Introduction
This is a companion article to our recent review of biomarkers related to the stress response to environmental perturbations in dairy animals (Almeida et al., Reference Almeida, Zachut, Hernández-Castellano, Šperanda, Gabai and Mobasheri2019), and deals with biomarkers related to metabolic health and productivity, including fertility.
Most of the milk and dairy derivatives consumed in the developed world are produced in intensive production systems. Such systems are typically based on one breed: the Holstein Friesian dairy cow. The yields for this breed have been increasing steadily for the last 70 years, reaching up to average yields of 20 000 kg milk/per 305-d lactation Such extraordinary increases were obtained mainly thanks to advances in genetics, nutrition and feeding, reproductive management, artificial insemination, health management, environmental control and milking parlour design and efficiency: Roche et al. (Reference Roche, Berry, Bryant, Burke, Butler, Dillon, Donaghy, Horan, Macdonald and Macmillan2017), Douphrate et al. (Reference Douphrate, Hagevoort, Nonnenmann, Lunner Kolstrup, Reynolds, Jakob and Kinsel2013) and Duncan et al. (Reference Duncan, Teufel, Mekonnen, Singh, Bitew and Gebremedhin2013). Conversely, in tropical countries, different dairy species and breeds are raised, particularly water buffalo (Bubalus bubalis) and Bos indicus cattle. In addition, these productions systems are not as intensified as those in temperate countries. Furthermore, small ruminants (sheep and goats) and dromedaries also contribute to the total amount of milk produced worldwide (Medhammar et al., Reference Medhammar, Wijesinha-Bettoni, Stadlmayr, Nilsson, Charrondiere and Burlingame2012). Despite being a small proportion of the world's dairy output, this sector has been growing steadily, particularly in industrialized countries (Pulina et al., Reference Pulina, Milán, Lavín, Theodoridis, Morin, Capote, Thomas, Francesconi and Caja2018). For more information, refer to the positioning paper by Hernández-Castellano et al. (Reference Hernández-Castellano, Nally, Lindahl, Wanapat, Alhidary, Fangueiro, Grace, Ratto, Bambou and de Almeida2019).
The dairy sector is facing numerous challenges (Baumgard et al., Reference Baumgard, Collier and Bauman2017; Boor et al., Reference Boor, Wiedmann, Murphy and Alcaine2017; Martin et al., Reference Martin, Russelle, Powell, Sniffen, Smith, Tricarico and Grant2017; McGuffey, Reference McGuffey2017; Polsky and von Keyserlingk, Reference Polsky and von Keyserlingk2017; Tan et al., Reference Tan, Bian, Yang, Li, Wu and Hu2017). On the one hand, it is vital to keep improving and optimizing production levels, particularly in the classical areas of animal production (nutrition, lactation physiology, reproductive biology and health management) as well as in relation to dairy farm facilities and milking parlour design and automation. On the other hand, such intensification processes have led to several undesirable aspects that are frequently associated with dairy production, such as perceived sustainability issues and environmental pollution. We shall not enter into that debate in this review, but we do recognize its importance. Another consequence of intensification is increased occurrence of several metabolic diseases that were not common in the past. Metabolic diseases in cattle and other domestic animals are a key feature of domestication that not only affects the animals' health and productivity, but also has negative consequences on human consumers through elevated use of drugs and antibiotics (Raboisson et al., Reference Raboisson, Barbier and Maigné2016). Such metabolic diseases include, for instance, ruminal acidosis, mastitis, ketosis and laminitis, among others. Reducing the occurrence of these diseases will be a major research area in the near future. Another aspect of dairy production concerns animal welfare and how this is compromised by intensification. This is a topic of growing importance for consumers, who place particular emphasis on animal transport, reduced individual space in barns, separation of dam and calf and the increased occurrence of the aforementioned metabolic diseases. These will also be aspects of growing economical and ethical importance for the dairy sector in the coming years. Therefore, finding and establishing proper biomarkers of welfare will be of great interest in the near future.
The term ‘animal fitness’ refers to the ability of one individual, relative to others, to leave viable offspring. Over the last few decades, dairy cow breeding programmes have focused mainly on characters and traits related to increased milk yield with negative consequences for cow fitness (Essl, Reference Essl1998). Further aspects about cow fitness can be found in our companion paper (Almeida et al., Reference Almeida, Zachut, Hernández-Castellano, Šperanda, Gabai and Mobasheri2019). Quantitative evaluations of fitness traits such as lameness and mastitis resistance, calving interval and lifespan show that, because of antagonistic relationships between production and these fitness traits, a trade-off may exist between the costs of lower milk yield and the benefits of better cow health status (Koolhaas and van Rennen, Reference Koolhaas and van Reenen2016). Therefore, identifying specific biomarkers related to fitness is of great interest to ensure optimal fitness in modern dairy cows
Based on these observations, the challenge for dairy researchers is to establish well-based, empiric and quantifiable biomarkers for metabolic diseases, welfare, fitness and wellbeing in dairy cows, and this challenge is the focus of the present review (Fig. 1).
