Introduction
It is becoming increasingly accepted that psychiatric diagnostic categories are pragmatic, man-made constructs that should be interpreted as guidelines for clinical communication rather than true representations of underlying disorders (Marshall, Reference Marshall2020). Symptom expression is not disorder-specific, possibly explaining findings of high rates of comorbidity, general treatment effects, heterogeneity within disorders, and a lack of objective biomarkers (Hyman, Reference Hyman2010; Ofrat & Krueger, Reference Ofrat and Krueger2012; Olbert, Gala, & Tupler, Reference Olbert, Gala and Tupler2014).
To remedy this, a transdiagnostic approach has been put forth to advance the understanding of psychopathology in which mental disorders are not deemed categorically distinct entities. This transdiagnostic approach acknowledges that expression of symptoms can manifest across disorders and takes into account other clinically relevant dimensions, including cognitive functioning. Consortiums and research initiatives, such as the Research Domain Criteria (RDoC) framework (Insel et al., Reference Insel, Cuthbert, Garvey, Heinssen, Pine, Quinn and Wang2010) and the Hierarchical Taxonomy of Psychopathology (HiTOP) consortium (Kotov et al., Reference Kotov, Krueger, Watson, Achenbach, Althoff, Bagby and Clark2017), have been created with the goal to elucidate the nature of psychopathology without being bound to diagnostic categories. The Across study is among these research initiatives in its effort to employ a transdiagnostic approach to increase insight into the relationship among cognitive functioning, psychiatric symptoms, and biological parameters across psychiatric disorders (Nieman et al., Reference Nieman, Chavez-Baldini, Vulink, Smit, van Wingen, de Koning and Denys2020).
Previous research indicates that individuals with mental disorders often experience cognitive deficits (Iosifescu, Reference Iosifescu2012; Millan et al., Reference Millan, Agid, Brüne, Bullmore, Carter, Clayton and DeRubeis2012). For instance, executive dysfunction and memory deficits are apparent in patients with schizophrenia, depression, obsessive−compulsive disorder, post-traumatic stress disorder, and bipolar disorder (Czepielewski et al., Reference Czepielewski, Massuda, Goi, Sulzbach-Vianna, Reckziegel, Costanzi and Gama2015; Dere, Pause, & Pietrowsky, Reference Dere, Pause and Pietrowsky2010; Snyder, Miyake, & Hankin, Reference Snyder, Miyake and Hankin2015). Cognitive dysfunction cuts across disorders and should therefore be considered a transdiagnostic dimension (East-Richard, R-Mercier, Nadeau, & Cellard, Reference East-Richard, R-Mercier, Nadeau and Cellard2019; McTeague, Goodkind, & Etkin, Reference McTeague, Goodkind and Etkin2016). Additionally, cognitive dysfunction associated with psychiatric disorders has been shown to persist into remission (Balanzá-Martínez et al., Reference Balanzá-Martínez, Tabarés-Seisdedos, Selva-Vera, Martínez-Arán, Torrent, Salazar-Fraile and Gómez-Beneyto2005; Iosifescu, Reference Iosifescu2012; Semkovska et al., Reference Semkovska, Quinlivan, O'Grady, Johnson, Collins, O'Connor and Gload2019) and is predictive for recurrences (Ahern, Bockting, & Semkovska, Reference Ahern, Bockting and Semkovska2019), suggesting that it is a construct partly independent from psychiatric symptoms. However, the psychopathological component of psychiatric disorders tends to garner the most attention while cognitive functioning remains neglected. Cognitive deficits, nonetheless, have been associated with psychosocial dysfunction in patients with schizophrenia (Shamsi et al., Reference Shamsi, Lau, Lencz, Burdick, DeRosse, Brenner and Malhotra2011), bipolar disorder (Depp et al., Reference Depp, Mausbach, Harmell, Savla, Bowie, Harvey and Patterson2012), and depression (McIntyre et al., Reference McIntyre, Cha, Soczynska, Woldeyohannes, Gallaugher, Kudlow and Baskaran2013). The transdiagnostic nature of cognitive dysfunction and its heavy impact on daily functioning makes it therefore an important target for treatment (Millan et al., Reference Millan, Agid, Brüne, Bullmore, Carter, Clayton and DeRubeis2012). Treating cognitive dysfunction in addition to psychopathology could lead to better outcomes for patients.
