1. Introduction
The discovery of a massive number of single nucleotide polymorphisms (SNPs) in the genome of several species has enabled exploration of genome-wide signatures of selection via an assessment of variation in marker allele frequencies among populations (e.g. Holsinger & Weir, Reference Holsinger and Weir2009). Several methods have been proposed for doing this, such as site frequency spectrum, linkage disequilibrium and population differentiation (Sabeti et al., Reference Sabeti, Schaffner, Fry, Lohmueller, Varilly, Shamovsky, Palma, Mikkelsen, Altshuler and Lander2006; Akey, Reference Akey2009). Concerning population differentiation, a parameter θ=F ST, measuring relatedness between pairs of alleles within a sub-population relative to that in an entire population, has been used for this purpose (Wright, Reference Wright1951; Cockerham, Reference Cockerham1969; Weir & Hill, Reference Weir and Hill2002); Lewontin & Krakauer (Reference Lewontin and Krakauer1973) and Robertson (Reference Robertson1975) discuss related approaches. Equivalently, θ can be interpreted as a measure of dispersion of gene frequencies among groups relative to the variation expected in the population from which such groups derived. For example, Akey et al. (Reference Akey, Zhang, Zhang, Jin and Shriver2002) analysed over 26 500 SNPs for which allele frequencies were available in three populations of humans. The θ parameter was estimated for every marker locus and the distribution of estimates over the entire genome, and by chromosome, was examined. By referring these estimates to their empirical genome-wide distribution, 174 candidate genes were identified as possible targets of selection.
Holsinger & Weir (Reference Holsinger and Weir2009) provide an account of the logic of the procedure. Briefly, given a set of loci in a given species, a reasonable assumption is that all share the same demographic history and patterns of migration. If these loci are neutral and have similar mutation rates, members of this set can be conceivably regarded as exchangeable realizations of the same evolutionary process. Loci showing departures from the resulting distribution may serve as flags of genomic regions that have been under the influence of selection. Under the hypothesis of selective neutrality, the distribution (over loci) of estimates of θ is expected to be driven by genetic drift, assumed to affect all loci in a similar fashion. On the other hand, when selection operates on one or several loci (as in a multifactorial model for complex traits), markers that are within genes or in nearby locations will display large or small values of θ, the latter occurring when some sort of balancing selection takes place (Cavalli-Sforza, Reference Cavalli-Sforza1966). This opens an avenue for identification of regions associated with population differentiation, e.g., high versus low producing breeds of dairy cattle. Knowledge of such regions may be useful for enhancing the effectiveness of breeding programs via marker-assisted selection, or for tagging variants associated with disease or quantitative traits. While unusual values of θ may point to genomic locations where selection may have operated, there is arbitrariness with respect to characterizing the type of selection that might have occurred. Typically, loci are classified as either neutral, or subject to balancing selection (low values of θ), or favoured by selection within some specific population or environment (large population differentiation, thus leading to large values of θ). If the values of θ arise from different evolutionary or artificial (such as in plant and animal breeding) processes, one would expect to observe a mixture of distributions leading to clusters representing the different kinds of mechanisms operating. There is no apparent reason why there should be only two or three such clusters; there may be several clusters harbouring loci undergoing different types of selection processes. On the other hand, if θ values vary completely at random due to genetic drift, a single cluster is to be expected.
Statistical issues associated with inferring θ-statistics have been discussed, e.g., by Weir & Cockerham (Reference Weir and Cockerham1984) and Weir & Hill (Reference Weir and Hill2002), with emphasis on methods of moments estimation; by Balding (Reference Balding2003) using maximum likelihood for beta-binomial and Dirichlet-multinomial distributions; and by Holsinger (Reference Holsinger1999), Beaumont & Balding (Reference Beaumont and Balding2004) and Guo et al. (Reference Guo, Dey and Holsinger2009) employing Bayesian procedures. None of these treatments have addressed the possible existence of a clustered structure.
The objective of this paper is to present a two-step procedure eventually leading to clusters of θ values. The first step, along the lines of Holsinger (Reference Holsinger1999), Balding (Reference Balding2003) and Beaumont & Balding (Reference Beaumont and Balding2004), uses a simple Bayesian structure for drawing samples from the posterior distributions of θ-parameters, but without constructing Markov chains. This step assigns a weakly informative prior to allelic frequencies and does not make any assumptions about evolutionary models. The second step regards samples from these posterior distributions as ‘data’ and fits a sequence of finite mixture models, with the aim of identifying clusters of θ-statistics. Hopefully, these would reflect different types of processes and would assist in interpreting results.
The paper is organized as follows. Section 2 reviews basic concepts. In section 3, the first step of the procedure is presented, contrasted with maximum likelihood, and illustrated with a hypothetical dataset and with data on type II diabetes in three populations. Section 4 describes the second step of the procedure, and illustrates it with a dataset containing allelic frequencies for 12 polymorphic isozyme loci in 12 populations of the argan tree (Argania spinosa L. Skeels) of Morocco presented in Petit et al. (Reference Petit, El Mousadik and Pons1998) and analysed by Holsinger (Reference Holsinger1999). The paper concludes with a discussion of the proposed methodology.
2. Background
(i) Basic concepts
The stage is set by reviewing essentials of a random effects treatment proposed by Cockerham (Reference Cockerham1969, Reference Cockerham1973). Suppose that genetic markers (e.g. SNPs) are screened in a set of individuals in each of R groups or populations, the latter viewed as drawn at random from some conceptual hyper-population from which such groups derive. Consider a bi-allelic locus (developments carry to multiple alleles as well) and let A l and a l be the two alleles at locus l (l=1, 2, …, L); define p l=Pr (A l) to be the true, unobserved, frequency of allele A l in the hyper-population, with 1−p l=Pr (a l) being the frequency of a l. Cockerham (Reference Cockerham1969) defines a l as any allele other than A l and uses an indicator variable x to denote allelic state (‘content’), such that

