National food consumption surveys are the main method used to monitor food consumption trends, nutritional status and exposure to hazardous substances in a population or to evaluate the impact of dietary policies. Ensuring the representativeness of the sample population and collecting accurate data are the biggest challenges(1). Since 2007, a decrease in response rates, defined as the ratio between the number of participants and all expected interviews (including unreachable and ineligible individuals), has been observed in many epidemiologic studies(Reference Galea and Tracy2), as reported in food consumption surveys in several European countries(Reference Ioannidou, Horváth and Arcella3,Reference Ax, Warensjö Lemming and Becker4) , and the USA(Reference Ahluwalia, Dwyer and Terry5). The reasons for refusal may include an increase in requests for study participation, declining trust in science, and increasingly complex research protocols(Reference Galea and Tracy2,Reference Ioannidou, Horváth and Arcella3) . As an example, in France, the previous 7-d self-administered paper food records methodology(Reference Dubuisson, Lioret and Touvier6,Reference Lioret, Dubuisson and Dufour7) has shifted to interview-led 24-h dietary recalls (24-h DR) in the most recent cross-sectional Individual and National Study on Food Consumption 3 (INCA3) conducted in 2014–2015. The new protocol required four contacts to complete the dietary recalls after having agreed to take part, compared to two contacts in the INCA2 survey. This change may have had a negative impact on the response rate which decreased by about 20% points compared to the INCA2 study. This led to an increase in the duration of fieldwork and in costs to ensure representative population sample(Reference Dubuisson, Dufour and Carrillo8). There is a need to shift towards more user-friendly tools and to adapt surveys to the population’s current lifestyle (e.g. longer working hours(Reference Galea and Tracy2)), while maintaining high data quality at an acceptable cost.
A wide range of technological options for dietary assessments are available(Reference Illner, Freisling and Boeing9). They can be categorised as computer-based (offline or online), mobile-based or image-based tools. Offline computer-based tools have already been used in several national surveys(Reference Slimani, Casagrande and Nicolas10–Reference Caswell, Talegawkar and Dyer14) and have shown some limitations, in particular for data management(Reference Dubuisson, Dufour and Carrillo8,Reference Amoutzopoulos, Steer and Roberts15–Reference Bel and De Ridder17) . For instance, adapting GloboDiet software to European national surveys, as well as checking and cleaning the collected data according to the FoodEx2 classification, was very time-consuming and costly(Reference Zhang, Geelen and Boshuizen16–Reference Dubuisson, Carrillo and Dufour18). Other technologies such as online computer-based, mobile-based or image-based tools have rarely been used in national dietary surveys, probably because of doubts about their acceptability within the population, or a lack of evidence about their validity and costs to collect data that are both nationally representative and accurate(Reference Amoutzopoulos, Steer and Roberts15).
Regarding mobile-based tools collecting dietary intakes, most were developed for commercial purposes(Reference Illner, Freisling and Boeing9,Reference Cade19,Reference Eldridge, Piernas and Illner20) , often with the aim of helping individuals to manage their weight(Reference Illner, Freisling and Boeing9,Reference Cade19,Reference Franco, Fallaize and Lovegrove21) . These tools may lack validity and transparency(Reference Cade19,Reference Bell, Colaiezzi and Prata22) , and they require that a large proportion of the population has a smartphone. A mobile-based solution not fully online, called INDDEX24, has been designed for low- and middle-income countries(23,Reference Wafa, Colaiezzi and Some24) to fill the lack of tools meeting specific constraints in those countries (low smartphone penetration, low literacy, lack of connectivity, etc.)(Reference Bell, Colaiezzi and Prata22,Reference Coates, Colaiezzi and Bell25) . The tool includes a tablet and mobile application available online and offline, as well as a web platform for data management. This tool is currently in the process of being validated and represents potential for specific national dietary surveys. Barcode scanning applications usually used on mobile might be valuable for dietary assessments, but current tools are not reliable for use in national surveys without an extensive development phase and validation studies(Reference Maringer, Wisse-Voorwinden and van’t Veer26). As mobile-based tools, various technologies of image-based tools are available but all require further development to be validated on a wide range of food products and on a large sample size of individuals(Reference Tay, Kaur and Quek27–Reference Kouvari, Mamalaki and Bathrellou29).
Online computer-based tools (mainly using 24-h DR) appear to be the most mature technology to be adapted to national food consumption surveys without requiring long and costly development steps. Importantly, some of them have already been used in large-scale epidemiological studies(Reference Lindroos, Petrelius Sipinen and Axelsson30–Reference Rowland, Adamson and Poliakov34), and they were designed to be easily adaptable to other populations(Reference Kirkpatrick, Gilsing and Hobin35–37). They can be adapted to smartphones, and many have been validated among children and/or adults(Reference Bell, Colaiezzi and Prata22,Reference Timon, van den Barg and Blain38) . To our knowledge, only one review focuses on web- and computer-based 24-h DR(Reference Timon, van den Barg and Blain38). In the Timon et al. review(Reference Timon, van den Barg and Blain38), common design features and the methods used to assess the ability of 24-h DR tools to accurately assess nutritional and dietary intakes have been fully detailed, but no information about user and researcher usability were reported(Reference Timon, van den Barg and Blain38).
To tackle the challenges encountered by national dietary surveys, such as maximising the response rate and collecting data from a representative sample of the population of interest while optimising the ratio between cost and data quality, existing online 24-h DR seem to have potential for the collection of good quality data while being less burdensome for the respondent and investigator. The aim of this study was to describe existing online 24-h DR tools in diverse aspects, such as functionalities, validation of nutrient estimations, user and researcher usability, and potential adaptability for integration into national dietary surveys.
Methods
Terminology
Here, validity means the extent to which a tool measures what it is intended to measure. The validity of dietary instruments is generally assessed by comparing nutrients and/or food intake estimates with another method considered the gold standard, which can be subjective (24-h DR, food diary, FFQ, etc.) or objective (biomarkers, observational studies, etc.)(39,40) . According to the ISO 9241-11:2018 Standard(41), user usability is a measure of how well a user can learn and correctly use the tool’s functions, the ease of use, and user satisfaction in terms of whether a user can achieve his or her goals when using the tool. User usability is assessed using retrospective methods such as questionnaires, administered after the experience of the tool and/or real-time methodologies such as concurrent think-aloud protocols(Reference Birns, Joffre and Leclerc42). In this paper, the term flexibility means the extent to which a tool can be easily modified and adapted to be used in a context other than the one for which the tool was developed. To simplify the manuscript, the term food is used instead of ‘food and beverages’ to describe the identification of all foods and beverages declared as consumed by the respondent.
Search strategy
Online computer-based self-administered 24-hD R tools were identified from reviews identified using a first search on Pubmed with the following terms, alone or in combination in the title or abstract: ‘survey’, ‘tool’, ‘instrument’, ‘assessment’, ‘questionnaire’, ‘measurement’, ‘diet’, ‘dietary’, ‘nutrient’, ‘food’, ‘intake’, ‘dietary pattern’, ‘dietary assessment’, ‘consumption’, ‘web’, ‘online’, ‘remote’, ‘digital’, ‘software’, ‘application’, ‘technology’, ‘ehealth’, and ‘review’, ‘meta-anal*’, and ‘systematic’. For the present paper, only two reviews including an evaluation and description of 24-h DR tools were retained (Timon et al. (Reference Timon, van den Barg and Blain38) and Bell et al. (Reference Bell, Colaiezzi and Prata22)). Keywords were also used to identify relevant grey literature in Google, such as Timmins et al. (Reference Timmins, Vowden and Husein43) and Coates et al. (Reference Coates, Colaiezzi and Bell25), leading to the identification of two reports. From these four reviews or reports, focusing on tools published between 2000 and 2016, the authors identified a list of 24-h DR tools. An additional search with the same keywords (except ‘review’, ‘meta-anal*’, and ‘systematic’) was conducted to update the list and identify other tools published after the reviews or reports (published between 2017 and 2019) on PubMed and on Google in order to identify commercial tools without scientific publications.
