Introduction
In first language (L1) listening comprehension, we continuously integrate various types of linguistic and contextual information not only to interpret what is being said but also to generate predictions about what will be said next (for reviews, see Huettig, Reference Huettig2015; Kuperberg & Jaeger, Reference Kuperberg and Jaeger2016; Pickering & Gambi, Reference Pickering and Gambi2018). Predictive (or anticipatory) processing allows for dialogic interaction, and communication in general, to proceed rapidly, smoothly, and efficiently (Kutas et al., Reference Kutas, DeLong, Smith and Bar2011; Pickering & Garrod, Reference Pickering and Garrod2004). However, recent research suggests that L1 listeners do not always engage in predictive processing (Huettig & Guerra, Reference Huettig and Guerra2019; Mishra et al., Reference Mishra, Singh, Pandey and Huettig2012), and that prediction may not be as automatic and unconscious as it was often assumed to be (see Brothers et al., Reference Brothers, Swaab and Traxler2017). Moreover, many questions remain regarding the extent to which prediction also plays a role in second language (L2) listening (for a review, see Godfroid, Reference Godfroid2020), and regarding the internal and external factors that influence prediction.
In this study, we further explored the nature of grammatical prediction in L1 and L2 sentence processing. So far, most studies on morphosyntax-based prediction have used gender markings in the noun phrase (NP) as predictive cue (e.g., Hopp & Lemmerth, Reference Hopp and Lemmerth2018; Huettig & Guerra, Reference Huettig and Guerra2019), and there is a need to complement this research area with new target structures. Therefore, we focused on verb morphology, which has rarely been the object of earlier prediction research. We investigated the predictive processing of stem-vowel alternations that occur in German strong verbs in the simple present – a subtle, subregular morphosyntactic feature that is often incompletely acquired in second language acquisition (SLA; see Godfroid, Reference Godfroid2016). The vowel alternation (e.g., /ε/ in fällt 3SG, “falls” vs. /a/ in fallt 2PL, “fall”) encodes grammatical number information and represents a potential cue to predict whether the upcoming subject in VSO sentences will be singular or plural. Using visual world eye tracking, we compared prediction in adult L1 speakers of German and advanced L2 learners of German with Dutch as their L1; furthermore, we explored whether prediction was influenced by working memory capacity (see Huettig & Janse, Reference Huettig and Janse2016), and by the language users’ (un)awareness that the strong verbs’ morphology provided a predictive number cue (see Curcic et al., Reference Curcic, Andringa and Kuiken2019). The participants in our study had previously participated in a related experiment (see Koch et al., Reference Koch, Bulté, Housen and Godfroid2021), revealing robust prediction effects based on number markings encoded in regular German verb affixation. It is thus interesting to investigate whether these same participants are also able to use strong verb morphology – in comparison a much more complex and challenging structure, especially for learners – for predictive number processing.
L1 and L2 predictive sentence processing
In its strict sense, predictive language processing means that expected upcoming linguistic information is preactivated in the brain before it is actually encountered in the input (see Huettig, Reference Huettig2015). A widely implemented method to experimentally measure such predictive processing is the visual world paradigm, exposing participants simultaneously to pictures on a computer screen and auditory stimuli, while their eye movements are being recorded (for reviews, see Huettig et al., Reference Huettig, Rommers and Meyer2011; Magnuson, Reference Magnuson2019). Most commonly, prediction is operationalized as more looks toward a target picture before onset of the target in the auditory input in trials containing a predictive cue as compared to uninformative trials. In addition to this strict definition, prediction is sometimes operationalized in a less strict sense as “facilitation,” meaning that certain information allows for subsequent words to be processed faster and more easily, an effect which may become visible only after target onset. Following Karaca et al. (Reference Karaca, Brouwer, Unsworth, Huettig, Kaan and Grüter2021), we see such facilitation as a part of the prediction process and therefore do not differentiate between facilitation and prediction in its strict sense in this study.
L1 listeners exploit a wide range of linguistic cues predictively, including lexical-semantic information (e.g., Kamide et al., Reference Kamide, Altmann and Haywood2003), grammar (e.g., Altmann & Kamide, Reference Altmann and Kamide2007), prosody (e.g., Henry et al., Reference Henry, Hopp and Jackson2017), or the wider discourse-context (e.g., Van Berkum et al., Reference Van Berkum, Brown, Zwitserlood, Kooijman and Hagoort2005). Based on such cues, they can for instance predict semantic aspects of upcoming words (e.g., Altmann & Kamide, Reference Altmann and Kamide1999), syntactic structure (e.g., Arai & Keller, Reference Arai and Keller2013), or turn ends (e.g., Ruiter et al., Reference De Ruiter, Mitterer and Enfield2006). As for L1 morphosyntax-based predictive processing, several studies have provided positive evidence (e.g., Dahan et al., Reference Dahan, Swingley, Tanenhaus and Magnuson2000; Lew-Williams & Fernald, Reference Lew-Williams and Fernald2007), but note that most studies on this subject used gender markings on determiners as their target structure.
Recently, there has been an increase in studies exploring L2 predictive processing (Godfroid, Reference Godfroid2020). Research has provided ample evidence that L2 listeners can make predictions based on lexical-semantic cues (e.g., Dijkgraaf et al., Reference Dijkgraaf, Hartsuiker and Duyck2017, Reference Dijkgraaf, Hartsuiker and Duyck2019; Peters et al., Reference Peters, Grüter and Borovsky2018). In contrast to L1 prediction, the outcomes regarding L2 prediction based on morphosyntactic cues have been more mixed. For instance, no L2 predictive processing could be found in some studies using case (e.g., Hopp, Reference Hopp2015; Mitsugi, Reference Mitsugi2017) or gender marking (Lew-Williams & Fernald, Reference Lew-Williams and Fernald2010). Positive evidence comes mainly from studies on gender-based prediction in L2 learners (e.g., Hopp & Lemmerth, Reference Hopp and Lemmerth2018; Morales et al., Reference Morales, Paolieri, Dussias, Valdés Kroff, Gerfen and Bajo2016). Some of these studies only found prediction in learners with an advanced proficiency (e.g., Dussias et al., Reference Dussias, Valdés Kroff, Guzzardo Tamargo and Gerfen2013), or in participants who exhibited solid knowledge of the target structure during production measures (e.g., Hopp, Reference Hopp2013). Moreover, prediction was facilitated when gender was congruent between the L2 and the learner’s L1 (e.g., Dussias et al., Reference Dussias, Valdés Kroff, Guzzardo Tamargo and Gerfen2013; Hopp & Lemmerth, Reference Hopp and Lemmerth2018), suggesting a dependency of prediction on L1–L2 similarity.
Studies comparing L1 and L2 predictive processing generally found prediction effects in the L2 to be smaller (e.g., Chun & Kaan, Reference Chun and Kaan2019; Grüter et al., Reference Grüter, Lew-Williams and Fernald2012), but this does not necessarily mean that the underlying mechanisms are different. Several factors may in fact account for the different prediction outcomes in L1 and L2 processing, suggesting that both rely on the same mechanisms (see Kaan, Reference Kaan2014): L2 processing in general may be affected by a diminished accuracy of lexical representations (Kaan, Reference Kaan2014), enhanced competing interlingual information (Weber & Broersma, Reference Weber, Broersma and Chapelle2012), and slower lexical access (Shook et al., Reference Shook, Goldrick, Engstler and Marian2015). Combined, this may contribute to an increased cognitive load in the L2, potentially reducing the cognitive resources necessary for prediction (Kaan, Reference Kaan2014; also see Grüter et al., Reference Grüter, Rohde, Schafer, Orman and Valleau2014, Reference Grüter, Rohde and Schafer2017, who express this idea in their Reduced Ability to Generate Expectations – or RAGE – hypothesis).
The predictive use of verb number markings
To complement existing, mainly gender-marking-based research on morphosyntax-based prediction, we introduced a new target structure by focusing on subregular German verb morphology providing predictive cues for upcoming subject number (see below, for details and examples). Relatively few studies have investigated the predictive processing of number markings in verb morphology, and existing studies exclusively investigated the L1 English processing of the copula be, comparing its third-person singular (3SG) and third-person plural (3PL) forms is and are of the simple present tense (PRES). Generally, they used visual world designs, contrasting single-object and multiple-objects pictures, accompanied by auditory stimuli in which the copula provided predictive number information. Kouider et al. (Reference Kouider, Halberda, Wood and Carey2006) used auditory stimuli such as Look, there is a/are some loopite(s), and reported a tendency in 2-year-old toddlers to cast anticipatory looks after hearing the verb; however, their design did not allow to assess with certainty whether predictive eye movements were based on the verb, the quantifier that immediately followed it, or a combination of both. Lukyanenko and Fisher (Reference Lukyanenko and Fisher2016) found predictive processing of the copula in 3-year-olds and adults when exposed to sentences such as Where is/are the good apple(s)?. Riordan et al. (Reference Riordan, Dye and Jones2015), however, could not find predictive eye movements in a study with adult participants. A possible explanation for this null result might be that the time slot during which prediction could have occurred was too brief to measure any anticipatory eye movements (which may also apply to Kouider et al., Reference Kouider, Halberda, Wood and Carey2006). Brown et al. (Reference Brown, Fox and Strand2021) found positive evidence for prediction; moreover, they found that the number cue provided by the copula reduced phonological competition effects when competitor words did not match the target number. Reuter et al. (Reference Reuter, Dalawella and Lew-Williams2021) found predictive processing of the copula in adults and children when two simple pictures were contrasted, but not when complex visual stimuli (photographs including four potential referents) were used.
In contrast to English, German has a relatively rich morphosyntactic agreement system and its native speakers may thus be more inclined to rely on morphosyntactic cues than speakers of English. Moreover, research should go beyond using only the copula as carrier of predictive information, as its conjugation is highly irregular and achieved through suppletion, resulting in a very salient singular–plural distinction, while conjugation through affixation or allomorphy is less salient and harder to learn and process (Krause et al., Reference Krause, Bosch and Clahsen2015). Prediction based on regular (“weak”) affixation in German verbs has been investigated in Koch et al. (Reference Koch, Bulté, Housen and Godfroid2021), a visual world study that is part of the same project as the present study and that involves the same sample (see Procedure). The German weak conjugation paradigm is still productive and marks morphosyntactic information (person, number, mood, and tense) uniquely through affixation. In the auditory stimulus sentences, the verb’s suffix (e.g., folg-t 3SG, “follows” vs. folg-en 3PL, “follow”) was the only morpheme allowing to distinguish between singular and plural and thus to anticipate the upcoming subject’s number. Both adult L1 and L2 speakers of German showed strong suffix-based prediction effects. All had become aware that the suffixes provided a number cue, suggesting prediction was controlled and strategic.
Here, we focus on German strong conjugation, which marks morphosyntactic information through a combination of affixation and stem-vowel alternations. In PRES, the most common alternations are a→ä (/a/→/ε/) and e→i(e) changes (realized as /e/→/i/ or /ε/→/ɪ/), marking second- and third-person SG. These alternations entail the coexistence of two variants of the same verb stem, each of which marks different functional properties. Table 1 provides examples. Opposing the 3SG and 2PL PRES forms of strong verbs (e.g., fäll-t 3SG vs. fall-t 2PL) creates a minimal pair in which only the stem vowel provides a disambiguating number cue. This is the target structure of the present study. The question is whether L1 German speakers and advanced L2 learners of German (L1 Dutch), who were already found to use the relatively “easy” suffixes of the weak paradigm predictively, also exploit such subtle cues predictively.