Here, r=1, 2, …, R denotes group or replicate, i indicates an individual, j is an index for a within-individual deviation and l=1, 2, …, L is an indicator for locus. Even though x rij,l is a binary variable (so a linear model is questionable), Cockerham (Reference Cockerham1969) uses the linear decomposition

where p l is as before and a r,l~(0, σa,l2), b ri,l~(0, σb,l2) and w rij,l~(0, σw,l2) are mutually uncorrelated zero-mean random deviates, specific to locus l; the σ2′s are variance components. Under the assumption that all alleles at locus l have the same marginal distribution,

and

for l=1, 2, …, L. Decomposition (1) induces the following covariance structure between allelic content variables:

A standard assumption is Cov(a r, a r′)=0. The following correlations (all positive) follow.
• Pairs of alleles drawn at random from different individuals in the same group are correlated as
(2)so 0⩽θl⩽1 for all l.
• Pairs of alleles drawn within individuals over all replicates bear a correlation equal to
where F is the total inbreeding coefficient, also known as F IT (e.g. Weir & Hill, Reference Weir and Hill2002).
• The correlation between alleles within individuals within the same replicate is
which is the within sub-population inbreeding coefficient f.
It is easy to show that

This expression satisfies

indicating that a reduction in heterozygosity has two sources: one that is due to population sub-division or Wahlund's effect, (1−F ST,l), and a reduction within subpopulation or group caused by ‘local’ inbreeding, (1−F IS,l).
Note that parameter F ST given in (2) can also be written as

In Cockerham (Reference Cockerham1969), the variance σa,l2 arises by drawing alleles from a random sample of populations. Under conceptual repeated sampling, this generates a distribution having such variance. However, in many applications, the R groups under study are targeted (as opposed to randomly sampled) populations, e.g., Myles et al. (Reference Myles, Hradetzky, Engelken, Lao, Nürnberg, Trent, Wang, Kayser and Stoneking2007) ; this defines a ‘fixed effects’ model. Now, since σa,l2 is the between-group variance in allelic content as per model (1), an alternative parametric definition of θl in terms of the unknown gene frequencies of the R groups is

where is the average (over groups) of the frequencies of allele A l at locus l. Note that
l is taken as an unweighted average; it does not seem sensible to express a parameter in terms of sample size (unless weights assigned to samples reflect true population sizes). Expressing θl explicitly in terms of the locus-specific gene frequencies yields

providing a mapping from the joint space of R allelic frequencies to the single-dimensional space of θl, which resides in (0, 1). If allelic frequencies for the different loci are driven from the same stochastic evolutionary process (e.g. as generated by random drift), this defines a distribution of values of θ expected under neutrality assumptions. From a Bayesian perspective, every unknown is a random variable and, since allelic frequencies are unknown, θ as given in (3) will posses a distribution, both a priori and a posteriori. In the first step of the method proposed in this paper, the posterior distribution of θl will result from assigning a vague prior to all allelic frequencies, corresponding in some sense to what could be termed as a fixed effects treatment from a frequentist perspective. The second step addresses the question of whether or not all θl stem from the same distribution or from different distributions resulting from heterogeneity of the underlying stochastic processes. This makes the treatment proposed here different from those in, e.g., Holsinger (Reference Holsinger1999) or Balding (Reference Balding2003).
3. Estimation of parameters
(i) Inferring gene frequencies
Gene frequencies can be inferred using a simple Bayesian approach. Suppose that n r individuals are genotyped in population r, so that the number of alleles screened at locus l is 2n r=n r,Al+n r,al, where n r,Al and n r,al are the observed numbers of copies of A l and a l, respectively.
A convenient assumption is that of mutual independence between the distributions of alleles at different loci (stronger than that of pairwise linkage equilibrium). Linkage disequilibrium is pervasive but the assumption made above facilitates matters and is widely used, e.g., by Corander et al. (Reference Corander, Waldmann and Sillanpää2003). Let p=(p1, p2, …, pR)′ be an RL×1 vector of allelic frequencies for all R groups, where pr=(p r,1, p r,2, …, p r,L)′ has order L×1. Under the mutual independence assumption, the likelihood conferred by the observed number of copies of alleles to the gene frequencies is

The maximum likelihood estimator of p r,l is r,l=n r,A l/2n r and its empirical variance is
. The maximum likelihood estimator is unbiased but unstable, and may take values at the boundaries of the parameter space in small samples.
In a Bayesian treatment, allelic frequencies are assigned a prior distribution that might be homogeneous or heterogeneous over populations, chromosomes or genomic regions (e.g. coding versus non-coding regions). For example, Holsinger (Reference Holsinger1999, Reference Holsinger, Clark and Gelfand2006) adopts a prior beta distribution, Beta (p l|a l, b l) (and interprets it as describing variation over populations) with parameters

and

Here θ is common to all loci (i.e. the hypothesis of neutrality) and x l is the mean allelic frequency at locus l (averaged over populations). Using properties of the beta distribution in the parametric definition of θ leads to