One statistical method ideally suited for transdiagnostic research is network analysis (Borsboom, Reference Borsboom2017). Network analysis has been at the forefront of the broader paradigm shift in psychiatry as an alternative to the more traditional perspective of disorders as latent causes of symptoms. A network approach to psychopathology instead views disorders as constituted by symptoms which cause and interact directly with each other (Borsboom, Reference Borsboom2017). By assessing the relationship between symptoms, network analysis can provide insights into which symptoms are more central, which symptoms cluster together, and which symptoms bridge different clusters (Blanken et al., Reference Blanken, Deserno, Dalege, Borsboom, Blanken, Kerkhof and Cramer2018; Cramer, Waldorp, van der Maas, & Borsboom, Reference Cramer, Waldorp, van der Maas and Borsboom2010). This has resulted in network models showing how symptoms, such as psychotic and depressive symptoms (van Rooijen et al., Reference van Rooijen, Isvoranu, Kruijt, van Borkulo, Meijer, Wigman and Cahn2017), interact with each other. Network analysis can also elucidate interactions of symptoms with other clinically relevant factors, such as anxiety with attentional bias (Heeren & McNally, Reference Heeren and McNally2016) and cognition with stress as assessed with cortisol levels (Hinkelmann et al., Reference Hinkelmann, Moritz, Botzenhardt, Riedesel, Wiedemann, Kellner and Otte2009). Although some network analyses on the relationship between cognitive functioning and psychopathology have been conducted (Galderisi et al., Reference Galderisi, Rucci, Kirkpatrick, Mucci, Gibertoni, Rocca and Aguglia2018; Heeren & McNally, Reference Heeren and McNally2016), there is still a lack of research using a transdiagnostic network approach combining cognitive functioning and psychopathology.
The present study aims to elucidate the relationship between domains of cognitive functioning (i.e. visual attention, executive function, verbal and episodic memory, and alertness) and psychiatric symptoms (e.g. depression and anxiety) using a transdiagnostic approach (i.e. across disorders). In a large naturalistic transdiagnostic sample of patients with psychiatric disorders, we aim to (1) conduct a network analysis with cognitive measures and psychiatric symptoms, (2) perform a centrality analysis to detect which variables are important within the network, and (3) detect clusters using exploratory graph analysis.
Methods
Sample
The naturalistic sample consists of 1016 psychiatric patients recruited during intakes at outpatient clinic of the Department of Psychiatry at the Amsterdam University Medical Centers (UMC), location Academic Medical Center (AMC), in Amsterdam, the Netherlands. Inclusion criteria were: age 14–75 years, ability to give informed consent, having a DSM-IV-TR or DSM-V diagnosis as determined by a trained psychiatrist, and being fluent in Dutch. Exclusion criteria were: acute high risk of suicide, unstable medical disorder, premorbid IQ < 70, history of seizure or clinically significant abnormality of the neurological system. Written informed consent was obtained from patients and their parents (if underage). Patients could discontinue participation from the study at any time.
Procedure
The Across study is an ongoing, observational longitudinal cohort study and consists of the assessment of cognitive performance, psychiatric symptoms, and collection of biological data (DOI 10.17605/OSF.IO/YHVTB). The study instruments and procedures are described in Nieman et al. (Reference Nieman, Chavez-Baldini, Vulink, Smit, van Wingen, de Koning and Denys2020). Patients underwent extensive psychiatric and medical assessments at the outpatient clinic, performed by experienced psychiatrists and psychologists, and were then invited to participate in the study. The current study used cross-sectional data on cognitive performance and psychiatric symptoms. Cognitive performance was assessed with a computerized battery, followed by self-report questionnaires about various symptoms that were filled out on a computer. Patients were not required to abstain from substance or medication use before participation and were able to participate at any point of their clinical trajectory (e.g. before, during, or after treatment), which could influence their cognitive functioning and/or symptomology. However, this is an observational, naturalistic study that aims to reflect the reality of patients regular functioning. The study protocol was approved by the Medical Ethical Review Committee of the Amsterdam UMC (ABR no. NL55751.018.15), and data are stored according European to privacy laws.
Materials
Table 1 shows the instruments used to assess the different variables (nodes). For a detailed description of the instruments, see online Table S1 in the Supplementary Materials. To aid interpretability, cognitive variables were coded positively (higher is better) and psychopathology/substance use variables were coded negatively (higher is worse). All variables were continuous.
Table 1. Overview of measures
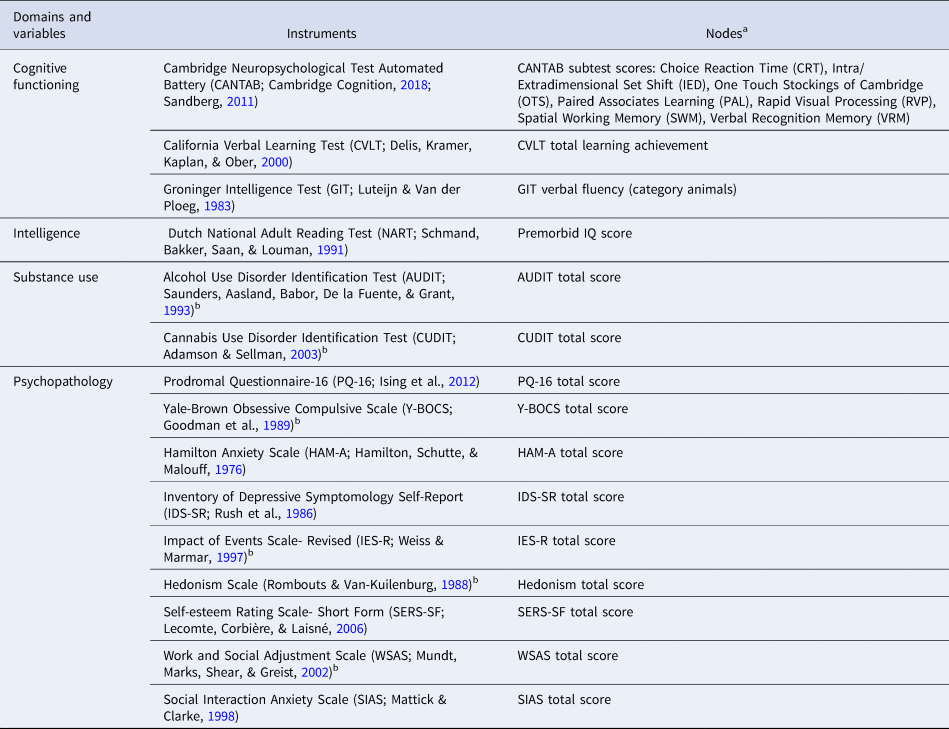
a The psychopathology and substance use nodes in this study were sum scores of symptom domains rather than individual symptoms.