Description criteria
For each tool, general characteristics, dietary intake collection methodology, as well as validation methodology and user usability were assessed based on the scientific literature and/or published reports. Functionalities and the method used to collect dietary intakes were described according to the United States Department of Agriculture (USDA) five-step multiple-pass 24-h DR method, a standardised and structured interview to record dietary intakes, during which several cues are used to help the respondent to remember and detail as accurately as possible of all foods consumed(Reference Conway, Ingwersen and Vinyard44). Additionally, information on the tools’ flexibility to be adapted to another context was collected. All criteria chosen to describe the tool are reported in Fig. 1.
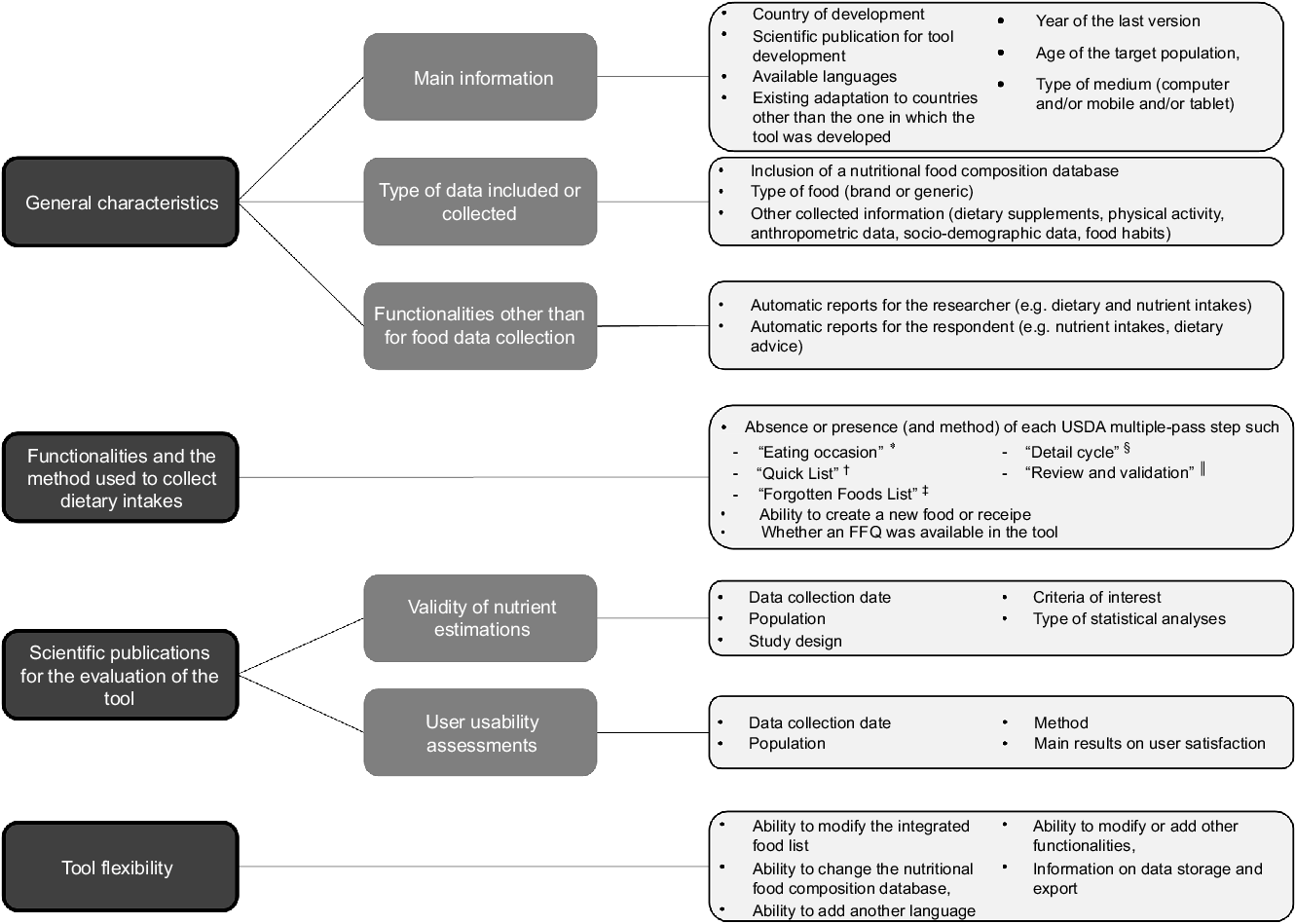
Fig. 1 Criteria used to describe the tools. 24-h DR, 24-h dietary recall
*‘Eating occasion’ step is the collection of time, name and place of consumption of each food reported.
†‘Quick list’ step is the identification of all foods that the respondent consumed during the previous day.
‡‘Forgotten food list’ step provides cues about the consumption of often forgotten foods.
§ ‘Detail cycle’ step is the collection of detailed information on each food such as the fat content, brand name, preservation method and the consumed amount.
|| ‘Review and validation’ step is the final review of the 24-h DR.
Once tables were considered to be as complete as possible, based on available published papers or reports, phone or online video unstructured interviews were conducted with the corresponding authors of the studies, or the owner or developer of each tool in October 2019. The aim of the interviews was to check the already collected information, to validate specific points or to add information that could not be found in the literature. All collected information on validation and user usability studies as well as functionalities to collect dietary intakes were from published papers, whereas certain general characteristics (in particular available languages, last version and type of medium), and all information on flexibility were directly collected from the tool owner or developer.
A letter was assigned to each tool and used in the tables and text to refer to it when necessary.
Results
General description
The identification of online 24-h DR tools cited in the reviews and reports led to the selection of thirteen tools as follows (with the corresponding letter) (Fig. 2): Automated Self-Administered 24-h DR (A, ASA24)(Reference Zimmerman, Hull and McNutt45), Children’s and Adolescents’ Nutrition Assessment and Advice on the Web (B, CANAA-W) (previously Young Adolescents’ Nutrition Assessment on Computer, YANA-C)(Reference Vereecken, Covents and Maes46,Reference Vereecken, Covents and Sichert-Hellert47) , Computer-Assisted Personal Interview System (C, CAPIS)(Reference Shin, Park and Sun48), Compl-Eat (D)(Reference Meijboom, van Houts-Streppel and Perenboom49), DietAdvice (E)(Reference Probst, Lockyer and Tapsell50), DietDay (F)(Reference Arab, Wesseling-Perry and Jardack51), Web-based Food Behaviour Questionnaire (G, FBQ)(Reference Hanning, Royall and Toews52), Food Record Checklist (H, FoRC)(Reference Comrie, Masson and McNeill53), INTAKE24 (I, previously Self-Completed Recall and Analysis of Nutrition, SCRAN24)(Reference Simpson, Bradley and Poliakov54), Measure Your Food On One Day (J, myfood24)(Reference Carter, Albar and Morris55), NutriNet-Santé (K)(Reference Touvier, Kesse-Guyot and Méjean31), Portuguese self-administered computerised 24-h DR (L, PAC24)(Reference Carvalho, Santos and Rito56) and Web-Survey of Physical Activity and Nutrition (M, Web-SPAN)(Reference Storey, Forbes and Fraser57). Five other online 24-h DR, published between 2016 and 2019, were added (Fig. 2): ClinShare (N), Creme Diet (O, published under the name foodbook24)(Reference Timon, Blain and McNulty58), Web-based 24-h DR (P, R24W)(Reference Jacques, Lemieux and Lamarche59), RiksmatenFlex (Q)(Reference Moraeus, Lemming and Hursti60) and Self-Administered Children, Adolescents, and Adult Nutrition Assessment (R, SACANA)(Reference Hebestreit, Wolters, Jilani, Bammann, Lissner and Pigeot61). In all, eighteen online 24-h DR tools were selected for this study (Fig. 2).