Table 1. Examples of German conjugation in the present tense

Note: Phonemic realization: a = /a/, ä = /ε/, e = /e/ (or /ε/ in other phonological contexts), i = /ɪ/, ie = /i/. Changed vowels are marked in bold. A Dutch cognate of fallen (vallen, strong verb) is provided for comparison: Dutch also has a strong conjugation paradigm, but stem-vowel alternations are limited to past tense forms.
The strong paradigm represents a challenge for learners for a number of reasons. The paradigm is no longer productive, and strong verbs are thus a closed verb class; nonetheless, they have a high frequency in everyday German (Köpcke, Reference Köpcke1998). When learners encounter a German verb infinitive that is novel to them, it is impossible to know what class the verb belongs to, making it hard to know when the alternation needs to be applied (Bybee & Newman, Reference Bybee and Newman1995). Yet, strong conjugation represents a subregular paradigm because – despite its unpredictable, irregular appearance – strong verbs are organized following specific functional and morphophonemic regularities (Godfroid, Reference Godfroid2016; Köpcke, Reference Köpcke1998).
Several studies (Godfroid, Reference Godfroid2016; Godfroid & Uggen, Reference Godfroid and Uggen2013; Koch et al., Reference Koch, de Vos, Housen, Godfroid and Lemhöfer2023; Krause et al., Reference Krause, Bosch and Clahsen2015) that have used the vowel change as their target structure provide evidence for its persistent learning difficulty and found the vowel-change-free weak paradigm to be the default conjugation system in learners’ interlanguages. It is a challenge for learners to create and store solid, correct morphological representations of the stem variants with the changed vowels and to map them onto the correct morphosyntactic properties; the coexisting, incorrect default representations with unchanged vowels compete with and easily overrule these target representations. Factors that contribute to this learning difficulty are the unpredictable nature of the vowel change, its limited perceptual salience (see Simoens et al., Reference Simoens, Housen, De Cuypere, Gass, Mackey, Spinner and Behney2017) and information redundancy (DeKeyser, Reference DeKeyser2005), because the morphosyntactic information encoded in the stem variants is also provided through the suffixes. Against this backdrop, one may expect prediction based on the vowel change in strong conjugation to be much more taxing – if at all possible – for L2 learners of German than prediction based on the weak suffixes.
Prediction and awareness
Besides broadening the range of morphosyntactic target structures, research also needs to explore potential moderating factors to better understand the nature of morphosyntactic prediction. In this study, we focus on awareness and working memory capacity (see below). Investigating awareness (or consciousness) – which we specify here as the participants’ conscious realization that certain linguistic structures provide cues to predict upcoming input – is interesting not only from a theoretical but also methodological perspective. Although prediction – especially in the L1 – is often assumed to be automatic and unaware, recent studies have questioned this by showing that prediction can also happen in a top-down, controlled and strategic way. Brothers et al. (Reference Brothers, Swaab and Traxler2017) conducted an electroencephalography (EEG) study on the L1 semantic prediction of final words in written English sentences. Every participant performed one task that was presented as a mere comprehension task, and another that explicitly encouraged them to predict the sentence-final word. Prediction effects were stronger when participants were made aware of the task’s prediction goal. Huettig and Guerra (Reference Huettig and Guerra2019) investigated the effect of several task-related parameters (slow vs. normal speech rate, short vs. long preview time, presence vs. absence of an explicit prediction instruction) on the predictive use of gender-marked determiners in L1 Dutch in a visual world eye tracking study. When speech rate was normal and preview short, a small prediction effect was found but only when participants were instructed to predict; no prediction was found when there was no prediction instruction.
Awareness also represents a much-debated topic within SLA research (e.g., Leow & Hama, Reference Leow and Hama2013; Williams, Reference Williams, Ritchie and Bhatia2009). Curcic et al. (Reference Curcic, Andringa and Kuiken2019) conducted a learning experiment in which Dutch native speakers learned a miniature language based on Fijian. They explored the effect of several cognitive aptitude variables on the anticipatory usage of gender-marked determiners and assessed the (spontaneous) emergence of what they call prediction awareness (i.e., participants realized “that determiners sometimes helped them during the test to arrive at the correct answer faster”, Curcic et al., Reference Curcic, Andringa and Kuiken2019, p. 57) by means of post-experiment interviews. The authors measured prediction as a higher proportion of looks toward the target and faster button-press reaction times for picture selection when determiners provided a predictive cue as compared to when they did not. The aptitude variables influenced prediction indirectly: they predicted the emergence of awareness, and only aware learners were found to engage in anticipatory processing. Similarly, in Andringa (Reference Andringa2020) – a study that not only measured if but also when learners of an artificial L2 became aware – anticipatory processing of determiners marked for animacy and distance occurred only after participants had developed awareness. Both studies underscore the importance of assessing participants’ awareness through careful debriefing.
Prediction and working memory
Morphosyntax-based prediction might also depend on working memory. In a visual world study, Huettig and Janse (Reference Huettig and Janse2016) explored the influence of individual differences on gender-marking-based prediction in L1 Dutch speakers and found a strong link between working memory capacity and prediction. Since then, several prediction studies investigated the role of working memory for predictive processing, though none of them used morphosyntactic target structures. In a semantic prediction experiment, Ito et al. (Reference Ito, Corley and Pickering2018) manipulated cognitive load by having half of the participants (either L1 or advanced L2 speakers of English) perform a memory task in addition to the prediction task. Increased cognitive load delayed prediction, and this was similar in the L1 and L2 groups. These findings indicate that cognitive resources play a role in prediction. Chun and Kaan (Reference Chun and Kaan2019) investigated the relation between working memory and L2 semantic predictive processing more directly. In their visual world experiment, complex stimulus sentences were used to augment cognitive load. Prediction effects were found in both the L1 and advanced L2 English groups, starting somewhat earlier in the L1 group. Against the authors’ expectations, L2 prediction was not modulated by working memory. More recently, in a study using ambiguous relative clauses, Chun (Reference Chun2020) did not find successful semantic prediction to depend on the L2 speakers’ working memory. Both Chun and Kaan (Reference Chun and Kaan2019) and Chun (Reference Chun2020) suggest small group size or a lack of variance among the participants as possible explanations for the absence of an effect of working memory on prediction. Favier et al. (Reference Favier, Meyer and Huettig2021) investigated whether L1 speakers of Dutch use passive constructions to predictively attribute agent roles. Working memory was a significant predictor of prediction, but the size of the effect was small. Clearly, there is a need for more experimental research to establish the precise nature of the relationship between working memory and predictive processing.
The current study
The present study further explores predictive L1 and L2 language processing in a visual world eye tracking experiment by using a new morphosyntactic target structure and by assessing the roles of awareness (i.e., the presence/absence of the spontaneously emerging awareness that one can focus on the verb morphology to anticipate subject number) and working memory for prediction. The L2 learners of German in our study were native speakers of Dutch, who had extensive prior knowledge of the weak and strong German conjugation paradigms. Dutch and German are typologically closely related Germanic languages that share many similarities. Crucially, Dutch also has a strong conjugation paradigm involving stem alternations in past tense forms, and many verbs are cognates in both languages. Yet, Dutch strong verbs never have alternating stems in PRES (see Table 1), potentially causing negative L1–L2 transfer involving blocking effects (Ellis, Reference Ellis2006), which makes it particularly difficult for Dutch speakers to fully master the strong conjugation paradigm (see Koch et al., Reference Koch, de Vos, Housen, Godfroid and Lemhöfer2023). That is, learners first learn the association between 3SG PRES and the regular -t suffix, which matches their L1 and therefore allows for direct positive L1–L2 transfer; subsequently, it may be more taxing for them to learn at a later stage that stem vowels of strong verbs also constitute a cue for the same morphosyntactic information, because the firstly learned cue “overshadows” the new cue.
Our investigation addressed the following research questions:
-
RQ1: To what extent do L1 and advanced L2 speakers of German exploit the morphosyntactic number information encoded in the strong verbs’ stem vowels (e.g., fällt 3SG vs. fallt 2PL) to predict the number of the upcoming subject?
-
RQ2: Is the prediction effect influenced by language status (L1 vs. L2)?
-
RQ3: Is the prediction effect influenced by prediction awareness?
-
RQ4: Is the prediction effect influenced by working memory capacity?
We measured prediction in a twofold manner, using the participants’ button-press reaction times and their eye gaze data. Note that while RQs 1–3 were analyzed using both measures, the analysis of RQ4 was based uniquely on the reaction-time data (for full details, see Analysis).
We expected the L1 group to engage in predictive processing, given the sensitivity of native speakers to number mismatches in subject–verb agreement (e.g., Wagers et al., Reference Wagers, Lau and Phillips2009). Because of the reduced saliency of the target structure, we expected these prediction effects to be small. In the L2 group, we only expected very limited prediction effects despite their advanced proficiency levels, given the difficulty of the vowel change for this population. This would also be in line with theories that claim form-function mappings in the L2 to be incomplete and/or weaker than in the L1, causing slower processing (e.g., weaker links hypothesis, Gollan et al., Reference Gollan, Montoya, Cera and Sandoval2008; Missing Surface Inflection Hypothesis, Prévost & White, Reference Prévost and White2000). Note that in the analyses, we only included strong verbs that the participants were able to produce correctly during a conjugation task. Overall, we hypothesized a facilitatory role of awareness (e.g., Curcic et al., Reference Curcic, Andringa and Kuiken2019), and a positive correlation between prediction and working memory (see Huettig & Janse, Reference Huettig and Janse2016).
Methods
Participants
Thirty-one L1 speakers of German (age range 18–45 years; mean age 30 years; 23 identified as female, eight as male) and 30 L2 learners of German with L1 Dutch (age range 18–50 years; mean age 27 years; 22 identified as female, eight as male), residing in Brussels, Belgium, participated in our study. All signed an informed participation consent form and were given monetary compensation. All participants were students or adults with academic degrees. None of them had hearing problems, dyslexia, or color vision deficiency. All participants spoke several other foreign languages besides German, in particular English and French, and Dutch in the L1 group. Most L2 speakers had started to learn German in high school or at university. On average, their first intensive encounter with the German language was at the age of 16.47 years (SD = 6.88); however, there was quite some variation across participants (range 1–47 years, or 6–20 years when disregarding two outliers). Their mean score on the German version of the LexTALE (www.lextale.com; see Lemhöfer & Broersma, Reference Lemhöfer and Broersma2012), an untimed lexical decision task that can be used to approximate general German proficiency, was 73.67% (SD = 10.31%). This score corresponds to the CEFR level B2 upper-intermediate and differed significantly from the L1 group (M = 90.40%, SD = 4.18%; W = 867, p < .001, r = −.74).
During the post-experiment interviews, all L2 participants reported being familiar with the phenomenon of the vowel-changing strong verbs. Almost all reported having learned about this through formal language instruction in the past. Most of them had the impression that they knew the strong paradigm well, though they also found it effortful to apply the vowel change correctly and reported this to be item-dependent, with frequent verbs being known best. Two participants had learned the paradigm exclusively via immersion and reported mastering it rather well.