b Assessed only for adult patients (18–75 years old) and put as missing in analyses for underage patients.
Network analysis
The analyses consisted of network estimation and visualization, cluster detection, and centrality analysis performed in R Version 1.2.5042 (R Core Team, 2020). The network estimation procedure resulted in a set of relationships between variables that can be visualized in a network in which the variables are nodes that are connected by a set of edges representing the estimated relationships. Network estimation was conducted with the R-package qgraph (Epskamp, Cramer, Waldorp, Schmittmann, & Borsboom, Reference Epskamp, Cramer, Waldorp, Schmittmann and Borsboom2012). The procedure started with a partial correlation network, in which correlations between two variables are corrected for all the other variables in the network. The extended Bayesian information criterion graphical least absolute shrinkage (EBICglasso) procedure (Chen & Chen, Reference Chen and Chen2008; Epskamp & Fried, Reference Epskamp and Fried2018; Foygel & Drton, Reference Foygel and Drton2010; Friedman, Hastie, & Tibshirani, Reference Friedman, Hastie and Tibshirani2008) was then applied to select edges by using a penalty, which decreases the strength of some of the parameter estimates, while others are set to zero. Following earlier network analyses, we used a γ of 0.5 for an optimal balance between density and sparseness (van Borkulo et al., Reference van Borkulo, Boschloo, Borsboom, Penninx, Waldorp and Schoevers2015). This resulted in a sparse network, in which the absence of an edge is interpreted as the conditional independence of two nodes given other variables (Friedman et al., Reference Friedman, Hastie and Tibshirani2008).
Visualization of the network was performed with qgraph (Epskamp et al., Reference Epskamp, Cramer, Waldorp, Schmittmann and Borsboom2012), using the Fruchterman−Reingold algorithm (Fruchterman & Reingold, Reference Fruchterman and Reingold1991), which placed nodes that are more connected closer together and nodes which have higher centrality indices closer to the center of the graph. Stronger edges were depicted as thicker lines between nodes. Positive associations were depicted in green and negative associations in red. Due to the coding, we expected most edges between domains of cognitive functioning and psychopathology to be red whereas edges between psychopathology nodes and edges between cognitive nodes were expected to be green.
Analysis of the network stability (Epskamp, Borsboom, & Fried, Reference Epskamp, Borsboom and Fried2018) was conducted (see online Appendix 1 in the Supplementary Materials) to give an indication of how reliable the estimated network is.
We computed the centrality index strength (Bringmann et al., Reference Bringmann, Elmer, Epskamp, Krause, Schoch, Wichers and Snippe2019; Epskamp et al., Reference Epskamp, Borsboom and Fried2018) using qraph (Epskamp et al., Reference Epskamp, Cramer, Waldorp, Schmittmann and Borsboom2012). Strength is calculated as the total of all edges of that node and indicates the degree of association of that node to its neighbors and the relative importance of each node in the full network. A node that has high strength is a node that has many and/or strong connections to its neighbors, whereas lower strength indicates a node with fewer and/or weaker connections.
Cluster detection was conducted with an exploratory graph analysis (EGA) as implemented in the R-package EGA (Golino & Epskamp, Reference Golino and Epskamp2017). EGA uses the ‘walktrap’ algorithm, which uses random walks to quantify the distance between any two nodes or clusters in a network (Pons & Latapy, Reference Pons and Latapy2006). Proximity between two nodes is defined as the degree of similarity of the distance of two nodes to the rest of the network. The walktrap algorithm then uses an agglomerative approach, grouping together the closest nodes or clusters step by step until all nodes are part of a cluster. The final step determines the point during the agglomeration in which the fraction of the strength of internal connections within the clusters compared to the external connections between clusters is optimized. This determines how the clusters within the network are finally defined. This step allowed us to identify subgroups of nodes that cluster together due to strong interconnectedness.