Then, the joint posterior distribution of all unknowns (allelic frequencies, θ and vector x={x l}) is

Holsinger (Reference Holsinger1999) took g(θ)=Beta(1, 2) distribution as prior for θ, and assumed that all x l were identically distributed according to the uniform process g(x l)=U(0, 1). Given θ and x, the allelic frequencies are conditionally independent with conditional posterior distributions:

where ELSE means all parameters other than p r,l and the data observed. However, the conditional posterior distributions of θ and x are not recognizable, so an elaborate sampling scheme, e.g., one based on Markov chain Monte Carlo (MCMC) methods, must be tailored. Holsinger (Reference Holsinger1999) found that inferences were insensitive with respect to the choices of beta and uniform prior distributions for θ and elements of x, respectively. However, it was assumed (as in a neutral model) that all loci share the same θ parameter. This produces a mutual borrowing of information among loci (shrinking p r,l towards a common value), but the procedures are not explicit with respect to the existence of heterogeneity over loci due to forces such as differential mutation or selective sweeps. As proposed by Beaumont & Balding (Reference Beaumont and Balding2004), one could estimate locus specific θ values and refer these estimates to the posterior distribution of θ under the homogeneity value. In this manner, outliers could be found with respect to the ‘neutral’ distribution, but this would not inform about the structure of any latent heterogeneity.
Here, an alternative approach is used. Jeffreys rule (Bernardo & Smith, Reference Bernardo and Smith1994; Sorensen & Gianola, Reference Sorensen and Gianola2002) is used to produce a reference prior, which is a distribution assigned to all loci in all populations. This reference prior distribution is minimally informative in a well defined sense (Bernardo and Smith, Reference Bernardo and Smith1994). Using Bayes theorem, the joint posterior density of all allelic frequencies is now

Thus, allelic frequencies at different loci are mutually independent, a posteriori, with p r,l following a beta distribution with parameters and
. Possible point estimates of allelic frequencies are the posterior mean

and the posterior mode

The variance of the posterior distribution of p r,l is

Even though a weakly informative prior is used, differences exist with respect to maximum likelihood. To illustrate this point, consider a hypothetical example with two groups, M and N. Suppose that 100 individuals are genotyped in group M and that the observed number of A l alleles is 199, i.e. the locus is nearly fixed. The maximum likelihood estimate of p M,l is 0·995 and its estimated standard error is 4·99×10−3; a calculation based on asymptotic normality (without truncation) yields that the probability of obtaining estimates larger than 1 is close to 0·16! Further, the probability of obtaining estimates between 0·9 and 0·995 is close to . On the other hand, the posterior distribution of p M,l is
. The posterior mean and posterior standard deviation are 0·993 (note some shrinkage away from the edge of the parameter space) and 6·06×10−3, respectively; the posterior probability of the frequency being larger than 1 is exactly zero, and the probability that p M,l takes values between 0·9 and 0·995 is about 0·57. Figure 1 displays the posterior distribution of the allelic frequency obtained with Jeffreys prior, overlaid against the normal approximation to the distribution of the maximum likelihood estimates. Clearly, the approach used makes a difference, even in a situation where allelic frequencies are estimated with reasonable precision, as indicated by the small standard error of the maximum likelihood estimate and the small posterior standard deviation in the Bayesian analysis (the coefficient of variation of the posterior distribution is less than 1%).

Fig. 1. Posterior density (thick line) of the allelic frequency p at a locus for which 199 copies have been observed out of 200 alleles counted in hypothetical population M; the posterior distribution is Beta. The thin line is the density of a normal approximation to the sampling distribution of the maximum likelihood estimator.
In the second population, N, 30 individuals are genotyped and 10 alleles are of the type A l; the maximum likelihood estimate of p N,l is then , much lower than in M, and its sampling variance is 2·31×10−3. The posterior distribution of p N,l is
. In N, the posterior density of p N,l and the normal approximation to the density of the distribution of the maximum likelihood estimator are very similar (not shown here).
Differences in allelic frequencies between populations M and N at the locus in question may be due to random drift or may suggest a signature of selection.
(ii) Inferring θ by maximum likelihood
A likelihood-based estimate of θ can be obtained by replacing in (3) or (4) the unknown allelic frequencies by their maximum likelihood estimates. For the example of populations M and N above, the estimate is