Stimuli
The main task of the experimental session was a picture-matching task with visual world eye tracking, combining visual and auditory stimuli (available at https://www.iris-database.org/details/QCXMA-3yDNr). The target structure was the stem-vowel alternation that occurs in German strong verbs in the simple present tense (see above; see Table 1): some of the auditory stimulus sentences contained strong verbs that were either inflected in 3SG (e.g., fäll-t) or 2PL (e.g., fall-t), that preceded the subject, and whose stem vowels (here: /ε/ vs. /a/) provided a potential predictive cue for subject number. A brief story introduced the task: a group of blue and green aliens has landed on Earth and started doing all kinds of comical things; a girl named Anna observes them and describes with surprise what she sees. Each trial consisted of two pictures that depicted the aliens carrying out actions, combined with a sentence in German – Anna’s statements – presented auditorily and matching only one of the pictures. The participants were instructed to listen to Anna and to indicate the target picture as fast and accurately as they could by pushing a button on a game controller, without turning their gaze away from the screen. Both the eye movement recordings and the reaction-time latencies of the button presses would later be used to measure prediction.
All items of the task consisted of German verbs, embedded in grammatical sentences describing the aliens’ actions. An items spreadsheet can be found in the supplementary materials of this paper, accessible through https://osf.io/vjcmz/. There were three item classes: strong items (32 German strong verbs), weak items (32 German weak verbs), and filler items (a mixture of 32 weak, strong, and irregular verbs). We selected verbs that were preferably highly frequent or cognate with Dutch to facilitate comprehension in the L2 group (90% of the 96 verbs were cognates). All verbs were inflected either in 3SG or 2PL PRES and thus always received the -t suffix (see Table 1). Only the strong verbs in 3SG would undergo a stem-vowel change in addition to the suffix, allowing the strong verbs’ stem vowel to become a predictive number cue. The weak and filler items could not be used predictively (weak items, because their 3SG and 2PL forms are fully identical, and filler items, because number was never contrasted in the picture pairs of filler trials); these items would not be analyzed and mainly served the purpose of making our prediction-related research aim less obvious.
The onsets of the auditory and visual stimuli were always synchronous. Figure 1 shows how the trials were presented (videos illustrating the course of trials in real time are available in the supplementary materials: https://osf.io/vjcmz/). All pictures were developed for the purpose of the experiment and always showed one or two aliens carrying out the actions referred to by the verb. The pictures had dimensions of 300 × 400 pixels and were contoured by black rectangles. The target and distractor pictures were aligned horizontally on a 1,024 × 768 pixels screen, with a 112 pixels distance between them. The target and distractor interest areas had dimensions of 350 × 450 pixels and were thus slightly larger than the corresponding pictures. All fixations within these interest areas would be registered as looks toward the target or distractor picture.

Figure 1. Screenshots illustrating the presentation of trials.
Note: In all screenshots, the left picture is the target. The verb’s stem vowel represents a predictive number cue in prediction trials (a, c) but not in baseline trials (b, d; in these examples, alien color is blue on the left picture and green on the right picture). The dotted rectangles indicate interest areas.
All audio stimuli were built up in the same way: they had a duration of 9,000 ms, and all subcomponents had the same duration across trials (see Figure 2). They started with an introduction that served as 4,500 ms of preview time, and part of which was Anna’s exclamation of surprise, enabling her next utterance to be formulated as a question with VSO word order (instead of the default SVO order in German declarative sentences). The verb thus always preceded the subject, enabling us to test whether participants would exploit the verbs’ morphology to anticipate subject number, or if they would wait for the subject NP to select the target. To facilitate measurement, we broadened the interval between verb and subject NP by inserting the German modal particle denn etwa, which emphasizes astonishment.
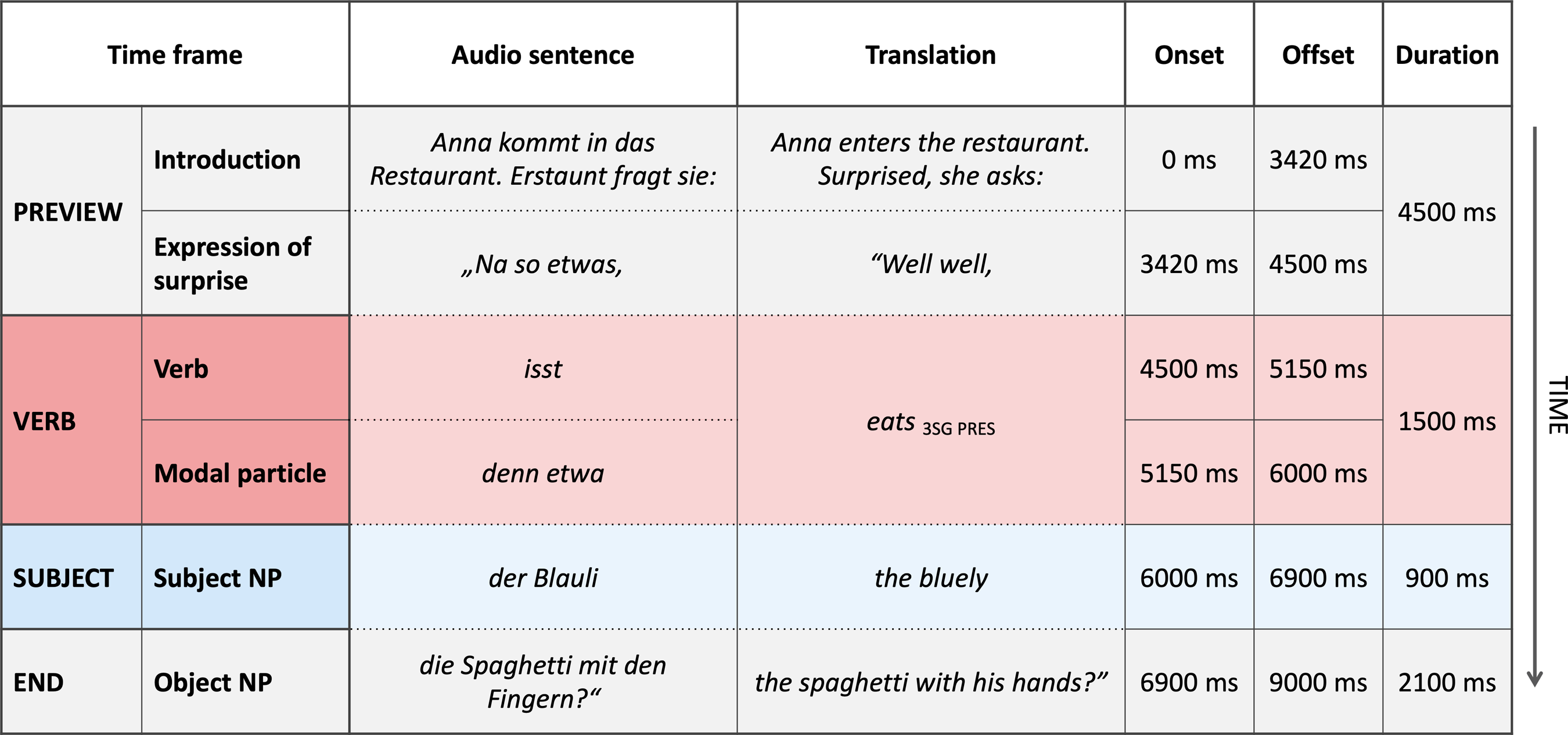
Figure 2. Structure and timing of the auditory stimulus sentences.
The subject always referred to the blue and green aliens; solely alien color (blue vs. green) and syntactic number and person (3SG vs. 2PL) varied systematically in order to create different trial conditions (see below). Blue aliens were called Blaulis (“bluelies”; 3SG: der Blauli-Ø; 2PL: ihr Blauli-s), and the green ones were called Grünlis (“greenlies”; 3SG: der Grünli-Ø; 2PL: ihr Grünli-s). Der is the 3SG determiner (nominative, masculine); ihr is the 2PL personal pronoun nominative, combined with the referent noun in apposition. Both der and ihr thus carry number information, as does the noun’s suffix (-Ø SG or -s PL). On pictures involving one alien, Anna would look toward the participant, as if talking about this alien (using 3SG); on pictures involving multiple aliens, she would look toward them, as if talking to them (justifying the use of 2PL).
All sound recordings were spoken by the experimenter, a female High German native speaker. She applied a rather slow pace (roughly 3.3 syllables per second; see Fernandez et al., Reference Fernandez, Engelhardt, Patarroyo and Allen2020) and stopped briefly after each sentence subcomponent (see Figure 2) to avoid coarticulation. All subcomponents were edited to have exactly the same duration across all trials; care was taken to remove all gaps between the different subcomponents within each sentence.
Main task design
All trials involving strong items systematically varied in the number of the target referent (SG vs. PL), and in the number displayed on the two pictures (same vs. different number). There were thus four possible trial types: (a) different-number pictures, SG target; (b) different-number pictures, PL target; (c) same-number pictures, SG target; and (d) same-number pictures, PL target (see Figure 1). In same-number trials, the only parameter differing between both pictures was alien color. All 32 strong items were only shown once to each participant, in one of the four trial types. This was counterbalanced between participants, using a Latin square rotation: if an item was shown to one participant in trial type a, the next participant would get the same item in type b, etcetera. Target alien color (blue and green) and target picture location (left and right) were counterbalanced over the four trial types.
To measure predictive processing, we distinguished two main trial conditions. Different-number trials were the prediction trial condition, in which the verb’s stem vowel represented the first reliable cue for target subject number. Same-number trials were the baseline trial condition: as number was not contrasted, the number information encoded in the verb was not informative; instead, participants were obliged to wait for the subject NP, providing the disambiguating color cue. We used a pseudo-random trial order: trials appeared in a new, random order for each participant, but there could never be two strong items in a row.
Familiarization task
An online, untimed familiarization task, taking place one week prior to the experiment and taking about 45 minutes to complete (see Procedure), introduced the participants to the materials of the picture-matching task. Our goal was to prevent any difficulties at the level of comprehension or interpretation during the picture-matching task, thereby maximally reducing the potential impact of external factors on processing and eye movements; furthermore, it also served as a production premeasure of the test verbs. The participants saw 96 pictures, one per verb and presented one by one. The pictures always showed a singular alien carrying out an action and also included the German labels of the verbs and objects (see Figure 3). The participants’ task was to write brief descriptions of each picture in German, using the labels (but no further linguistic aids).