Results
Sample characteristics
Data of 1016 patients were included in the analyses. Overall, patients had an average premorbid IQ and a majority of the sample were of Caucasian ethnicity. On average, the sample consisted mostly of young and middle-aged adults and about half were male. The distribution of the primary diagnosis reflects the naturalistic patient population of the Amsterdam UMC, an expertise center for misophonia, early psychosis, anxiety and depressive disorders. Further results are presented in Table 2. Cognitive and psychopathology variable scores are shown in online Table S2 and CANTAB standard scores are shown in online Table S3 in the Supplementary Materials. Differences in cognitive and psychopathology variable scores per medication category are shown in online Table S4 in the Supplementary Materials. Except for alcohol use, cognitive flexibility, and alertness, there were significant differences in scores over types of medication, suggesting a possible influence of medication on cognitive and psychopathology variables. Generally, antidepressants, benzodiazepines, and sleep medication differed significantly and were related to worse symptomology. Furthermore, antipsychotic medication differed significantly and was related to worse cognitive function.
Table 2. Demographic and clinical characteristics of participants
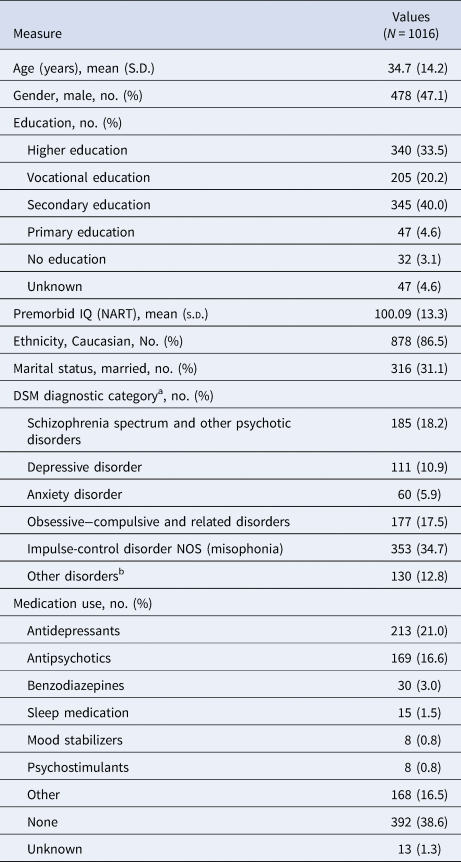
NART, Dutch National Adult Reading Test; DSM, Diagnostic and Statistical Manual of Mental Disorders; NOS, not otherwise specified.
a Diagnostic category is only for the primary diagnosis.
b Other disorder category includes: substance use disorders (n = 20), eating disorders (n = 5), neurodevelopmental disorders (n = 29), sexual disorders (n = 2), sleep disorders (n = 2), dissociative disorders (n = 1), adjustment disorders (n = 4), bipolar disorders (n = 28), and personality disorders (n = 12).
Network analysis
The network of the cognitive functioning and psychopathology domains is visualized in Fig. 1. Three clusters were detected, indicated by different colors in the figure which were labelled: general psychopathology symptoms (blue), substance use (yellow), and cognition (purple). A weights matrix of the network can be seen in online Table S5 in the Supplementary Materials. The stability analysis revealed that most of the edges in the network were stable, indicating that the estimated network was robust (online Fig. S4 in the Supplementary Materials). Variables with the highest strength were depression, anxiety, verbal memory, and visual attention.
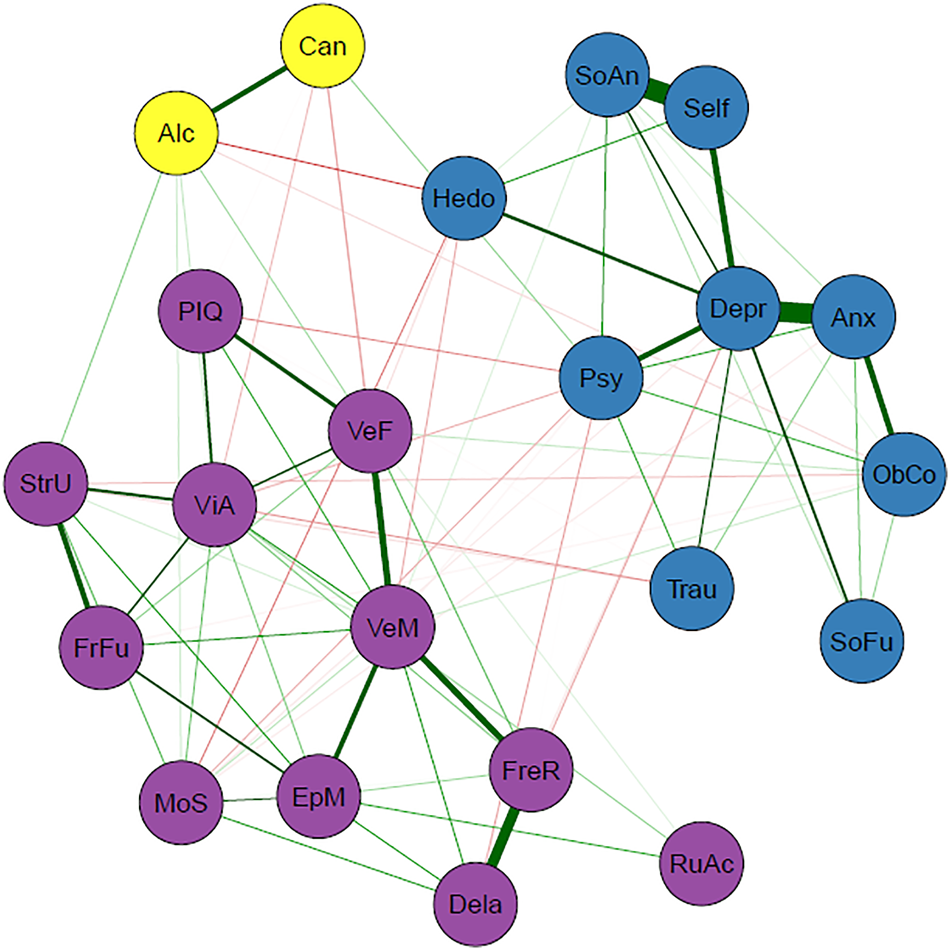
Fig. 1. Transdiagnostic network of cognitive and psychopathology domains (N = 1016). Nodes represent the variables included in the network and edges indicate an association between two nodes. Green edges represent positive associations whereas red edges represent negative associations, and thickness of an edge represents the strength of association between two nodes. The color of each node indicates to which cluster it belongs according to the EGA: cognition (purple), general psychopathology (blue), or substance use (yellow). Psychopathology domains: Alc = alcohol (ab)use (AUDIT); Anx = anxiety symptoms (HAM-A); Can = cannabis (ab)use (CUDIT); Depr = depressive symptoms (IDS); Hed = hedonism questionnaire; ObCo = obsessive−compulsive symptoms (Y-BOCS); Psy = (subclinical) psychotic experiences (PQ-16); Self = self-esteem (SERS-SF); SoAn = social anxiety symptoms (SIAS); SoFu = poor psychosocial functioning (WSAS); Trau = post-traumatic stress symptoms (IES-R). Cognitive domains: Dela = verbal recognition memory-delayed (VRM); EpM = episodic memory and learning (PAL); FreR = verbal recognition memory-immediate (VRM); FrFu = planning test (OTS); MoS = alertness and motor speed (CRT); PIQ = premorbid IQ (NART); RuAc = rule acquisition and attentional set shifting, cognitive flexibility (IED); StrU = strategy use (SWM); VeF = verbal fluency (GIT); VeM = verbal memory (CVLT); ViA = sustained visual attention and processing speed (RVP).
