a gap in the test is filled in correctly or not. Table 2 shows the summary for three models illustrating relevant characteristics.
Table 2. Mixed effects logistic regression models with test type and group as fixed effects, and random intercepts for items, learners, and teachers. Model 2 adds gender, and Model 3 adds the last English grade.

***p < 0.001, **p < 0.01, *p < 0.05
Model 1 includes a fixed effect for the test kind (pretest/posttest) and the group (control/intervention), the interaction between the two, and three random intercepts to account for the variability across the test items, the learners, and the teachers (= classes). The Intercept here represents the pretest control case. The posttest significantly increases this, adding 0.68 (SE = 0.05). The intervention group pretest is not significantly different from the control group pretest (−0.07), confirming the t test we started the section with. The interaction between posttest and intervention shows that the posttest results for the intervention group were significantly higher (0.43, SD = 0.08), so the intervention led to better posttest results.
At the bottom of the table, we see the variance captured by the random intercepts. Little variability is allotted to the teacher (0.05); that is, the performance of students in our study is thus not influenced much by general performance differences between teachers (= classes). The items on the test differ much more substantially (0.52), but understanding test item difficulty is not a primary interest here. On the other hand, the substantial variability between learners (0.30) is of interest in that it raises the question whether there are learner properties we could make explicit to explain some of that variance. To illustrate this, in Model 2 we add the gender of the student from the questionnaire data as a predictor, that is, an additional fixed effect. The student number drops by five students who did not report that information. We see that the girls have significantly higher scores (0.34, SE = 0.09). Adding this predictor reduces the variability captured by the random intercept for Learner from 0.30 to 0.26. Model 3 adds last year's English grade as provided by the student in the questionnaire, which six more students did not report. In the German grading scale, 1 is the best grade and 6 is the worst. The grade as a fixed effect is a significant predictor, further reducing the remaining variance left to the Learner random intercept to 0.21.
Summing up the results, both an analysis based on change scores and a more fine-grained mixed-effects logistic regression allow us to answer the research question in the affirmative. From an ISLA research perspective, the study confirms that scaffolded written feedback on forms is an effective intervention method. Providing secondary school students with immediate scaffolded feedback on grammar while they work on their homework significantly improves their mastery of those grammar aspects. This effect is visible even for students fully embedded in their authentic, varied school environment, with all the teaching and learning otherwise going on there—and it shows up despite the fact that the system provides students in both the control and intervention groups with substantial feedback. It seems to be the availability of specific, scaffolded grammar feedback that results in the significant difference, with children learning 62% more.
Limitations of the Study
Given the rotation design, where both groups benefit from feedback on different constructions throughout the school year, it is not meaningful to measure global differences in overall English proficiency. We plan to conduct such a comparison with the two business-as-usual control classes at the end of the school year. The result of that comparison will only be indicative, though, since we did not randomly select the two control classes. By moving all testing to an individual, web-based setup, reducing the burden of testing for the teacher, and by offering options for switching between printed and web-based workbook during the school year, we envisage more options becoming realistic.
The analysis presented here also does not make full use of the overall rotation design covering the full year since we only analyzed the learning gains for the grammatical constructions targeted in Theme 2. Once we have finished collecting and analyzing the data for Theme 3, where the control group of Theme 2 becomes the intervention group, it will be possible to compare learning gains for students in both groups across different grammar topics. At the same time, the analysis of the Theme 2 data we presented as it stands does address the research question we set out to investigate. All students used the system, with within-class randomization determining who saw the scaffolded feedback for which grammar topic, and the other system behavior being comparable for all students—so the results are unlikely to be due to a novelty effect. Importantly, the differences in learning gains between the two groups for the selected grammatical constructions arise in the authentic school contexts, with different teachers teaching as usual in different types of schools, including regular, bilingual, and CLIL classes—with the intervention results arising regardless of these substantial differences.
Using Learner Data for Task Analysis
While we have so far focused on the learners, we have also mentioned that the valid interpretation of learner data also requires taking the task into account. When we aggregate the information that is collected by the FeedBook about the learner interactions with a given task and relate this to the individual learner models, we can study the aptitude-treatment interaction. For practice, we can inspect whether a given task is at the right level for a given group of learners—whether it, for example, is too hard or too easy for a workbook for seventh grade. If we aim for exercises that are in the ZPD of a given set of learners, then some form of scaffolding would generally be needed to successfully tackle it. Indeed, this can be empirically verified, and the FeedBook system includes an interface to do so. In Figure 9 we see an exercise where image and language input is provided to elicit past progressive sentences. To complete the exercise, three sentences must be entered. The task performance view below the exercise shows a snapshot of the database, in which 267 students completed the exercise, with the number of interactions with the system on the x-axis and the green/red graph indicating the number of successful/erroneous responses on the y-axis. We can see that of the students who interacted three times, 17 were successful, and 27 were not. When students interacted with the system more than three times before submitting the exercise, they more often than not managed to complete the exercise correctly, as indicated by the lighter green line mostly being above the darker red one to the right of three attempts. In sum, this seems to be an exercise that is appropriately difficult for our students to tackle in that it takes them some effort to work through it, but they are able to ultimately deal with it successfully given the system feedback. Needless to say, in the exercises we encoded from the printed workbook, there are some where the instructions, the input, and the linguistic or task complexity make them too hard for most students in our seventh-grade group to tackle successfully.

Figure 9. FeedBook interface showing performance on a given exercise.