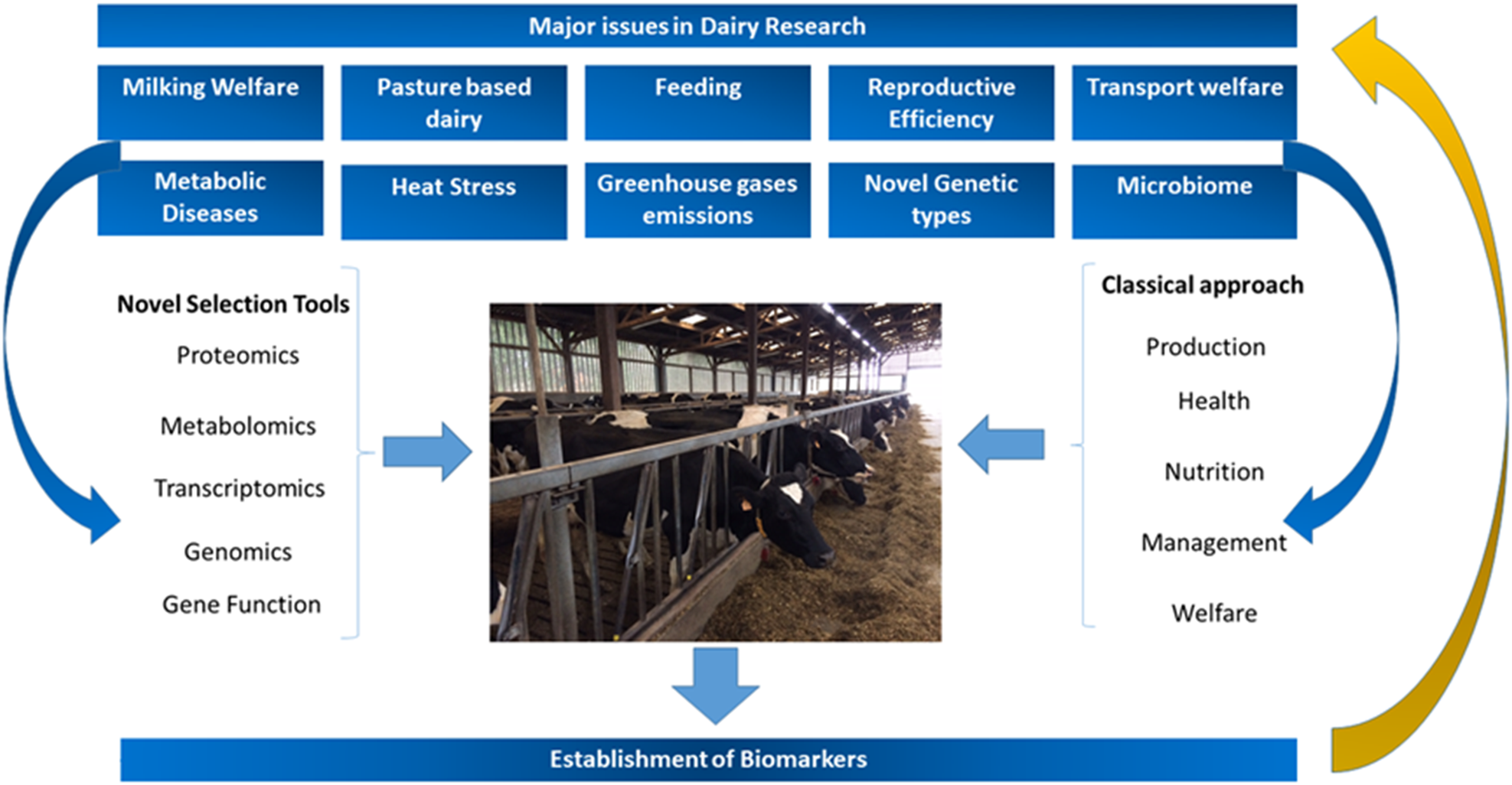
Fig. 1. Major challenges and areas of research in modern dairy production systems and how to address them, highlighting the importance of classical and novel selection tools as well as the establishment of biomarkers.
Biomarkers of energy balance and oxidative stress
Due to the sudden demand of energy for milk production, the transition from late pregnancy to lactation represents an important metabolic challenge for modern dairy cows. During this period, energy intake does not meet energy requirements for body maintenance and milk production, which results in negative energy balance (NEB; Bell and Bauman, Reference Bell and Bauman1997; Drackley, Reference Drackley1999) and high adipose tissue mobilization. If adaptation to NEB fails, the risk of metabolic disorders such as ketosis, hypocalcaemia, fatty liver mastitis and others increases considerably. In addition, NEB is related to lower conception rates, early embryonic mortality, and silent oestrus in high yielding dairy cows.
Traditional ways for detecting or preventing NEB are based on blood metabolites (i.e. non-esterified fatty acids, NEFA), and body condition score (BCS; a subjective score of body fattening), all of which require complicated data collection (individual feed intake and body weight), or invasive and laborious blood collection, along with trained staff. Hence, there is a need for accurate, objective and preferably non-invasive biomarkers that indicate energy status in dairy cows postpartum. In this section, potential biomarkers of NEB in blood and milk of dairy cows will be discussed. These are by far the most important media for current analytical approaches, but it is worth pointing out that other tissues and/or fluids might also prove to have value in the future, for instance, a recent report has examined possible metabolic biomarkers in hair samples (Möller et al., Reference Möller, Dannenberger, Nürnberg, Strucken and Brockmann2019).
Biomarkers in blood for negative energy balance diagnosis
Non-esterified fatty acids (NEFA) and β-hydroxybutyrate (BHBA) are probably the most-known blood parameters used to asses NEB in dairy cows. Blood NEFA concentrations reflect the extent of fat mobilization, while BHBA indicates fat oxidation in the liver. Therefore, both analytes have been extensively used in the field as indicators of NEB (McArt et al., Reference McArt, Nydam, Oetzel, Overton and Ospina2013; Ospina et al., Reference Ospina, McArt, Overton, Stokol and Nydam2013). Elevated concentrations of NEFA and BHBA in blood have been shown to be associated with reduced milk yield (Duffield et al., Reference Duffield, Lissemore, Mcbride and Leslie2009; Ospina et al., Reference Ospina, Nydam, Stokol and Overton2010; Chapinal et al., Reference Chapinal, LeBlanc, Carson, Leslie, Godden, Capel, Santos, Overton and Duffield2012),and impaired periparturient immunity and increased risk of infectious diseases (Moyes et al., Reference Moyes, Larsen, Friggens, Drackley and Ingvartsen2009; Ospina et al., Reference Ospina, Nydam, Stokol and Overton2010). NEFA concentrations higher than 0.3 and 0.6 mmol/l pre- and postpartum, respectively, are associated with an increased risk of displaced abomasum, clinical ketosis, retained placenta and metritis (Ospina et al., Reference Ospina, Nydam, Stokol and Overton2010).