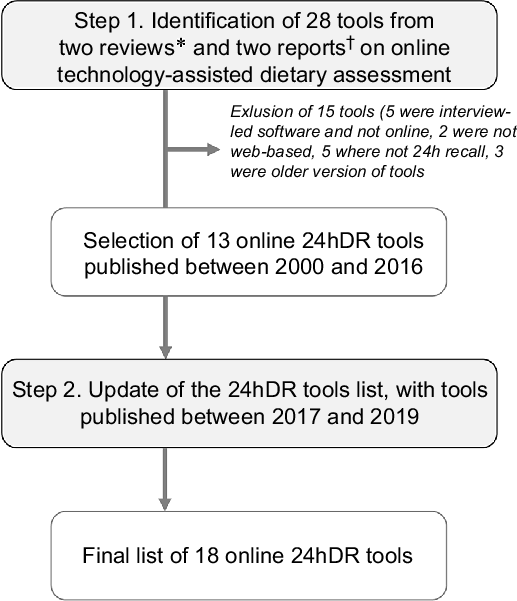
Fig. 2 Flow chart for the selection of the online 24-h DR tools. 24-h DR, 24-h dietary recall
* The two reviews were the followings (38 and 43).
† The two reports were the followings (44 and 25).
A general description of the eighteen identified tools is available in Table 1. Among them, eleven (B(Reference Vereecken, Covents and Maes46), D(Reference Meijboom, van Houts-Streppel and Perenboom49), H(Reference Comrie, Masson and McNeill53), I(Reference Simpson, Bradley and Poliakov54), J(Reference Carter, Albar and Morris55), K(Reference Touvier, Kesse-Guyot and Méjean31), L(Reference Carvalho, Santos and Rito56), N, O(Reference Timon, Blain and McNulty58), Q(Reference Moraeus, Lemming and Hursti60) and R(Reference Hebestreit, Wolters, Jilani, Bammann, Lissner and Pigeot61)) were developed in Europe, five (A(Reference Zimmerman, Hull and McNutt45), F(Reference Arab, Wesseling-Perry and Jardack51), G(Reference Hanning, Royall and Toews52), M(Reference Storey and Mccargar70) and P(Reference Jacques, Lemieux and Lamarche59)) in North America (USA and Canada), one (E(Reference Probst, Lockyer and Tapsell50,Reference Probst, Jones and Lin64) ) in Australia and one (C(Reference Shin, Park and Sun48)) in South Korea. Five (A(71), I(Reference Foster, Lee and Imamura72), J(Reference Koch, Conrad and Hierath36), K(73) and R(Reference Hebestreit, Wolters, Jilani, Bammann, Lissner and Pigeot61)) have already been adapted to be used in another country, and in particular, two (I(Reference Foster, Lee and Imamura72) and J(Reference Scarpa, Berrang-Ford and Bawajeeh74)) have already been adapted for low-income countries (Middle East countries, Peru or the South-Asia region). Only one language is available in twelve tools (C–H, K, L–O and Q) (among them six in English: E–H, M and O), while the other six tools (C, D, K, L, N and Q) are in various languages. Three tools (D, I and Q) have been adapted or are being adapted for all populations (including infants), while the others were developed for teenagers and/or adults. Eight tools (A, I–K and N–Q) can (or will) be used on computers, mobiles and tablets, thanks to an automatic adjustment of the web page to the tool’s size (i.e. responsive design). Except four tools (G, H, K andf M), all have an integrated food composition database, allowing for automatic assessment of individual food and nutrient intakes for the researcher. Eleven tools (A–C, F, G, I–K, M, O and R) have a functionality to provide the respondent with a summary of their dietary intakes and for some tools, dietary advice(75–Reference Rowland, Rose and McLean78). While four tools (E, I, L and R) collect food intake data only, some tools collect other information such as dietary supplements (A, D, F, J and O), the level of physical activity (via a questionnaire) (B, C, K, M, N and Q), anthropometry (B, C, K, M and N), sleeping habits (A) or other information on food habits (G, H, K, M, N, P and Q).
Table 1 General description of the online 24-hD R tools*

ANT, anthropometric data; ASA24, Automated Self-Administered 24-h diet recall; B, Brand level; C, computer; CANAA-W, Children’s and Adolescents’ Nutrition Assessment and Advice on the Web; CAPIS, Computer-Assisted Personal Interview System; DS, dietary supplement; FBQ, Web-based Food Behaviour Questionnaire; FH, food habits; FoRC, Food Record Checklist; G, generic; M, mobile; myfood24, Measure Your Food On One Day; N, No; NA, missing information; PA, physical activity; PAC24, Portuguese self-administered computerised 24-h dietary recall; SD, socio-demographic data; R24W, Web-based 24-h dietary recall; Ref, References; SACANA, Self-Administered Children, Adolescents, and Adult Nutrition Assessment; T, tablet; Web-SPAN, Web-Survey of Physical Activity and Nutrition; Y, yes.
* All information was validated by the tools’ owners or developers, except for the tools Creme Diet, CAPIS, CANAA-W, Diet Advice, DietDay, FoRC and FBQ.
† The name is underlined when information was validated by the developer/owner of the tool.
‡ Publications of tool development.
§ In the most recent version of the tool.
|| In the version published.
¶ Initially developed by Newcastle University, Newcastle, UK, with funding from Food Standards Scotland, Adaptation by the University of Cambridge, Cambridge, UK.
Method of dietary intake collection
Table 2 describes the main functionalities of the tools to collect dietary intakes.