While visible in the just presented FeedBook task view, this typically also shows up as substantial variability of the learner responses that is unrelated to the intended learning goal, which can be inspected using another interface in the FeedBook. Analyses such as the ones provided by the FeedBook interface and analyses based on such system logs (e.g., Quixal & Meurers, Reference Quixal and Meurers2016), as far as we can see, can play a useful role in relating tasks and learners. For practice, it can help improve the exercise materials for a given student population. On the research side, it should make it possible to develop empirically rich and sufficiently explicit models of exercise and task complexity and connect them to authentic models of learner aptitude and proficiency, ultimately supporting the selection or generation of individually adaptive materials and dynamic difficulty adjustment.
Conclusion and Outlook
In this article, we explored how an RCT can be set up to investigate the effectiveness of individual scaffolded feedback on grammar in an authentic school context. We took a typical seventh-grade English class setup in Germany and integrated an intelligent workbook in place of the traditional printed one. This establishes a space for individualized, interactive learning that can fully scale to authentic school contexts. While this is an ecologically valid context we have virtually no control over, fortunately the workbook platform makes it possible to individually tailor and log the interaction of the school children doing their homework. We showed that this setup makes it possible to answer our research question in the affirmative: Individual corrective grammar feedback is effective in this context. We hope that conducting experiments in the field in this way will help validate results established in the lab and strengthen the impact of those results on real life, where it can again be fed back to provide data for empirically broader models of instructed SLA.
This article focused on establishing and illustrating a research perspective using a computational platform to support the scaling up of feedback research in a way that is fully integrated in real-life school teaching. As such, there unfortunately is not enough space in this article to provide a more extended discussion of current research strands on corrective feedback, its theoretical foundation, and the role of corrective feedback research and individual differences in instructed SLA. Presenting the first results of a field study confirming the effectiveness of scaffolded feedback on grammar, our article does provide a fully worked-out link between SLA research on feedback as motivated in the introduction, on the one hand, and interventions of practical relevance for real-life learning, on the other. We hope this will help ground the public and political discussion of the digitization of education in actual evidence linked to SLA research. On the research side, it can open the door to focused studies targeting current questions in feedback research. Note that individual feedback delivered through the FeedBook platform could also be combined with in-class interventions. For example, in a study also highlighting the value of seamlessly deploying interventions in genuine classroom contexts, Sato and Loewen (Reference Sato, Loewen, Keyser and Botana2019) provided meta-cognitive instruction to students about the benefits of receiving corrective feedback and show that this indeed helps learners benefit from corrective feedback. Such an in-class instruction component could readily be combined with the FeedBook platform delivering individual feedback to learners working on homework—which substantially reduces the work required to carry out such a study. As the feedback provided by the system is individually delivered, it can also be individually tailored to take into account individual differences, for example, providing more explicit, meta-linguistic feedback for students with higher working memory capacity, as motivated by the results of Ruiz Hernández (Reference Ruiz Hernández2018). Our approach is fully in line with the idea of a shared platform for studying SLA argued for by MacWhinney (Reference MacWhinney2017), though our focus is on fully integrating such a platform in real-life secondary schools as the place where most foreign language teaching happens in Europe. An online workbook such as the FeedBook providing individual support to students practicing a foreign language readily supports such seamless integration.
In terms of a more specific outlook, we discussed the relevance of scaffolding completion of exercises involving both form and meaning, which we illustrated with a reading comprehension exercise. Extending the system in the direction of more such meaning-based activities in our opinion would be attractive, especially when extending it to more advanced learners. Currently, the highlighting of the information sources is manually encoded for a given question and text since it only involves minimal effort and ensures high-quality annotation. Information source detection as well as the automatic analysis of short-answer exercises could be automated, though, which would open up new possibilities for adaptive learning. The question of how to automatically determine whether the information provided in a response is sufficient to answer a reading comprehension question given a text is addressed in the CoMiC project, and the results presented in Ziai and Meurers (Reference Ziai and Meurers2018) improve the state-of-the-art of automatic meaning assessment of short answers to new questions (i.e., that were not part of the training material of the supervised machine learning). It thus in principle becomes possible to generate questions on the fly given a text chosen by the reader and still assess the learner responses automatically. Such a scenario becomes interesting when moving from publisher-provided exercises to the automatic generation of exercises adapted to the interests and proficiency of individual students. For this, language-aware search engines such as FLAIR (Chinkina & Meurers, Reference Chinkina and Meurers2016) support input enrichment, ensuring frequent representation of structures to be acquired, that in addition can also be made more salient with automatically generated visual (Ziegler et al., Reference Ziegler, Meurers, Rebuschat, Ruiz, Moreno Vega, Chinkina and Grey2017) or functionally driven input enhancement (Chinkina & Meurers, Reference Chinkina and Meurers2017).
Author ORCIDs
Detmar Meurers, 0000-0002-9740-7442
Acknowledgments
We would like to thank the three reviewers for their detailed helpful comments, and Verena Möller for her rich contributions as a teacher and researcher on the FeedBook project during the first year, and Christoph Golla for the friendly and supportive collaboration as project partner at the Westermann Gruppe. We are grateful to Harald Baayen for his inspiring wisdom and friendliness in discussing statistical analysis. We would also like to thank Elizabeth Bear, Madeeswaran Kannan, Jekaterina Kaparina, Tobias Pütz, Simón Ruiz, Tamara-Katharina Schuster, and Frederica Tsirakidou for their contributions as graduate research assistants on the project. The FeedBook project was supported by the German Science Foundation (DFG) as Transfer Project T1 of the SFB 833. Detmar Meurers's perspective on the topic also substantially benefited from the sustained interdisciplinary collaboration in the LEAD Graduate School & Research Network (GSC1028), funded by the Excellence Initiative of the German federal and state governments.