In addition to NEFA, specific fatty acids (FA) in blood could be used as potential and alternative biomarkers for NEB in transition cows. Imhasly et al. (Reference Imhasly, Bieli, Naegeli, Nyström, Ruetten and Gerspach2015) examined changes in the blood plasma lipidome in transition dairy cows and found that the levels of a number of triacylglycerides (TGs) were higher prepartum than postpartum: TG 48:3, TG 48:1, TG 49:2, TG 49:1, TG 50:4, TG 50:3, TG 50:2, TG 51:3, TG 51:2, TG 51:1, TG 52:4, TG 52:3, TG 53:3, TG 54:6 and TG 56:6. In addition, the levels of two fatty acid amides (i.e. linoleamide and anandamide) decreased only at calving (Imhasly et al., Reference Imhasly, Bieli, Naegeli, Nyström, Ruetten and Gerspach2015), which suggests enhanced energy requirement postpartum. In contrast, the levels of lyso-phosphatidylcholine (LPC) and phosphatidylcholine (PC), specifically: LPC 16:0, LPC 18:3, LPC 18:2, LPC 18:1, LPC 20:5, PC P-34:2, PC P-36:5, PC P-36:4 and PC 36:6, as well as the sphingomyelins39:1 and 43:3 were increased postpartum (Imhasly et al., Reference Imhasly, Bieli, Naegeli, Nyström, Ruetten and Gerspach2015). However, in this study the relationship between these lipids and individual NEB was not analysed. Therefore, it is not clear whether NEB might alter the blood lipidome in cows.
In postpartum cows that are in NEB, increased inflammatory markers are found in plasma, such as tumour necrotizing factor alpha, the acute phase proteins haptoglobin, serum amyloid A and others (Bradford et al., Reference Bradford, Yuan, Farney, Mamedova and Carpenter2015). These inflammatory markers can also be used as indicators of the degree of NEB, since cows with severe NEB have a higher degree of systemic inflammation. This topic is reviewed thoroughly in Bradford et al. (Reference Bradford, Yuan, Farney, Mamedova and Carpenter2015).
Biomarkers in milk for negative energy balance diagnosis
The potential of milk biomarkers for NEB diagnosis is enormous, as sensors for these specific biomarkers could be implemented in milking parlours and milking robots to provide individual information about energy status in cows. BHBA, for instance, can be measured in milk, and has the potential to be measured frequently in individual cows in early lactation as an indicator of NEB (Duplessis et al., Reference Duplessis, Santschi, Plante, Bergeron, Lefebvre, Durocher and Cue2019).
Fatty acids
Milk FA may be used as biomarkers of EB in dairy cows. Milk FA are derived from four major pathways (1) directly from the diet, (2) de novo synthesis in the mammary gland, (3) formation in the rumen by biohydrogenation or bacterial degradation, and (4) fat depots (Stoop et al., Reference Stoop, Bovenhuis, Heck and van Arendonk2009). Changes in energy status across lactation also imply changes in milk FA composition (Gross et al., Reference Gross, van Dorland, Bruckmaier and Schwarz2011). In cows under NEB, the de novo synthesis of fatty acids by the mammary gland (i.e. C6:0 to C14:0) is reduced in favour of increased body fat mobilization (van Knegsel et al., Reference van Knegsel, van den Brand, Dijkstra, Tamminga and Kemp2005). Indeed, under severe negative EB, short-chain and medium-chain FA concentrations in milk are reduced, while long-chain FA concentrations are increased (Nogalski et al., Reference Nogalski, Wroski, Sobczuk-Szul, Mochol and Pogorzelska2012). This can be explained by the fact that oleic acid (C18:1-cis9) is the predominant FA in adipocytes, and it is released primarily through lipolysis during NEB (Rukkwamsuk et al., Reference Rukkwamsuk, Geelen, Kruip and Wensing2000). Actually, Gross et al. (Reference Gross, van Dorland, Bruckmaier and Schwarz2011) found a correlation between NEB and the proportion of C18:1-cis9 in milk (r 2 = 0.77). Therefore, the proportion of this fatty acid could be used as a biomarker for EB diagnosis in dairy cows.
Glucose
Glucose is another possible biomarker for NEB diagnosis. Glucose is an essential metabolite for the mammary epithelial cells. Mammary epithelial cells do not synthesize glucose because they lack the enzyme glucose-6-phosphatase (Scott et al., Reference Scott, Beuman and Clark1976). Therefore, glucose concentration in mammary epithelial cells depends on the glucose transferred from blood. Consequently, glucose concentrations in milk reflect its concentration in the mammary epithelial cell cytoplasm (Faulkner et al., Reference Faulkner, Chaiyabutr, Peaker, Carrick and Kuhn1981; Zhao, Reference Zhao2014). Glucose-6-phosphate (G6P) is a central metabolite in the glycolytic pathway as it is an intermediate compound during lactose synthesis and participates in the first step for glycolysis and the pentose phosphate pathway (PPP; Zhao, Reference Zhao2014). On this basis, G6P has been also proposed as a biomarker for NEB diagnosis. Larsen and Moyes (Reference Larsen and Moyes2015) analysed 3200 milk samples from Holstein and Jersey cows for free glucose and G6P. During the first 21 weeks of lactation, free glucose concentrations increased whereas G6P concentrations in milk decreased. Accordingly, Zachut et al. (Reference Zachut, Kra, Portnik, Shapiro and Silanikove2016) reported that milk glucose concentrations were positively correlated to days in lactation. In contrast, the average concentration of G6P in the milk was the highest during the first week of lactation, and was negatively correlated to days in lactation (Zachut et al., Reference Zachut, Kra, Portnik, Shapiro and Silanikove2016). Therefore, milk G6P/glucose ratio was suggested as a biomarker of the oxidative stress in the mammary epithelial cells (Zachut et al., Reference Zachut, Kra, Portnik, Shapiro and Silanikove2016). In addition, that study showed that G6P/glucose ratio was highly correlated to plasma NEFA concentrations (r 2 = 0.81, Zachut et al. Reference Zachut, Kra, Portnik, Shapiro and Silanikove2016). More research is required to validate the use of free glucose and G6P as biomarkers of EB, which may potentially be used in the future for in-line surveillance systems on-farm.