Table 2 Step number and method of the multiple-pass methodology and main functionalities to collect dietary intakes
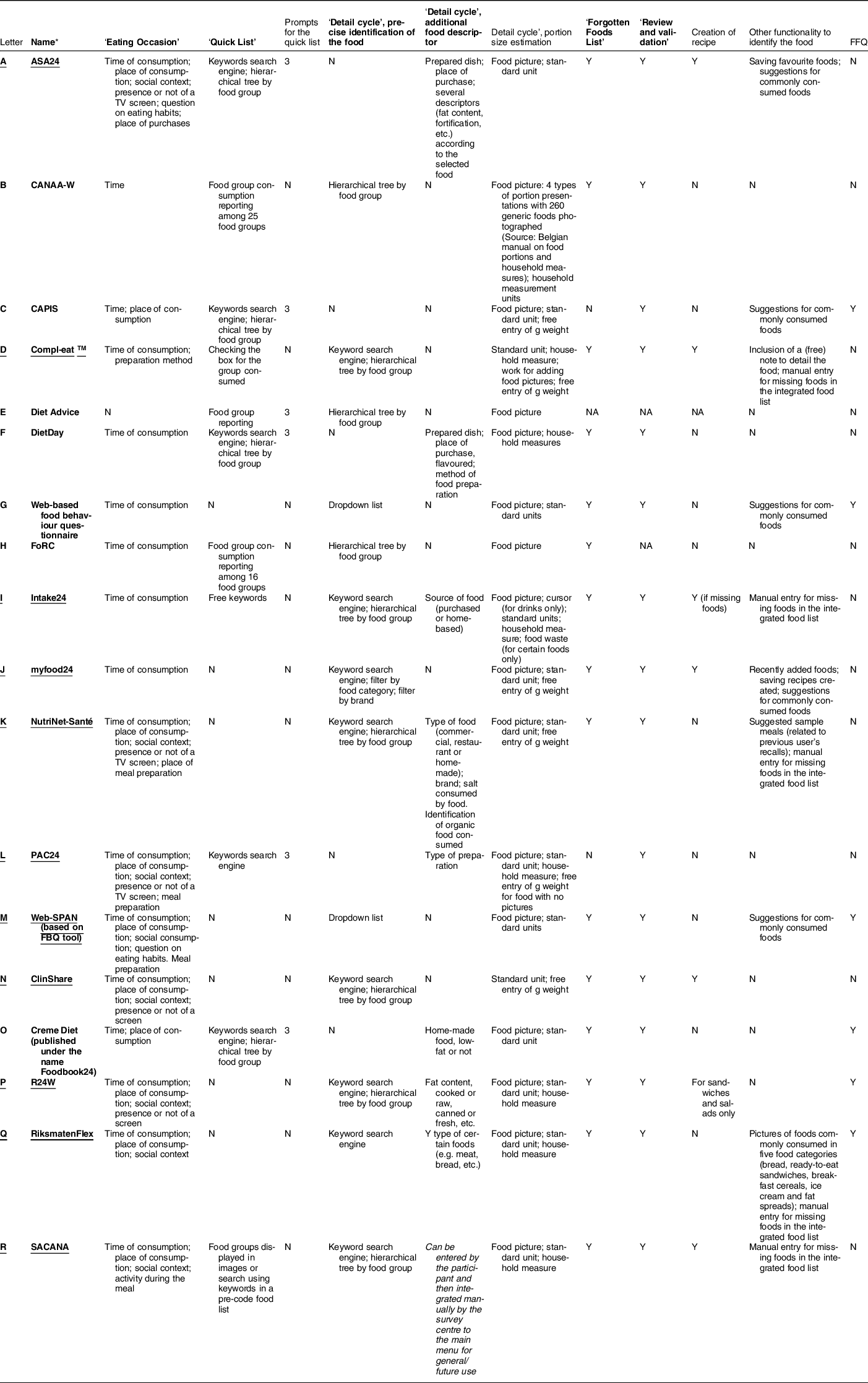
ASA24, Automated Self-Administered 24-h diet recall; CANAA-W, Children’s and Adolescents’ Nutrition Assessment and Advice on the Web; CAPIS, Computer-Assisted Personal Interview System; FBQ, Web-based Food Behaviour Questionnaire; FoRC, Food Record Checklist; myfood24, Measure Your Food On One Day; N, No; PAC24, Portuguese self-administered computerised 24-hour dietary recall; R24W, Web-based 24-h dietary recall; SACANA, Self-Administered Children, Adolescents, and Adult Nutrition Assessment; Web-SPAN, Web-Survey of Physical Activity and Nutrition; Y, yes.
* The name is underlined when information was validated by the developer/owner of the tool.
Eight tools (A, B, D, F, H, I, O and R) display the same steps as the USDA multiple-pass method, but not necessarily in the same order and not necessarily using the same method to collect the ‘Quick list’ (e.g. identification in a pre-defined list of foods, using free keywords or food group checkboxes). Other tools either do not include the ‘Forgotten food list’ step (n 3; C, E, L) or do not include the ‘Quick list’ step (n 7; G, J, K, M, N, P, Q). Tools without a ‘Quick list’ ask the respondent to provide all information (identification, description and quantification of the food) in one step for each consumption occasion of the day. The time of consumption is always requested, and other information, including the place of consumption (n 10; A, C and K–R), place of meal preparation (n 1; K), social context (n 8; A, K–N and P–R) and presence of a screen (n 5; A, K, L, N and P) can be requested depending on the tool.
The whole list of foods from which the respondent selects the one consumed depends on the study and version of the tool and can contain either generic foods only (often from national food composition databases), or generic and specific brand products (Table 1). In order to ease food selection by the user, the selected tools use different food identification systems (either in the ‘Quick list’ or ‘Detail cycle’ steps):
-
- using a keyword search engine (n 13; A, C, D, F, I–L and N–R),
-
- by selecting within a hierarchical tree (n 13; A–F, H, I, K, N, O, P and R),
-
- by selecting within a dropdown list (n 2; M and G),
-
- by filtering foods (n 2; A and J) by category, brand, type of food (generic or brand) or from a list of favourite foods,
-
- by selecting from pictures (n 1, for specific food groups; R).
Five tools (B(Reference Vereecken, Covents and Maes76), I(Reference Rowland, Adamson and Poliakov34), J(Reference Carter, Albar and Morris55), O(Reference Timon, Blain and McNulty58) and Q(Reference Lindroos, Petrelius Sipinen and Axelsson30)) have improved their keyword search engine by including synonyms and different spelling options or brand names to help participants find the correct food or to allow the identification of foods by matching more than one search term (e.g. chocolate biscuits). Other functionalities helping the respondent to report the correct food consumed were identified, such as the creation of personal recipes (n 7; A, D, I, J, N, P and R), or reporting a new food (free text entry) not yet in the integrated food list (n 5; D, I, K, Q and R).
Portion size estimation is requested, either directly after having identified a food (n 7; G, J, K, M, N, P and Q) or in the second step after having identified all foods consumed during the day (n 11; A–F, H, I, L, O and R). Quantification can be entered directly in grams or volumes (n 6; C, D, J–L and N), or using portion size estimation aids such as food portion pictures (n 16; A–C, E–M and O–R), standard units of consumption (n 14; A, C, D, G and I–R) or household measures (n 8; B, D, F, I, L, P, Q and R). Only two tools do not use food pictures (D and N). To our knowledge, only one tool (I) also requests, for some foods, the amount of food that is left over. The type of packaging or way of consumption can also be asked to refine the picture to display (e.g. consumption of an entire fruit or in pieces, consumption of a soda in a bottle, a can or a cardboard container)(Reference Simpson, Bradley and Poliakov54). For beverages, one tool (I) uses a cursor to fill the container chosen by the respondent (glass, bowl, etc.).
Method of validity assessment
Table 3 describes the methods used to validate nutrient and/or food group intake estimates using the tool, and Table 4 describes user usability assessment studies.