As expected, within-cluster edges between psychopathology and within-cluster edges between cognitive domain were mostly positive. Cross-cluster edges between cognitive domains and psychopathology contained mostly negative edges, in which increased symptom severity was associated with worse cognitive functioning. The total strengths of edges within clusters are notably higher than the total strength of edges between clusters, which is in line with our expectations because nodes cluster together due to strong interconnectedness. An overview of the edges in the network can be seen in Table 3. Closer inspection of the edges between cognitive domains and psychopathology showed that cognitive nodes have both positive and negative associations with different psychopathology nodes, whereas psychopathology nodes have either positive or negative associations with different cognitive nodes (online Table S6 in the Supplementary Materials). The obsessive−compulsive symptoms node was the only exception. Furthermore, (subclinical) psychotic experiences, cannabis use, and anhedonia had the strongest total negative relationships with cognitive variables while alcohol use had the strongest total positive relationship with cognitive variables (online Table S6 in the Supplementary Materials).
Table 3. Overview of the edges in the networka

a First three columns are the number of edges, whereas the last three columns are the sums of the strengths of the edges. Rows indicate the set of edges under consideration.
b Substance use nodes are included.
To further assess the stability of the cluster detection in our analyses, we performed a bootstrap analysis of the EGA procedure for all networks, including the control networks. This analysis revealed that amongst all the networks, the original network exhibited the most stable cluster structure (online Table S7 in the Supplementary Materials). Stability checks also demonstrated that networks were stable despite changes in sample size, indicating that sample size did not largely influence this (online Fig. S5 in the Supplementary Materials).
Lastly, further network analyses were conducted as controls: one using binarized data to control for differences in measurement methods and scales and others to account for sample variation in the variables based on diagnostic category, age, sex, education level, and medication use (online Appendices 1 and 2 in the Supplementary Materials). All the control networks reproduced the original three-cluster network, except for one diagnostic network (without depressive disorders) and the without medication network, which produced four clusters. In both of these networks (without depressive disorders and without medication), the psychopathology cluster was split into two separate clusters. The separation of cognitive and psychopathological variables remained in all control networks (online Figs S6–S14 in the Supplementary Materials).
Discussion
The present study used a network approach to investigate how domains of cognitive functioning and psychopathology cluster interact in a large transdiagnostic sample of patients with psychiatric disorders. This resulted in a fully connected network, showing that the domains are all closely associated. Cluster analysis detected three clusters in the network, labelled: cognition, general psychopathology, and substance use.