Glycolytic enzymes
Based on the relation between milk G6P and EB, glucose-6-phosphate-dehydrogenase (G6PDH), the first enzyme in the pentose phosphate pathway, has been also suggested as a milk biomarker for NEB diagnosis in dairy cows. Only a few studies have reported G6PDH activity in milk from cows. Similar to milk G6P concentrations, Zachut et al. (Reference Zachut, Kra, Portnik, Shapiro and Silanikove2016) showed that milk G6PDH activity in cows was highest on the first and second week of lactation, the decreasing until the fifth week of lactation. Moreover, G6PDH activity was correlated with milk G6P (r 2 = 0.68), with G6P/glucose ratio (r 2 = 0.53) and negatively correlated to days in lactation (r 2 = −0.69), dry matter intake (r 2 = −0.65) and EB (r 2 = −0.52; Zachut et al. Reference Zachut, Kra, Portnik, Shapiro and Silanikove2016). It was observed that milk G6PDH activity was found to be 2-fold higher in cows that were under NEB on the third week of lactation compared to those under positive energy balance (unpublished data). Based on these findings, G6PDH activity could be used as a biomarker in milk for NEB diagnosis in early lactation cows, however, more research is required to validate this biomarker.
Biomarkers of oxidative stress in blood and milk
Oxidative stress is affected during NEB as a consequence of the pro-inflammatory effects of fat mobilization (Sordillo et al., Reference Sordillo, Contreras and Aitken2009; Bradford et al., Reference Bradford, Yuan, Farney, Mamedova and Carpenter2015). The metabolic demands associated with late pregnancy, parturition and initiation of lactation are thought to increase the production of reactive oxygen species (ROS) (Esposito et al., Reference Esposito, Irons, Webb and Chapwanya2014). The bulk of the oxidants are ROS, but reactive nitrogen species (RNS) contribute to the pools of oxidants and both are produced during physiological and pathological states in the organism. All macromolecules like lipids, proteins and DNA are targets for oxidative stress (Mavangira et Sordillo, Reference Mavangira and Sordillo2018). There are a lot of useful biomarkers of oxidative stress connected to lipid and protein metabolism, the most known biomarker of protein oxidation being the measurement of carbonyl groups. When ROS attack the amino acid side chains of proline, arginine, lysine and threonine, carbonyl groups are generated. A more specific parameter for protein oxidation is 2,4-dinitrophenylhydrazone (DNPH) which allows the determination of total protein carbonyl groups (Mavangira et Sordillo, Reference Mavangira and Sordillo2018). Hypochlorous acid-induced products are generated during inflammation (Shacter, Reference Shacter2000). The level of dityrosine reflects the oxidative damage of proteins and measurement is possible by chemiluminescence (Bordignon et al., Reference Bordignon, Da DAlt, Marinelli and Gabai2014). Under transitional stages cows are exposed to different oxidative processes which can compromise antioxidative status: parturition, NEB, ketosis risk, fertilization, early embryo development, concurrent pregnancy and lactation, inflammation in connection with the udder (subclinical mastitis) and uterus (subclinical endometritis), gut health etc. It has been observed that the ratio between the plasma level of advanced oxidation protein products and albumin (AOPP/albumin) is a sensitive indicator of oxidative stress (Celi et al., Reference Celi, Merlo, Da Dalt, Stefani, Barbato and Gabai2011). Because of the lack of antioxidants in maize silage, feeding cows with maize silage increase AOPP concentration (Celi and Raadsma, Reference Celli and Raadsma2010). Immune cells are particularly sensitive to oxidative stress due to the high content of polyunsaturated FA present in the cellular membrane, which are susceptible to peroxidation, increasing the production of ROS (Spears and Weiss, Reference Spears and Weiss2008; Esposito et al., Reference Esposito, Irons, Webb and Chapwanya2014, Celi and Gabai, Reference Celi and Gabai2015). Recently, Alharthi et al. (Reference Alharthi, Zhou, Lopreiato, Trevisi and Loor2018) reported a gradual increase in reactive oxygen metabolites (ROM) between −10 and 20 d relative to parturition, and a previous report demonstrated that cows losing more BCS, which is indicative of NEB, had lower superoxide dismutase (SOD) activity and higher ROM in the bloodstream (Bernabucci et al., Reference Bernabucci, Ronchi, Lacetera and Nardone2005). Also, a significant correlation between milk AOPP and somatic cells count has been observed (Guzzo et al., Reference Guzzo, Balloni, Mantovani, Da Dalt and Gabai2015). Therefore, it seems that ROM and SOD activity in blood may be used as biomarkers of oxidative stress in dairy cows. The widely used biomarker of lipid peroxidation, malondialdehyde (MDA), a low-molecular-weight product created during the decomposition of polyunsaturated fatty acid (PUFA) may reflect the oxidative stress of the animal. In milk of PP cows, MDA concentration was found to be highest in early lactation and then exponentially decayed, and was inversely correlated with days in lactation (Zachut et al., Reference Zachut, Kra, Portnik, Shapiro and Silanikove2016). In agreement, milk anti-oxidative capacity (ORAC values) tended to be negatively and exponentially correlated with days in lactation (r 2 = −0.29) and EB (r 2 = −0.30), and to be positively and linearly correlated to milk G6P (r 2 = 0.25) (Zachut et al., Reference Zachut, Kra, Portnik, Shapiro and Silanikove2016). This suggests that milk MDA and ORAC can serve as biomarkers of oxidative stress in milk. However, MDA has been shown to be an inconsistent and variable marker (Celi, Reference Celi2010). A more reliable marker of lipid oxidation may be ELISA based isoprostanes, as increased levels of 15-F2-IsoP were determined during coliform mastitis (Mavangira et al., Reference Mavangira, Mangual, Gandy and Sordillo2016) and related to inflammation (Mavangira and Sordillo, Reference Mavangira and Sordillo2018).
A recent study demonstrated a positive correlation between reactive oxygen and nitrogen species (RONS) and oxidant status index (OSi, which is defined as the ratio between reactive oxygen and nitrogen species) and total antioxidant potential (r 2 = 0.75), as well as a negative correlation between OSi and serum antioxidant potential (AOP; r 2 = −0.58). An increase in the ratio indicates a higher risk for oxidative stress due to an increase in pro-oxidant production or defensive antioxidant depletion (Ling et al., Reference Ling, Hernández-Jover, Sordillo and Abuelo2018).