Table 3 Methodological characteristics of the validation studies for the online 24-h DR tools
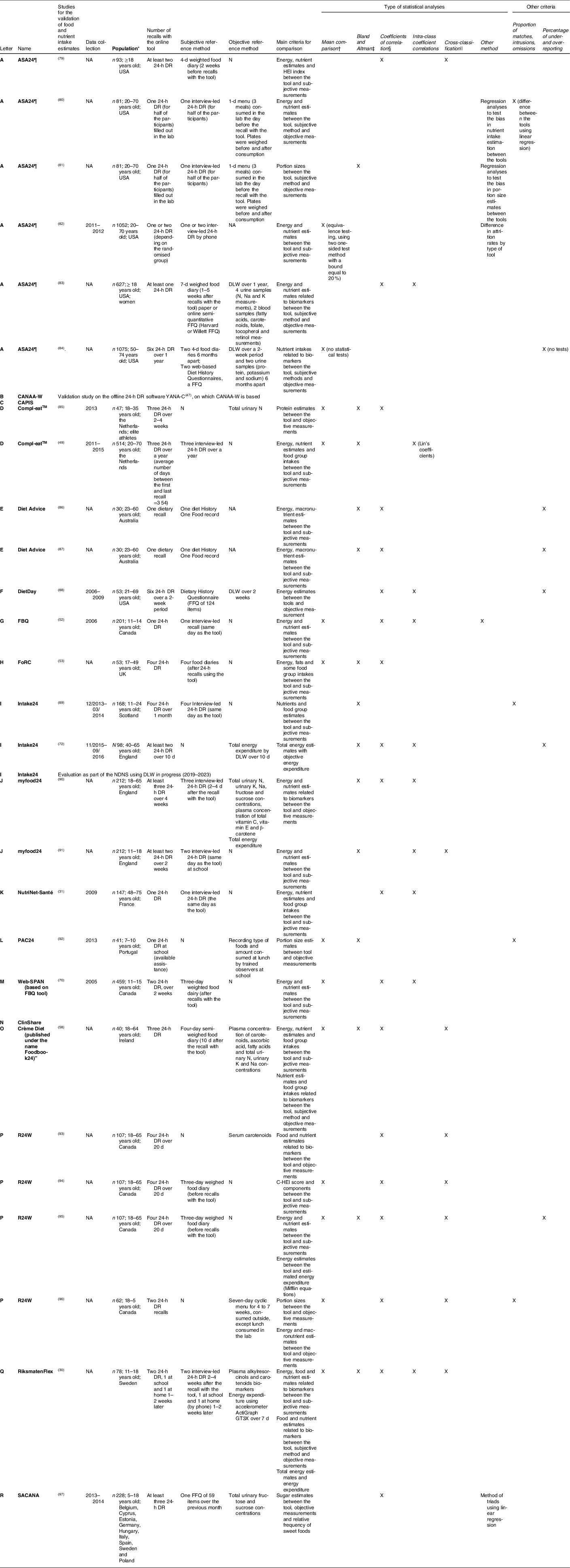
ASA24, Automated Self-Administered 24-h diet recall; CANAA-W, Children’s and Adolescents’ Nutrition Assessment and Advice on the Web; CAPIS, Computer-Assisted Personal Interview System; FBQ, Web-based Food Behaviour Questionnaire; FoRC, Food Record Checklist; myfood24, Measure Your Food On One Day; N, No; NA, missing values; PAC24, Portuguese self-administered computerised 24-h dietary recall; R24W, Web-based 24-h dietary recall; Web-SPAN, Web-Survey of Physical Activity and Nutrition; SACANA, Self-Administered Children, Adolescents, and Adult Nutrition Assessment; HEI, Health Eating Index; C-HEI, Canadian Healthy Eating Index; DLW, doubly labelled water; NDNS, National Diet and Nutrition Survey; 24-h DR, 24-h dietary recall.
Grey cells are tools without publications on the tool.
* Final sample size, age, country and specificity if needed
† t-test or paired t-tests or Wilcoxon signed rank test;
‡ graphical method and limit of agreements
§ Spearman or Pearson, de-attenuated or raw correlation;
|| Cross-classification and weighted kappa coefficient;
¶ ASA24 was also validated among specific subpopulations such as low-income individuals, children 10–13 years of age, overweight and obese women, multi-ethnic older adults. All publications are available here: https://epi.grants.cancer.gov/asa24/resources/publications.html.
Table 4 Methodological characteristics of the user usability studies for the online 24-h DR tools
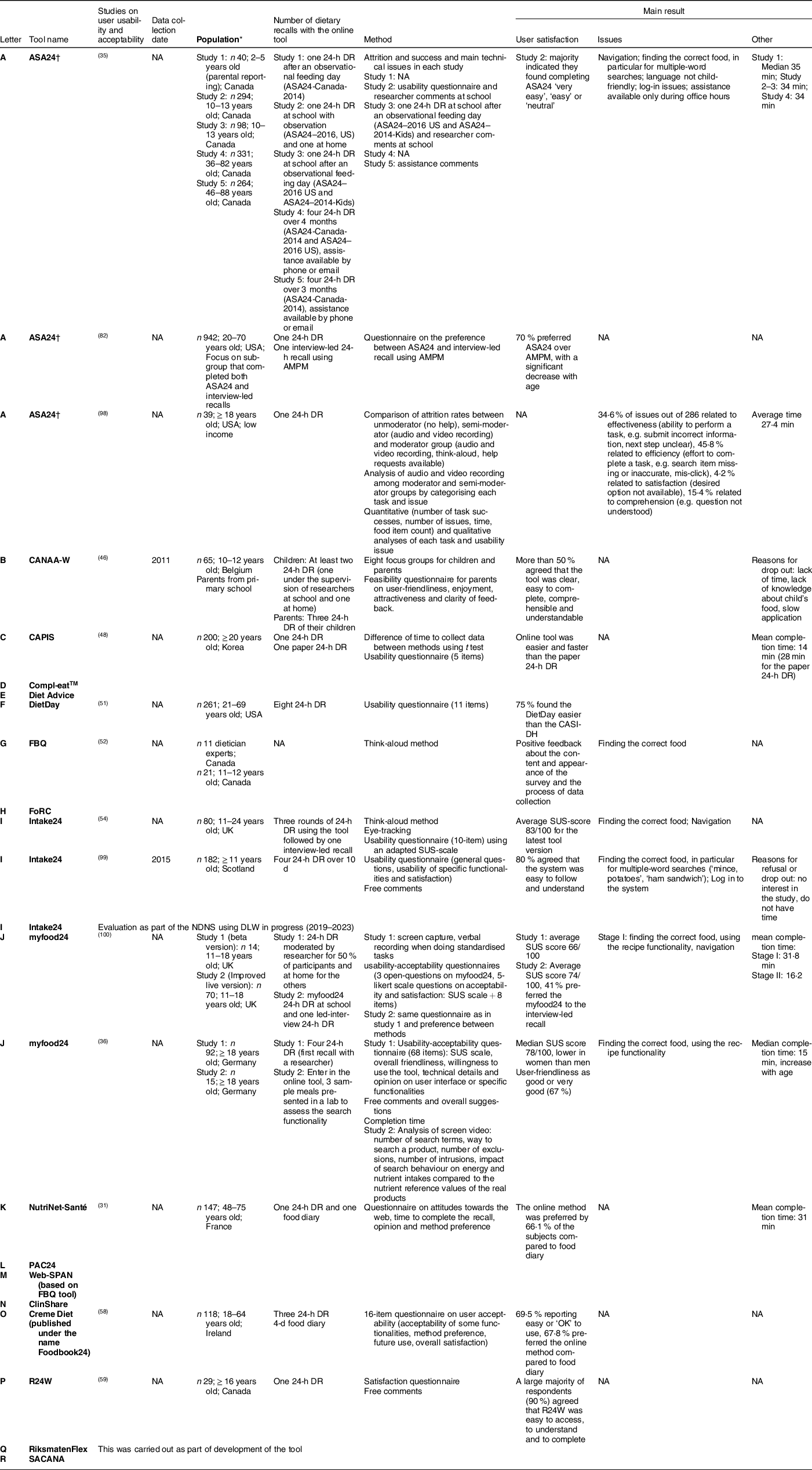
ASA24, Automated Self-Administered 24-h diet recall; CANAA-W, Children’s and Adolescents’ Nutrition Assessment and Advice on the Web; CAPIS, Computer-Assisted Personal Interview System; FBQ, Web-based Food Behaviour Questionnaire; FoRC, Food Record Checklist; myfood24, Measure Your Food On One Day; PAC24, Portuguese self-administered computerised 24-h dietary recall; R24W, Web-based 24-h dietary recall; SACANA, Self-Administered Children, Adolescents, and Adult Nutrition Assessment; SUS scale, System Usability Scale; Web-SPAN, Web-Survey of Physical Activity and Nutrition; DLW, doubly labelled water; NDNS, National Diet and Nutrition Survey.