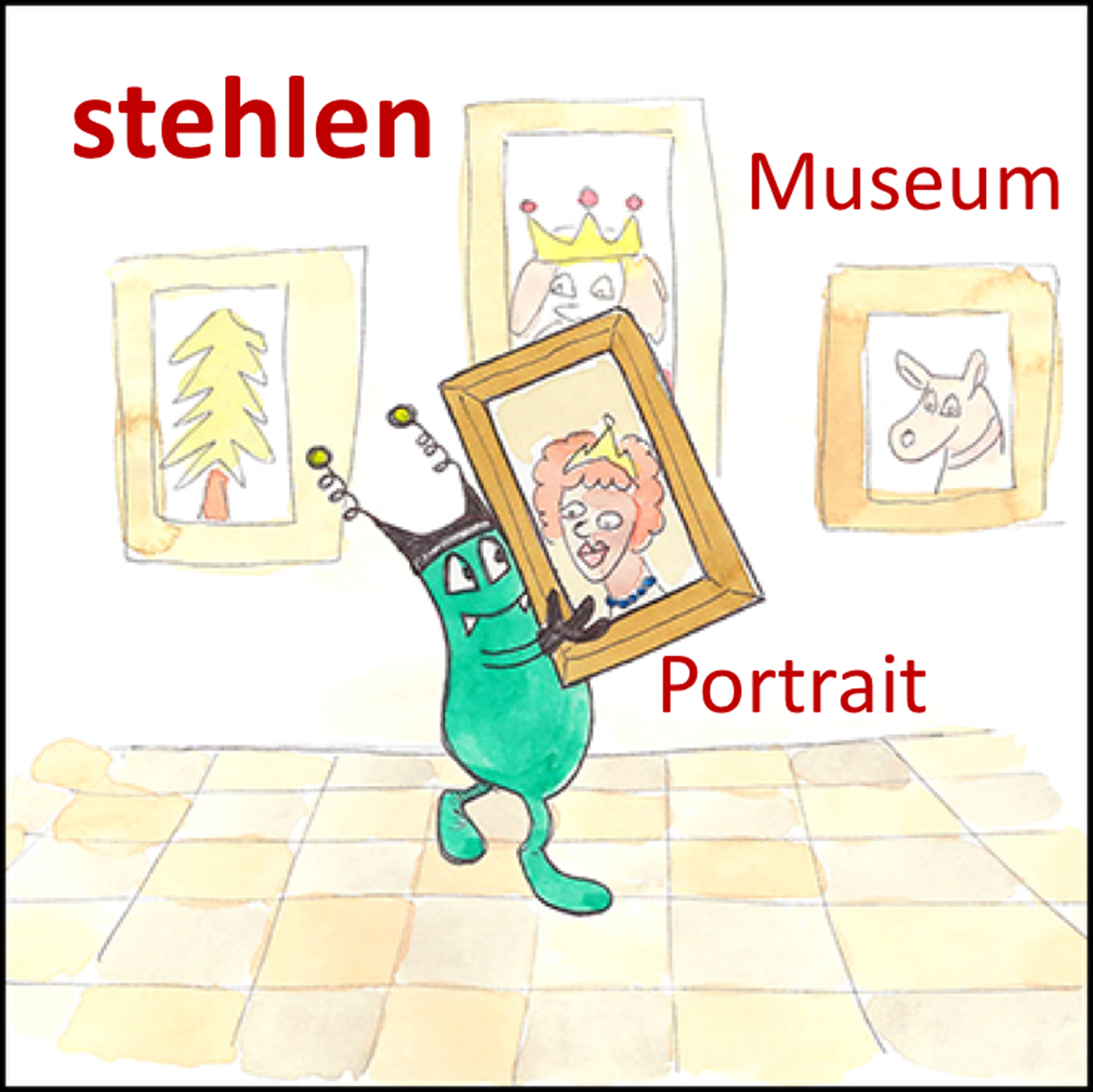
Figure 3. Sample visual stimulus of the familiarization task.
Operation span task
During the experimental session in the laboratory, we administered the operation span task by Klaus and Schriefers (Reference Klaus and Schriefers2016, www.socsci.ru.nl/memory/) as a measure of working memory capacity. The online test had a duration of about 12 minutes. Instructions were provided in the participants’ L1. The participants judged the correctness of mathematical equations while memorizing series of two to six digits, presented to them in 14 blocks of two to six trials. Cronbach’s alpha for the 14 test blocks was .77. The mean of the percentage of correctly recalled digits per test block represented the participants’ operation span score (see Zheng & Lemhöfer, Reference Zheng and Lemhöfer2019).
Awareness interview
We administered an extensive, carefully structured retrospective interview immediately after the second picture-matching task, following the guidelines of Rebuschat (Reference Rebuschat2013). The protocol is available in the supplementary materials (https://osf.io/vjcmz/). The experimenter started by asking general questions about how the participants had experienced the eye tracking task. She also invited the participants to report what they thought the experiment was about, and whether they had used any strategies. She then asked increasingly specific questions about language, first inquiring about the general role of grammar in the experiment, and eventually asking questions about verb form and its predictive function. The participants of the L2 group were also asked to provide information about their prior knowledge of German strong verb conjugation.
The main purpose of this interview was to assess the status of prediction awareness (see Curcic et al., Reference Curcic, Andringa and Kuiken2019): participants would be categorized as aware or unaware, depending on whether or not they had consciously realized that they could focus on the strong verbs’ morphology to determine the target picture in different-number trials, without having to wait for the subject NP.
Oral conjugation task
During the oral conjugation task at the session end, participants conjugated all 64 strong and weak verbs aloud in 3SG PRES, and we explicitly asked to produce the stem vowels as accurately as possible. After each oral production, participants were prompted to provide confidence ratings and source attributions (adapted from Rebuschat, Reference Rebuschat2013): they had to indicate how certain they were that they had produced the right stem vowel (not certain, a little certain, very certain) and which knowledge source they had relied on (guess, intuition, explicitly learned knowledge). All strong verbs that were produced with an incorrect stem vowel or for which participants had indicated “guess” and “not certain” were considered unknown and excluded from the final dataset for these particular participants. This was necessary because such verbs could not be expected to be used predictively. Note that even if participants were unable to produce the verbs accurately, they still may have some implicit, passive knowledge of the target forms. In that sense, a production measure to exclude unknown items is a rather conservative way of assessing knowledge of the target structure.
Procedure
All participants were first familiarized with the experimental materials through the online familiarization task that they did from home. To hide the experiment’s actual focus on morphosyntactic processing, we portrayed our study as a pilot project targeted at assessing the usability of newly developed drawings as language learning materials for children.
One week after familiarization, we tested the participants individually in a quiet lab. The main tasks of the experimental session were two picture-matching tasks with visual world eye tracking, which were always administered in the same order, and each of which took approximately 25 minutes to complete. Both tasks shared the same design, yet they differed in their target structures. The first task focused on prediction based on German weak verb inflection by contrasting 3SG and 3PL PRES and investigating whether the regular suffixes -t and -en could be used predictively. The second task is the picture-matching task described above, which had the stem-vowel alternation in strong verbs as its target structure. In this paper, we focus uniquely on the second task; the first task (for a full report, see Koch et al., Reference Koch, Bulté, Housen and Godfroid2021) is only reported on to the extent necessary to understand and interpret the second task. The first task revealed robust, suffix-based prediction effects; moreover, these were found to be of an aware and strategic kind (also see subsection Awareness, under Results).
Between the picture-matching tasks, we administered the operation span task, the LexTALE, and a background survey.
The second picture-matching task started with five practice trials and a 13-point calibration, after which participants completed 96 experimental trials. These were always preceded by drift checks, and there were brief breaks after every 24 trials. The mean accuracy of target picture identification on test trials was 98.99% in the L1 group (SD = 1.87%, range = 93.75%–100%) and 98.44% in the L2 group (SD = 2.43%, range = 90.63%–100%); we can thus be confident that there were no comprehension problems. Given that the second picture-matching task was preceded by the first picture-matching task, the participants’ experience with the first task could be assumed to have shaped their expectations regarding the second task. Keep in mind, however, that although verb form still contained a number cue, the target structure from the first task no longer provided predictive information. Care was taken to prevent any learning effects between the two tasks: throughout the experimental session, a participant would never encounter the same verb twice in SG or twice in PL, and never twice in the prediction condition or twice in the baseline condition.
The second picture-matching task was immediately followed by the retrospective awareness interview. The oral conjugation task concluded the session. The full session duration was approximately 100 minutes. We audio-recorded the interviews and the conjugation task.
Analysis
A laptop-mount EyeLink Portable Duo (SR Research Ltd., 2016) registered the participants’ eye movements with a sampling rate of 500 Hz. Each sample was coded as either looks toward the target picture, looks toward the distractor picture, or as falling outside of these regions. The original dataset contained 1,952 trials, but we excluded all incorrect responses (25 trials removed) and, subsequently, any unknown strong items (an additional 156 trials removed), resulting in a data loss of 9.27% and a dataset consisting of 1,771 trials.
We assessed predictive processing by means of two outcome variables: the proportion of looks toward the target picture (computed exclusively across all samples assigned to the target or distractor picture regions, excluding samples during blinks or saccades)Footnote 1 , and reaction-time latency of the button-press responses (RT; measured in milliseconds from trial onset until button press). As for the eye movement data, prediction of subject number based on the verb’s stem vowel was operationalized as a higher proportion of looks toward the target picture in prediction trials than in baseline trials during the prediction time frame. This time frame is the critical interval between verb onset and subject NP onset (see Figure 2), ranging from 4,710 to 6,219 ms (corrected for 10 ms of audio delay caused by the experiment computer, plus 200 ms for the time needed to plan and execute eye movements; Saslow, Reference Saslow1967). Prediction at the level of the RTs was operationalized in a different manner, independently from the prediction time frame, as faster button presses in prediction trials than in baseline trials (comparable to Curcic et al., Reference Curcic, Andringa and Kuiken2019). While the operationalization of eye-gaze-based prediction matches the strict definition of prediction (i.e., effects measured before target onset, see above), our measurement of RT-based prediction can strictly speaking be seen as facilitation, since our measurement does not depend on target onset (i.e., effects occurring before or after target onset would be taken into account). We analyzed the eye movement data and the RT data separately by means of two different but complementary analyses in R (R Core Team, 2018; data and scripts are available in the supplementary materials: https://osf.io/vjcmz/).
Analysis of the eye movement data
For the eye movement analyses, we excluded an additional 29 trials for which more than 50% of the gaze data were looks outside the interest areas or missing (see Curcic et al., Reference Curcic, Andringa and Kuiken2019). For the remaining trials, the average of samples contributing per trial was 85.97% (SD = 4.75%). We analyzed these data by means of cluster-based permutation analysis (Maris & Oostenveld, Reference Maris and Oostenveld2007; for examples using eye tracking, see Andringa, Reference Andringa2020; Barr et al., Reference Barr, Jackson and Phillips2014; Guerra et al., Reference Guerra, Bernotat, Carvacho and Bohner2021) with the EyetrackingR package (Dink & Ferguson, Reference Dink and Ferguson2015). This method allows to identify time intervals (so-called time clusters) during which participants are significantly more likely to look at the target picture in prediction trials than in baseline trials (or vice versa). If a significant time cluster signaling more target looks in prediction trials overlaps with the prediction time frame, this represents a prediction effect, and the cluster’s range informs us about its timing. In step one of this analysis, the participant-averaged gaze data are subdivided into time bins of 50 ms, and for each bin, paired sample t tests are run to test the difference in the proportion of target looks in prediction versus baseline trials. This results in the identification of potential clusters of adjacent bins for which a significant difference was found. In step two, 2,500 iterations of randomly shuffling the labels of the conditions being tested, repeating step one, and comparing the resulting clusters among each other are performed, ultimately resulting in probabilities indicating whether or not the clusters identified in step one are significant or due to chance.