The sampling variance of the maximum likelihood estimator of θl can be approximated using a Taylor series expansion. As shown in Appendix A, the first derivative of θl with respect to the allelic frequency at locus l in group r is

for r=1,2, …, R; l=1,2, …, L, where is as before and
. Further, let

be an RL×1 vector of first derivatives evaluated at the maximum likelihood estimates of the allelic frequencies. Then, approximately

where is a diagonal matrix containing the estimates of the sampling variances of the maximum likelihood estimates of allelic frequencies p r,l. For the hypothetical example,
. The asymptotic normal approximation to the distribution of the estimates assigns nil probability to ‘estimates’ outside of (0,1); the probability of obtaining estimates of θ between 0·67 and 0·74 for this two-population situation is 0·9996.
(iii) Bayesian inference of θ
Consider now finding the posterior distribution of θl as defined in (4) and without making the assumption that the θs are realizations from the same stochastic process, i.e. without borrowing information across loci over and above the shrinkage of allelic frequencies produced by Jeffreys prior. The posterior distribution is analytically difficult to arrive at because θl is a non-linear function of gene frequencies in all R groups. However, since it is easy to obtain independent samples from each of the processes, Monte Carlo estimates of features of the posterior distribution of θl can be obtained without using MCMC methods at all. Let p r,l(s), s=1,2, …, S, be samples from the posterior (beta) distribution of p r,l, the frequency of allele A l at locus l. Then, a draw from the posterior distribution of θl is given by

which is a random variable with support in (0,1) (Holsinger, Reference Holsinger, Clark and Gelfand2006). Then, from S samples, the mean, median, variance, etc., of the posterior distribution of θl can be estimated. Each θl (l=1, 2, …, L) will have a point estimate and an assessment of uncertainty, e.g., a credibility interval of size 95% given by the 2·5% and 97·5% percentiles of the corresponding posterior distribution estimated either from samples or from the normal theory approximation given in Appendix B.
In the hypothetical populations M and N, the posterior distributions of the frequency of A l are Beta(199·5,1·5) and Beta(10·5,50·5), respectively. With draws denoted as B (s) (.,.), S samples from the posterior distribution of θl can be obtained as

To illustrate, 5000 samples were drawn from each of the two beta distributions, to form S=5000 corresponding draws from the posterior distribution of θl. The mean and median were 0·6966 and 0·6972, respectively; the standard deviation was 0·070 and the range of values samples spanned from 0·4268 to 0·8883. The posterior density of θl and the empirical cumulative distribution function are in Figs 2 and 3, respectively. Values of θl appearing with appreciable density range from about 0·5 to 0·9 (Fig. 2), with small posterior probability assigned to values smaller than 0·6 (Fig. 3).

Fig. 2. Posterior density of θl for the hypothetical example of populations M and N.

Fig. 3. Empirical cumulative distribution function of θl for the hypothetical example of populations M and N.
(iv) A Bayesian ‘null’ distribution for assessing sampling variation uncertainty
It is important to check whether or not posterior estimates of θl depart from what would be expected by chance alone. A posterior distribution consistent with expectations under a ‘null’ model is formulated next. The θl statistics calculated from the ‘full’ model above can then be referred to this null distribution. Note that the ‘null’ distribution given below describes the uncertainty to be expected from drawing random samples from the same population, but not the variability to be expected due to genetic drift. If estimates of θl fall in this null distribution, this would indicate that the study lacks power to answer evolutionary questions in any meaningful manner.
A ‘null’ distribution is arrived at by stating that p r , l=p l is the same random variable for all R populations. Under this assumption, the posterior distribution of the vector of gene frequencies (now of dimension L×1) under the ‘null’ model is
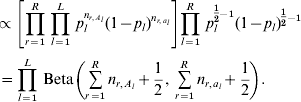
Hence, allelic frequencies p l are mutually independent, a posteriori, with p l|DATA, Null being a beta distribution with parameters and
A draw from the posterior distribution of the F ST statistic under this model takes the form

where p l(r,s) is a draw from , with R such draws involved in a realization of θl(s), and
is the average of the R draws. A set of samples from the posterior distribution of θl under the null model is obtained by repeating the sampling process S times. This distribution serves as a reference against which the θl statistics calculated from the ‘full’ model can be compared. If the posterior mean of θl obtained from the ‘full’ model falls outside of a high density area of the posterior distribution of θ in the null model, then the divergence between populations would be probably due to drift or selection (assuming mutation rates are constant over populations), but not due to chance alone.
For the example of populations M and N, and
. Figure 4 depicts the Beta(209·5,51·5) distribution of the allelic frequency under the ‘null’ model. Note that the maximum likelihood estimates of the allelic frequencies in the M and N populations, of 0·995 and
respectively, are not assigned any appreciable density under this model. Upon drawing 5000 independent samples from the beta distribution of the allelic frequency under the null model, 5000 draws for θl,Null(s) were obtained by evaluating (12) for each of the samples. Draws ranged from 8·24×10−13 to 0·0503; the mean (standard deviation) was 0·0038(0·0053) and the posterior median was 0·0017. The posterior density of θl,Null was very sharp as shown in Fig. 5. In the full model, the estimated posterior mean (standard deviation) of θl was 0·6966, which is unlikely to have been generated under the null distribution. This would make the locus a reasonable candidate for further examination.

Fig. 4. Posterior density of the allelic frequency p under the ‘null’ model for hypothetical populations M and N: 209 copies of A l are observed out of 260 alleles screened.