Cognitive functioning and psychopathology each form their own clusters, indicating that they are independent but related dimensions. The edges between the cognition and psychopathology clusters were relatively weak, which emphasizes their independence and further supports that cognitive function should be investigated in addition to and separately from psychiatric symptoms. Nonetheless, any associations, even if weak, may still be an important part of the etiology. The separation of these clusters also held across the control networks that were conducted to check the possible influence of diagnostic categories, suggesting that this interaction between cognitive domains and psychopathology domains is transdiagnostic and does not adhere to traditional diagnostic boundaries.
The formation of the separate clusters is in line with the hot−cold cognitive model in depression (Ahern et al., Reference Ahern, Bockting and Semkovska2019; De Raedt & Koster, Reference De Raedt and Koster2010; Roiser & Sahakian, Reference Roiser and Sahakian2013), in which ‘cold’ cognition is information processing without emotional influence (e.g. attentional control) and ‘hot’ cognition is information processing with emotional influence (e.g. mood-congruent attentional bias). The hot−cold cognitive model posits that non-affective cold cognitive dysfunctions contributes to the development of hot cognition (e.g. negative/catastrophic thoughts/beliefs), subsequently leading to psychiatric symptoms and further exacerbating cold cognitive dysfunctions. While we did not specifically measure hot cognition, it could be theorized that the psychiatric symptoms in our sample could also arise from cold cognitive deficits through hot cognition. Future research could investigate whether hot cognition acts as a bridge between cold cognition and symptoms. This model could be extended to other disorders as cognitive−affective processes are transdiagnostic (Iosifescu, Reference Iosifescu2012; Mansell, Harvey, Watkins, & Shafran, Reference Mansell, Harvey, Watkins and Shafran2008). Although temporal relationships cannot be assessed in the current study, this also supports our result regarding the association between worse cognitive functioning and higher symptom severity.
Another possibility for the separate clusters is that cognitive functioning and psychopathology follow different clinical trajectories, as suggested by findings of premorbid and persisting cognitive deficits despite symptom remission (Allott, Fisher, Amminger, Goodall, & Hetrick, Reference Allott, Fisher, Amminger, Goodall and Hetrick2016; Balanzá-Martínez et al., Reference Balanzá-Martínez, Tabarés-Seisdedos, Selva-Vera, Martínez-Arán, Torrent, Salazar-Fraile and Gómez-Beneyto2005; Caspi et al., Reference Caspi, Reichenberg, Weiser, Rabinowitz, Kaplan, Knobler and Davidson2003; Semkovska et al., Reference Semkovska, Quinlivan, O'Grady, Johnson, Collins, O'Connor and Gload2019). This could further tie in with the hot−cold cognitive model, in which premorbid cognitive deficits lead to psychiatric symptoms, and explain how cognitive functioning and psychopathology are related despite different trajectories.
Within the general psychopathology cluster, self-esteem, depressed mood, and (social) anxiety were strongly related. Low self-esteem can be considered a general transdiagnostic risk and maintenance factor in psychopathology (Zeigler-Hill, Reference Zeigler-Hill2011) and is often related to depression and anxiety (Sowislo & Orth, Reference Sowislo and Orth2013). The depressive symptoms node had the highest number of edges and highest total edge strength within the psychopathology cluster, meaning that it had the most relationships with other psychopathology domains. Depressive symptoms are often reported in patients with a variety of other disorders, such as subclinical psychosis, bipolar disorder, and obsessive−compulsive disorders (Fusar-Poli, Nelson, Valmaggia, Yung, & McGuire, Reference Fusar-Poli, Nelson, Valmaggia, Yung and McGuire2012; Goldberg & Fawcett, Reference Goldberg and Fawcett2012; Quarantini et al., Reference Quarantini, Torres, Sampaio, Fossaluza, Mathis, do Rosário and Koenen2011). Depressive symptoms could be considered a normal response to living with a psychiatric disorder, which can induce lowered self-esteem (Silverstone & Salsali, Reference Silverstone and Salsali2003), (self)stigma, and reduced possibilities in life. Successful treatment of the psychiatric disorder may subsequently reduce the depressive symptoms. There is also the possibility of bidirectional relationships between depressive symptoms and other clinical factors; however, this cannot be inferred with the current undirected network.
The substance use cluster is composed of the AUDIT and CUDIT. The formation of this cluster may have been influenced by the fact that the scales measure closely related constructs and are highly similar in form (i.e. the CUDIT was developed from the AUDIT) and/or because of the low prevalence of cannabis and alcohol use (i.e. a high number of individuals scored zeros on both scales). These possibilities were controlled for and the results led us to conclude that the original three clusters represent the most reliable clusters. This suggests substance use is related to the general psychopathology cluster but exhibits a certain independence at the same time. The general psychopathology cluster mostly contains affective symptoms, and while affective symptoms and substance (ab)use are related (Boden & Fergusson, Reference Boden and Fergusson2011; Kedzior & Laeber, Reference Kedzior and Laeber2014), they are nonetheless different psychometrically and conceptually (emotions v. behavior) and fall under different spectra (internalizing v. externalizing; Kotov et al., Reference Kotov, Krueger, Watson, Achenbach, Althoff, Bagby and Clark2017).