We performed seven cluster-based permutation analyses. For analysis 1, we used the full dataset and the procedure just described to establish whether there was a prediction effect in the sample as a whole (RQ1). To test if prediction depended on language status (RQ2), we directly compared the L1 and L2 groups by computing for each 50 ms time bin the proportion of target looks in prediction trials minus the proportion of target looks in baseline trials, and using the resulting difference score as dependent variable for the t tests (analysis 2). In addition, we repeated the procedure from analysis 1 in the L1 and L2 groups separately (analyses 3 and 4). To establish whether prediction was influenced by awareness (RQ3), we directly compared the unaware and aware groups by again using the difference between the proportion of target looks in prediction and baseline trials as dependent variable (analysis 5), and we also repeated analysis 1 in the unaware and aware groups separately (analyses 6 and 7).
Analysis of the reaction-time data
We analyzed the RTs of the button presses by means of linear mixed-effects modeling, using the lme4 package (Bates et al., Reference Bates, Mächler, Bolker and Walker2015; version 1.1-23). We used treatment-coded contrasts and restricted maximum likelihood for model estimation; effects were reported as significant at p < .05. The model took the following form: (reaction time) ∼ 1 + Trial condition*Target + Trial condition*Group + Trial condition*Working memory + Trial condition*Awareness*Trial number + (Trial condition|Participant) + (1|Item). The term left of the tilde (∼) is the outcome variable; the terms at its right are the model terms. The number 1 represents an intercept. As fixed effects, the model included the within-subject factors Trial condition (baseline, prediction) and Target (singular, plural), the between-subjects factors Group (L1, L2) and Awareness (unaware, aware), and the continuous variables Working memory and Trial number. The asterisks mark interactions. The terms including the bar character (|) are the random effects. In modeling the variance components, we tried to balance type I and type II errors by fitting the maximal random structure (as recommended by Barr et al., Reference Barr, Levy, Scheepers and Tily2013), but only if it is supported by the data (see e.g., Matuschek et al., Reference Matuschek, Kliegl, Vasishth, Baayen and Bates2017). We started with a model containing crossed random intercepts for participants and items and included theoretically motivated additional variance components that both yielded a stable (i.e., converging) model and significantly improved model fit (as indicated by the Akaike information criterion and the likelihood ratio test). The resulting model included random intercepts over participants and over items (thus, the model took into account variation based on individual differences among subjects and item-level differences), as well as random slopes for participants depending on Trial condition.
We included all fixed effects for theoretical reasons, regardless of their contributions to model fit. Trial condition was the key variable for assessing prediction (RQ1); its respective two-way interactions with Group, Awareness, and Working memory allowed us to establish whether the prediction effect differed in the L1 and L2 groups (RQ2), whether it depended on awareness (RQ3), and whether it was modulated by working memory (RQ4). Trial number was included to assess potential changes in the course of the experiment. As for Target, this variable reflects whether the target referent was singular (picture with one alien + verb in 3SG) or plural (picture with two aliens + verb in 2PL). This factor needed to be included to account for potential effects of verb form (e.g., 3SG might be processed more easily than 2PL for having a higher frequency in general language use) and of picture complexity (i.e., more complex pictures have been found to attract more fixations than less complex pictures; e.g., Schlenter, Reference Schlenter2019).
Results
Awareness
The interviews confirmed that the participants’ expectations regarding the second picture-matching task were shaped by the experience they had had with the first task during which all of them had engaged in strategic processing: at trial onset in task one, they generally scanned the two pictures for their differences (number, color, objects, and setting or action) and subsequently waited for the element in the sentence that would provide the disambiguating cue. This also means that all of them had developed prediction awareness of the verbs’ suffixes, and they perceived this as relatively easy. For the second task, they expected verb form to again provide a reliable cue in different-number trials; however, almost half of the participants reported that they soon thought that the verb no longer provided a cue “because singular and plural now had identical forms” – not realizing at all that the verb could still be used predictively under specific circumstances. The other half did develop prediction awareness of the stem vowels, though they generally reported that this was more difficult and took more effort to apply than during the first task. We categorized 26 participants as unaware (14 L1, 12 L2) and 35 participants as aware (17 L1, 18 L2).
Eye movements
Table 2 summarizes the results of the cluster-based permutation analyses. Analysis 1 revealed a significant cluster in the sample as a whole, ranging from 5,300 to 6,750 ms (for visualization, see Figure 4). This effect had a positive directionality, indicating there were significantly more looks toward the target picture in prediction trials than in baseline trials. As this cluster overlapped with the prediction time frame (4,710–6,210 ms), we can be confident that it reflects a prediction effect in the full sample (RQ1), starting 800 ms after verb onset and spilling over into the subject time frame (6,000–6,900 ms; see Figure 2). In baseline trials, participants started looking more toward the target around 6,400 ms (Figure 4).
Table 2. Outcomes of the cluster-based permutation analyses
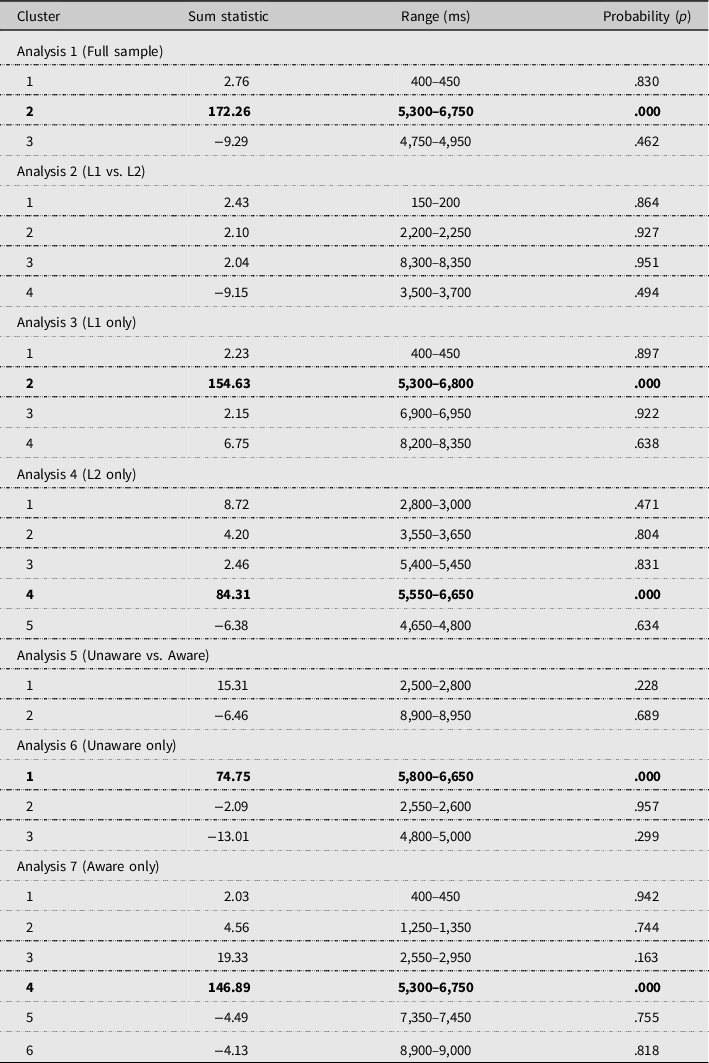
Note: Significant time clusters are marked in bold. Dependent variable of analyses 1, 3, 4, 6, and 7: proportion of looks toward the target picture, with factor Trial condition (prediction, baseline). Dependent variable of analyses 2 and 4: difference between the proportion of looks toward the target picture in prediction and baseline trials, with factors Group (L1, L2) and Awareness (unaware, aware), respectively.
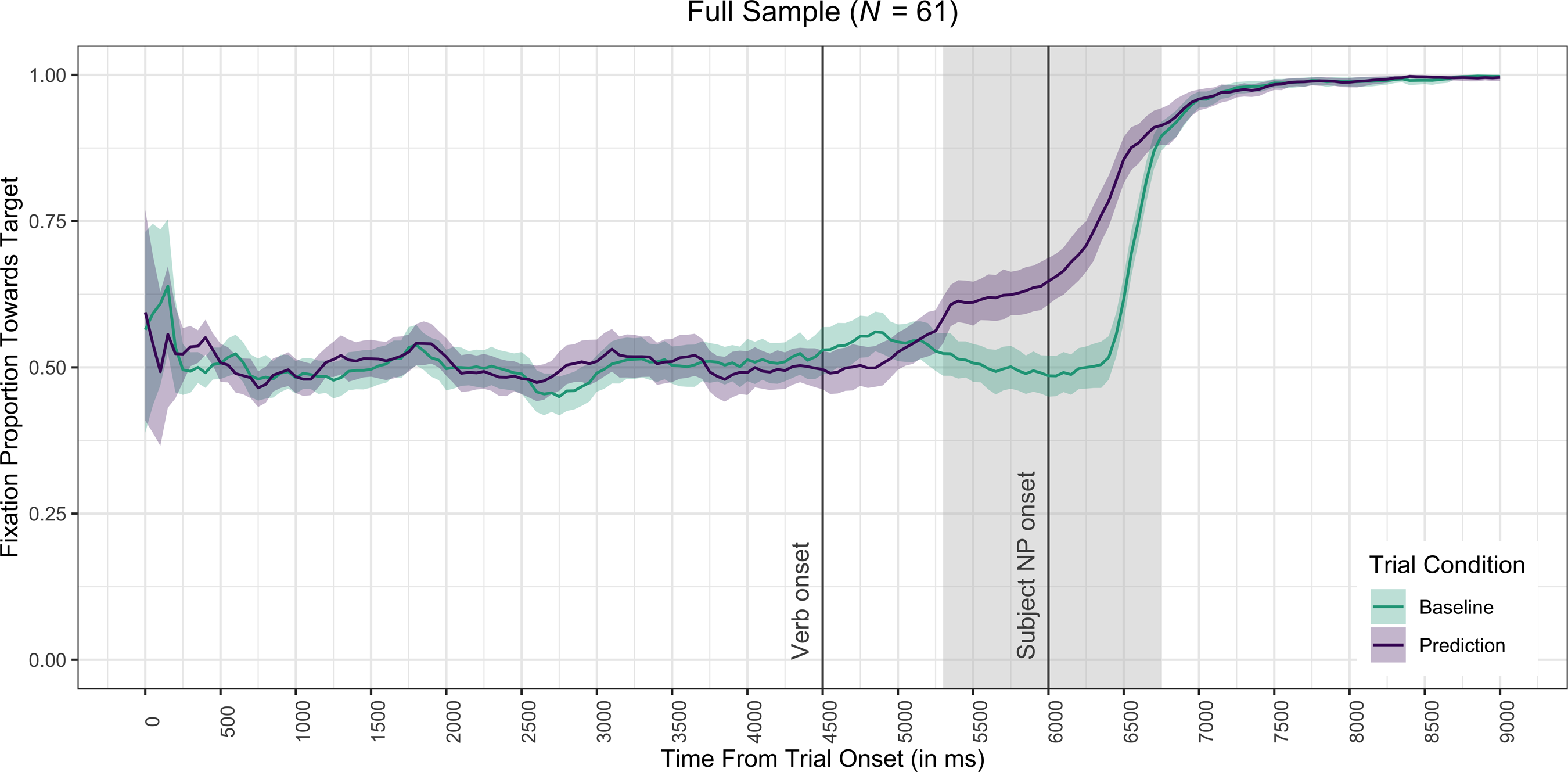
Figure 4. Proportion of looks toward the target picture unfolding over time in the full sample.
Note: Error bands show 95% confidence intervals. Shaded areas indicate time clusters during which there was a significant difference between prediction and baseline trials.