Fig. 5. Posterior density of θl under the null model for the hypothetical example of populations M and N.
(v) Illustration of sampling variation with candidate genes for type-II diabetes
The Bayesian method was applied to data pertaining to identification of candidate gene variants for type II diabetes in Polynesians (Myles et al., Reference Myles, Hradetzky, Engelken, Lao, Nürnberg, Trent, Wang, Kayser and Stoneking2007). Prevalence of this disease is high in several Pacific populations, e.g. 40% of adults living in the island of Nauru. DNA samples were obtained from 23 Polynesians, 23 New Guineans and 19 Han Chinese from Beijing. Type II diabetes-associated alleles were from 10 SNP loci having evidence of association. Estimated frequencies and θl statistics are shown in page 587 of Myles et al. (Reference Myles, Hradetzky, Engelken, Lao, Nürnberg, Trent, Wang, Kayser and Stoneking2007). To illustrate the Bayesian procedure, data for the KCNJ11 locus was used, and susceptibility allele frequencies (A l in our notation) were 0·30, 0·25 and 0·34 in the three populations above, respectively. Their figures do not lead to an integer number of alleles, due to rounding error, so the number of observed A l alleles used here was set to 14 (Polynesians), 12 (New Guineans) and 13 (Han Chinese). Myles et al. (Reference Myles, Hradetzky, Engelken, Lao, Nürnberg, Trent, Wang, Kayser and Stoneking2007) employed an ‘unbiased estimator’ of θl for calculating population pairwise differences, and their estimates were 0·003 (New Guinea–China), −0·024 (China–Polynesia) and −0·017 (New Guinea–Polynesia). Note the two negative estimates of a parameter that resides in (0,1); standard errors or significance levels were not provided. Their analysis suggests that this locus is not associated with prevalence of the disease.
The posterior distributions of A l were BetaPolynesians(14·5,32·5), BetaNewGuineans(12·5,34·5) and BetaHanChinese(13·5,25·5). The number of samples drawn from each of these 3 posterior distributions was S=1000, and 1000 draws from the posterior distribution of θKCNJ11 were obtained by evaluation of (10). Values of θKCNJ11 ranged from 2·423×10−5 to 0·1500, with an estimated posterior mean (standard deviation) of 0·019 (0·0193); this estimate is higher than that of Myles et al. (Reference Myles, Hradetzky, Engelken, Lao, Nürnberg, Trent, Wang, Kayser and Stoneking2007). The non-parametric estimate of the posterior density of θKCNJ11 is shown in Fig. 6, illustrating that the true value of the F ST parameter is very likely below 0·10. The posterior inter-correlation structure between allelic frequencies and θKCNJ11 in the full model was examined and, as expected, draws from the posterior distributions of allelic frequencies in the three populations were uncorrelated. Samples of θKCNJ11 were positively correlated (0·55) with those for allelic frequency in Chinese Han, and the 95% confidence interval for the correlation was 0·51–0·60. However, draws for θKCNJ11 were negatively correlated with allele frequencies in Polynesians (−0·07) and New Guineans (−0·39); the confidence intervals for these two correlations were (−0·13,−0·01) and (−0·44,−0·34), respectively.

Fig. 6. Density of the posterior distribution of θKCNJ11 obtained from allelic frequencies in Myles et al. (Reference Myles, Hradetzky, Engelken, Lao, Nürnberg, Trent, Wang, Kayser and Stoneking2007).
For the ‘null’ model, the 1000 samples from the posterior distribution of θKCNJ11,Null ranged from 3·62×10−6 to 0·1460, with the posterior mean (standard deviation) estimated at 0·002 (0·002); the posterior median was 0·002 as well. The posterior mean (standard deviation) estimate of θKCNJ11 under the ‘full’ model was 0·019, and it did not enter with high density in the ‘null’ model (not shown). Although variation in allelic frequency at locus KCNJ11 among the three populations departs from what would be expected from chance alone (statistical sampling), the observed θ value is very small. This may support the hypothesis that this locus may not be associated with differences in prevalence of type II diabetes, in agreement with Myles et al. (Reference Myles, Hradetzky, Engelken, Lao, Nürnberg, Trent, Wang, Kayser and Stoneking2007). Allelic frequencies were uncorrelated, as it should be, given that the three replicates were drawn from the same Beta(209·5,51·5) distribution. The θKCNJ11,Null statistic was uncorrelated with allelic frequencies, and the correlations were −0·08, −0·11 and 0·03 in the three replicates, with all confidence intervals including 0.
4. Clustering of θ-parameters
The second step of the procedure consists of clustering a set of estimates of θ values (in this case, posterior means) from a multi-locus analysis into data driven groups. The expectation is that these clusters might be representative of different processes taking place in the populations such as balancing or directional selection, neutrality or anything else.
The method is illustrated with data from a study of Petit et al. (Reference Petit, El Mousadik and Pons1998) in which alleles were sampled for 12 isozyme loci of the Argania genus tree in each of 12 areas (populations) of Morocco. The data, given in page 847 of Petit et al. (Reference Petit, El Mousadik and Pons1998), were modified as shown in Table 1. The modification consisted of treating all loci as bi-allelic by lumping alleles for loci with more than two variants into two classes. The number of individuals sampled per population ranged between 20 and 50, and the number of alleles per locus varied originally between 2 and 5. Note that, at some loci, one of the alleles was fixed in almost all populations. For example, for locus 3, the only population in which segregation was observed was TA.
Table 1. Allelic frequencies at 12 isozyme loci in each of 12 Argan tree populations, adapted from Petit et al. (Reference Petit, El Mousadik and Pons1998) by making all loci bi-allelic. A1–A12 represent frequencies of the ‘A’ allele at loci 1–12; No. A1–No. A12 are the observed number of copies of the alleles. The number of ‘a’ alleles can be calculated from the number of individuals samples and the number of ‘A’ alleles observed