Within the cognition cluster, the verbal memory node had the highest number of edges and the highest total edge strength, indicating that verbal memory was related to many other cognitive domains. Verbal memory had the strongest association with verbal fluency. Interestingly, verbal memory has been shown to be predictive of remission for at-risk mental states individuals (Simon et al., Reference Simon, Grädel, Cattapan-Ludewig, Gruber, Ballinari, Roth and Umbricht2012) and treatment response for PTSD (Scott et al., Reference Scott, Harb, Brownlow, Greene, Gur and Ross2017) and comorbid depression−anxiety (Braund, Tillman, Palmer, & Harris, Reference Braund, Tillman, Palmer and Harris2020). This may suggest that it could be beneficial to improve verbal memory deficits in patients with psychiatric disorders to improve treatment response and increase the chance of remission. Many therapies are verbal and verbal memory deficits may hamper therapeutic success because patients have more difficulty remembering the content of their therapy sessions.
Depression, anxiety, verbal memory, and visual attention have the highest strength in the network, implying that they play a central role in psychiatric disorders. These nodes have a strong influence on other nodes, and could have a significant impact on overarching psychopathology. A common factor here may be stress, which plays a role in the development and maintenance of many disorders (Conway, Raposa, Hammen, & Brennan, Reference Conway, Raposa, Hammen and Brennan2018; Nolen-Hoeksema & Watkins, Reference Nolen-Hoeksema and Watkins2011). Dysregulation of the central stress response system, the hypothalamic−pituitary−adrenal (HPA) axis, can lead to memory deficits (Wingenfeld & Wolf, Reference Wingenfeld, Wolf, Szabo and Hennerici2014). Stress is also implicated in the etiology of emotional disturbances (McLaughlin, Conron, Koenen, & Gilman, Reference McLaughlin, Conron, Koenen and Gilman2010; Pechtel & Pizzagalli, Reference Pechtel and Pizzagalli2011), including excess of negative emotion and distress, explaining the relative importance of depression and anxiety symptoms, which are often observed across psychiatric disorders (Kring, Reference Kring, Lewis, Haviland-Jones and Barret2008).
Attentional deficits are also evident in patients with psychiatric disorders transdiagnostically (Millan et al., Reference Millan, Agid, Brüne, Bullmore, Carter, Clayton and DeRubeis2012). In the network, visual attention displays the strongest associations with strategy use (working memory), verbal fluency, and planning ability, which is in line with the executive-attention framework (Buckholtz & Meyer-Lindenberg, Reference Buckholtz and Meyer-Lindenberg2012; Millan et al., Reference Millan, Agid, Brüne, Bullmore, Carter, Clayton and DeRubeis2012). Previous studies have shown that dorsolateral prefrontal−cingulate−parietal network underpins the executive-attention framework (Buckholtz & Meyer-Lindenberg, Reference Buckholtz and Meyer-Lindenberg2012; Millan et al., Reference Millan, Agid, Brüne, Bullmore, Carter, Clayton and DeRubeis2012). Atypical connectivity within this network is often found across disorders, which may contribute to deficits in working memory, attention, and cognitive control (Buckholtz & Meyer-Lindenberg, Reference Buckholtz and Meyer-Lindenberg2012). Furthermore, associations of the visual cortex with the frontoparietal and default mode networks have been implicated in information processing (e.g. attention; Chadick & Gazzaley, Reference Chadick and Gazzaley2011). In addition, hyperconnectivity between visual association cortex and both frontoparietal and default mode networks has been associated with a general liability for mental illness (i.e. p-factor; Elliott, Romer, Knodt, & Hariri, Reference Elliott, Romer, Knodt and Hariri2018). This could provide an underlying mechanism for the shared cognitive deficits that are present across disorders.
Interestingly, we found that nodes representing psychopathology tended to have consistent relationships with cognitive domains, while nodes representing cognitive domains had mixed relationships with psychopathology. For instance, depression was consistently related to worse functioning across cognitive domains. However, verbal memory functioning had different relationships to certain psychopathology domains: (subclinical) psychotic experiences were related to worse verbal memory, whereas obsessions and compulsions were related to better verbal memory. This encourages future research to focus on symptom-specific interactions with different cognitive domains, rather than investigating cognitive functioning within diagnostic categories. Research within diagnostic categories could also explain why clear findings on cognitive functioning in psychiatric diagnostic categories are hard to come by: specific symptoms and cognitive deficits may vary considerably within one diagnostic category, and patterns could emerge more clearly by investigating relationships between cognitive functioning and symptoms directly, rather than comparing them across categories.