Comparable significant positive clusters representing prediction effects (with spillovers into the subject) were found in the L1 and L2 groups separately (RQ2; Table 2: analyses 3 and 4; see Figure 5). Although the effect started earlier in the L1 (5,300–6,800 ms) than in the L2 group (5,550–6,650 ms), this 250 ms difference was not significant according to analysis 2 that directly compared both groups (for visualization, see Supplementary Figure S1 in the supplementary materials).
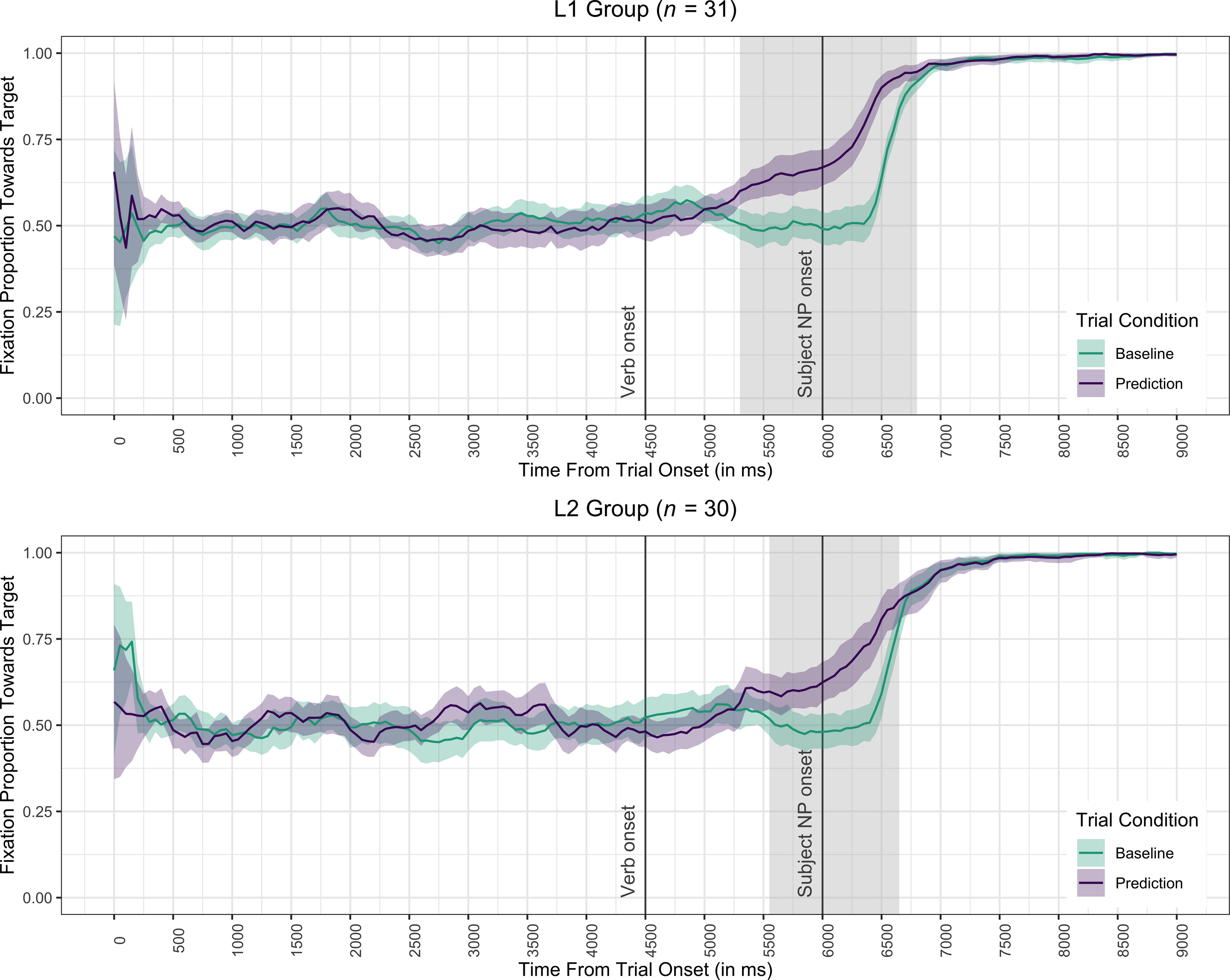
Figure 5. Proportion of looks toward the target picture unfolding over time in the L1 and L2 groups.
Note: Error bands show 95% confidence intervals. Shaded areas indicate time clusters during which there was a significant difference between prediction and baseline trials.
Similarly, significant clusters reflecting prediction were found in the unaware and aware groups separately (RQ3; Table 2: analyses 6 and 7; see Figure 6). While prediction started 500 ms earlier in the aware (5,300–6,750 ms) than in the unaware group (5,800–6,650 ms), this difference was not significant according to analysis 5 which directly compared the unaware and aware participants (also see Supplementary Figure S2).
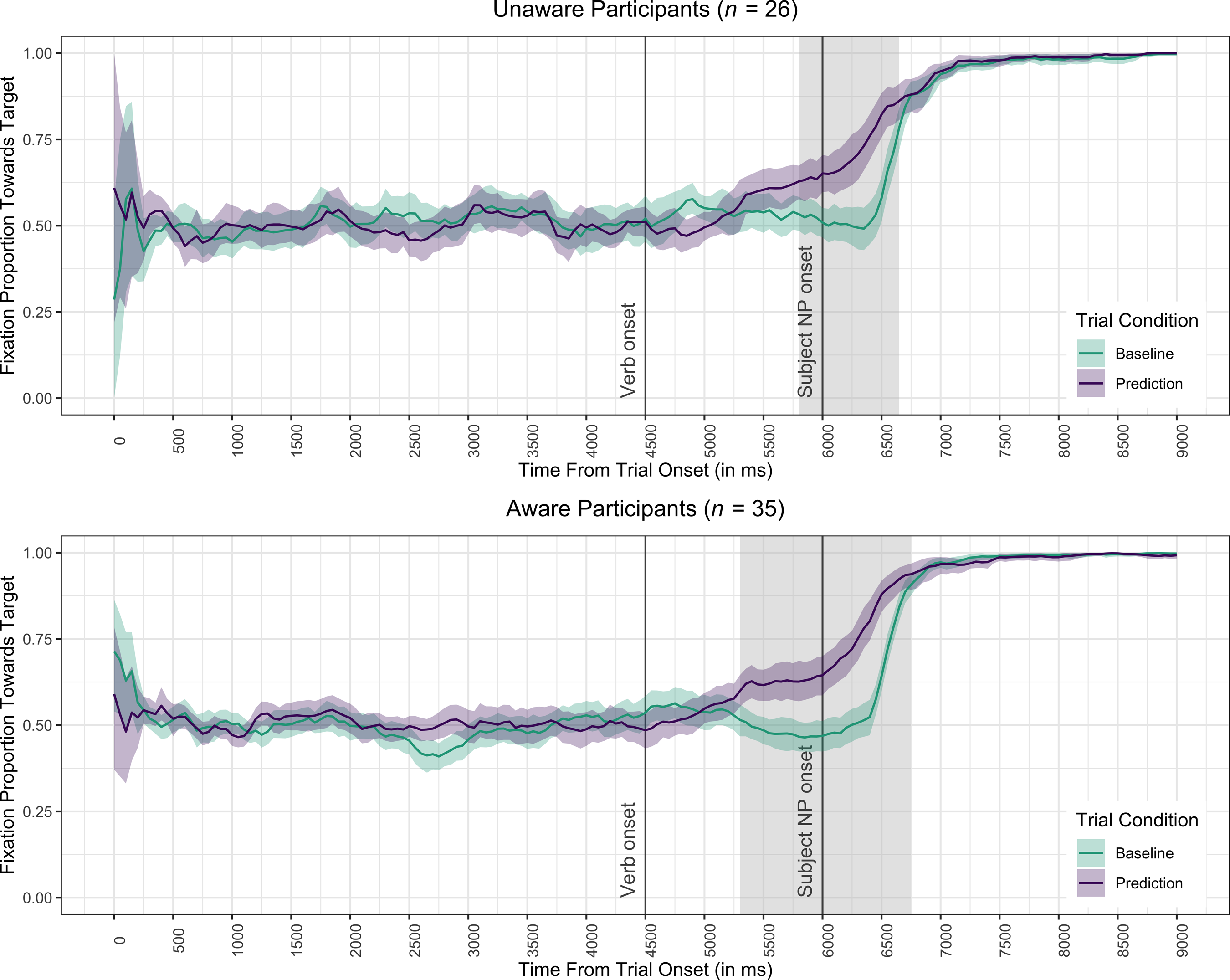
Figure 6. Proportion of looks toward the target picture unfolding over time in unaware and aware participants.
Note: Error bands show 95% confidence intervals. Shaded areas indicate time clusters during which there was a significant difference between prediction and baseline trials.
Reaction times
The RT model output, which can be found in Supplementary Table S1, revealed three significant parameters: the two-way interactions between Trial condition and Group (p = .009) and Trial condition and Working memory (p = .019), and the three-way interaction between Trial condition, Awareness, and Trial number (p = .004). To interpret effects involving categorical variables, we used estimated marginal means (see Table 3) and pairwise comparisons (least square means method). We examined 10 planned contrasts (see Table 4) and applied the Benjamini–Hochberg correction for multiple testing (Benjamini & Hochberg, Reference Benjamini and Hochberg1995), which involves using an adjusted significance threshold for p-values based on a 5% false discovery rate and the number of contrasts.
Table 3. Estimated means based on the reaction-time mixed-effects model

Note: Estimated means and 95% confidence intervals by Trial condition, by Trial condition and Target, by Trial condition and Group, and by Trial condition and Awareness.
Table 4. Pairwise comparisons among estimated means, based on the reaction-time mixed-effects model
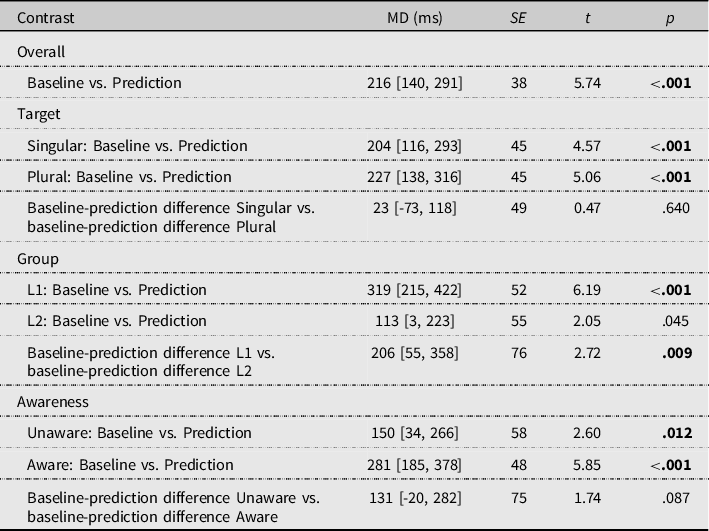
Note: Estimated mean differences (MD) and their 95% confidence intervals (squared brackets); p-values in bold print are significant after a correction for multiple testing. Contrasts were specified for Trial condition (baseline vs. prediction) overall, and at each level of Target, Group, and Awareness. We also compared the mean difference between baseline and prediction trials between the two levels of Target, Group, and Awareness, respectively.