For each locus, 2000 samples were drawn from the beta posterior distributions of allelic frequencies. For example, the posterior distribution of p AB , 1 was Beta(21·5,19·5). From these samples, 2000 draws from the posterior distribution of θ for each locus were formed as in (10). The posterior means were as follows:

so estimates of θ varied over loci from about 0·095 (locus 10) to 0·791 (locus 3); all these estimates did not enter into the corresponding ‘null’ distributions. Box plots of the posterior distributions of the θ parameters are in Fig. 7. Visually, it is tempting to suggest four clusters: the first one would include locus 3, with the posterior mean of θ close to 0·79; the second cluster would include locus 9, with an estimate of θ of 0·59. The third cluster would include loci 5, 7 and 8 with estimates ranging between 0·30 and 0·39, and the fourth cluster would be represented by loci 1, 2, 4, 6, 10, 11 and 12 having the lowest estimates of θ.

Fig. 7. Box plot of the posterior distributions of θ-parameters in 12 isozyme loci of the argan tree in Morocco (data originally from Petit et al. Reference Petit, El Mousadik and Pons1998).
The existence of an underlying structure is suggested by the distribution of all 24 000 samples, presented in Fig. 8. In the left panel, a non-parametric density estimate was obtained from these samples treated as if all draws (2000 for each of the 12 loci) had been made from the same process; the densities in the middle and right panels correspond to the logit, i.e., log(θ/(1−θ)), and Gompit, −log(−log(θ)), transforms of the sampled θ values, respectively. The three densities suggest that θ values cluster around 3, perhaps 4, modes.

Fig. 8. Non-parametric density estimates of θ values (based on 2000 sample for each of 12 loci), logit(θ) and Gompit(θ). All samples treated as homogeneous, i.e. as generated from the same stochastic process.
The structure was explored more formally by fitting a sequence of finite mixture models to the means of the posterior distribution of the θ values for each of the 12 loci. These posterior means are independent (under the assumptions made for the allelic frequency models), but not identically distributed, since they are estimated with different precision, due to unequal numbers of individuals sampled and varying allelic frequencies. The distributions of θ values among loci are not normal (the logit and Gompit transforms would be expected to be more nearly so). This should not be an issue because the mixture model was not used for testing hypotheses; its objective, rather, was to explore a clustered structure. Since there are only 12 posterior means, the mixture models must have less than 12 parameters; otherwise, a perfect fit would be obtained. The mixture model fitted to the posterior mean estimates postulated that

where K is the number of components of the mixture (clusters of posterior means of θ values or transforms thereof), πk is the probability that belongs to cluster k (subject to
), and μk and σk2 are the mean and variance, respectively, of component k. For example, if k=2, there are 5 ‘free’ parameters in the mixture; if k=4, there are 11 such parameters, so it is not sensible to fit a model with more than 4 components. Mixture model parameters were estimated by maximum likelihood via the expectation–maximization algorithm as implemented in the FlexMix package (Leisch, Reference Leisch2004) in the R project (R development core team, 2008). Upon convergence (assuming the stationary point was a global maximum), the conditional probability that
(or its transformation) belongs to cluster k is calculated as

The locus was assigned to the cluster with the largest conditional probability. Models with different values of k=1, 2, 3 and 4 were compared using Akaike's information criterion (AIC), that is

where p K is the number of parameters for a model with K components (McLachlan & Peel, Reference McLachlan and Peel2000). Models with the smallest AIC values are preferred. It is known that this criterion tends to overstate the number of components due to violation of regularity conditions in mixture models (Celeux & Soromenho, Reference Celeux and Soromenho1996).
Results of the mixture model analysis, by number of components fitted, are shown in Table 2. The AIC favoured a mixture with two clusters when the response was either θ or its Gompit transform, and a single component when the logit transformation was used. Clearly, with data from only 12 loci, the analyses did not have enough power to resolve heterogeneity in a finer manner. This would certainly not be the case with SNP data, where the number of marker loci typically oscillates between a few thousands in some animal species to close to a million in humans. Classification probabilities using K=2 and estimates of cluster mean and standard deviation are shown in Table 3. Irrespective of whether θ values were transformed or not, loci were clustered into two groups, one consisting of loci 3, 5, 7, 8 and 9, possibly reflecting a selection signature, and the other one including the remaining loci, presumably representing neutral loci. The maximum likelihood estimates of the mean and variance of θ values in the cluster with loci 3, 5, 7, 8 and 9 were 0·41±0·21, whereas the corresponding estimates in the other cluster were 0·12±0·03. This assignment into clusters is consistent with the picture emerging from visual consideration of the box plots in Fig. 7.
Table 2. Comparison of mixture models with 2, 3 or 4 components fitted to the 12 posterior means of θ-parameters and their logit or Gompit transforms in the argan tree data of Petit et al. (Reference Petit, El Mousadik and Pons1998). AIC (models with smallest values are favoured and indicated in boldface)

Table 3. Conditional probabilities of membership to one of two clusters for mixture models fitted to the posterior means of θ for the 12 loci in the argan tree, and their logit, log(θ/1−θ), and Gompit, −log(−log(θ)), transformations (boldfaced probability indicates the cluster with largest probability of membership)