Cannabis use, (subclinical) psychotic experiences, and anhedonia had the strongest overall negative association with cognitive functioning. Both (subclinical) psychotic-like experiences and cannabis use have been associated with cognitive dysfunction (Lindgren et al., Reference Lindgren, Manninen, Laajasalo, Mustonen, Kalska, Suvisaari and Therman2010; Volkow et al., Reference Volkow, Swanson, Evins, DeLisi, Meier, Gonzalez and Baler2016). The relation between anhedonia and worse cognitive functioning, specifically motor speed, verbal memory, and fluency, may be partly explained by decreased connectivity within reward-related brain regions (Felger et al., Reference Felger, Li, Haroon, Woolwine, Jung, Hu and Miller2015). Interestingly, psychosocial functioning was included in the symptom cluster and demonstrated no associations with cognitive functioning. This is somewhat unexpected because psychosocial functioning tends to be more strongly associated with cognitive functioning than with psychiatric symptoms (Depp et al., Reference Depp, Mausbach, Harmell, Savla, Bowie, Harvey and Patterson2012; McIntyre et al., Reference McIntyre, Cha, Soczynska, Woldeyohannes, Gallaugher, Kudlow and Baskaran2013; Shamsi et al., Reference Shamsi, Lau, Lencz, Burdick, DeRosse, Brenner and Malhotra2011), although associations with psychopathology, such as depression (Fried & Nesse, Reference Fried and Nesse2014) have been reported.
The main strengths of the current analysis are the inclusion of various cognitive and psychopathology domains and the large transdiagnostic sample. This means that the results may be generalizable to patients with a wide range of psychiatric disorders. The main limitation of the current study is the cross-sectional design, meaning that neither causal interactions nor the direction of relationships can be assessed. Using cross-sectional data is a problem in most network papers published so far, and the results must be interpreted with caution (Guloksuz, Pries, & Van Os, Reference Guloksuz, Pries and Van Os2017). However, cross-sectional networks could still give insight into the co-occurrence of symptoms (Bos et al., Reference Bos, Snippe, de Vos, Hartmann, Simons, van der Krieke and Wichers2017). The Across study is ongoing; hence, future analyses will entail longitudinal data and biological markers. Another limitation is that sum scores of questionnaires were used. This was done to reduce the number of nodes in the network at the expense of losing information about relationships between individual items. Questionnaires also had differences in scoring and scaling properties, which could have influenced the pattern of edges and clusters detected. Most questionnaires were also self-reported, introducing a possible interpretation and self-report bias. However, the self-report questionnaires that were used are psychometrically valid and provide important information from the patients’ perspective. Additionally, due to the naturalistic character of our sample and the fact that our department does not specialize in treatment for these patient groups, some diagnostic groups were relatively underrepresented in our sample (e.g. substance use and neurodevelopmental disorders) and were therefore grouped together in an ‘Other disorders’ category.
Furthermore, the lack of a healthy control group is a limitation in the paper. Although we attempt to use a dimensional approach when assessing the psychiatric domains included in this study, we cannot capture the entire continuum without a healthy control or general population group. Due to a lack of a well-matched healthy control group, it is not clear to what extent cognitive deficits are present and whether these deficits are clinically relevant. A further limitation is that the network was not adjusted for medication use, which can impact cognitive function. This is, however, a naturalistic study of patients with psychiatric disorders, reflecting clinical reality of medication use. We would also like to note that there is probably an interaction effect between the disorder, clinical severity, and medication use, which would make it difficult to disentangle the effect of medication and the effect of the disorder on cognitive functioning.
Moreover, while the use of a transdiagnostic approach is a strength, we recognize the potential shortcoming of not analyzing specific relationships across diagnostic categories. However, control networks with diagnostic categories removed demonstrated that the structure of network is fairly robust to potential differences between subgroups. Finally, labelling of clusters is, as always with such techniques, rather subjective. Altogether, this study should be seen as a first investigation of the structure of the relationship between psychopathology and cognitive functioning. Future exploration can focus on parts of the network to investigate how specific items relate to each other and to cognitive measures.
The results of this study support the notion that cognitive functioning and psychopathology are independent but related dimensions, which interact in a transdiagnostic manner. Thus, it cannot be assumed that treating symptoms will alleviate cognitive deficits, and future studies should specifically assess if typical treatments influence cognitive dysfunction. Cognitive deficits, however, are usually undertreated, suggesting a need for treatments specifically targeting cognitive dysfunction in patients with psychiatric disorders, regardless of diagnosis. Interventions for cognitive dysfunction, such as cognitive remediation, which tend to be heavily geared towards patients with schizophrenia or bipolar disorders, should perhaps be considered transdiagnostic. Furthermore, depression, anxiety, verbal memory, and visual attention seem to play central roles across disorders and should therefore be the focus of transdiagnostic research and treatment.
Supplementary material
The supplementary material for this article can be found at https://doi.org/10.1017/S0033291721001781
Acknowledgement
We would like to thank all the participants for partaking in the study and all psychiatry staff responsible for recruitment and data collection.
Financial support
This research received no specific grant from any funding agency, commercial or not-for-profit sectors. KJHV is supported by the Foundation Volksbond Rotterdam. JTWW is supported by a Netherlands Organization for Scientific Research (NWO) Veni grant no. 016.156.019.
Conflict of interest
The authors declare no competing interests.
Ethical standards
The authors assert that all procedures contributing to this work comply with the ethical standards of the relevant national and institutional committees on human experimentation and with the Helsinki Declaration of 1975, as revised in 2008.