Environmental heat stress can increase oxidative stress in dairy cows. Bernabucci et al. (Reference Bernabucci, Ronchi, Lacetera and Nardone2002) reported that transition cows exposed to heat stress during summer had higher erythrocyte activity, glutathione peroxidase activity, intracellular thiols, and MDA compared to spring cows, indicating a condition of oxidative stress in the summer transitioning dairy cows. Plasma concentrations of the oxidative stress marker MDA were higher in transition dairy cows calving during summer heat stress compared to those calving in winter (Zachut et al., Reference Zachut, Kra, Livshitz, Portnick, Yakoby, Friedlander and Levin2017). In studies conducted in mid-lactation heat-stressed cows, a reduction in plasma antioxidant activity was found (Harmon et al., Reference Harmon, Lu, Trammel and Smith1997). Further research is required to establish the utility of oxidative stress as putative biomarkers of heat stress in cattle.
Consequences and applications
Biomarkers of production-related diseases
Dairy cows are one of the most intensively farmed animals worldwide. High-yielding dairy cows have been genetically selected for high milk production, which increases the susceptibility of these animals to develop certain diseases such as mastitis, hypocalcaemia, rumen acidosis, ketosis and laminitis. The establishment of biomarkers for early detection of these diseases is one of the most important aspects of current dairy research.
Mastitis, defined as the inflammation of the udder, is the most prevalent production-related disease in dairy herds worldwide. In dairy ruminants, mastitis is frequently caused by intra-mammary infections. Those infections often impact animal welfare and contribute to economic losses for farmers (Halasa et al., Reference Halasa, Huijps, Osteras and Hogeveen2007; Hernández-Castellano et al., Reference Hernández-Castellano, Torres, Alavoine, Ruiz-Diaz, Arguello, Capote and Castro2011). Currently, the most sensitive technique available for clinical and subclinical mastitis detection is SCC or somatic cell count (Schukken et al., Reference Schukken, Wilson, Welcome, Garrison-Tikofsky and Gonzalez2003), while the identification of pathogens requires bacteriological culture (Nyman et al., Reference Nyman, Persson Waller, Bennedsgaard, Larsen and Emanuelson2014) or molecular methods, namely PCR. Cow-side or point of care diagnostic tests for bacterial identification are becoming available, but are not yet in widespread use (Jones et al., Reference Jones, Bork, Ferguson and Bates2019). In addition to the innate immune response, represented by increasing SCC, immunoglobulins (mainly IgG) are important components of the specific immune response transferred from blood to milk during mastitis (Wall et al., Reference Wall, Hernández-Castellano, Ahmadpour, Bruckmaier and Wellnitz2016a, Reference Wall, Wellnitz, Hernández-Castellano, Ahmadpour and Bruckmaier2016b). The increase of IgG in milk appears to be pathogen-dependent and its use combined with SCC has been proposed for the prediction of the pathogen causing mastitis (Hernández-Castellano et al., Reference Hernández-Castellano, Wall, Stephan, Corti and Bruckmaier2017a). In-line IgG measurements are currently under development for use at farm level (Lemberskiy-Kuzin et al., Reference Lemberskiy-Kuzin, Lavie, Katz, Merin and Leitner2019), but the technology is not yet available to farmers. Therefore, alternative markers such as lactate dehydrogenase (LDH) and differential somatic cell count (DSCC) have been proposed as markers for early mastitis detection and diagnosis (Chagunda et al., Reference Chagunda, Friggens, Rasmussen and Larsen2006; Damm et al., Reference Damm, Holm, Blaabjerg, Bro and Schwarz2017; Wall et al., Reference Wall, Wellnitz, Bruckmaier and Schwarz2018).
The use of omics technologies in the field of mastitis in dairy cows has provided knowledge about diverse components involved in the course of the disease and how those components may be affected by the mastitis causing pathogen. For instance, Thomas et al. (Reference Thomas, Mudaliar, Tassi, McNeilly, Burchmore, Burgess, Herzyk, Zadoks and Eckersall2016a, Reference Thomas, Mullen, Tassi, Ramirez-Torres, Mudaliar, McNeilly, Zadoks, Burchmore and David Eckersall2016b) and Mudaliar et al. (Reference Mudaliar, Tassi, Thomas, McNeilly, Weidt, McLaughlin, Wilson, Burchmore, Herzyk, Eckersall and Zadoks2016) used peptidomics, metabolomics and quantitative proteomics to analyse milk from mastitis caused by Streptococcus uberis. These authors established several components such as casein derived peptides, peptides of glycosylation dependent cell adhesion molecule and serum amyloid A, antimicrobial peptides and different inflammation related metabolites. However, Kusebauch et al. (Reference Kusebauch, Hernández-Castellano, Bislev, Moritz, Rontved and Bendixen2018) described that gram-negative bacteria cause faster and more intense changes in the milk proteome compared to gram-positive bacteria. Based on this differential expression in milk, the authors proposed potential biomarkers to distinguish between mastitis caused by gram-negative and gram-positive bacteria. These biomarkers were α-2 macroglobulin, α-1 antitrypsin, haptoglobin, Serum Amyloid A3, cluster of differentiation CD14, calgranulin B, calgranulin C, cathepsin C, vanin-1, galectin-1, galectin-3 and interleukin 8. This field is growing all the time and it is likely that in the future it will extend to other species such as small ruminants (Katsafadou et al., Reference Katsafadou, Tsangaris, Billinis and Fthenakis2015; Hernández-Castellano et al., Reference Hernández-Castellano, Almeida, Renaut, Arguello and Castro2016a, Reference Hernández-Castellano, Ferreira, Nanni, Grossmann, Argüello, Capote, Cai, Lippolis, Castro and de Almeida2016b; Vasileiou et al., Reference Vasileiou, Chatzopoulos, Sarrou, Fragkou, Katsafadou, Mavrogianni, Petinaki and Fthenakis2019) and water buffalo (Patbandha et al., Reference Patbandha, Ravikala, Maharana, Marandi, Ahlawat and Gajbhiya2015). Different biomarkers may be more applicable in non-bovine species, for instance, cathelicidin has been proposed for use in goats (Tedde et al., Reference Tedde, Bronzo, Puggioni, Pollera, Casula, Curone, Moroni, Uzzau and Addis2019). The area has been recently reviewed by Almeida and Eckersall (Reference Almeida, Eckersall, de Almeida, Eckersall and Miller2018) and Boschetti et al. (Reference Boschetti, Hernández-Castellano and Righetti2019).