The two-step procedure is simple and does not require the tailoring of problem-specific software. However, it has the drawback of not taking into account the uncertainty associated with the posterior distributions of the θ-parameters, inferred in the first step. In principle, a better approach is to feed the entire set of posterior samples to the clustering procedure, such that not only the location of the posterior distributions of the θs is considered but also their uncertainty as well. Although this is very appealing conceptually, it may create difficulties with the EM algorithm, leading to convergence failure. For instance Qanbari et al. (personal communication) employed the procedure with posterior means (each calculated with 1 million samples from the corresponding posterior distribution) with about 35 000 SNPs in Hereford and Simmental cattle. When posterior means were used as data, the mixture model approach revealed the existence of 4–5 clusters. However, when the 35 million samples were used as data points, the Expectation–Maximization (EM) algorithm, as implemented in FlexMix, failed to converge. An alternative to using the entire collection of samples is to feed a selected set of percentiles of the posterior distribution of each θl, so that a proxy for the dispersion of the individual posterior distributions enters into the analysis.
5. Discussion
The use of F-statistics for the study of genetic divergence between population dates back to Wright (Reference Wright1931). Holsinger & Weir (Reference Holsinger and Weir2009) have provided a justification for their usefulness, e.g., in association mapping and in detecting genomic regions affected by evolutionary processes, such as selection. These authors also reviewed different types of statistical methods for inferring F ST, including Bayesian procedures. Method of moments estimation was prompted by the linear model formalism of Cockerham (Reference Cockerham1969, Reference Cockerham1973), and a review is in Weir & Hill (Reference Weir and Hill2002). There has been an increased interest in Bayesian methods, and important contributions in this front have been made by Holsinger (Reference Holsinger1999, Reference Holsinger, Clark and Gelfand2006), Beaumont & Balding (Reference Beaumont and Balding2004) and Guo et al. (Reference Guo, Dey and Holsinger2009).
In the Bayesian approaches that have been suggested, e.g., Holsinger (Reference Holsinger1999), the model poses a product binomial (or product multinomial in the case of multiple alleles) likelihood function for allelic frequencies, with conjugate prior distributions, such as beta or Dirichlet processes. Marginalizing over the allelic frequencies yields the beta binomial or Dirichlet-multinomial distributions used by Balding (Reference Balding2003) for likelihood-based inference. Holsinger (Reference Holsinger1999) matched the mean and variance of, e.g., the beta distribution, to the definition of θ, and obtained a joint posterior distribution which is a function of the unknown allelic frequencies, of θ (assumed exchangeable over all loci) and of the mean allelic frequencies in an undivided population. The implementation, as well as those of Beaumont & Balding (Reference Beaumont and Balding2004) and of Guo et al. (Reference Guo, Dey and Holsinger2009) requires MCMC. While the power and flexibility of hierarchical models coupled with MCMC are well known (Sorensen & Gianola, Reference Sorensen and Gianola2002), implementations are not trivial and monitoring of convergence to the equilibrium distribution is a delicate matter (Cowles & Carlin, Reference Cowles and Carlin1996). The idea in these methods is that, under a neutral model, all θ (over loci) should be realizations of the same stochastic process. Outlying θ values may be suggestive of genomic regions affected by selection. Typically, it is argued that loci are either neutral, subject to balancing selection or to directional selection favouring alleles in specific environments, e.g. Akey et al. (Reference Akey, Zhang, Zhang, Jin and Shriver2002). However, the assignment of loci to specific types of processes is often arbitrary.
The present paper follows ideas of Holsinger (Reference Holsinger1999), but it differs in two important respects. The proposed method has two steps. First, allelic frequencies are assigned a non-informative prior, so that the mutual borrowing of information between loci is limited, leading to less shrinkage of frequencies towards a common value; in maximum likelihood there is no shrinkage at all, an issue criticized by Haldane (Reference Haldane1948). Samples of allelic frequencies can be obtained directly (actually, their posterior distributions are tractable, analytically), and these draws are used to form draws from the posterior distribution of locus-specific θ-parameters, using the parametric definition of F ST as a function of allelic frequencies. The first step was illustrated with hypothetical data and with type II diabetes data in Myles et al. (Reference Myles, Hradetzky, Engelken, Lao, Nürnberg, Trent, Wang, Kayser and Stoneking2007). The step leads to estimates of the posterior distribution of the θs, which can be used to explore underlying structure, presumably caused by different evolutionary forces. In the second step, the structure is explored by using features of the posterior distribution of the θs (posterior means or transformations thereof) as response variables in a mixture model. Data from Petit et al. (Reference Petit, El Mousadik and Pons1998) on 12 isozyme loci in 12 populations of the argan tree in Morocco were used to illustrate the second step. Here, the posterior means of θ are treated as belonging to a mixture of normal distributions, which is then resolved into data-supported components. Since the final objective is that of clustering loci according to their similarity in θ values, departures from normality are arguably of little consequence. Here, logit and Gompit transformations were examined, and the clustering procedure produced exactly the same results. Using AIC as a gauge for model comparison, it was suggested that the 12 estimates of θ clustered into two groups, one representing putatively neutral loci (provided that this group reflects variation due to drift), and another one possibly corresponding to genomic regions affected by selection. With 12 loci only, it is unreasonable to expect a finer clustering structure. An ongoing study is applying the two-step procedure to large-scale SNP data in an animal population and this will be reported in a future communication.
As mentioned earlier in the paper, the two-step procedure has the disadvantage of not incorporating the uncertainty about the posterior distributions inferred in the first step. Although this can be remedied by using all posterior samples as input into the mixture model analysis, it can create numerical difficulties with the EM algorithm. This is an issue that needs additional research.
The method proposed here extends naturally to multiple alleles. In this case the likelihood is product multinomial, and the beta prior distribution is replaced by a Dirichlet distribution with minimum information content. The posterior distribution of the allelic frequencies is product Dirichlet, which is simple to sample from. Then, samples from the posterior distribution of θl would be drawn by evaluation of formulae similar to those in Nei (Reference Nei1973) where θ-parameters are averaged over alleles. For example, one could define