The pairwise comparisons (Table 4) revealed a significant prediction effect in the full sample (RQ1): the model estimated that overall, participants made button-press responses that were on average 216 ms faster on prediction trials than on baseline trials (see Table 3, for the estimated average RTs). The prediction effect was not further modulated by Target: Table 4 shows that the prediction effect was present and similar within singular-target and plural-target trials.
The significant interaction between Trial condition and Group shows that prediction was different in the L1 and L2 groups (RQ2). This is confirmed by the significance of the contrast between the prediction effects (i.e., the estimated RT difference between baseline and prediction trials) in both groups (Table 4). Moreover, whereas the estimated difference between prediction and baseline trials in the L1 group (319 ms) was significant, this was not the case for the L2 group (113 ms; note that p was .045, but this was not significant after the correction for multiple testing).
There was no significant interaction between Trial condition and Awareness (RQ3). Both the aware and unaware groups showed a significant prediction effect (see Table 4). Although the aware participants’ estimated prediction effect was on average 131 ms larger than that of the unaware participants, this difference was not significant. The significant interaction between Trial condition, Awareness, and Trial number indicates that Trial number significantly modulated the RTs, but only on prediction trials and only in the aware group (see Figure 7): under those conditions, the model estimated the RTs to become 5 ms faster with every trial. Figure 7 illustrates this: while RTs on baseline trials remain steady in the aware group, those on prediction trials become faster. The plot for the unaware group suggests that participants engaged in predictive processing only at task outset, but that this effect vanished over time because responses became slower on prediction trials but faster on baseline trials.

Figure 7. Reaction time as a function of Trial condition, Awareness, and Trial number.
The significant interaction between Trial condition and Working memory indicates that prediction was modulated by working memory capacity (RQ4). While Working memory did not significantly influence RT on baseline trials, the model estimated that on prediction trials, every unit increase of the operation span test score was accompanied by a 19-ms reduction in RTs. Thus, the higher the participants working memory capacity, the faster they were at responding on prediction trials (see Figure 8).
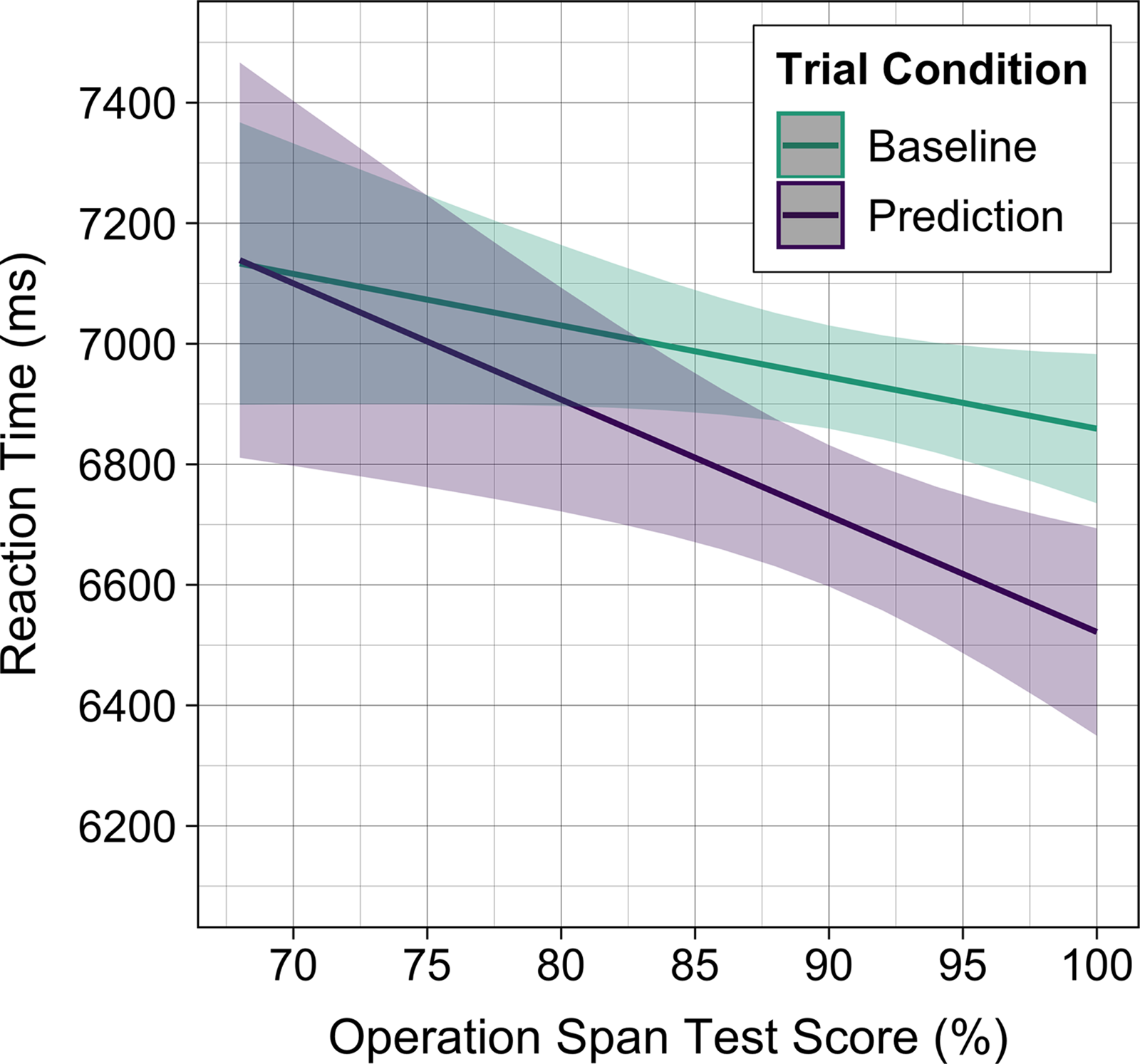
Figure 8. Reaction time as a function of Trial condition and Working memory.
Discussion
The present study investigated the extent to which L1 and advanced L2 speakers of German exploit the stem allomorphy in strong verbs to anticipate subject number. The analyses revealed a significant prediction effect in the full sample (RQ1): in the eye gaze data, this effect started 800 ms after verb onset; in the RT data, the effect manifested itself as button-press responses that were 216 ms faster in prediction trials than in baseline trials. Comparable RT-based prediction effects were present in both singular-target trials and plural-target trials, indicating that both the changed and unchanged stems were used predictively. In the gaze data, there was significant prediction in both language groups, though this effect started 250 ms earlier in the eye movements of the L1 group than in the L2 group. In the RT data, the estimated difference between baseline and prediction trials was significantly larger (by 206 ms) in the L1 group than in the L2 group. In fact, the difference in RTs between baseline and prediction trials was only significant in the L1 group but not in the L2 group. Prediction awareness modulated prediction, but only to a certain extent (RQ3). Significant prediction effects in the gaze and RT data were present in both the unaware and aware groups. In the aware group, prediction in the gaze data started 500 ms earlier than in the unaware group. The prediction effect in the RTs was 131 ms larger in the aware group than in the unaware group, but this difference was not significant. Throughout the experiment, the aware but not the unaware group became faster at responding on prediction trials. Working memory positively influenced prediction (RQ4): the higher the participants’ working memory capacity, the faster they were at responding on prediction trials.
L1 and L2 morphosyntax-based prediction
Our study extends the evidence of morphosyntax-based L1 and L2 predictive processing to a new target structure. So far, the most common target structure in this domain has been gender marking (e.g., for L1 processing: Dahan et al., Reference Dahan, Swingley, Tanenhaus and Magnuson2000; Huettig & Guerra, Reference Huettig and Guerra2019; for L2 processing: Hopp & Lemmerth, Reference Hopp and Lemmerth2018; Morales et al., Reference Morales, Paolieri, Dussias, Valdés Kroff, Gerfen and Bajo2016); thus, it is interesting to see that prediction also extends to number information processing, and to a target structure that is of similar or even greater difficulty than gender markings because of its low saliency and informative redundancy. Our finding of successful predictive L1 processing is in accordance with the fact that German has a relatively rich morphological system and that its users even rely on subtle morphosyntactic cues for smooth, anticipatory processing.
Although no (or, at best, only a marginal) prediction effect was found in the RT data of the L2 group, the eye gaze data did reveal a significant prediction effect. To date, only a limited number of studies has found positive evidence for morphosyntax-based prediction in adult L2 sentence processing (e.g., Curcic et al., Reference Curcic, Andringa and Kuiken2019; Trenkic et al., Reference Trenkic, Mirkovic and Altmann2014), and some studies found negative evidence (e.g., gender: Lew-Williams & Fernald, Reference Lew-Williams and Fernald2010; case: Hopp, Reference Hopp2015; Mitsugi, Reference Mitsugi2017). That we found prediction in L2 speakers is therefore noteworthy, given the learning and processing difficulties that strong conjugation represents in L2 learners, particularly when their L1 is Dutch (Koch et al., Reference Koch, de Vos, Housen, Godfroid and Lemhöfer2023). The learners’ advanced proficiency level potentially played an important role in this respect (see Grüter et al., Reference Grüter, Rohde and Schafer2017; Hopp, Reference Hopp2013); perhaps the results would have looked different in learners with lower proficiency. Furthermore, despite the hypothesized blocking effects in this population (see above), the typological proximity between German and Dutch may have played in the learner group’s favor. It may be interesting to replicate this study with learners whose L1 is typologically less related to the target language, as for instance Swedish, which does not have subject–verb agreement and whose speakers may thus be less inclined to rely on the grammatical number cues.
A comparison between the L1 and L2 groups revealed that prediction started slightly earlier in the gaze data of the L1 group; in the RT data, a clear prediction effect could only be found in the L1 but not the L2 data. This L1–L2 difference is in line with prior research (e.g., Chun & Kaan, Reference Chun and Kaan2019; Grüter et al., Reference Grüter, Lew-Williams and Fernald2012) and likely is a consequence of overall slower lexical access, increased cognitive load (see Kaan, Reference Kaan2014) and less stable mental representations of the different stem variants in the L2 group. Recall that all participants had been familiarized extensively with the experimental materials and that we had only kept those items in the dataset for which the participants demonstrated accurate conjugation knowledge, ruling out a lack of comprehension of the stimulus materials in the learner group as a potential cause for the L1–L2 differences.
It remains important to keep in mind that our findings are based on two different outcome measures. Eye movements are a covert, automatic response type, reflecting online interpretive processes, while button presses are an overt, controlled response that occur after the interpretive processes and the additional operations following them (e.g., action or response planning; see Ayasse et al., Reference Ayasse, Lash and Wingfield2017). Furthermore, as the eye-gaze-based prediction effects in our data were observable before the target onset, they correspond to a strict definition of prediction; our RT-based prediction effects, however, were observable only after the target onset (which can be assumed to be a direct consequence of the additional steps of controlled processing that are involved in the button-press responses), and some may prefer to refer to such effects as facilitation.