Metabolic diseases are usually linked to an increased demand for a specific nutrient that has become deficient under certain conditions. In the case of dairy cows, a special focus should be placed on the onset of lactation, when the sudden high demand of nutrients for milk production increases the metabolic load on the animal (Weaver et al., Reference Weaver, Prichard, Maerz, Prichard, Endres, Hernández-Castellano, Akins, Bruckmaier and Hernández2017). Most common metabolic diseases in dairy cows are hypocalcaemia (HC), ketosis (KT) and ruminal acidosis (RA). Hypocalcaemia mainly occurs at the onset of lactation when the fast and high demand for calcium by the mammary gland for milk production decreases circulating calcium concentrations below 1.4 mmol/l (clinical HC) or below 2 mmol/l (subclinical HC) (Hernández-Castellano et al., Reference Hernández-Castellano, Hernández, Weaver and Bruckmaier2017b). Similar to HC, KT occurs in cattle when energy demands exceed energy intake, resulting in negative energy balance (Zarrin et al., Reference Zarrin, De Matteis, Vernay, Wellnitz, van Dorland and Bruckmaier2013). In order to prevent KT, sufficient energy has to be provided through the feed. However, when these high dietary requirements for milk production are reached by feeding diets with high amounts of rapidly fermentable carbohydrates (i.e. starch) and low fibre content, bacterial populations are altered in the rumen. Consequently, acids (i.e. lactate) and glucose accumulate, decreasing ruminal pH (pH < 4.8) leading to RA (the animal is not able to restore pH levels itself) or subacute ruminal acidosis (SARA, the animal restores normal pH levels within hours). This metabolic disease damages the ruminal and intestinal wall and decreases blood pH, leading to the metabolic acidosis.
The rapid development of new sensor technologies has allowed the creation of tools that provide individual and dynamic information about the animals (Caja et al., Reference Caja, Castro-Costa and Knight2016). Additionally, innovations in robotics have provided opportunities to develop powerful systems for the individualized feeding of dairy cows according to their specific and singular nutritional and physiological requirements. The constant flow of information can be used to monitor dairy cows and therefore prevent these metabolic diseases in dairy herds, as highlighted below.
It is quite evident that blood calcium is the best indicator for HC diagnosis. However, several additional markers can be used to determine the calcium status in the animal and prevent HC. Urine pH could be used as indicator of the acid–base balance in blood (Thilsing-Hansen et al., Reference Thilsing-Hansen, Jorgensen and Ostergaard2002). Around parturition, it is convenient to create a physiological state of compensated systemic acidosis in cows by feeding diets with a negative dietary cation–anion difference. This acidosis will be compensated in part by bone calcium resorption (Lemann et al., Reference Lemann, Bushinsky and Hamm2003). Therefore, cows with urinary pH within 5.5 to 6.2 around parturition are considered to be in temporary acidosis, which is associated with reduced HC (Horst et al., Reference Horst, Goff, Reinhardt and Buxton1997). In the case of KT, BHB concentration in blood >1.4 mmol/l is the common marker used for KT diagnosis. However, other factors such as NEFA, glucose, glucagon or insulin are also affected during KT. Geishauser et al. (Reference Geishauser, Leslie, Kelton and Duffield1998) and Koeck et al. (Reference Koeck, Jamrozik, Schenkel, Moore, Lefebvre, Kelton and Miglior2014) showed how BHB measured in milk correlates with BHB measured in blood. Based on this principle, modern milking robots incorporate BHB measurements in milk, which provides constant information about the energy status of the animal, contributing to the prevention of KT. One of the most obvious markers for ruminal acidosis is the pH value of the ruminal fluid. Wireless pH probes located in ruminal boluses provide ruminal pH measurement in real time, but pH recording can differ depending on the compartment where the probe is placed (Neubauer et al., Reference Neubauer, Humer, Kroger, Braid, Wagner and Zebeli2018). Besides ruminal pH, other markers for detection of either RA or SARA can be measured in blood, urine, faeces or milk. In blood, d-lactate has been proposed as a marker for SARA (Larsen, Reference Larsen2017) as it is exclusively synthesized in the rumen by lactobacilli and bifidobacteria (Ewaschuk et al., Reference Ewaschuk, Naylor and Zello2005) and it is poorly metabolized by mammals. Consequently, d-lactate accumulates in body fluids such as milk and it could be used as a biomarker for RA diagnosis (Ewaschuk et al., Reference Ewaschuk, Naylor and Zello2005). In addition to d-lactate, Danscher et al. (Reference Danscher, Li, Andersen, Khafipour, Kristensen and Plaizier2015) also described that RA or SARA do not affect milk protein content, but do reduce fat content in milk compared to control cows (4.14 and 5.08%, respectively). Therefore, animals with fat:protein ratio below 1 in milk are susceptible to suffer RA or SARA (Danscher et al., Reference Danscher, Li, Andersen, Khafipour, Kristensen and Plaizier2015; Vlček et al., Reference Vlček, Žitný and Kasarda2016; Rojo-Gimeno et al., Reference Rojo-Gimeno, Fievez and Wauters2018).
Biomarkers of reproductive state
An efficient management of fertility requires a tight collaboration between farmers and veterinarians, a consistent analysis of the farm records and accurate clinical data. Moreover, reduced fertility can be considered an indicator of poor health and welfare (Walsh et al., Reference Walsh, Williams and Evans2011, Gabai et al. Reference Gabai, Amadori, Knight and Werling2018). Therefore, the development and continuous validation of specific biomarkers for fertility is relevant in dairy research.