Grey cells are tools without publications on the user usability;
* Final sample size, age, country and specificity if needed;
† ASA24 usability was also assessed among children and multi-ethnic older adults.
Validation of nutrient intake estimates was assessed in twenty-seven studies (n 15 tools). Three tools (B, C and N) had no publication on the validation of nutrient intake estimates. Six tools (A(Reference Frankenfeld, Poudrier and Waters79,Reference Yuan, Spiegelman and Rimm83,Reference Park, Dodd and Kipnis84) , E(Reference Probst, Sarmas and Tapsell86,Reference Probst, Sarmas and O’Shea87) , H(Reference Comrie, Masson and McNeill53), M(Reference Storey and Mccargar70), O(Reference Timon, Blain and McNulty58) and P(Reference Lafrenière, Laramée and Robitaille94,Reference Lafrenière, Laramée and Robitaille95) ) compared nutrient intake estimates to those from food diaries, seven (A(Reference Kirkpatrick, Subar and Douglass80–Reference Thompson, Dixit-Joshi and Potischman82), D(Reference Meijboom, van Houts-Streppel and Perenboom49), G(Reference Hanning, Royall and Toews52), I(Reference Bradley, Simpson and Poliakov89), J(Reference Wark, Hardie and Frost90,Reference Albar, Alwan and Evans91) , K(Reference Touvier, Kesse-Guyot and Méjean31) and Q(Reference Lindroos, Petrelius Sipinen and Axelsson30)) to nutrient intakes estimated by interview-led 24-h DR and three (A(Reference Yuan, Spiegelman and Rimm83), F(Reference Arab, Tseng and Ang88) and R(Reference Intemann, Pigeot and De Henauw97)) to estimates from FFQ. The number of days of dietary measurements as well as the time between data collection using the tool and the reference method varied widely between studies. For instance, from one (A(Reference Kirkpatrick, Potischman and Dodd81,Reference Yuan, Spiegelman and Rimm83,Reference Gilsing, Mayhew and Payette101) , E(Reference Probst, Sarmas and Tapsell86,Reference Probst, Sarmas and O’Shea87) , G(Reference Hanning, Royall and Toews52), K(Reference Touvier, Kesse-Guyot and Méjean31) and L(Reference Carvalho, Baranowski and Foster92)) to six consumption days (A(Reference Park, Dodd and Kipnis84) and F(Reference Arab, Tseng and Ang102)) were collected using the online 24-h DR tool in validation studies. Four tools (G(Reference Hanning, Royall and Toews52), I(Reference Bradley, Simpson and Poliakov89), J(Reference Albar, Alwan and Evans91) and K(Reference Touvier, Kesse-Guyot and Méjean31)) were validated against a reference method administered the same day(Reference Touvier, Kesse-Guyot and Méjean31,Reference Hanning, Royall and Toews52,Reference Bradley, Simpson and Poliakov89,Reference Albar, Alwan and Evans91) , whereas other tools administered the reference method a few weeks before or after use of the tool. Ten tools (A(Reference Kirkpatrick, Subar and Douglass80,Reference Kirkpatrick, Potischman and Dodd81,Reference Yuan, Spiegelman and Rimm83,Reference Park, Dodd and Kipnis84) , D(Reference Wardenaar, Steennis and Ceelen85), F(Reference Arab, Tseng and Ang88), I(Reference Foster, Lee and Imamura72), J(Reference Wark, Hardie and Frost90), L(Reference Carvalho, Baranowski and Foster92), O(Reference Timon, Blain and McNulty58), P(Reference Lafrenière, Couillard and Lamarche93,Reference Lafrenière, Lamarche and Laramée96) , Q(Reference Lindroos, Petrelius Sipinen and Axelsson30) and R(Reference Intemann, Pigeot and De Henauw97)) had validation studies using objective measurements (biomarkers or energy expenditure n 10 studies, corresponding to nine tools A(Reference Kirkpatrick, Subar and Douglass80,Reference Yuan, Spiegelman and Rimm83,Reference Park, Dodd and Kipnis84) , D(Reference Wardenaar, Steennis and Ceelen85), F(Reference Arab, Tseng and Ang88), I(Reference Foster, Lee and Imamura72), J(Reference Wark, Hardie and Frost90), O(Reference Timon, Blain and McNulty58), P(Reference Lafrenière, Couillard and Lamarche93,Reference Lafrenière, Lamarche and Laramée96) , Q(Reference Lindroos, Petrelius Sipinen and Axelsson30) and R(Reference Intemann, Pigeot and De Henauw97); feeding studies n 1 study: A(Reference Kirkpatrick, Potischman and Dodd81); or direct observation n 1 study: L(Reference Carvalho, Baranowski and Foster92)), nine (A, D, F, I, J and O–R) of which also had a validation study with a subjective reference measurement (in the same or another study). Six tools (A(Reference Kirkpatrick, Subar and Douglass80,Reference Yuan, Spiegelman and Rimm83,Reference Park, Dodd and Kipnis84) , F(Reference Arab, Tseng and Ang88), J(Reference Wark, Hardie and Frost90), O(Reference Timon, Blain and McNulty58), Q(Reference Lindroos, Petrelius Sipinen and Axelsson30) and R(Reference Intemann, Pigeot and De Henauw97)) were validated with both subjective and objective reference measurements in the same study, as recommended by Timon et al. (Reference Timon, van den Barg and Blain103). Four tools (A(Reference Kirkpatrick, Subar and Douglass80), I(Reference Bradley, Simpson and Poliakov89), L(Reference Carvalho, Baranowski and Foster92) and P(Reference Lafrenière, Lamarche and Laramée96)) assessed the proportion of exact ‘matches’, ‘omissions’ or ‘inclusions’.
Data were often analysed using a combination of statistical methods, measuring either the strength of an association at the individual level (correlation coefficients), the overall agreement between two measurements (mean comparisons), the agreement at the individual level (cross-classification and weighted Kappa coefficient), or the presence, direction and extent of bias between two measurements (graphics of Bland and Altman). The number of statistical analyses was between 2 and 5, with four studies out of twenty-seven (G(Reference Hanning, Royall and Toews52), O(Reference Timon, Blain and McNulty58), P(Reference Lafrenière, Laramée and Robitaille94) and Q(Reference Lindroos, Petrelius Sipinen and Axelsson30)) having more than three different statistical tests, as recommended by Lombard et al. to reflect each facet of validity(Reference Lombard, Steyn and Charlton104). Publication results indicated overall moderate to good validity of online 24-h DR according to the statistical tests, and estimated nutrient intakes were comparable to the reference values. For instance, in a control feeding study, gaps between true and reported energy, nutrient and food group intakes were comparable between the online tool A and the interview-led offline AMPM software(Reference Kirkpatrick, Subar and Douglass80). Validation criteria were comparable between the online tool J and interview-led 24-h DR, with several biomarkers(Reference Wark, Hardie and Frost90). Spearman’s correlations for urinary and plasma biomarkers were similar for both the online tool O and 4-d semi-weighed food diaries(Reference Timon, Blain and McNulty58). Overall, based on their validation studies, each tool is valid to estimate nutritional intakes (data not shown).