where p r,l,m is the frequency of allele m at locus l in population r and is the unweighted average over the R populations.
In common with the studies of Holsinger (Reference Holsinger1999), Beaumont & Balding (Reference Beaumont and Balding2004), Weir et al. (Reference Weir, Cardon, Anderson, Nielsen and Hill2005) and Guo et al. (Reference Guo, Dey and Holsinger2009), the procedure presented here assumes that allelic frequencies are in linkage equilibrium, so that the likelihood of all allelic frequencies is either product binomial or product multinomial. Accommodating linkage disequilibrium, especially with dense batteries of marker loci, represents a formidable task and it is a challenge for future research. For example, Akey et al. (Reference Akey, Zhang, Zhang, Jin and Shriver2002) and Weir et al. (Reference Weir, Cardon, Anderson, Nielsen and Hill2005) reported that θ values of loci in regions of high linkage disequilibrium were similar. Guo et al. (Reference Guo, Dey and Holsinger2009) address correlations due to linkage, but not due to linkage disequilibrium, and do so by introducing a spatial structure for loci located in the same chromosome. Specifically, they proposed an autoregressive model in which logit transforms of θ values are correlated according to physical distance. The model is quite involved and requires MCMC computations. However, loci may be in linkage disequilibrium even though not being physically linked (Crow & Kimura, Reference Crow and Kimura1970), and such disequilibrium is very common in animal populations (Sandor et al., Reference Sandor, Farnir, Hansoul, Coppieters, Meuwissen and Georges2006; de Roos et al., Reference de Roos, Hayes, Spelman and Goddard2008; Lipkin et al., Reference Lipkin, Straus, Stein, Bagnato, Schiavini, Fontanesi, Russo, Medugorac, Foerster, Sölkner, Dolezal, Medrano, Friedmann and Soller2009; Qanbari et al., Reference Qanbari, Pimentel, Tetens, Thaller, Lichtner, Sharifi and Simianer2009), where finite size and selection under epistasis are factors in building up linkage disequilibrium. The two-step approach considered here could be enhanced by exploring algorithms alternative to EM as well as by consideration of different types of mixtures, e.g., of beta distributions, which are more appropriate for random variables taking values in (0,1).
Part of this work was carried out while the senior author was a Visiting Professor at Georg-August-Universität, Göttingen (Alexander von Humboldt Foundation Senior Researcher Award). Support by the Wisconsin Agriculture Experiment Station, and by grant NSF DMS-NSF DMS-044371 is acknowledged. This study is part of the project FUGATO-plus GenoTrack and was financially supported by the German Ministry of Education and Research, BMBF, the Föderverein Biotechnologieforschung e.V. (FBF), Bonn, and Lohmann Tierzucht GmbH, Cuxhaven. S. Qanbari thanks the H. Wilhelm Schaumann Stiftung Hamburg for financial support. Professor W. G. Hill and three anonymous reviewers are thanked for useful comments.
Appendix A: First derivatives of θ with respect to allelic frequencies
Let From (4), the derivative is

Appendix B: Approximate Bayesian analysis
An approximate Bayesian analysis without sampling from the posterior distribution is also possible. An approximation to the mean and variance of the posterior distribution of θl can be obtained using a Taylor series expansion about the modes of the allelic frequencies. Let now
be an R×1 vector of first derivatives evaluated at the posterior mode estimates (8) of the allelic frequencies. Then, approximately

where is the vector of posterior mode estimates of allele frequencies in the R groups. Then, approximately
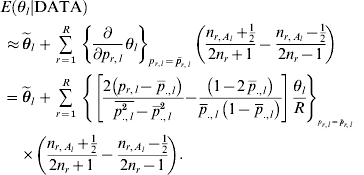
Likewise

Since allelic frequencies have mutually independent distributions, the R×R variance–covariance matrix Var (pl|DATA) is diagonal with elements given by (9). Thus

In short, each θl (l=1, 2, …, L) statistic will have a point estimate and an assessment of uncertainty, e.g., a credibility interval of size 95% given by the 2·5% and 97·5% percentiles of the corresponding posterior distribution estimated from samples, or from using a normal theory approximation, e.g.,
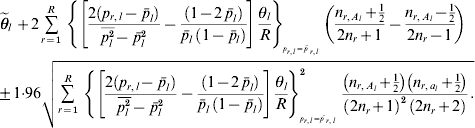