To decide a suitable reproductive management strategy, several factors need to be monitored. The importance of oestrus detection on the reproductive efficiency is widely recognized, but the assessment of the resumption of ovarian activity and uterine health during the puerperium also needs to be considered. Pregnancy diagnosis should be performed as soon as possible after artificial insemination (AI), and conception failure should be discriminated from embryonic loss.
Progesterone indicates the presence of an active corpus luteum. Therefore, it has been used as a biomarker of reproduction efficiency for decades (Veronesi et al., Reference Veronesi, Gabai, Battocchio, Mollo, Soldano, Bono and Cairoli2002). As progesterone is transferred from blood to milk, milk progesterone is a suitable non-invasive biomarker in dairy animals for reproductive status (Xu et al., Reference Xu, Velasco-Garcia and Mottram2005, Reference Xu, Liang, Shi, Zhao, Liu, Wu, Song, Zhang and Wang2013; Kappel et al., Reference Kappel, Proll and Gauglitz2007; Posthuma-Trumpie et al., Reference Posthuma-Trumple, van Amerongen, Korf and van Berkel2009; Oku et al., Reference Oku, Osawa, Hirata, Kon, Akasaka, Senosy, Takahashi and Izaike2011), although measurement of progesterone is usually too expensive to be extensively applied over periods of several weeks in a large number of animals. Moreover, manual sampling is not practical in commercial farms, where large herds need to be monitored, and the use of automated sampling systems is needed.
As described above, oestrus detection is essential to keep high reproductive efficiency in dairy herds. Considerable progress has been achieved to automatically detect oestrus on farm level. Most systems are activity-based and monitor the behavioural signs of mating, using detectors for standing heat and/or activity-meters (Saint-Dizier and Chastant-Maillard, Reference Saint-Dizier and Chastant-Maillard2012). These systems display high degrees of sensitivity and specificity if tested in experimental settings, but their efficiency can be affected by environmental conditions (e.g.: housing and flooring conditions) and animal health (e.g. lameness) (Saint-Dizier and Chastant-Maillard, Reference Saint-Dizier and Chastant-Maillard2012). The use of milk progesterone in combination with activity-based systems has led to increased oestrus detection efficiency. A fully automated system for milk progesterone measurement (Herd Navigator®, Lattec, DK) has become available in Europe and Canada, which can be combined with DeLaval® milking robot or parlour (Mazeris, Reference Mazeris2010) and allows the analysis and interpretation of frequently taken samples (Friggens and Chagunda, Reference Friggens and Chagunda2005; Friggens et al., Reference Friggens, Bjerring, Ridder, Højsgaard and Larsen2008).
The analysis of both plasma and milk progesterone profiles in combination with clinical findings is used for diagnosing atypical ovarian patterns and identifying potentially sub fertile cows (Lamming and Darwash, Reference Lamming and Darwash1998). Delayed postpartum resumption of the ovarian activity and prolonged luteal phases commonly cause reduced fertility (Lamming and Darwash, Reference Lamming and Darwash1998; Gautam et al., Reference Gautam, Nakao, Yamada and Yoshida2010; Ranasinghe et al., Reference Ranasinghe, Nakao, Yamada, Koike, Hayashi and Dematawewa2011) and reduced embryo survival (Santos et al., Reference Santos, Rutigliano and Sa Filho2009). Adequate endocrine regulation during the follicular phase is highly relevant for a good fertilization (Starbuck et al., Reference Starbuck, Gutierrez, Peters and Mann2006). Accurate monitoring of both pre-ovulatory decline and post-insemination rise in milk progesterone can be used to identify animals with compromised fertility, as progesterone secretion is directly responsible for embryonic development since the very early stage of pregnancy (Green et al., Reference Green, Hunter and Mann2005; Stronge et al., Reference Stronge, Sreenan, Diskin, Mee, Kenny and Morris2005; McNeill et al., Reference McNeill, Diskin, Sreenan and Morris2006). For instance, low milk progesterone concentrations around days 4–7 after insemination are associated with low fertility and increased risk of embryonic losses (McNeill et al., Reference McNeill, Diskin, Sreenan and Morris2006).
Progesterone concentrations can also be altered by hepatic metabolism (Rhinehart et al., Reference Rhinehart, Starbuck-Clemmer, Flores, Milvae, Yao, Poole and Inskeep2009). In dairy cows, the cytochrome CYP2C (converting progesterone to 21-hydroxyprogesterone) and the aldo–keto reductase AKR1C (converting progesterone to 20α-hydroxyprogesterone) are the most active enzymes in the liver (Lemley and Wilson, Reference Lemley and Wilson2010). Future studies should test progesterone metabolites as potential biomarkers of fertility. It is worth noting that food intake plays an important role in regulating progesterone metabolism by altering liver blood flow and hepatic enzymes (Sangsritavong et al., Reference Sangsritavong, Combs, Sartori, Armentano and Wiltbank2002; Lemley et al., Reference Lemley, Vonnahme, Tager, Krause and Wilson2011; Hart et al., Reference Hart, Camacho, Swanson, Vonnahme and Lemley2014).
Progesterone profiles can be mathematically modelled (Friggens and Chagunda, Reference Friggens and Chagunda2005; Blavy et al., Reference Blavy, Derks, Martin, Hoglund and Friggens2016) to define ‘typical’ and ‘atypical’ progesterone profiles. The retrospective analysis of in-line progesterone records matched by accurate clinical information offers a unique possibility of developing biological models useful for management purposes. Some of the potential applications are the identification of abnormal oestrous cycle responsible for poor fertility (Bruinjé et al., Reference Bruinjé, Colazo, Gobikrushnth and Ambrose2017a) or milk progesterone profiles that may be helpful in predicting the AI outcome (Bruinjé et al., Reference Bruinjé, Gobikrushnth, Colazo and Ambrose2017b). In the future, information obtained with activity-based devices and progesterone profiles may be combined with novel indicators measured in milk, which show slight but significant variation related to the reproductive cycle (Toledo-Alvarado et al., Reference Toledo-Alvarado, Vazquez, de los Campos, Tempelman, Gabai, Cecchinato and Bittante2018).