User usability assessment
User usability was assessed in fifteen studies (n 11 tools, A–C, F, G, I–K and O–Q), among which one tool (Q) assessed usability but without publishing the results. In eight studies (n 7 tools, A(Reference Kirkpatrick, Gilsing and Hobin35,Reference Thompson, Dixit-Joshi and Potischman82) , C(Reference Shin, Park and Sun48), F(Reference Arab, Wesseling-Perry and Jardack51), I(Reference Rowland, Poliakov and Christie99), K(Reference Touvier, Kesse-Guyot and Méjean31), O(Reference Timon, Blain and McNulty58) and P(Reference Jacques, Lemieux and Lamarche59)), user usability was assessed only using a retrospective questionnaire administered after data collection. The System Usability Scale (SUS)(Reference Brooke, Jordan, Thomas, Weerdmeester and McClelland105), a validated questionnaire of ten items measuring the overall usability of a system (i.e. software, website and application) was used in three studies (n 2 tools, I(Reference Simpson, Bradley and Poliakov54) and J(Reference Koch, Conrad and Hierath36,Reference Albar, Carter and Alwan100) ). SUS scores at least equal to 70 (out of 100) are considered ‘good’ by Bangor et al. (Reference Bangor, Kortum and Miller106). Concerning methods other than questionnaires, we can mention focus groups(Reference Vereecken, Covents and Maes46) (n 1 tool, B), a retrospective methodology to collect qualitative information and real-time methods such as think-aloud protocols(Reference Hanning, Royall and Toews52,Reference Simpson, Bradley and Poliakov54,Reference Carvalho, Santos and Rito56,Reference Kupis, Johnson and Hallihan107) (n 4 tools, A, G and I) as well as eye-tracking(Reference Simpson, Bradley and Poliakov54) (n 1 tool, I). In four studies (n 3 tools, A(Reference Kirkpatrick, Gilsing and Hobin35), I(Reference Simpson, Bradley and Poliakov54) and J(Reference Koch, Conrad and Hierath36,Reference Albar, Carter and Alwan100) ), both retrospective and real-time methods were used. Overall satisfaction could be considered good, but several common issues were reported: difficulties in identifying the correct food (A(Reference Kirkpatrick, Gilsing and Hobin35,Reference Kupis, Johnson and Hallihan107) , I(Reference Simpson, Bradley and Poliakov54,Reference Rowland, Poliakov and Christie99) and J(Reference Koch, Conrad and Hierath36,Reference Albar, Carter and Alwan100) ), in particular when the respondent used several words (e.g. ‘mince, potatoes’), issues in navigating within the system (A(Reference Kirkpatrick, Gilsing and Hobin35,Reference Kupis, Johnson and Hallihan107) , I(Reference Simpson, Bradley and Poliakov54) and J(Reference Albar, Carter and Alwan100)), and difficulties logging in (A(Reference Kirkpatrick, Gilsing and Hobin35) and I(Reference Rowland, Poliakov and Christie99)).
Tool flexibility
Among the eighteen tools, thirteen (B–H and L–Q) have not been adapted for use in another country (Table 1). Information about how the tool could be adapted from the investigator of the study and/or from the tool’s technical support team was collected for eleven tools. For eight tools (A, D, I–K, N, O and R), changes to the food list and addition of full nutritional composition are feasible by providing the data to technical support, as a template file with a specific structure. Addition of another language is feasible for six tools (A, I–K, O and R). A web platform is available for the investigator of the study for eight tools (A, D, I, J, N, O, Q and R). On the platform, it is possible, depending on the tool, to edit certain parameters: adding new foods, changing nutritional composition, amending portion size pictures, activating functionalities or questions, and managing a study (sending invitation emails, checking responses and exporting the databases). Finally, tools A, I, J, O and R seemed to be the most easily flexible to a new context (web platform for the investigator of the study, possible addition of another language and modification of the input data). Only three tools (I, O and soon A) allow flexibility to store the collected data on a server of the investigator team. For two other tools (K and R), data can only be exported on request, limiting ongoing monitoring of the study.
Discussion
Eighteen online 24-h DR tools were identified and described in detail. Most were developed in Europe, for children 10 years of age and older and/or for adults. All tools are self-administered and collect time of consumption, identification of all foods and beverages consumed, and quantification of the amount consumed, before checking and validating the entries. The common information collected by all tools makes it possible to obtain high-quality intake estimates, showing promising capabilities for their use in national food intake surveys. Beyond these similarities, each tool has its own specificities regarding the order and functionalities of the multiple-pass steps to help identify and quantify the foods consumed. These specificities may have an impact on user usability, which was assessed for fewer tools than the validity of nutritional intakes. User usability should be assessed more often, especially for tools to be used in national dietary surveys because usability is a major driver of the response rate, a significant challenge in such surveys. Moreover, the ability of these tools to be adapted to new environments needs to be carefully evaluated, in view of implementing them in different countries. This point is, however, rarely addressed in reports or articles. This is why the authors of the present study needed to conduct unstructured interviews with the owner or developer of each tool to obtain more information.
Eleven tools were assessed regarding user usability, mainly through retrospective data collection of user satisfaction using questionnaires. Initiated by Eysenbach in 2005(Reference Eysenbach108), the impact of design features on adherence, that is, the degree to which the user correctly uses the tool as designed and intended by the developer(Reference Sieverink, Kelders and van Gemert-Pijnen109), has been studied in particular in online intervention programmes on mental health, lifestyle or chronic care, to prevent non-usage and dropout attrition(Reference Ludden, van Rompay and Kelders110,Reference Ryan, Bergin and Wells111) . For instance, it is recognised that personalisation of functionalities (e.g. using an avatar for children) or content (e.g. providing tailored messages) for a specific target group or individual increases user efficiency(Reference Ludden, van Rompay and Kelders110). Theoretical models on adherence to web-based interventions have been developed(Reference Ryan, Bergin and Wells111) and could help to identify recommendations for designers to make the tool more attractive and easier to use. Among American adults, ASA24 (tool A) was preferred to interview-led AMPM software for 70 % of individuals(Reference Thompson, Dixit-Joshi and Potischman82). The attrition rate, defined as the percentage of individuals lost between the first and second 24-h DR, was slightly lower using ASA24 (tool A) (6 %) compared to AMPM (11 %), but no analyses were conducted to further understand the effect of the web-based system on this difference(Reference Thompson, Dixit-Joshi and Potischman82). More research is needed in this field to better identify, quantify and qualitatively describe issues and find opportunities to improve available tools.
Among the issues raised in user usability studies, a common one observed across tools is the ability to easily identify the correct food. Some tools have improved the keyword search engine(Reference Lindroos, Petrelius Sipinen and Axelsson30,Reference Timon, Blain and McNulty58,Reference Vereecken, Covents and Maes76,Reference Albar, Carter and Alwan100) , but optimising the search mechanism remains a field of development to improve attractiveness and user success. Doing so may improve user adherence, response rates and the validity of dietary data. Identifying the correct food is also highly dependent on the quality of the integrated food list, which must be diversified enough and representative of the population’s food habits. With the development of online platforms (e.g. OpenFoodFact(112)), dedicated to providing product labelling information on branded foods available on the market, the possibility of integrating these exhaustive databases into 24-h DR tools could be considered. There is no absolute agreement on the advantages of using branded products rather than generic foods in the database of the recall tools(Reference Koch, Conrad and Hierath36) but for the researcher, the collection of dietary data at branded level can provide many descriptors with less data management: the type of packaging, presence of a nutrition or health claim and fortification. However, when foods are at brand level, the challenge is to link each food to full nutritional composition (macronutrient and micronutrient content), generally available for generic foods. To reduce data management for researchers, automatic or semi-automatic procedures have recently been proposed to match foods with food composition tables, using fuzzy matching (comparison between two character strings) to provide a similarity score between food names and/or machine learning classifiers(Reference Lamarine, Hager and Saris113,Reference Chin, Simmons and Bouzid114) , or by estimating the percentage of agreement based on the available nutritional content between the brand and generic food(Reference Carter, Hancock and Albar115). When the choice is to use a generic food database, the tool must be adapted to collect additional information about the food consumed concerning aspects relevant to the study aims (e.g. source of food: purchased or home-made). For instance, ASA24 (tool A) uses an extensive database of more than 13 million pathways to collect detailed information on the foods consumed(116), but collection of the additional facets increases respondent burden. The development and integration of barcode scanning to identify foods(Reference Maringer, Wisse-Voorwinden and van’t Veer26) may improve usability in the next few years and could ease data collection for the user and investigator of the study. Barcode scanning is, however, not yet integrated in published online 24-h DR tools.