Prediction and awareness
Aware and unaware predictive processing
Our study provides evidence that morphosyntax-based predictive processing can happen spontaneously and unconsciously. Nonetheless, while prediction is often tacitly assumed to be an unconscious, automatic process and while time-sensitive measures such as EEG or eye tracking are often associated with natural, unaware processing, our findings also demonstrate that L1 and L2 prediction can happen consciously and strategically (see Andringa, Reference Andringa2020; Curcic et al., Reference Curcic, Andringa and Kuiken2019). The significant interaction between trial condition, awareness, and trial number underscores this strategic behavior: responses became faster on prediction trials throughout the task, but only for aware participants. Thus, future prediction research should not take unaware processing for granted and integrate careful debriefing to assess awareness.
Keep in mind that we coded awareness in a binary way, thus applying strict cutoffs to categorize data that might in reality be of a much more complex nature. That is, perhaps there was variation with respect to the moment in time in which the participants became aware, or perhaps some aware participants found it more difficult to use the stem vowels for prediction than others. We did not quantify such nuances.
As for prediction in the unaware group, the trends in Figure 7 might provide further insight: the model estimated prediction in the unaware group to only occur early in the task; after that, RTs become faster on baseline trials and slower on prediction trials. Possibly, the unaware participants first tried to strategically focus on the verbs to identify the target in different-number trials, just like they had done in the first task. This actually led to successful predictive processing, but without reaching these participants’ consciousness. After that, participants dropped this strategy that they believed to no longer work in the second task; they thus started to pay less attention to the verb and to only focus on the subject instead, and processing was no longer predictive. This may also explain why RTs on baseline trials became faster. What is interesting is that prediction, in this context, seems to be linked to attention to form, without implying awareness. Though such an interpretation is based on the visual inspection of trends and not on significant results, it would be in line with what the unaware participants reported during the interviews.
Limited effect of awareness on prediction
Our study suggests that awareness had a limited, facilitatory effect on prediction, which is in line with prior research (Brothers et al., Reference Brothers, Swaab and Traxler2017; Huettig & Guerra, Reference Huettig and Guerra2019). It would be interesting to investigate how awareness and language status interact; however, our subgroups were too small to integrate such an interaction in our analyses. Nonetheless, visual inspection of the eye gaze data plotted over time (see Appendix S1 in the supplementary materials) shows predictive trends within both the aware and unaware subgroups of the L1 and L2 groups. This would suggest that our findings deviate from those of Curcic et al. (Reference Curcic, Andringa and Kuiken2019) and Andringa (Reference Andringa2020), who found predictive L2 processing – which they took as evidence for L2 learning – to fully depend on the emergence of awareness. Note, however, that their studies were learning experiments involving an artificial miniature language and thus no prior knowledge of the target structure, while we investigated L2 prediction based on existing linguistic knowledge. The divergence between our findings and theirs might indicate that the role of awareness for prediction also depends on the stage of knowledge development: possibly, awareness only plays a decisive role for prediction at the beginning stages of learning; in L1 processing or at more advanced stages of L2 learning, knowledge is (more) consolidated and allows for automatized predictive processing, leaving less room for awareness to play a role.
As we had expected a more important effect of awareness, we studied the unaware group in more detail. Note that this group also included 11 participants (6 L1, 5 L2) who recalled having noticed the presence of vowel-changing strong verbs in the task, without realizing they could be used predictively. To rule out the possibility that prediction in the unaware group was contingent on whether or not participants noticed the target structure, we compared the noticing and non-noticing subgroups. Visual inspection of the eye movement data (see Appendix S2 in the supplementary materials) revealed predictive looking behavior in the unaware non-noticing subgroup, too, both within L1 and L2 speakers; in fact, prediction seemed slightly stronger in the non-noticing than in the noticing subgroup. In the unaware L2 subgroup, noticing the strong verbs appeared to be linked to a RT slowdown (average RTs were even slightly slower on prediction trials than on baseline trials). In the unaware L1 subgroup, noticing seemed to have a small beneficial effect. On the basis of these explorative observations of descriptive statistics, we tentatively conclude that prediction in the unaware group was not dependent on noticing.
What predicted awareness?
When discussing why some participants were aware while others were not, it is important to keep in mind that the picture-matching task reported on here was always administered 15 minutes after the first picture-matching task (see Koch et al., Reference Koch, Bulté, Housen and Godfroid2021). The participants’ experience of the second task was thus affected by their experience of the first task. Note that this task-order effect affected all participants in a similar way, as confirmed by the interviews; thus, it does not jeopardize the validity of the results. Also note that counterbalancing task order was not a suitable alternative. The 3SG–2PL contrast in the second picture-matching task was more unusual than the 3SG–3PL contrast in the first task: when we describe pictures, we typically use a third and not a second person to refer to the agents visible in the pictures. If the experimental session had started with the 3SG–2PL contrast, participants could have thought during the remainder of the session that plural-agent pictures corresponded not only to 3PL but also to 2PL in the audio. This might have compromised cue reliability in the suffix-based prediction task, because weak verbs in 3SG and 2PL share identical forms. Exposing all participants first to picture-matching task 1 and subsequently to task 2 prevented such undesired confounding effects.
Considering that the entire sample had engaged in aware predictive processing in the first picture-matching task, it is noteworthy that only half of our participants developed prediction awareness during the second picture-matching task. The context in which participants performed the second task was thus clearly an awareness-inducing one. Both picture-matching tasks were almost identical and only differed in their target structure, suggesting that (un)awareness was closely linked to the target structure’s degree of difficulty. As pointed out earlier, the suffixes of the regular, productive weak paradigm were perceived as easy, while the vowel change of the subregular, unproductive strong paradigm required more effortful processing in both participant groups. In the L2 group, participants had robust knowledge of the regular suffixes and positive transfer facilitated prediction, while their knowledge of the strong paradigm was less robust, and transfer was negative.
A question that arises is why some participants developed awareness, while others did not. We therefore performed a post hoc exploratory analysis, using binomial logistic regression. As potential predictors of awareness, we considered working memory and the LexTALE scores (as an index of German proficiency). To this list, we added target structure knowledge: this was the average percentage of correctly produced strong verbs (i.e., with application of the correct stem vowel) during the (written) familiarization task, which should serve as an indicator of how well participants mastered the target structure. Table 5 provides the descriptive statistics. The model outcomes (for details, see Appendix S3 ) revealed that none of the variables predicted awareness in the L1 group, suggesting the cause for the emergence of awareness in this group lies elsewhere. A potential and tentative explanation is that different levels of metalinguistic awareness in both subgroups may have played a role here.
Table 5. Means and standard deviations (between parentheses) for potential predictors of awareness

In the L2 group, target structure knowledge but not the other variables significantly predicted awareness (p = .044), with mean accuracy scores of 89% in the aware subgroup against only 68% in the unaware subgroup. This finding suggests that the emergence of awareness was associated with a deep level of knowledge of the target structure. One possibility is to interpret this association in the context of theories of L2 learning, in which awareness represents a central topic. More traditional models of learning assume that learning starts with explicit, conscious forms of knowledge, and that practice subsequently leads to more implicit, unconscious forms of knowledge at more advanced stages of development (e.g., Skill Acquisition Theory; DeKeyser, Reference DeKeyser, Doughty and Williams1998). However, that we found an association between more robust forms of knowledge and awareness does not directly fit into such a model of learning; rather, it fits Cleeremans’ Radical Plasticity Thesis (Cleeremans, Reference Cleeremans, Banerjee and Chakrabarti2007, Reference Cleeremans2011), which assumes a reversed order of the stages of L2 knowledge development: learners first acquire implicit/unconscious, weak representations; then, based on input and experience, these representations start becoming more robust, of improved quality, and explicit/conscious, involving access to aware inspection of the knowledge; ultimately, knowledge becomes automatic. Thus, awareness is not seen as a starting point for learning, but as a sign of knowledge having reached a robust stage, which would apply to our L2 sample. Alternatively, it may also be the case that participants with better target structure knowledge simply had more opportunities to predict throughout the experiment than participants with less extensive knowledge. Assuming that each prediction trial of an item for which a participant knows the target conjugation increases the odds of participants becoming aware of the predictive cue, this may explain why the emergence of awareness depended on target structure knowledge.
Prediction and working memory
The facilitatory effect of higher working memory capacity on predictive button presses is in line with prior research (Favier et al., Reference Favier, Meyer and Huettig2021; Huettig & Janse, Reference Huettig and Janse2016). Recall that some studies (Chun, Reference Chun2020; Chun & Kaan, Reference Chun and Kaan2019) did not find an effect of working memory on semantic prediction. A tentative explanation of why working memory appears to modulate morphosyntactic but not semantic prediction could be that semantic prediction is achieved with much more “ease,” leaving no room for working memory to be of influence.
Our finding applies to the sample overall; due to limited (sub)sample size, we could not integrate interactions between working memory and awareness or language status in our model. Nonetheless, our post hoc exploratory analyses suggest that working memory scores were similar in the aware and unaware subgroups of the L1 and L2 groups. This is interesting, given that working memory is often associated with explicit learning aptitude (e.g., Li, Reference Li2015, Reference Li2016): on such an account, working memory could be expected to determine the emergence of awareness, yet this was not the case for our data. We invite future research to further explore the precise relationship between working memory and prediction.
Conclusions
This study extends the positive evidence for morphosyntax-based predictive processing in L1 speakers and L2 learners to stem-vowel alternations in German strong verbs, a new target structure with high difficulty due to subregularity, redundancy, and low salience. Both native speakers and learners used the strong verbs’ stem vowels to predict upcoming subject number, though this effect remained limited in the learner group. About half of the participants spontaneously developed prediction awareness and thus engaged in strategic, controlled processing – in the L2 group, this depended on how robust their knowledge of the target structure was. The other half remained unaware, meaning prediction happened unconsciously. Awareness facilitated prediction, but only to a limited extent. Working memory capacity had a facilitatory effect on predictive RTs.
Our study suggests a clear reliance on subtle morphosyntactic cues during online processing in L1 speakers and, to some extent, also in advanced learners of German, a language with a rich morphosyntactic system. Future prediction research may further explore how language status, awareness, working memory, and other individual differences (e.g., processing speed; Huettig & Janse, Reference Huettig and Janse2016) interact, preferably with an increased sample size that allows for the analysis of complex interactions and/or subgroup analysis. Moreover, our experiment could easily be combined with learning treatments (see Cintrón-Valentín & Ellis, Reference Cintrón-Valentín and Ellis2015) or instruction manipulations (e.g., Huettig & Guerra, Reference Huettig and Guerra2019).
Replication package
For supplementary material (appendices, supplementary tables and figures, videos illustrating experimental trials) accompanying this paper, visit https://osf.io/vjcmz/. This OSF site also provides research materials allowing replication (data, analysis code, interview protocol). The stimulus materials (audio recordings and pictures) are available via the IRIS database: https://www.iris-database.org/details/QCXMA-3yDNr.
Acknowledgments
This study was conducted within the framework of Eva Koch’s doctoral research, supported by an FWO (Fonds Wetenschappelijk Onderzoek, Flanders) grant awarded to Alex Housen and Aline Godfroid. The authors would like to thank Marije Michel for her excellent idea to use aliens as referents in the experimental stimuli, Florian Hintz for his advice regarding the general study design, and Falk Huettig for his recommendation to increase the length of the critical prediction time frame. Finally, the authors thank all reviewers for their highly valuable feedback and suggestions for improvement.
Conflict of interest
The authors declare none.
Ethical approval
This project was approved by the Ethical Committee in Humane Sciences (Vrije Universiteit Brussel) on the 4 December 2014.