As described above, progesterone concentrations cannot be considered as a sensitive biomarker for performing pregnancy diagnosis or detecting embryonic losses, in particular when embryonic death occurs before CL regression (Szenci et al., Reference Szenci, Humblot, Beckers, Sasser, Sulon, Baltusen, Varga, Bajcsy and Taverne2000). The measurement of pregnancy specific metabolites is a synergistic tool for diagnosing pregnancy and embryonic death. Pregnancy-associated glycoproteins (PAGs) constitute a large family of glycoproteins specifically expressed in the trophectoderm of the placenta in ungulate species. Pregnancy-associated glycoproteins can be found in the maternal blood from approximately 3 weeks of pregnancy (Wallace et al., Reference Wallace, Pohler, Smith and Green2015). Plasma PAG-1 concentrations in cows seem to be a good biomarker for pregnancy diagnosis and embryonic loss from day 28 after AI, if the time interval between calving and insemination is of at least 60 d (Haugejorden et al., Reference Haugejorden, Waage, Dahl, Karlberg, Beckers and Ropstad2006; Friederick and Holtz, Reference Friederick and Holtz2010; Celi et al., Reference Celi, Merlo, Da Dalt, Stefani, Barbato and Gabai2011; Barbato et al., Reference Barbato, Merlo, Celi, Sousa, Guarneri, Beckers and Gabai2013). Milk PAG concentrations are 20–30 times lower than in plasma (Friederick and Holtz, Reference Friederick and Holtz2010). Therefore, most of the available assays are not suitable for measuring PAG in milk before day 60 of pregnancy (Friederick and Holtz, Reference Friederick and Holtz2010; LeBlanc, Reference LeBlanc2013; Lawson et al., Reference Lawson, Shahzad, Dolecheck, Martel, Velek, Ray, Lawrence and Silva2014). However, an immunoradiometric assay that is able to measure milk PAG (picogram levels) has been developed by Melo Sousa et al. (Reference Sousa N, Tchimbou and Beckers2015) and it may be used in low fertility herds for pregnancy diagnosis.
Reduced uterine health during the first 45 d postpartum decreases fertility in dairy cows. In addition, risk for suffering clinical or subclinical endometritis is increased under these conditions (Kasimanickam et al., Reference Kasimanickam, Duffield, Foster, Gartley, Leslie, Walton and Johnson2004; Sheldon et al., Reference Sheldon, Price, Cronin, Gilbert and Gadsby2009; Walsh et al., Reference Walsh, Williams and Evans2011). Reported prevalence of endometritis in dairy cows is very variable (5–68%). Some of the factors that affect this prevalence variability are the timing of examination after calving and the diagnostic methods (de Boer et al., Reference de Boer, LeBlanc, Dubuc, Meier, Heuwieser, Arlt, Gilber and McDougall2014). In order to reduce such variability, the development of an in vivo cow test for uterine inflammation (specific electronic noses, for instance) would be useful. Electronic noses consist of an array of electronic sensors for chemical detection of volatile molecules. In addition, electronic noses are cheaper, faster, portable, and easier to manipulate than gas chromatography techniques. However, low specificity is one of the most limiting factors for electronic noses (Kou et al., Reference Kou, Zhang and Liu2017). Although imperfect, electronic noses are a reasonable tool to improve odour assessment of vaginal discharges (Sannmann et al., Reference Sannmann, Burfeind, Suthar, Bos, Bruins and Heuwieser2013). This system displays higher intra-assay repeatability compared to the human nose, although this device is not able to fully discriminate between pathogens causing endometritis (Burfeind et al., Reference Burfeind, Bruins, Bos, Sannmann, Voigtsberger and Heuwieser2014).
Conclusions and future prospects
This paper summarizes the current knowledge of biomarkers for some important aspects affecting animal production and welfare in dairy herds and sets the focus of the future research that needs to be done to improve performance and welfare in dairy animals.
In the future, the emergence of new technologies (omics approaches and systems biology) will probably contribute to identify biomarkers for specific health and welfare problems at a much earlier stage. Faster analytical procedures with enhanced analytical sensitivity are also required. For instance, for mastitis detection a huge simplification of the milk proteome complexity can be achieved by exploiting the selectivity derived by the peculiar surface topography of surface active maghemite nanoparticles, which allow the rapid determination of hidden putative biomarkers by a cutting edge diagnostic strategy (Magro et al., Reference Magro, Zaccarin, Miotto, Da Dalt, Baratella, Fariselli, Gabai and Vianello2018).
Some biomarkers, although recognized as very specific, still need to be integrated in automated systems, platforms and technologies so they can be used by farmers and veterinarians. For instance, although PAGs can be considered specific biomarkers for pregnancy diagnosis and foetal welfare, such biomarkers have not yet been implemented at the farm level. Biomarkers can be mathematically modelled to create biological models that contribute to management decisions. Therefore, in the near future artificial intelligence technology could take advantage of retrospective examination of the available databases. For instance, progesterone and BHB concentrations can be measured in milk and, therefore, can be used to obtain large datasets.
Effective strategies for improving performance, health and welfare in dairy animals will require collaboration across a broad range of specialisms and must embrace farmers, consultants, veterinarians, and bio-informaticians. Many methods are available under the term precision livestock farming, which is defined as real-time monitoring technologies aimed at managing the smallest manageable production unit. This implies a novel machine-based approach about the most significant diseases in intensive dairy farming (lameness, mastitis, ketosis; Halachmi and Guarino, Reference Halachmi and Guarino2016) as well as quantifying pain and stress, NEB, heart rate, odour etc. (Halachmi et al. Reference Halachmi, Guarino, Bewley and Pastell2019). Animal monitoring will inform farmers about disturbances at early stages of specific diseases, improving animal performance, health and welfare.
Acknowledgements
This article is based upon work from COST Action FA1308 DairyCare (DairyCare, 2016), supported by COST (European Cooperation in Science and Technology). COST is a pan-European intergovernmental framework. Its mission is to enable break-through scientific and technological developments leading to new concepts and products and thereby contributing to strengthening Europe's research and innovation capacities.