One challenge for 24-h DR tools to be used at national level is to ensure representativity and ideally to be adaptable to different countries. Ensuring representativity at the national level is challenging because studies have shown that age(Reference Rowland, Adamson and Poliakov34,Reference Carter, Albar and Morris55,Reference Thompson, Dixit-Joshi and Potischman82,Reference Ward, McLellan and Udeh-Momoh117) and income or educational level(Reference Rowland, Adamson and Poliakov34,Reference Kupis, Johnson and Hallihan98,Reference Kirkpatrick, Guenther and Douglass118) affected user usability with online 24-h DR. As a consequence, protocols must be tailored to the subpopulation (e.g. data collection at school(Reference Lindroos, Petrelius Sipinen and Axelsson30,Reference Vereecken, Covents and Sichert-Hellert47,Reference Bradley, Simpson and Poliakov89,Reference Albar, Alwan and Evans91) , to provide 24-h support, to allow collection of data with an interviewer(Reference Rowland, Adamson and Poliakov34), to provide public internet access, to offer a specific version for children by simplifying the language and adding an avatar(Reference Krehbiel, DuPaul and Hoffman119)). If the protocol or tool cannot be adapted, the dietary survey could be supplemented with an external study. For instance in France, the Nutri-Bébé 2013 survey, an observational cross-sectional study of children aged 15 d to 35 months living in France, collected detailed food consumption using food diaries filled by the parents and could supplement national INCA dietary surveys(Reference Chouraqui, Tavoularis and Emery120). Adapting 24-h DR to other countries can be very time-consuming and expensive, as previously shown with adaptation of GloboDiet(Reference Dubuisson, Dufour and Carrillo8,Reference Bel and De Ridder17,Reference König, Hasenegger and Rust121,Reference van Rossum, Nelis and Wilson122) . Most of the online 24-h DR tools reviewed in this study were developed for a highly specific context, limiting their potential adaptation. Furthermore, probably because our search criteria included online tools, most of the selected online 24-h DR were developed for high-income countries, as already highlighted by Bell et al. (Reference Bell, Colaiezzi and Prata22). Therefore, the tools identified may not be suitable for countries with specific constraints, such as low- and middle- income countries, in which a limited literacy and numeracy may be source of error when using a self-administered tool(Reference Gibson, Charrondiere and Bell123), and where the tool may be unusable in some region with a low internet connectivity(Reference Bell, Colaiezzi and Prata22,Reference Coates, Colaiezzi and Bell25) . But, as mentioned in the results, some of the tools identified in this paper were already or currently being adapted for being used in some low- and middle-income countries. The development for a new population, such as a new country or age class, requires an update of the pre-integrated food list, food composition database and food portion pictures to be representative of the population’s food habits. This must be followed by new assessment of validity and user usability, as done by Koch et al. for adaptation of myfood24 (tool J) to the German population(Reference Koch, Conrad and Hierath36). The available languages must also be adapted, if needed. Even though some tools have developed a web platform, easing the integration of new data, or were specifically developed to allow simple updates using file templates, considerable work will be required to construct the integrated database.
A few limitations of this review should be noted. First, our descriptions of the tools were mainly based on information available in papers or reports. Except for six tools (tools A, I–K; O, R), which had a demo version freely available or a presentation video, the authors of this study did not test the tools, and some information may have been missed. However, for eleven tools, the owner and/or developer reviewed and validated the requested information, limiting inaccuracies. Second, we chose to describe only the method used in validation studies without providing the results, which may limit appraisal of each tool. As noted by Timon et al. (Reference Timon, van den Barg and Blain103), high heterogeneity in the design of validation studies means that studies must be assessed in isolation, without any robust comparison between tools. Additionally, validation and user usability assessment studies are specific to the population studied and must be renewed when applied to a different context. Nevertheless, our results provide an overview of the quality of the validation and user usability studies conducted with each tool. Third, in all publications, there is little evidence that using 24-h DR is cost-effective, although this argument was often put forward in papers on new technologies(Reference Timon, van den Barg and Blain38,Reference Cade124) . Fourth, we choose to not assign a ranking of the tool, because each decision-makers have their own criteria and needs. Our objective was to describe as precisely as possible the tools, regarding various aspects, in order to provide enough information for decision-makers to identify the best opportunities. Finally, the aim of this review was to focus on online 24-h DR tools, but technologies are moving rapidly and other technologies, in particular smartphone applications with visual recognition could evolve quickly and be validated for use in large-scale surveys. Likewise, some new validation studies(Reference Lafrenière, Couillard and Lamarche93,Reference Brassard, Laramée and Robitaille125–Reference Osadchiy, Poliakov and Olivier128) or user’s usability studies(Reference Timon, Walton and Flynn77,Reference Osadchiy, Poliakov and Olivier128) have been published since 2019, after the literature search conducted for this paper. Those articles published since 2019, not described in detail in this paper, are related to tools which were already described in this paper.
Conclusion
Eighteen online self-administered 24-h DR tools developed and validated in several contexts were identified. Tools that were validated to estimate nutritional and food intakes, that have confirmed user and researcher usability and that are sufficiently flexible to be adapted to different contexts, are probably the best candidates for use in national dietary surveys, as they are likely to improve response rates and to collect high-quality data. Regardless of the tool, adaptation to another context will require time and funding, and this is probably the most challenging step(131).
Acknowledgements
The authors would like to thank all persons who reviewed and supplemented the information collected: Simone Lemieux from Université Laval, Canada; Polly Page and Toni Steer, University of Cambridge, UK; Ivan Poliakov, Newcastle University, UK; Maria Ana Kadosh, University of Lisboa, Portugal; the National dietary assessment research team from the Swedish Food Agency; Ms Sarah Beer, University of Leeds, UK; Antje Hebestreit from the I.Family project; Valérie Deschamp, University of Paris, France; My Good Life team, Paris, France; Kate Storey, University of Alberta, Canada; ASA24 Support team, National Cancer Institute, USA; Jeanne de Vries, Wageningen University, the Netherlands.
Financial Support
The study was supported by ANSES.
Conflict of Interest
S.H. and C.D. have no conflicts of interest. R.G, S.M. and F.V. are employees of MS-Nutrition.
Authorship
R.G. designed the study, collected, analysed and interpreted the data, and wrote the manuscript. C.D. and S.H. designed the study and contributed to interpretation of the data. S.M. contributed to data collection and interpretation of the data. F.V. contributed to the analysis and assisted in writing the paper. All the authors reviewed the manuscript.
Ethical Standards Disclosure
Not applicable